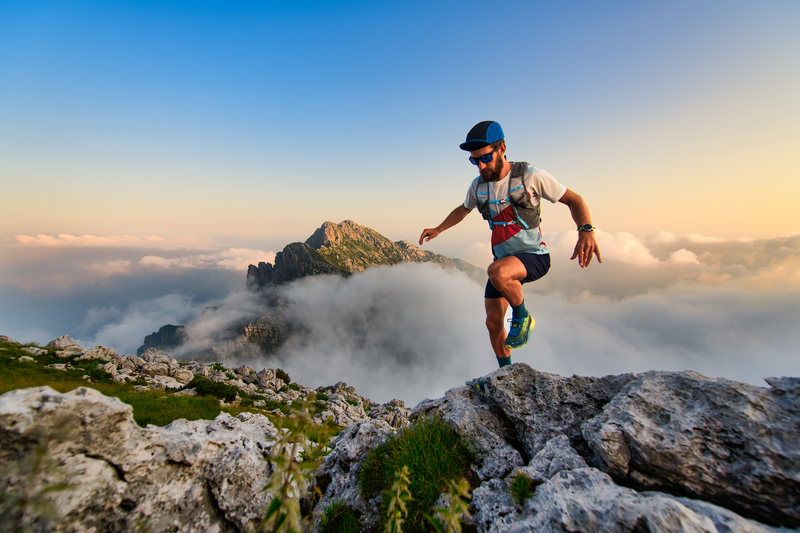
94% of researchers rate our articles as excellent or good
Learn more about the work of our research integrity team to safeguard the quality of each article we publish.
Find out more
ORIGINAL RESEARCH article
Front. Med.
Sec. Geriatric Medicine
Volume 12 - 2025 | doi: 10.3389/fmed.2025.1533507
The final, formatted version of the article will be published soon.
You have multiple emails registered with Frontiers:
Please enter your email address:
If you already have an account, please login
You don't have a Frontiers account ? You can register here
Background: Biological age (BA) is regarded as a more accurate marker of aging than chronological age and is commonly used to assess associations with age-related diseases. The relationship between BA measures and the colorectal cancer (CRC) has not yet been investigated.Methods: This study utilized data from the National Health and Nutrition Examination Survey. BA was quantified using the Klemera-Doubal Method (KDMAge) and Phenotypic Age (PhenoAge), based on 13 common clinical biomarkers. The prevalence of CRC across quartiles of BA indicators was compared using weighted chi-square tests. Weighted multivariable logistic regression models were used to assess the association between BA indicators and CRC.Results: A total of 36,684 participants were included. The weighted prevalence of CRC showed a significant and consistent upward trend across ascending quartiles of chronological age, KDMAge, and PhenoAge, even within gender and age subgroups (all P for trend < 0.05). In the total population and gender subgroups, higher quartiles of PhenoAge acceleration showed a higher weighted prevalence of CRC compared to lower quartiles (P for trend < 0.05). Accelerated PhenoAge was significantly associated with a higher prevalence of CRC (OR = 1.767, 95% CI: 1.236-2.524, p = 0.002). However, accelerated PhenoAge was associated with the increased prevalence of CRC only in individuals older than 65 years (OR = 1.655, 95% CI: 1.143-2.397, p = 0.008).Biological aging are positively associated with the prevalence of CRC regardless of gender, particularly among the elderly.
Keywords: biological age, KDMAge, PhenoAge, colorectal cancer, Elderly
Received: 24 Nov 2024; Accepted: 11 Feb 2025.
Copyright: © 2025 Wang, Wang and Wang. This is an open-access article distributed under the terms of the Creative Commons Attribution License (CC BY). The use, distribution or reproduction in other forums is permitted, provided the original author(s) or licensor are credited and that the original publication in this journal is cited, in accordance with accepted academic practice. No use, distribution or reproduction is permitted which does not comply with these terms.
* Correspondence:
Xiu Wang, Suqian First People's Hospital, Suqian, China
Disclaimer: All claims expressed in this article are solely those of the authors and do not necessarily represent those of their affiliated organizations, or those of the publisher, the editors and the reviewers. Any product that may be evaluated in this article or claim that may be made by its manufacturer is not guaranteed or endorsed by the publisher.
Research integrity at Frontiers
Learn more about the work of our research integrity team to safeguard the quality of each article we publish.