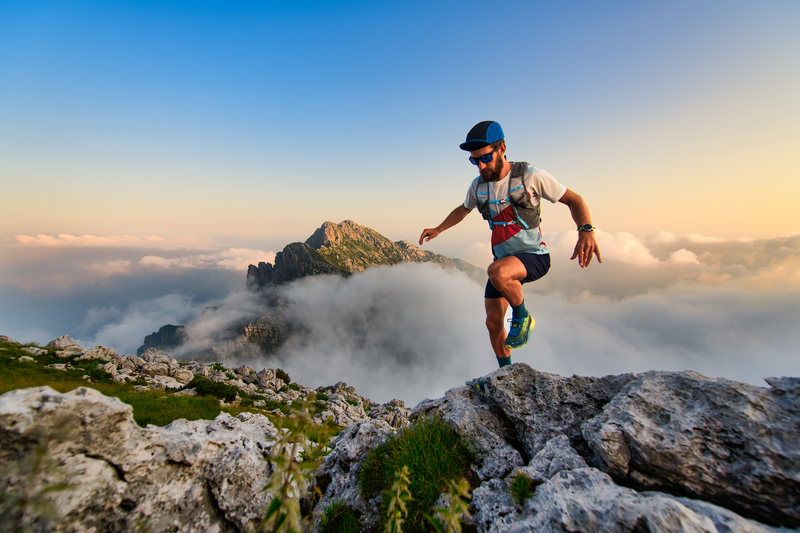
95% of researchers rate our articles as excellent or good
Learn more about the work of our research integrity team to safeguard the quality of each article we publish.
Find out more
ORIGINAL RESEARCH article
Front. Med.
Sec. Translational Medicine
Volume 12 - 2025 | doi: 10.3389/fmed.2025.1524286
The final, formatted version of the article will be published soon.
You have multiple emails registered with Frontiers:
Please enter your email address:
If you already have an account, please login
You don't have a Frontiers account ? You can register here
The Internet of Medical Things (IoMT) is transforming healthcare by enabling continuous remote patient monitoring, diagnostics, and personalized therapies. However, the widespread deployment of these devices introduces significant security vulnerabilities due to limited resources and inadequate network protocols. Intrusions within IoMT networks can compromise patient privacy, disrupt critical medical services, and jeopardize patient safety. To address these challenges, we propose HCLR-IDS, an advanced Intrusion Detection System (IDS) specifically designed for IoMT networks. The system integrates Convolutional Neural Networks (CNN), Long Short-Term Memory (LSTM) networks, and Reinforcement Learning (RL) techniques, namely Deep Q-Network (DQN) and Proximal Policy Optimization (PPO), to enhance the detection of evolving threats. The methodology begins with Enhanced Mutual Information Feature Selection (MIFS) to preprocess the CICIoMT2024 dataset, selecting the most relevant features while reducing noise and computational complexity. These selected features are then passed through a hybrid CNN-LSTM architecture. The CNN captures spatial patterns in network traffic, while the LSTM identifies temporal patterns. This dual feature extraction approach enables the system to effectively detect both static and dynamic characteristics of IoMT data. After feature extraction, the model incorporates DQN and PPO for decision-making. DQN optimizes actions based on Q-values, enhancing detection rewards, while PPO ensures stability in dynamic environments through a clipping mechanism. This combination of adaptive Q-learning and stable policy optimization significantly improves system robustness, ensuring effective real-time intrusion detection. The model demonstrates exceptional performance with binary classification accuracy of 0.9958, outperforming traditional IDS models. Additionally, it performs effectively in multi-class classification across 18 classes, achieving an accuracy of 0.7773. These results highlight that HCLR-IDS offers a reliable and efficient solution for securing IoMT healthcare systems.
Keywords: Internet of medical things, Intrusion detection system, CNN, LSTM, reinforcement learning
Received: 17 Dec 2024; Accepted: 17 Feb 2025.
Copyright: © 2025 Shaikh, Wang, Wajeeh Us Sima, Muhammad, Owais, Hassan, Alkanhel and Muthanna. This is an open-access article distributed under the terms of the Creative Commons Attribution License (CC BY). The use, distribution or reproduction in other forums is permitted, provided the original author(s) or licensor are credited and that the original publication in this journal is cited, in accordance with accepted academic practice. No use, distribution or reproduction is permitted which does not comply with these terms.
* Correspondence:
Chengliang Wang, Department of Computer Science and Technology, College of Computer Science, Chongqing University, Chongqing, China, Chongqing, China
Reem Alkanhel, Department of Information Technology, College of Computer and Information Sciences, Princess Nourah bint Abdulrahman University, Saudi Arabia, Riyadh, 84428, Riyadh, Saudi Arabia
Disclaimer: All claims expressed in this article are solely those of the authors and do not necessarily represent those of their affiliated organizations, or those of the publisher, the editors and the reviewers. Any product that may be evaluated in this article or claim that may be made by its manufacturer is not guaranteed or endorsed by the publisher.
Research integrity at Frontiers
Learn more about the work of our research integrity team to safeguard the quality of each article we publish.