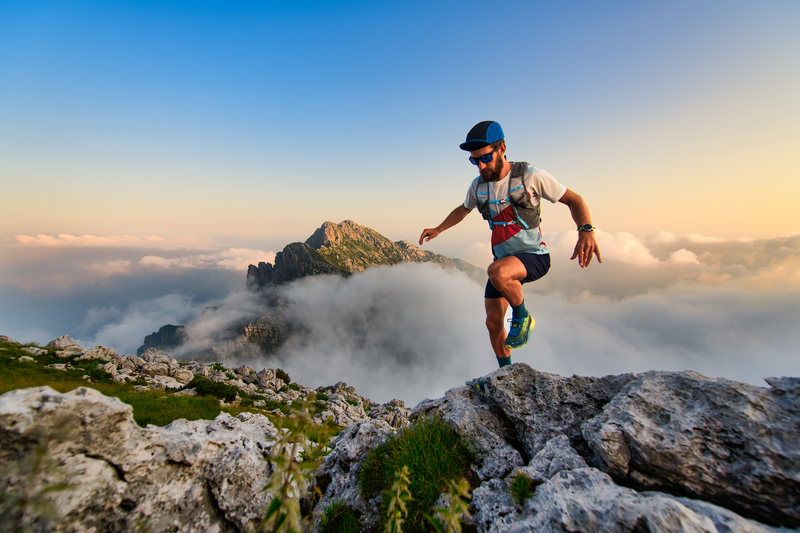
95% of researchers rate our articles as excellent or good
Learn more about the work of our research integrity team to safeguard the quality of each article we publish.
Find out more
REVIEW article
Front. Med. , 29 January 2025
Sec. Gastroenterology
Volume 12 - 2025 | https://doi.org/10.3389/fmed.2025.1510742
This article is part of the Research Topic Clinical prediction models in cancer through bioinformatics View all 5 articles
Gastric cancer, as one of the malignant tumors with a significant disease burden globally, emphasizes the importance of early diagnosis and personalized treatment for improving patient prognosis. In recent years, clinical prediction models (CPMs) have played a crucial role in predicting disease risks, assisting medical decision-making, and evaluating clinical prognosis and benefits as tools for risk–benefit assessment. Nomograms, as an important visualization form of clinical prediction models, have been increasingly applied in tumor-related research. Numerous studies have constructed multiple nomogram models by integrating clinical, pathological, laboratory, imaging data, and genetic characteristics, providing an accurate and effective tool for predicting the risk of gastric cancer, early diagnosis, treatment response assessment, and prognosis analysis. This article aims to review the current clinical applications and research progress of nomograms in gastric cancer, with the goal of providing robust references and theoretical support for clinical practice.
Gastric cancer (GC) is prevalent malignant tumors of the digestive system, ranking fifth and fourth in cancer incidence and mortality, respectively. Although a recent decrease in its incidence, GLOBOCAN 2020 statistics indicate that there are still over 1 million new cases of GC and 700,000 deaths worldwide each year (1–3). These data highlight the disease burden of GC globally, especially in East Asia where prevention and control efforts face severe challenges. The early symptoms of GC are usually unobvious and lack specificity, resulting in most patients being diagnosed at an advanced stage, missing the optimal treatment window and leading to poor prognosis (4). Early screening, diagnosis, and personalized treatment plan for GC hold great significance for improving patient prognosis. In addition, traditional prognostic evaluation methods mainly rely on tumor staging systems, often failing to fully consider individual differences among patients (5). Accurate prognostic evaluation is essential for improving treatment decisions and quality of life for GC patients.
In recent years, clinical prediction models (CPMs) have played an important role in predicting disease risks, assisting medical decision-making, and evaluating clinical prognosis and benefits as a tool to assess risks and benefits, becoming an effective way to translate clinical research into clinical practice (6, 7). Nomograms, as a common visualization form of CPMs, have been increasingly applied in tumor-related research. Especially in the field of GC, the application of nomograms in efficacy monitoring and prognostic evaluation has received widespread attention, and there are also related studies exploring its potential value in GC, risk prediction and disease assessment (8–10). This article aims to review the current clinical applications and research progress of nomograms in GC, providing a reference and theoretical basis for clinical practice.
This article presents a comprehensive review of relevant English- articles in the PubMed database as of July 16, 2024. The search strategy employed the following terms: (“Gastric cancer” OR “Gastric carcinoma” OR “Cancer of Stomach” OR “Stomach neoplasms” OR “Stomach cancer”) AND (Nomograms OR “Predictive Nomograms”). The review included all research articles, systematic reviews, and meta-analyses. However, case reports, brief communications, editorials, articles in non-English languages, and those with little relevance to the scope of this literature review were excluded.
The nomogram is a data visualization tool. The basic principle is to build a multi-factor regression model, assign points to each influencing factor according to their contribution to the outcome variable, and then aggregated to get a total score. Finally, a functional transformation relationship between the total score and the probability of the outcome event is obtained. Thus, the predicted value of the outcome event of the individual is calculated, and the prediction model is finally displayed in a graphical way (11, 12). The process of constructing a nomogram generally includes: determining outcome variables and study population, selecting inclusion variables, and constructing a model. In order to evaluate the predictive performance of the nomogram and to judge its applicable population, the model needs to be validated and evaluated. Model validation includes internal validation and external validation. Current studies often evaluate the differentiation, calibration, and clinical utility of the nomogram (13, 14).
