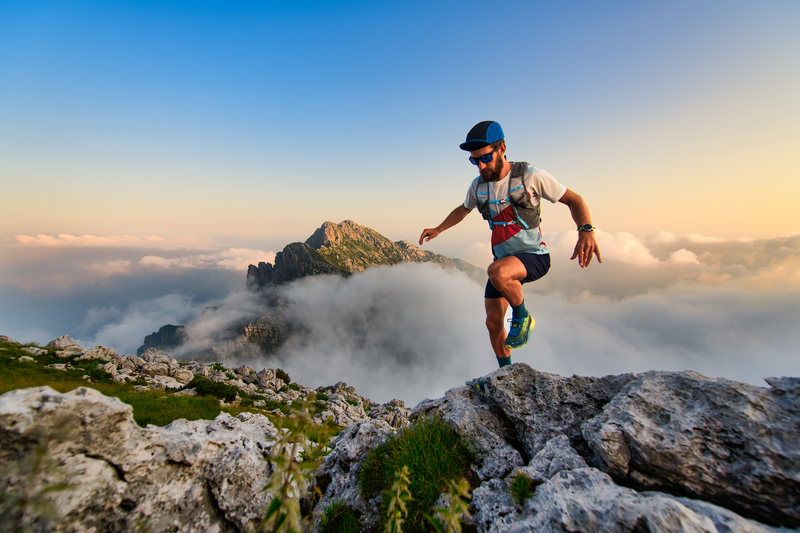
95% of researchers rate our articles as excellent or good
Learn more about the work of our research integrity team to safeguard the quality of each article we publish.
Find out more
ORIGINAL RESEARCH article
Front. Med.
Sec. Precision Medicine
Volume 12 - 2025 | doi: 10.3389/fmed.2025.1511487
This article is part of the Research Topic Advances in Precision Medicine for Minimally Invasive Treatment of Pelvis/Hip Fractures: Integration of Digital and Intelligent Technologies View all 3 articles
The final, formatted version of the article will be published soon.
You have multiple emails registered with Frontiers:
Please enter your email address:
If you already have an account, please login
You don't have a Frontiers account ? You can register here
Accurate segmentation of pelvic fractures from computed tomography (CT) is crucial for trauma diagnosis and image-guided reduction surgery. The traditional manual slice-by-slice segmentation by surgeons is time-consuming, experience-dependent, and error-prone. The complex anatomy of the pelvic bone, the diversity of fracture types, and the variability in fracture surface appearances pose significant challenges to automated solutions. We propose an automatic pelvic fracture segmentation method based on deep learning, which effectively isolates hipbone and sacrum fragments from fractured pelvic CT. The method employs two sequential networks: an anatomical segmentation network for extracting hipbones and sacrum from CT images, followed by a fracture segmentation network that isolates the main and minor fragments within each bone region. We propose a distance-weighted loss to guide the fracture segmentation network's attention on the fracture surface. Additionally, multi-scale deep supervision and smooth transition strategies are incorporated to enhance overall performance. Tested on a curated dataset of 150 CTs, which we have made publicly available, our method achieves an average Dice coefficient of 0.986 and an average symmetric surface distance of 0.234 mm. The method outperformed traditional max-flow and a transformer-based method, demonstrating its effectiveness in handling complex fracture.
Keywords: CT segmentation, deep learning, Pelvic fracture, reduction planning, image-guided surgery
Received: 15 Oct 2024; Accepted: 28 Mar 2025.
Copyright: © 2025 Liu, Yibulayimu, Zhu, Shi, Liang, Zhao, Wu, Sang and Wang. This is an open-access article distributed under the terms of the Creative Commons Attribution License (CC BY). The use, distribution or reproduction in other forums is permitted, provided the original author(s) or licensor are credited and that the original publication in this journal is cited, in accordance with accepted academic practice. No use, distribution or reproduction is permitted which does not comply with these terms.
* Correspondence:
Yudi Sang, Beijing Rossum Robot Technology Co., Ltd., Beijing, China
Yu Wang, Beihang University, Beijing, 100083, Beijing Municipality, China
Disclaimer: All claims expressed in this article are solely those of the authors and do not necessarily represent those of their affiliated organizations, or those of the publisher, the editors and the reviewers. Any product that may be evaluated in this article or claim that may be made by its manufacturer is not guaranteed or endorsed by the publisher.
Research integrity at Frontiers
Learn more about the work of our research integrity team to safeguard the quality of each article we publish.