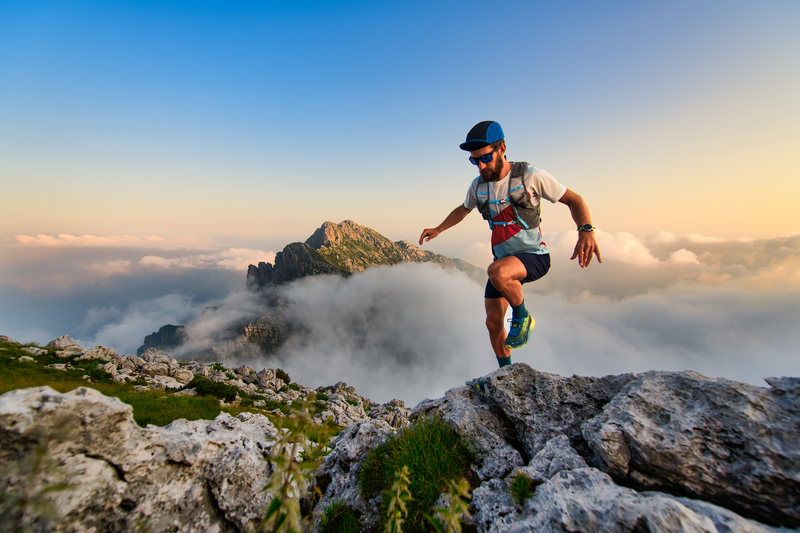
94% of researchers rate our articles as excellent or good
Learn more about the work of our research integrity team to safeguard the quality of each article we publish.
Find out more
SYSTEMATIC REVIEW article
Front. Med.
Sec. Precision Medicine
Volume 12 - 2025 | doi: 10.3389/fmed.2025.1523902
This article is part of the Research Topic AI-Driven Advances in Immunology and Immune-Mediated Disorders View all articles
The final, formatted version of the article will be published soon.
You have multiple emails registered with Frontiers:
Please enter your email address:
If you already have an account, please login
You don't have a Frontiers account ? You can register here
Artificial intelligence (AI) is increasingly recognized in the fields of allergy and immunology, profoundly influencing its development. This study employed bibliometric methods to provide a comprehensive research of AI applications in allergy and immunology, deeply discussing the current status, development trends, and future research hotspots. The goal is to help researchers quickly identify research hotspots and foster collaboration to advance the field. Our research reveals that 21,552 authors have published 3,883 related articles across 1,247 journals, with the USA and China leading in output and impact. Currently, we extensively use AI, particularly machine learning (ML) and deep learning (DL), in drug prediction and development, allergy and immunological disease classification and prediction, immune response exploration and modeling, decision support, diagnostics, digitalization and smart integration of healthcare systems, and medical education. It is evolving towards integration with personalized medical systems, offering substantial future application opportunities. Despite serious challenges in technology, ethics, and regulation, we believe AI will play a positive role globally, improving health conditions and enhancing well-being worldwide.
Keywords: artificial intelligence, Allergy and Immunology, immunology, machine learning, deep learning, Health management, Bibliometric Study
Received: 08 Nov 2024; Accepted: 27 Mar 2025.
Copyright: © 2025 Xiao, Huang, Wu, Li, Zang, Shinwari, Tuzankina, Chereshnev and Liu. This is an open-access article distributed under the terms of the Creative Commons Attribution License (CC BY). The use, distribution or reproduction in other forums is permitted, provided the original author(s) or licensor are credited and that the original publication in this journal is cited, in accordance with accepted academic practice. No use, distribution or reproduction is permitted which does not comply with these terms.
* Correspondence:
Guojun Liu, Inner Mongolia University of Science and Technology, Baotou, 130012, Inner Mongolia Autonomous Region, China
Disclaimer: All claims expressed in this article are solely those of the authors and do not necessarily represent those of their affiliated organizations, or those of the publisher, the editors and the reviewers. Any product that may be evaluated in this article or claim that may be made by its manufacturer is not guaranteed or endorsed by the publisher.
Research integrity at Frontiers
Learn more about the work of our research integrity team to safeguard the quality of each article we publish.