The timing of diagnosis and treatment is closely related to the prognosis of patients with GC. The 5-year survival rate of patients with early GC after active treatment such as surgery is as high as 90%, while the 5-year survival rate of patients with advanced GC is less than 30% (15, 16). Therefore, early identification of high-risk individuals for GC and prompt diagnosis are crucial for improving patient survival and prognosis. However, it is inefficient and difficult to use gastroscopy and pathological biopsy as universal screening tools. In response to this challenge, Wang et al. (17) conducted a multicenter cross-sectional study, retrospectively analyzing clinical data from 7,945 patients in 115 hospitals in China. Within the study, two nomogram models were established based on individual characteristics, laboratory testing indicators, and dietary habits to predict the Operative link for gastritis assessment (OLGA) arbitrary stage and OLGA III-IV stage in high-risk GC populations in China. These nomogram models can predict the presence and severity of gastric atrophy, contributing to the early identification and screening of high-risk individuals for GC and potentially reducing the reliance on endoscopic examinations in clinical practice.
In addition, Sun et al. (18) developed and validated a nomogram model for the diagnosis of gastric low-grade intraepithelial neoplasia (LGIN) in patients with chronic atrophic gastritis (CAG) who underwent gastroscopy biopsy. The model incorporated 10 predictive factors, including age, sex, lesion location, intestinal metaplasia, multiple location, lesion size, erosion, edema, surface white fur, and form, to present the results in a visual nomogram. This nomogram can be used for the diagnosis of high-risk LGIN patients, significantly improving the detection rate of precancerous lesions of GC through enhanced surveillance and active treatment, thereby reducing the incidence of GC. Consequently, this predictive model can be used for individualized prediction of LGIN or as a further supplement to the indications for endoscopic biopsy.
At present, endoscopic forceps biopsy (EFB) is an essential tool for the histopathological diagnosis of early gastric neoplasms (EGN). However, when used in isolation, EFB has limited clinical value in the preoperative assessment of EGN lesions (19, 20). To enhance the accuracy of prediction, Zhao et al. (10) developed a nomogram model based on clinical, laboratory, and endoscopic observation characteristics. This model can objectively and accurately predict the individual risk of pathological upgrading in patients with EGN before undergoing endoscopic submucosal dissection (ESD), demonstrating excellent calibration and discrimination. Another study conducted the first whole-genome analysis of long non-coding RNA (lncRNA) in tissue and plasma samples, identifying five new plasma lncRNAs (TINCR, CCAT2, AOC4P, BANCR, and LINC00857) as potential diagnostic biomarkers for GC. Building upon these lncRNAs, the researchers further developed a nomogram model aimed at promoting its clinical application in diagnosis (21). Table 1 describes nomograms on the prediction of the risk associated with GC. These nomograms can be used to predict the risk grade of GC patients and the individual risk of pathological escalation, enabling early identification and screening of GC while reducing reliance on endoscopy.
Lymph node metastasis (LNM), peritoneal dissemination, and other adverse pathological features are significant indicators of GC progression, usually closely associated with poor prognosis (22, 23). Accurate prediction of adverse pathological stages prior to radical resection of GC is of great significance for comprehensively assessing the patient’s condition, formulating individualized treatment plans, and improving patient prognosis. Currently, for GC patients diagnosed with lymph node metastasis, the standard treatment strategy is still total or subtotal gastrectomy combined with systemic D1+/D2 lymph node dissection (24, 25). Therefore, the presence or absence of lymph node metastasis is a key factor that needs comprehensive assessment in early GC patients. Numerous studies (2, 26–33) have identified a series of clinicopathological factors related to LNM in GC patients. By integrating various clinical, pathological, laboratory test results, and imaging characteristics, researchers have constructed multiple nomogram models. These nomogram models can promote individualized preoperative prediction of LNM in GC patients, providing valuable auxiliary tools for clinicians to making treatment decisions, thus improving the overall treatment outcomes and quality of life for GC patients.
Peritoneal Metastasis (PM) represents the most common form of distant metastasis in GC patients, carrying an extremely poor prognosis (34). Once PM occurs in GC patients, it often leads to a significant decline in quality of life and a marked shortening of survival time (35, 36). In clinical practice, there are some limitations in the sensitivity of traditional imaging examinations to detect PM (37). Therefore, it is particularly crucial to identify the presence of PM using more accurate non-invasive methods preoperatively. Chen et al. (38) retrospectively analyzed clinical, pathological, and demographic parameters of 1,112 GC patients, and identified eight independent risk factors for peritoneal dissemination, including age, sex, tumor location, tumor size, signet-ring cell carcinoma (SRCC), T stage, N stage and Borrmann classification IV. A nomogram model was constructed based on these factors to predict peritoneal dissemination, and its predictive efficacy was confirmed through internal and external data validation. On the other hand, Zhao et al. (39) established a novel nomogram model based on serum glycation biomarkers and clinicopathological characteristics. This model also demonstrated good diagnostic performance (AUC: 0.892), achieving individualized assessment of the risk of peritoneal metastasis in GC patients. Consequently, the above two nomogram models hold great value in accurately predicting PM in patients with GC, and can assist clinicians to make more accurate clinical decisions before surgery.
