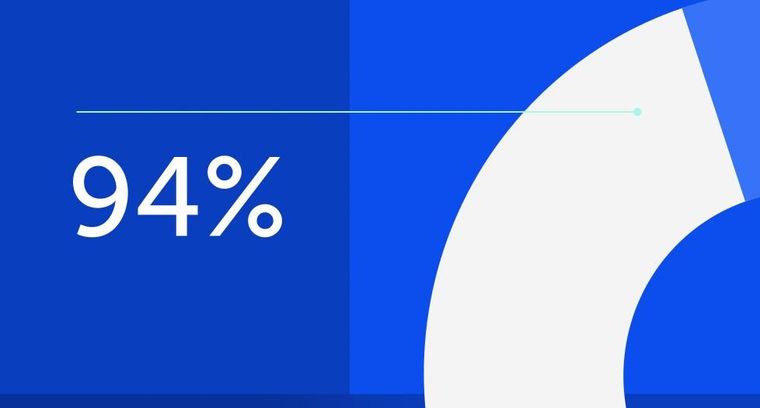
94% of researchers rate our articles as excellent or good
Learn more about the work of our research integrity team to safeguard the quality of each article we publish.
Find out more
ORIGINAL RESEARCH article
Front. Med.
Sec. Hepatobiliary Diseases
Volume 12 - 2025 | doi: 10.3389/fmed.2025.1533132
This article is part of the Research TopicAdvancing Personalized Cancer Care: Novel Genetic and Statistical ApproachesView all 6 articles
The final, formatted version of the article will be published soon.
Select one of your emails
You have multiple emails registered with Frontiers:
Notify me on publication
Please enter your email address:
If you already have an account, please login
You don't have a Frontiers account ? You can register here
Liver metastasis is the most common site of metastasis in pancreatic neuroendocrine tumors (PaNETs), significantly affecting patient prognosis. This study aims to develop machine learning algorithms to predict liver metastasis in PaNETs patients, assisting clinicians in the personalized clinical decision-making for treatment.We collected data on eligible PaNETs patients from the Surveillance, Epidemiology, and End Results (SEER) database for the period from 2010 to 2021. The Boruta algorithm and the Least Absolute Shrinkage and Selection Operator (LASSO) were used for feature selection. We applied 10 different machine learning algorithms to develop models for predicting the risk of liver metastasis in PaNETs patients. The model's performance was assessed using a variety of metrics, including the area under the receiver operating characteristic curve (AUC), the area under the precision-recall curve (AUPRC), decision curve analysis (DCA), calibration curves, accuracy, sensitivity, specificity, F1 score, and Kappa score. The SHapley Additive exPlanations (SHAP) were employed to interpret models, and the best-performing model was used to develop a web-based calculator.The study included a cohort of 7,463 PaNETs patients, of whom 1,356 (18.2%) were diagnosed with liver metastasis at the time of initial diagnosis. Through the combined use of the Boruta and LASSO methods, T-stage, N-stage, tumor size, grade, surgery, lymphadenectomy, chemotherapy, and bone metastasis were identified as independent risk factors for liver metastasis in PaNETs.Compared to other machine learning algorithms, the gradient boosting machine (GBM) model exhibited superior performance, achieving an AUC of 0.937 (95% CI: 0.931-0.943), an AUPRC of 0.94, and an accuracy of 0.87. DCA and calibration curve analyses demonstrate that the GBM model provides better clinical decision-making capabilities and predictive performance. Furthermore, the SHAP framework revealed that surgery, N-stage, and T-stage are the primary decision factors influencing the machine learning model's predictions. Finally, based on the GBM algorithm, we developed an accessible web-based calculator to predict the risk of liver metastasis in PaNETs.The GBM model excels in predicting the risk of liver metastasis in PaNETs patients, outperforming other machine learning models and providing critical support for developing personalized medical strategies in clinical practice.
Keywords: Pancreatic neuroendocrine tumors, liver metastasis, machine learning, prediction, Surveillance Epidemiology and End Results (SEER) database
Received: 23 Nov 2024; Accepted: 16 Apr 2025.
Copyright: © 2025 Jinzhe and YAQUN. This is an open-access article distributed under the terms of the Creative Commons Attribution License (CC BY). The use, distribution or reproduction in other forums is permitted, provided the original author(s) or licensor are credited and that the original publication in this journal is cited, in accordance with accepted academic practice. No use, distribution or reproduction is permitted which does not comply with these terms.
* Correspondence: YU YAQUN, Affiliated Hospital of Guilin Medical University, Guilin, Guangxi Zhuang Region, China
Disclaimer: All claims expressed in this article are solely those of the authors and do not necessarily represent those of their affiliated organizations, or those of the publisher, the editors and the reviewers. Any product that may be evaluated in this article or claim that may be made by its manufacturer is not guaranteed or endorsed by the publisher.
Supplementary Material
Research integrity at Frontiers
Learn more about the work of our research integrity team to safeguard the quality of each article we publish.