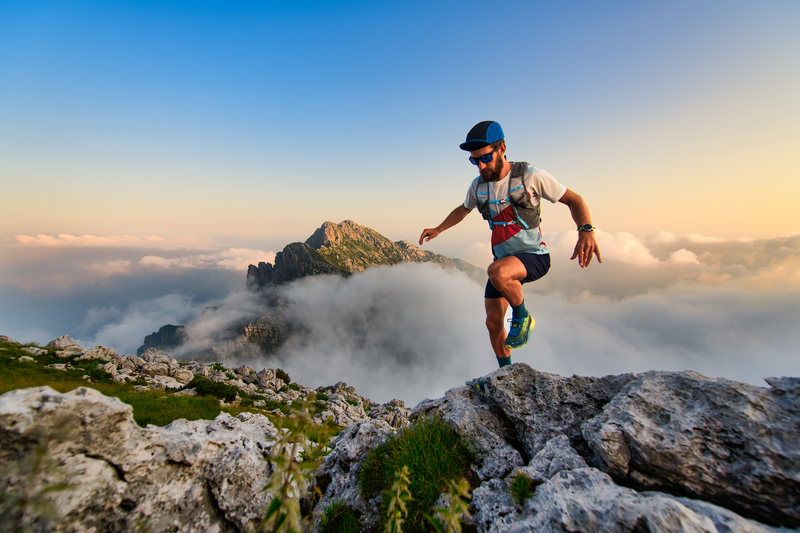
94% of researchers rate our articles as excellent or good
Learn more about the work of our research integrity team to safeguard the quality of each article we publish.
Find out more
ORIGINAL RESEARCH article
Front. Med.
Sec. Pulmonary Medicine
Volume 12 - 2025 | doi: 10.3389/fmed.2025.1525100
The final, formatted version of the article will be published soon.
You have multiple emails registered with Frontiers:
Please enter your email address:
If you already have an account, please login
You don't have a Frontiers account ? You can register here
Background: Pleural effusion (PE), frequently encountered in clinical practice, can arise from a variety of underlying conditions. Accurate differential diagnosis of PE is crucial, as treatment and prognosis are heavily dependent on the underlying etiology. However, diagnosing the cause of PE remains challenging, relying on mycobacteriological methods that lack sensitivity and are time-consuming, or on histological examinations that require invasive biopsies. The recent advancements in metagenomic next-generation sequencing (mNGS) have shown promising applications in the diagnosis of infectious diseases. Despite this, there is limited research on the utility of mNGS as a comprehensive diagnostic tool for simultaneously identifying the causes of PE, particularly in cases of tuberculosis or malignancy.Methods: This study aimed to assess the efficacy of mNGS in detecting tuberculous pleural effusion (TPE) and malignant pleural effusion (MPE). A total of 35 patients with PE were included, and their PE samples were analyzed using mNGS.Results: Among the participants, 8 were ultimately diagnosed with TPE, and 10 were diagnosed with MPE, with lung adenocarcinoma being the most prevalent pathological type (50%, 5/10), according to established diagnostic criteria.Additionally, 7 patients were diagnosed with non-infectious PE. However, mNGS identified only 2 cases of TPE and 8 cases of MPE. The sensitivity of mNGS for detecting Mycobacterium tuberculosis was 25% (2/8), while the specificity was 100%.For tumor detection, mNGS demonstrated a sensitivity of 80%, a specificity of 92.6%, and an AUC of 0.882.mNGS is effective in distinguishing MPE from non-MPE, but is not suitable for diagnosing TPE.
Keywords: metagenomic next-generation sequencing, Pleural Effusion, malignant pleural effusion, Tuberculous pleural effusion, Mycobacterium tuberculosis
Received: 08 Nov 2024; Accepted: 06 Mar 2025.
Copyright: © 2025 Li, Yan, Sun, Tang, Cao, Lv, Liang, Wei, Zhong, Zhao and Zhao. This is an open-access article distributed under the terms of the Creative Commons Attribution License (CC BY). The use, distribution or reproduction in other forums is permitted, provided the original author(s) or licensor are credited and that the original publication in this journal is cited, in accordance with accepted academic practice. No use, distribution or reproduction is permitted which does not comply with these terms.
* Correspondence:
Yujun Li, Guangdong Medical University, Zhanjiang, 524023, Guangdong, China
Zhuxiang Zhao, Department of Pulmonary and Critical Care Medicine,, Guangzhou First People's Hospital, Guangzhou, China
Disclaimer: All claims expressed in this article are solely those of the authors and do not necessarily represent those of their affiliated organizations, or those of the publisher, the editors and the reviewers. Any product that may be evaluated in this article or claim that may be made by its manufacturer is not guaranteed or endorsed by the publisher.
Research integrity at Frontiers
Learn more about the work of our research integrity team to safeguard the quality of each article we publish.