Table 2 delineates the various nomogram models employed to predict LNM, PM, lymphovascular invasion, perineural invasion, and thickening of the distal gastric wall prior to radical gastrectomy. These predictive models frequently incorporated variables such as tumor size, depth of invasion, T stage, and CT parameters, among others. In conclusion, the precise preoperative prediction of GC progression in patients is crucial for formulating the most effective clinical treatment plans, thereby maximizing therapeutic outcomes and enhancing patient prognosis.
Neoadjuvant chemotherapy (NAC) has been demonstrated to effectively reduce tumor burden, downstage the disease, enhance rates of surgical resection, and improve the prognosis of patients with GC (40, 41). Numerous international guidelines recommend NAC as a critical therapeutic approach to improve the therapeutic effect of patients with advanced GC (42, 43). However, the survival benefit conferred by NAC depends on the pathological response to chemotherapeutic agents (44), and there exists considerable inter-individual variability in patient responses to NAC (45). Patients who achieve a complete pathological response often exhibit longer overall survival (OS) and disease-free survival (44), whereas those with poor responses to NAC may face a worse prognosis (46). Therefore, the early and accurate prediction of a GC patient’s response to NAC is of paramount importance for avoiding ineffective treatments and devising personalized therapeutic strategies.
Table 3 describes the various nomogram models used to predict the response to NAC in patients with advanced GC. In recent years, nomograms constructed based on imaging characteristics have emerged as important tools to evaluate the treatment response in GC patients, and provide significant guidance for devising personalized treatment plans. These studies utilized imaging techniques such as computed tomography (CT) and magnetic resonance imaging (MRI), combined with artificial intelligence (AI) technologies like machine learning and deep learning, to construct a series of nomogram models. These nomogram models not only incorporate imaging characteristics but also integrate clinical data, making the predictive models more comprehensive and accurate. The application of AI enhances precision and enables extraction of complex features for constructing nomograms. This approach enables a more precise assessment of patients’ treatment response, providing clinicians with more reliable decision support.
In summary, nomograms based on imaging characteristics and AI technologies hold broad application prospects in predicting the response to neoadjuvant chemotherapy in GC and in aiding therapeutic decision-making. Subsequent studies can further optimize nomogram models, take full advantage of AI technologies, integrate a variety of imaging techniques and clinical data, and conduct more external validations with the aim of achieving more precise predictions of treatment responses, thereby providing stronger support for personalized treatment of GC patients.
In the field of GC treatment, despite significant progress in recent years, radical gastrectomy remains the principal modality of treatment for GC (47). However, postoperative complications not only escalate the cost and duration of hospitalization but also may adversely affect patient prognosis (48, 49). Therefore, the early prediction and identification of the risk of postoperative complications is of great significance for guiding clinical treatment, reducing complications incidence and mortality rates, and improving the quality of life for patients. In the field of predicting postoperative complications in GC, the nomograms have been applied and developed in numerous studies as efficient risk assessment tools. Table 4 describes in detail various models used to predict the risk of complications following radical treatment for GC.
Postoperative infection is one of the common complications in GC patients. HWANG et al. retrospectively collected clinical and pathological data from 237 GC patients who underwent radical gastrectomy, and used logistic regression analysis to construct a nomogram model to predict the occurrence of infection after radical treatment for GC. This model incorporates clinical and laboratory parameters such as age, approach, operation time, WBC count, NLR, and CRP (50). Using this model before discharge can assist in identifying individuals requiring additional treatment, thereby minimizing the risk of patient readmission. Furthermore, a retrospective study confirmed that body mass index, glucose, hemoglobin, albumin, surgical duration, and bleeding volume were independent risk factors for intra-abdominal infection, and the nomogram model constructed based on these factors demonstrated excellent predictive performance in both the training set (AUC = 0.933) and the validation set (AUC = 0.951) (51). This model can effectively screen high-risk patients with postoperative intraperitoneal infection and guide clinicians in optimizing perioperative management for such individuals to reduce the incidence of postoperative infection. It is worth noting that the model was established based on an elderly patient population, which reflects the applicability and importance of nomograms in different patient groups. Selection bias is inevitable in these retrospective studies, and it is necessary to externally validate the model in the future to improve their clinical applicability.
Nomograms have also demonstrated high accuracy and practicality in predicting specific complication risks, such as anastomotic leakage (AL), lower extremity deep vein thrombosis (DVT), and hyperactive delirium. Shi et al. conducted the first prospective cohort study with independent internal validation and constructed a nomogram model for early diagnosis of anastomotic leakage after radical treatment for GC by analyzing inflammatory factors in abdominal drainage fluid. The model showed a C-index of 0.93 in the train cohort and a C-index of 0.82 in the validation cohort, demonstrating good predictive power (52). Postoperative venous thromboembolic events, such as lower extremity deep vein thrombosis (DVT), are major risk factors for GC patients after radical gastrectomy. Accurate prediction and management of these risks is essential is critical to improving postoperative care and patient outcomes for GC patients. Zhou et al. (53) developed a nomogram model to predict DVT in the lower extremities after radical gastrectomy for GC, based on various machine learning methods. The model exhibited high predictive value in both the training set (AUC = 0.936) and the validation set (AUC = 0.875), becoming an important tool for clinicians to identify and manage the risk of lower extremity DVT in this patient population. Additionally, nomograms are helpful in predicting hyperactive delirium after laparoscopic radical gastrectomy in GC patients (54) and delayed postoperative neurocognitive recovery in elderly GC patients (55).
