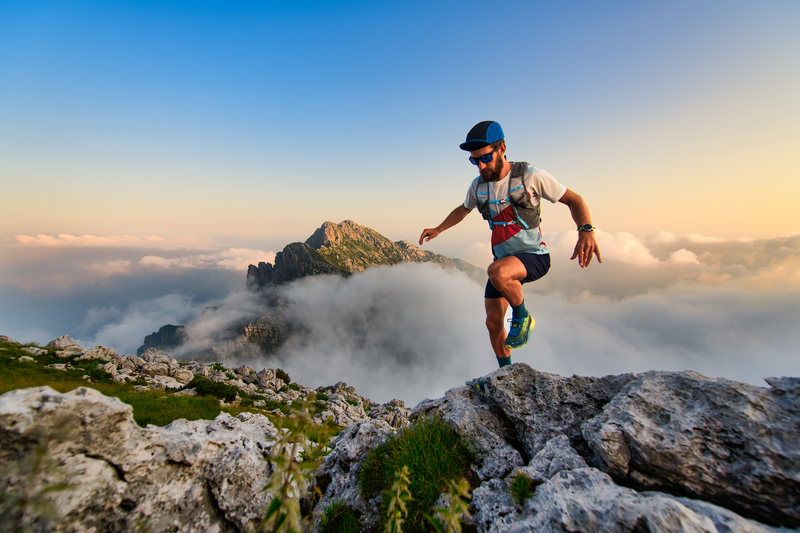
95% of researchers rate our articles as excellent or good
Learn more about the work of our research integrity team to safeguard the quality of each article we publish.
Find out more
ORIGINAL RESEARCH article
Front. Med.
Sec. Precision Medicine
Volume 12 - 2025 | doi: 10.3389/fmed.2025.1526144
This article is part of the Research Topic Application of Deep Learning in Biomedical Image Processing View all 3 articles
The final, formatted version of the article will be published soon.
You have multiple emails registered with Frontiers:
Please enter your email address:
If you already have an account, please login
You don't have a Frontiers account ? You can register here
Objective: This research aimed to create a dataset of Iliac CTV scans for automated May-Thurner Syndrome (MTS) detection using deep learning and radiomics. In addition, it sought to establish an automated segmentation model for Iliac Vein CTV scans and construct a radiomic signature for MTS diagnosis.We collected a dataset of 490 cases meeting specific inclusion and exclusion criteria, anonymized to comply with HIPAA regulations. Iliac Vein CTV scans were prepared with contrast agent administration, followed by image acquisition and evaluation. A deep learningbased segmentation model, UPerNet, was employed using ten-fold cross-validation. Radiomic features were extracted from the scans and used to construct a diagnostic radiomic signature.Dice values and ROC analysis, was conducted to evaluate segmentation and diagnostic performance. Results: The dataset consisted of 201 positive cases of May-Thurner Syndrome (MTS) and 289 negative cases. The UPerNet segmentation model exhibited remarkable accuracy in identifying MTS regions. A Dice coefficient of 0.925 (95% confidence interval: 0.875-0.961) was observed, indicating the precision and reliability of our segmentation model. Radiomic analysis produced a diagnostic radiomic signature with significant clinical potential. ROC analysis demonstrated promising results, underscoring the efficacy of the developed model in distinguishing MTS cases. The radiomic signature demonstrated strong diagnostic capabilities for MTS. Within the training dataset, it attained a notable area under the curve (AUC) of 0.891, with a 95% confidence interval ranging from 0.825 to 0.956, showcasing its effectiveness. This diagnostic capability extended to the validation dataset, where the AUC remained strong at 0.892 (95% confidence interval: 0.793-0.991). These results highlight the accuracy of our segmentation model and the diagnostic value of our radiomic signature in identifying May-Thurner Syndrome (MTS) cases. Conclusion: This study presents a comprehensive approach to automate MTS detection from Iliac CTV scans, combining deep learning and radiomics. The results suggest the potential clinical utility of the developed model in diagnosing MTS, offering a non-invasive and efficient alternative to traditional methods.
Keywords: May-Thurner Syndrome, deep learning, Computed Tomography Venography, Iliac vein compression, Convolutional Neural Networks
Received: 11 Nov 2024; Accepted: 24 Mar 2025.
Copyright: © 2025 Chen, Li, Zheng and Qiu. This is an open-access article distributed under the terms of the Creative Commons Attribution License (CC BY). The use, distribution or reproduction in other forums is permitted, provided the original author(s) or licensor are credited and that the original publication in this journal is cited, in accordance with accepted academic practice. No use, distribution or reproduction is permitted which does not comply with these terms.
* Correspondence:
Chengzhi Qiu, The Second Affiliated Hospital of Fujian Medical University, Quanzhou, China
Disclaimer: All claims expressed in this article are solely those of the authors and do not necessarily represent those of their affiliated organizations, or those of the publisher, the editors and the reviewers. Any product that may be evaluated in this article or claim that may be made by its manufacturer is not guaranteed or endorsed by the publisher.
Research integrity at Frontiers
Learn more about the work of our research integrity team to safeguard the quality of each article we publish.