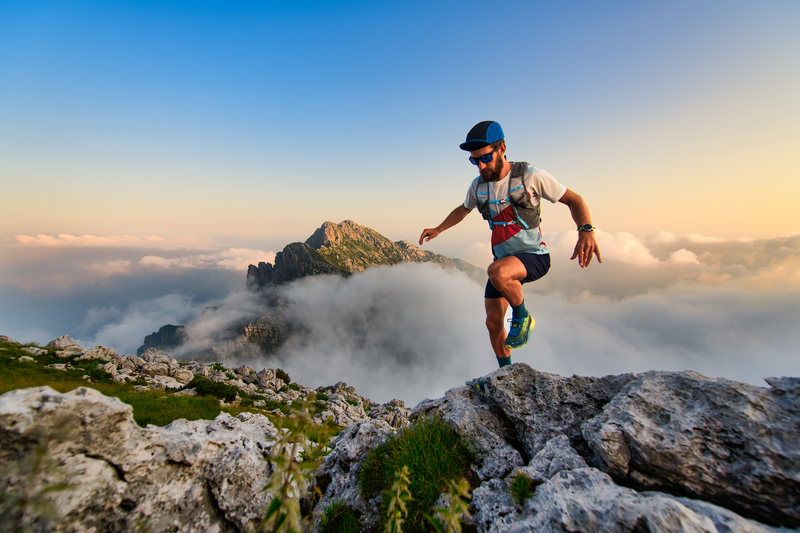
94% of researchers rate our articles as excellent or good
Learn more about the work of our research integrity team to safeguard the quality of each article we publish.
Find out more
ORIGINAL RESEARCH article
Front. Med.
Sec. Pulmonary Medicine
Volume 12 - 2025 | doi: 10.3389/fmed.2025.1545095
The final, formatted version of the article will be published soon.
You have multiple emails registered with Frontiers:
Please enter your email address:
If you already have an account, please login
You don't have a Frontiers account ? You can register here
Objective: This study aims to systematically identify differential gene expression profiles in patients with allergic asthma through whole-transcriptome sequencing and validate the role of these genes in asthma pathogenesis, thereby uncovering potential molecular mechanisms.Methods: This study recruited a cohort of eighty individuals diagnosed with allergic asthma and forty healthy controls. RNA was extracted from both peripheral blood and airway samples, and sequencing was performed using the Illumina NovaSeq 6000 platform. Potential differential genes were confirmed through three independent techniques to validate the findings: quantitative reverse transcription polymerase chain reaction (qRT-PCR), immunohistochemistry, and Western blot analysis. Differential gene expression was analyzed using DESeq2 software, providing a rigorous statistical framework for RNA-Seq data interpretation. Gene Ontology (GO) enrichment analysis and Kyoto Encyclopedia of Genes and Genomes (KEGG) pathway analysis were employed to Conclusion: Differential gene expression profiles play a crucial role in the pathogenesis of asthma.This study provides important evidence for understanding the molecular mechanisms of asthma and developing novel targeted therapeutic strategies.
Keywords: Allergic Asthma, whole-transcriptome sequencing, Differentially expressed genes, Functional enrichment analysis, Asthma pathogenesis
Received: 14 Dec 2024; Accepted: 21 Mar 2025.
Copyright: © 2025 Cui, Liu, Wang, He and Xu. This is an open-access article distributed under the terms of the Creative Commons Attribution License (CC BY). The use, distribution or reproduction in other forums is permitted, provided the original author(s) or licensor are credited and that the original publication in this journal is cited, in accordance with accepted academic practice. No use, distribution or reproduction is permitted which does not comply with these terms.
* Correspondence:
Yanxia Xu, Yanan University Affiliated Hospital, Yanan, China
Disclaimer: All claims expressed in this article are solely those of the authors and do not necessarily represent those of their affiliated organizations, or those of the publisher, the editors and the reviewers. Any product that may be evaluated in this article or claim that may be made by its manufacturer is not guaranteed or endorsed by the publisher.
Research integrity at Frontiers
Learn more about the work of our research integrity team to safeguard the quality of each article we publish.