In summary, nomograms have broad application prospects in predicting complications after GC treatment. By integrating clinical, laboratory, and imaging parameters, nomograms can provide personalized risk assessment for clinicians, thereby optimizing treatment decisions and patient management. However, these nomograms still need to be validated for their effectiveness and universality through larger sample sizes and further prospective studies.
The recurrence of GC after treatment significantly impacts prognosis. In recent years, nomograms have been widely used as effective predictive tools to assess the risk of recurrence in patients with GC. Endoscopic mucosal dissection (ESD) is an effective treatment for early gastric cancer (EGC) (56), and accurately predicting postoperative local recurrence risk is particularly important. Through in-depth analysis, Li et al. (57) identified multiple risk factors for recurrence after ESD in patients with EGC, including diabetes, alcohol consumption, lymphatic infiltration, complications, and multiple lesions. Based on these factors, the study established the first high-precision nomogram model for predicting the risk of EGC recurrence after ESD. In addition, another study developed a nomogram model with higher predictive efficiency (AUC = 0.933) based on the two predictors of lymph node positivity and helicobacter pylori (HP) infection (58). These models provide important reference for clinicians to identify high-risk patient groups and plan more reasonable follow-up strategies, as well as reliable data support for the prevention and treatment of postoperative recurrence.
Early detection of recurrence after radical gastrectomy is essential to improve the prognosis and survival rate of GC patients. Huang et al. developed a practical GC recurrence risk prediction model based on Lasso-Cox regression analysis. This model incorporates seven variables, including pathological stage, tumor size, the number of total lymph nodes, the number of metastatic lymph nodes, intraoperative blood loss (IBL), and levels of AFP and CA199, enabling rapid assessment of patients’ risk of recurrence (59). Subsequently, Cao et al. (60) further integrated clinical and pathological characteristics of GC patients to establish a risk model for predicting early postoperative recurrence. This nomogram not only accurately predicts the early postoperative recurrence rate in GC patients and identifies high-risk individuals for postoperative GC recurrence but also provides important guidance for clinicians to formulate appropriate treatment plans and effective follow-up strategies.
Table 5 outlines the various nomogram models utilized to predict postoperative recurrence in patients with GC. In summary, multiple nomograms constructed by integrating clinicopathological features, imaging features and biomarkers of patients is of great significance in predicting postoperative recurrence of patients with GC. These models not only improve the accuracy of clinical decision-making, but also provide a scientific basis for personalized treatment of patients.
Accurate prediction of patient survival is crucial for optimizing treatment strategies and improving prognosis in the field of GC treatment. As efficient personalized prediction tools, nomograms have been widely used for survival prediction in GC patients, and have been continuously improved and optimized in research. Wang et al. (61) developed a nomogram based on inflammation, nutritional and pathological factors to predict the overall survival of patients with GC after radical gastrectomy. In addition, a retrospective dual-center cohort study (62) conducted an in-depth analysis of clinical data of stage II/III GC patients undergoing radical resection and perioperative adjuvant chemotherapy (PAC) at two tertiary hospitals. Based on multivariate Cox regression analysis of the training group, the investigators constructed a nomogram integrating four independent predictors: BMI, total gastrectomy, TNM stage and peri-operative adjuvant chemotherapy (PAC) to estimate the survival probability of patients with GC. The AUC and calibration curve of this nomogram indicated good discrimination and calibration performance. The results of decision curve analysis (DCA) further confirmed that the model has better net benefit compared to the eighth TNM staging system. This nomogram provides a tool for clinicians to assess the probability of survival in GC patients receiving incomplete PAC and helps encourage patients to complete established chemotherapy regimens.
For the special subtype of gastric signed-ring cell carcinoma (GSRCC), several studies have developed nomograms to provide accurate assessment tools for predicting the survival probability of this patients. Jiang et al. (63) developed and verified a nomogram model for predicting overall survival (OS) and cancer-specific survival (CSS) of GSRCC patients by including six variables, such as age, race, tumor size, tumor site, N stage and AJCC stage. The study also created a dynamic web application based on the nomogram to facilitate clinical decision-making. Shao et al. (64) developed another nomogram based on age, TNM staging system, surgery, and chemotherapy as four independent prognostic factors. Compared with the traditional AJCC staging system, the constructed nomograms demonstrated more significant net clinical benefit and predictive value. It is evident that nomograms play an important role in the survival prediction of GSRCC patients. Not only does they provide clinicians with an accurate tool for predicting survival rates for individual patients, they also help develop more personalized treatment strategies.
Furthermore, the role of specific biomarkers or genetic markers in predicting the prognosis of GC has also attracted the attention of researchers (9, 65, 66). For example, a nomogram based on nine differentially expressed genes associated with gut Microflora was developed to predict prognosis and overall survival time in patients with GC (65). The nomogram constructed by Zhong et al. based on five T-cell marker genes and clinical factors could accurately predict the possibility of survival at 1, 3, and 5 years after diagnosis in GC patients (66). The introduction of machine learning technology provides new technical support for the establishment of nomogram models and further improves the accuracy of prediction (67).
