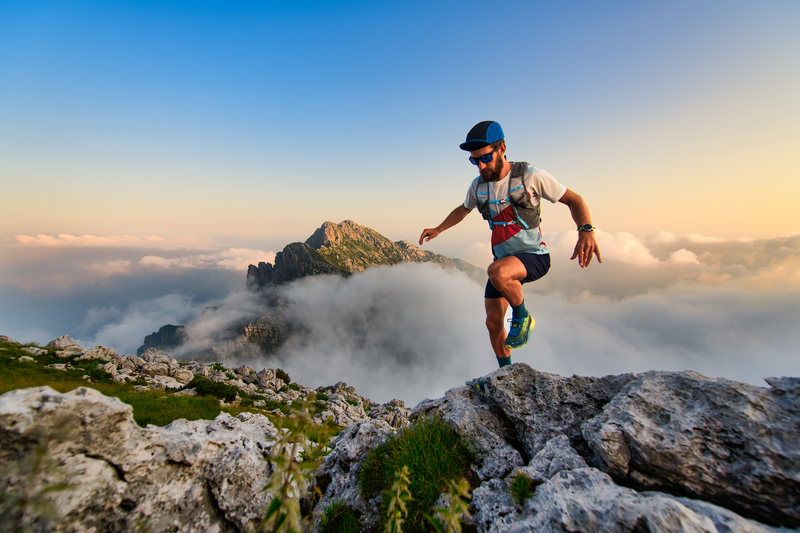
95% of researchers rate our articles as excellent or good
Learn more about the work of our research integrity team to safeguard the quality of each article we publish.
Find out more
ORIGINAL RESEARCH article
Front. Med.
Sec. Intensive Care Medicine and Anesthesiology
Volume 12 - 2025 | doi: 10.3389/fmed.2025.1531094
The final, formatted version of the article will be published soon.
You have multiple emails registered with Frontiers:
Please enter your email address:
If you already have an account, please login
You don't have a Frontiers account ? You can register here
Reintubation is an adverse postoperative complication in patients with Type A aortic dissection (AAD) that correlates to poor outcomes. This study aims to employ machine learning algorithms to establish a practical platform for the prediction of reintubation.Methods: A total of 861 patients diagnosed with AAD and undergoing surgical procedures, 688 patients as training and testing cohort from a single center, and 173 patients as validation cohort from four centers were enrolled. The least absolute shrinkage and selection operator (LASSO) was used for screening risk variables associated with reintubation for subsequent model construction. Subsequently, seven machine-learning models were built. The model with the best discrimination and calibration performance was used to predict reintubation. Finally, the SHapley Additive exPlanation (SHAP) was employed to explain the prediction model.Reintubation was performed in 107 patients (12.43%). The LASSO analysis identified readmission to the intensive care unit (ICU), continuous renal replacement therapy, length of stay in the ICU, and duration of invasive mechanical ventilation as significant risk factors for reintubation. The XGBoost model was selected as the final prediction model due to its better performance than other models, with the AUC, sensitivity, and specificity of 0.969, 0.8889, and 0.8611 in the testing cohort.SHAP values demonstrated the effects of individual features on the overall model. Finally, a web calculator was developed based on XGBoost model the for clinical use.We have developed and validated a high-performing risk prediction model for postoperative reintubation in patients with AAD. It can provide valuable guidance to clinicians in predicting reintubation and in developing timely preventative measures.
Keywords: Type a aortic dissection, Reintubation, machine learning, predictive model, SHAP value
Received: 08 Jan 2025; Accepted: 01 Apr 2025.
Copyright: © 2025 Wen, Zhang, Zhang, Zhou, Xu, Xie, Zhang, Zeng, Wu, Qiao, Hu and Dong. This is an open-access article distributed under the terms of the Creative Commons Attribution License (CC BY). The use, distribution or reproduction in other forums is permitted, provided the original author(s) or licensor are credited and that the original publication in this journal is cited, in accordance with accepted academic practice. No use, distribution or reproduction is permitted which does not comply with these terms.
* Correspondence:
Xingjian Hu, Department of Cardiovascular Surgery, Union Hospital, Tongji Medical College, Huazhong University of Science and Technology, Wuhan, China
Nianguo Dong, Department of Cardiovascular Surgery, Union Hospital, Tongji Medical College, Huazhong University of Science and Technology, Wuhan, China
Disclaimer: All claims expressed in this article are solely those of the authors and do not necessarily represent those of their affiliated organizations, or those of the publisher, the editors and the reviewers. Any product that may be evaluated in this article or claim that may be made by its manufacturer is not guaranteed or endorsed by the publisher.
Research integrity at Frontiers
Learn more about the work of our research integrity team to safeguard the quality of each article we publish.