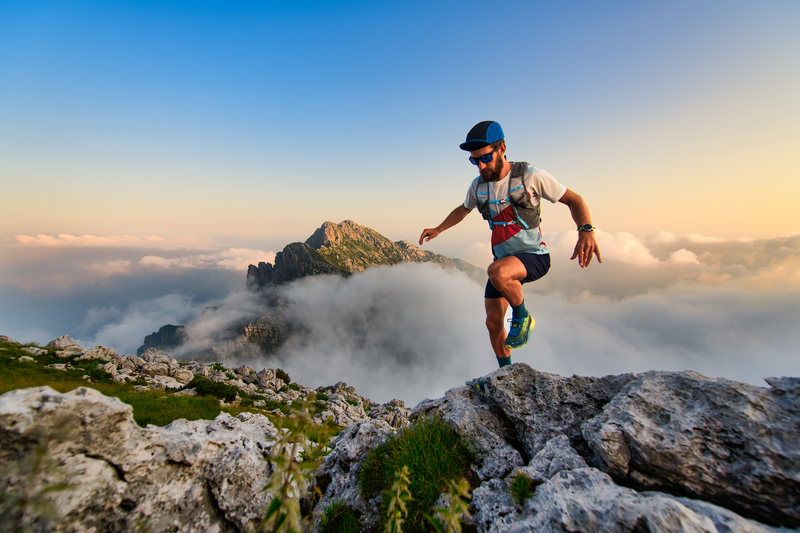
94% of researchers rate our articles as excellent or good
Learn more about the work of our research integrity team to safeguard the quality of each article we publish.
Find out more
ORIGINAL RESEARCH article
Front. Med.
Sec. Translational Medicine
Volume 12 - 2025 | doi: 10.3389/fmed.2025.1547588
The final, formatted version of the article will be published soon.
You have multiple emails registered with Frontiers:
Please enter your email address:
If you already have an account, please login
You don't have a Frontiers account ? You can register here
Background: Early diagnosis of nonunion fractures is vital for treatment planning, yet studies using bone morphometric parameters for this purpose are scarce. This study aims to create a fracture micro-CT image dataset, design a deep learning algorithm for fracture segmentation, and develop an early diagnosis model for fracture nonunion.Methods: Using fracture animal models, micro-CT images from 12 rats at various healing stages (days 1, 7, 14, 21, 28, and 35) were analyzed. Fracture lesion frames were annotated to create a high-resolution dataset. We proposed the Vision Mamba Triplet Attention and Edge Feature Decoupling Module UNet (VM-TE-UNet) for fracture area segmentation. And we extracted bone morphometric parameters to establish an early diagnostic evaluation system for the nonunion of fractures.Results: A dataset comprising 2,448 micro-CT images of the rat fracture lesions with fracture Region of Interest (ROI), bone callus and healing characteristics was established and used to train and test the proposed VM-TE-UNet which achieved a Dice Similarity Coefficient of 0.809, an improvement over the baseline's 0.765, and reduced the 95th Hausdorff Distance to 13.1. Through ablation studies, comparative experiments, and result analysis, the algorithm's effectiveness and superiority were validated. Significant differences (p<0.05) were observed between the fracture and fracture nonunion groups during the inflammatory and repair phases. Key indices, such as the average CT values of hematoma and cartilage tissues, BS/TS and BS/TV of mineralized cartilage, BS/TV of osteogenic tissue, and BV/TV of osteogenic tissue, align with clinical methods for diagnosing fracture nonunion by assessing callus presence and local soft tissue swelling. On day 14, the early diagnosis model achieved an AUC of 0.995, demonstrating its ability to diagnose fracture nonunion during the soft-callus phase.Conclusion: This study proposed the VM-TE-UNet for fracture areas segmentation, extracted micro-CT indices, and established an early diagnostic model for fracture nonunion. We believe that the prediction model can effectively screen out samples of poor fracture rehabilitation caused by blood supply limitations in rats 14 days after fracture, rather than the widely accepted 35 or 40 days. This provides important reference for the clinical prediction of fracture nonunion and early intervention treatment.
Keywords: micro-CT, Fracture nonunion prediction, Bone morphometric parameters, deep learning, Mamba network
Received: 18 Dec 2024; Accepted: 07 Mar 2025.
Copyright: © 2025 Yu, Mu, Wang, Guo, Zhao, Wang, Wang, Meng, Dong, Wang and Sun. This is an open-access article distributed under the terms of the Creative Commons Attribution License (CC BY). The use, distribution or reproduction in other forums is permitted, provided the original author(s) or licensor are credited and that the original publication in this journal is cited, in accordance with accepted academic practice. No use, distribution or reproduction is permitted which does not comply with these terms.
* Correspondence:
Shuo Wang, Department of Biomedical Engineering, Tianjin University School of Medicine, Tianjin, China
Jinglai Sun, Haihe Laboratory of Brain-computer Interaction and Human-machine Integration, Tianjin University School of Medicine, Tianjin, China
Disclaimer: All claims expressed in this article are solely those of the authors and do not necessarily represent those of their affiliated organizations, or those of the publisher, the editors and the reviewers. Any product that may be evaluated in this article or claim that may be made by its manufacturer is not guaranteed or endorsed by the publisher.
Research integrity at Frontiers
Learn more about the work of our research integrity team to safeguard the quality of each article we publish.