Table 6 describes the various nomogram models used to predict survival in patients with GC. To sum up, nomograms play a key role in predicting the survival of GC patients. By integrating multiple factors such as inflammation, nutrition, pathology, tumor markers and genes, nomograms provide more accurate prediction tools for prognosis assessment of patients with GC. Future studies need to further explore and integrate more biomarkers and clinical data to continuously verify and improve the accuracy and clinical practicability of the model, so as to provide more scientific guidance for the treatment and management of GC patients.
CPMs, as important tools for evaluating the risk and prognosis of disease, have been widely used in the field of oncology. As an intuitive prediction tool, nomogram comprehensively take into account individual differences, thereby significantly enhancing the early identification rate of the high-risk GC population. They can optimize therapeutic strategies and provide patients with personalized prognostic information, while concurrently offering robust support for the decision-making process of clinical physicians. Despite remarkable progress in research and clinical application in the field of GC, nomogram still faces some challenges and limitations. Firstly, the data sources for most nomogram models are from single centers, and mainly undergo internal validation, lacking external validation from multi-center and large sample cohorts. Secondly, most models are constructed based on retrospective data, which may be the risk of bias. In addition, some nomograms rely on features that are relatively difficult to obtain, such as gene expression or sequencing data, as variables. This significantly restricts their clinical applicability.
Looking forward to the future, with the continuous progress of science and technology and deepening research, the application of nomogram in GC will become more extensive and accurate. Subsequent studies can further integrate multivariate data such as imaging techniques, biomarkers, and genetic information to optimize nomograms to improve prediction accuracy and clinical practicability. At the same time, larger sample sizes and multi-center studies are necessary to enhance external validation of nomograms, ensuring their applicability and accuracy across different patient populations. Moreover, combined with AI technologies such as machine learning and deep learning, the accuracy of nomogram models and the ability to extract complex features can be further improved, providing more scientific basis for personalized diagnosis and treatment of GC patients. Future studies should also incorporate patients’ lifestyle and environmental factors to construct more comprehensive predictive models. Additionally, developing dynamic web applications and mobile medical tools to enhance the usability of nomograms for clinicians and patients is also an important direction for future work. In summary, the application of nomograms in the field of GC will continue to provide important references and theoretical basis for clinical practice, and make greater contributions to improving the diagnosis, treatment, prognosis and quality of life of patients.
HW: Conceptualization, Data curation, Investigation, Methodology, Writing – original draft. YD: Methodology, Project administration, Supervision, Writing – original draft. MZ: Methodology, Project administration, Supervision, Writing – original draft. KL: Investigation, Methodology, Supervision, Writing – review & editing. SZ: Methodology, Project administration, Visualization, Writing – review & editing. DL: Conceptualization, Data curation, Methodology, Writing – review & editing.
The author(s) declare that no financial support was received for the research, authorship, and/or publication of this article.
The authors declare that the research was conducted in the absence of any commercial or financial relationships that could be construed as a potential conflict of interest.
The authors declare that no Gen AI was used in the creation of this manuscript.
All claims expressed in this article are solely those of the authors and do not necessarily represent those of their affiliated organizations, or those of the publisher, the editors and the reviewers. Any product that may be evaluated in this article, or claim that may be made by its manufacturer, is not guaranteed or endorsed by the publisher.
1. Sung, H, Ferlay, J, Siegel, RL, Laversanne, M, Soerjomataram, I, Jemal, A, et al. Global Cancer statistics 2020: GLOBOCAN estimates of incidence and mortality worldwide for 36 cancers in 185 countries. CA Cancer J Clin. (2021) 71:209–49. doi: 10.3322/caac.21660
2. You, H, Chen, S, and Wang, S. A nomogram for predicting lymph node metastasis in early gastric signet ring cell carcinoma. Sci Rep. (2023) 13:15039. doi: 10.1038/s41598-023-40733-1
3. Wei, L, Fu, B, Bo, J, Jia, H, Sun, M, Jiang, X, et al. Development of a nomogram based on body composition analysis of quantitative computed tomography combined with clinical prognostic factors to predict disease-free survival after surgery and adjuvant chemotherapy in patients with gastric cancer. Quant Imaging Med Surg. (2023) 13:8489–503. doi: 10.21037/qims-23-309
4. Guan, W-L, He, Y, and Xu, R-H. Gastric cancer treatment: recent progress and future perspectives. J Hematol Oncol. (2023) 16:57. doi: 10.1186/s13045-023-01451-3
5. Zhang, M, Ding, C, Xu, L, Ou, B, Feng, S, Wang, G, et al. Comparison of a tumor-ratio-metastasis staging system and the 8th AJCC TNM staging system for gastric Cancer. Front Oncologia. (2021) 11:11. doi: 10.3389/fonc.2021.595421
6. van Smeden, M, Reitsma, JB, Riley, RD, Collins, GS, and Moons, KG. Clinical prediction models: diagnosis versus prognosis. J Clin Epidemiol. (2021) 132:142–5. doi: 10.1016/j.jclinepi.2021.01.009
7. Collins, GS, Dhiman, P, Ma, J, Schlussel, MM, Archer, L, Van Calster, B, et al. Evaluation of clinical prediction models (part 1): from development to external validation. BMJ. (2024) 384:e074819. doi: 10.1136/bmj-2023-074819
8. Chen, Y-H, Xiao, J, Chen, X-J, Wang, H-S, Liu, D, Xiang, J, et al. Nomogram for predicting pathological complete response to neoadjuvant chemotherapy in patients with advanced gastric cancer. World J Gastroenterol. (2020) 26:2427–39. doi: 10.3748/wjg.v26.i19.2427
9. Li, X, and Lei, Y. Construction of a prognostic risk model for stomach adenocarcinoma based on endoplasmic reticulum stress genes. Wien Klin Wochenschr. (2024) 136:319–30. doi: 10.1007/s00508-023-02306-0
10. Zhao, YH, Zheng, Y, Sha, J, Hua, HJ, Li, KD, Lu, Y, et al. A prediction model based on the risk factors associated with pathological upgrading in patients with early-stage gastric neoplasms diagnosed by endoscopic forceps biopsy. Gut Liver. (2023) 17:78–91. doi: 10.5009/gnl220060
11. Zhou, Z-R, Wang, W-W, Li, Y, Jin, K-R, Wang, X-Y, Wang, Z-W, et al. In-depth mining of clinical data: the construction of clinical prediction model with R. Ann Transl Med. (2019) 7:796. doi: 10.21037/atm.2019.08.63
12. Balachandran, VP, Gonen, M, Smith, JJ, and DeMatteo, RP. Nomograms in oncology: more than meets the eye. Lancet Oncol. (2015) 16:e173–80. doi: 10.1016/S1470-2045(14)71116-7
13. Alba, AC, Agoritsas, T, Walsh, M, Hanna, S, Iorio, A, Devereaux, PJ, et al. Discrimination and calibration of clinical prediction models: Users' guides to the medical literature. JAMA. (2017) 318:1377. doi: 10.1001/jama.2017.12126
14. Van Calster, B, Wynants, L, Verbeek, JFM, Verbakel, JY, Christodoulou, E, Vickers, AJ, et al. Reporting and interpreting decision curve analysis: a guide for investigators. Eur Urol. (2018) 74:796–804. doi: 10.1016/j.eururo.2018.08.038
15. Katai, H, Ishikawa, T, Akazawa, K, Isobe, Y, Miyashiro, I, Oda, I, et al. Five-year survival analysis of surgically resected gastric cancer cases in Japan: a retrospective analysis of more than 100, 000 patients from the nationwide registry of the Japanese gastric Cancer association (2001-2007). Gastric Cancer. (2018) 21:144–54. doi: 10.1007/s10120-017-0716-7
16. Wang, H, Zhang, H, Deng, P, Liu, C, Li, D, Jie, H, et al. Tissue metabolic profiling of human gastric cancer assessed by (1) H NMR. BMC Cancer. (2016) 16:371. doi: 10.1186/s12885-016-2356-4
17. Wang, S, Ye, F, Sheng, Y, Yu, W, Liu, Y, Liu, D, et al. Development and validation of nomograms to predict operative link for gastritis assessment any-stage and stages III-IV in the Chinese high-risk gastric Cancer population. Front Med (Lausanne). (2021) 8, 724566. doi: 10.3389/fmed.2021.724566
18. Sun, T, Ke, X-Q, Wang, M, and Wang, Q-Z. Establishment and validation of a clinical diagnostic model for gastric low-grade intraepithelial neoplasia. Medicine (Baltimore). (2023) 102:e35515. doi: 10.1097/MD.0000000000035515
19. Kwack, WG, Ho, WJ, Kim, JH, Lee, JH, Kim, EJ, Kang, HW, et al. Understanding the diagnostic yield of current endoscopic biopsy for gastric neoplasm: a prospective single-center analysis based on tumor characteristics stratified by biopsy number and site. Medicine (Baltimore). (2016) 95:e4196. doi: 10.1097/MD.0000000000004196
20. Soh, JS, Lim, H, Kang, HS, Kim, JH, and Kim, KC. Does the discrepancy in histologic differentiation between a forceps biopsy and an endoscopic specimen necessitate additional surgery in early gastric cancer? World J Gastrointest Oncol. (2017) 9:319–26. doi: 10.4251/wjgo.v9.i8.319
21. Zhang, K, Shi, H, Xi, H, Wu, X, Cui, J, Gao, Y, et al. Genome-wide lncRNA microarray profiling identifies novel circulating lncRNAs for detection of gastric Cancer. Theranostics. (2017) 7:213–27. doi: 10.7150/thno.16044
22. Zheng, Z, Liu, Y, Bu, Z, Zhang, L, Li, Z, Du, H, et al. Prognostic role of lymph node metastasis in early gastric cancer. Chin J Cancer Res. (2014) 26:2. doi: 10.3978/j.issn.1000-9604.2014.04.06
23. Rau, B, Brandl, A, Piso, P, Pelz, J, Busch, P, Demtröder, C, et al. Peritoneal metastasis in gastric cancer: results from the German database. Gastric Cancer. (2020) 23:11–22. doi: 10.1007/s10120-019-00978-0
24. Kishida, Y, Takizawa, K, Kakushima, N, Kawata, N, Yoshida, M, Yabuuchi, Y, et al. Endoscopic submucosal dissection versus surgery in elderly patients with early gastric cancer of relative indication for endoscopic resection. Dig Endosc. (2022) 34:497–507. doi: 10.1111/den.14105
25. Lim, JH, Kim, J, Kim, SG, and Chung, H. Long-term clinical outcomes of endoscopic vs. surgical resection for early gastric cancer with undifferentiated histology. Surg Endosc. (2019) 33:3589–99. doi: 10.1007/s00464-018-06641-6
26. Li, J, Fang, M, Wang, R, Dong, D, Tian, J, Liang, P, et al. Diagnostic accuracy of dual-energy CT-based nomograms to predict lymph node metastasis in gastric cancer. Eur Radiol. (2018) 28:5241–9. doi: 10.1007/s00330-018-5483-2
27. Yu, Z, Liang, C, Gao, J, He, P, Xu, Q, Gao, Y, et al. Clinicopathologic factors correlated with lymph node metastasis in gastric cancer: a retrospective cohort study involving 5606 patients. J Gastrointest Surg. (2024) 28:1242–9. doi: 10.1016/j.gassur.2024.05.014
28. Pan, S, An, W, Tan, Y, Chen, Q, Liu, P, and Xu, H. Prediction model of lymph node metastasis risk in elderly patients with early gastric Cancer before endoscopic resection: a retrospective analysis based on international multicenter data. J Cancer. (2021) 12:5583–92. doi: 10.7150/jca.56702
29. Wang, X, Yang, X, Cai, F, Cai, M, Liu, Y, Zhang, L, et al. The key role of tumor budding in predicting the status of lymph node involvement in early gastric Cancer patients: a clinical multicenter validation in China. Ann Surg Oncol. (2024) 31:4224–35. doi: 10.1245/s10434-024-15229-5
30. Wang, W, Xia, Y, and He, C. Development and validation of a predictive model associated with lymph node metastasis of gastric signet ring carcinoma patients. Medicine (Baltimore). (2023) 102:e36002. doi: 10.1097/MD.0000000000036002
31. Wu, H, Liu, W, Yin, M, Liu, L, Qu, S, Xu, W, et al. A nomogram based on platelet-to-lymphocyte ratio for predicting lymph node metastasis in patients with early gastric cancer. Front Oncol. (2023) 13:13. doi: 10.3389/fonc.2023.1201499
32. Yoo, HJ, Lee, H, Lee, HH, Lee, JH, Jun, K-H, Kim, J-J, et al. A nomogram for predicting Extraperigastric lymph node metastasis in patients with early gastric Cancer. J Gastric Cancer. (2023) 23:355–64. doi: 10.5230/jgc.2023.23.e18
33. Jiang, X-C, Yao, X-B, Xia, H-B, Su, Y-Z, Luo, P-Q, Sun, J-R, et al. Nomogram established using risk factors of early gastric cancer for predicting the lymph node metastasis. World J Gastrointest Oncol. (2023) 15:665–76. doi: 10.4251/wjgo.v15.i4.665
34. Sun, J, Song, Y, Wang, Z, Chen, X, Gao, P, Xu, Y, et al. Clinical significance of palliative gastrectomy on the survival of patients with incurable advanced gastric cancer: a systematic review and meta-analysis. BMC Cancer. (2013) 13:13. doi: 10.1186/1471-2407-13-577
35. Kono, K, Yong, W-P, Okayama, H, Shabbir, A, Momma, T, Ohki, S, et al. Intraperitoneal chemotherapy for gastric cancer with peritoneal disease: experience from Singapore and Japan. Gastric Cancer. (2017) 20:122–7. doi: 10.1007/s10120-016-0660-y
36. Allen, CJ, Newhook, TE, Vreeland, TJ, Das, P, Minsky, BD, Blum, M, et al. Yield of peritoneal cytology in staging patients with gastric and gastroesophageal cancer. J Surg Oncol. (2019) 120:1350–7. doi: 10.1002/jso.25729
37. Wang, Z, and Chen, J-Q. Imaging in assessing hepatic and peritoneal metastases of gastric cancer: a systematic review. BMC Gastroenterol. (2011) 11:11. doi: 10.1186/1471-230X-11-19
38. Chen, X, Chen, S, Wang, X, Nie, R, Chen, D, Xiang, J, et al. Analysis and external validation of a nomogram to predict peritoneal dissemination in gastric cancer. Chin J Cancer Res. (2020) 32:197–207. doi: 10.21147/j.issn.1000-9604.2020.02.07
39. Zhao, J, Qin, R, Chen, H, Yang, Y, Qin, W, Han, J, et al. A nomogram based on glycomic biomarkers in serum and clinicopathological characteristics for evaluating the risk of peritoneal metastasis in gastric cancer. Clin. Proteomics. (2020) 17:34. doi: 10.1186/s12014-020-09297-4
40. Reddavid, R, Sofia, S, Chiaro, P, Colli, F, Trapani, R, Esposito, L, et al. Neoadjuvant chemotherapy for gastric cancer. Is it a must or a fake? World J Gastroenterol. (2018) 24:274–89. doi: 10.3748/wjg.v24.i2.274
41. Cunningham, D, Allum, WH, Stenning, SP, Thompson, JN, Van de Velde, CJH, Nicolson, M, et al. Perioperative chemotherapy versus surgery alone for resectable gastroesophageal cancer. N Engl J Med. (2006) 355:11–20. doi: 10.1056/NEJMoa055531
42. Japanese gastric cancer treatment guidelines 2018 (5th edition). Gastric Cancer (2021) 24, 1–21. doi: 10.1007/s10120-020-01042-y
43. Ajani, JA, D'Amico, TA, Bentrem, DJ, Chao, J, Cooke, D, Corvera, C, et al. Gastric Cancer, version 2.2022, NCCN clinical practice guidelines in oncology. J Natl Compr Cancer Netw. (2022) 20:167–92. doi: 10.6004/jnccn.2022.0008
44. Achilli, P, De Martini, P, Ceresoli, M, Mari, GM, Costanzi, A, Maggioni, D, et al. Tumor response evaluation after neoadjuvant chemotherapy in locally advanced gastric adenocarcinoma: a prospective, multi-center cohort study. J Gastrointest Oncol. (2017) 8:1018–25. doi: 10.21037/jgo.2017.08.13
45. Coccolini, F, Nardi, M, Montori, G, Ceresoli, M, Celotti, A, Cascinu, S, et al. Neoadjuvant chemotherapy in advanced gastric and esophago-gastric cancer. Meta-analysis of randomized trials. Int J Surg. (2018) 51:120–7. doi: 10.1016/j.ijsu.2018.01.008
46. Biondi, A, Lirosi, MC, D'Ugo, D, Fico, V, Ricci, R, Santullo, F, et al. Neo-adjuvant chemo (radio) therapy in gastric cancer: current status and future perspectives. World J Gastrointest Oncol. (2015) 7:389. doi: 10.4251/wjgo.v7.i12.389
47. Yu, X, Lei, W, Zhu, L, Qi, F, Liu, Y, and Feng, Q. Robotic versus laparoscopic distal gastrectomy for gastric cancer: a systematic review and meta-analysis. Asian J Surg. (2024) 48:21–31. doi: 10.1016/j.asjsur.2024.06.051
48. Tokunaga, M, Kurokawa, Y, Machida, R, Sato, Y, Takiguchi, S, Doki, Y, et al. Impact of postoperative complications on survival outcomes in patients with gastric cancer: exploratory analysis of a randomized controlled JCOG1001 trial. Gastric Cancer. (2021) 24:214–23. doi: 10.1007/s10120-020-01102-3
49. Liu, F, Huang, C, Xu, Z, Su, X, Zhao, G, Ye, J, et al. Morbidity and mortality of laparoscopic vs open Total gastrectomy for clinical stage I gastric Cancer: the CLASS02 multicenter randomized clinical trial. JAMA Oncol. (2020) 6:1590. doi: 10.1001/jamaoncol.2020.3152
50. Hwang, SH, and Kim, DJ. Nomogram for predicting infectious complications following curative gastrectomy using clinical and laboratory parameters. Anticancer Res. (2024) 44:1781–90. doi: 10.21873/anticanres.16978
51. Yu, X, Tang, W, Bai, C, Li, R, Feng, B, Wu, J, et al. A predictive model for intraabdominal infection after radical gastrectomy in elderly patients. Medicine (Baltimore). (2024) 103:e37489. doi: 10.1097/MD.0000000000037489
52. Shi, J, Wu, Z, Wu, X, Shan, F, Zhang, Y, Ying, X, et al. Early diagnosis of anastomotic leakage after gastric Cancer surgery via analysis of inflammatory factors in abdominal drainage. Ann Surg Oncol. (2022) 29:1230–41. doi: 10.1245/s10434-021-10763-y
53. Zhou, H, Jin, Y, Chen, G, Jin, X, Chen, J, and Wang, J. Predictive modeling of lower extreme deep vein thrombosis following radical gastrectomy for gastric cancer: based on multiple machine learning methods. Sci Rep. (2024) 14:15711. doi: 10.1038/s41598-024-66754-y
54. Liu, J, Zhong, Q, Tan, H, Zhuo, M, Zhong, M, and Cai, T. Risk factors for hyperactive delirium after laparoscopic radical gastrectomy under general anesthesia in patients with gastric cancer. Am J Transl Res. (2023) 15:9.
55. Yong, R, and Jiang, L. Predicative factors and development of a nomogram for postoperative delayed neurocognitive recovery in elderly patients with gastric cancer. Aging Clin Exp Res. (2023) 35:1497–504. doi: 10.1007/s40520-023-02422-x
56. Forbes, N, Elhanafi, SE, Al-Haddad, MA, Thosani, NC, Draganov, PV, Othman, MO, et al. American Society for Gastrointestinal Endoscopy guideline on endoscopic submucosal dissection for the management of early esophageal and gastric cancers: summary and recommendations. Gastrointest Endosc. (2023) 98:271–84. doi: 10.1016/j.gie.2023.03.015
57. Li, S, Nahar, A, Zhang, Q, Xing, J, Li, P, Zhang, S, et al. Risk factors and a nomogram for predicting local recurrence in adult patients with early gastric cancer after endoscopic submucosal dissection. Dig Liver Dis. (2024) 56:1921–9. doi: 10.1016/j.dld.2024.04.004
58. Xu, H, Yang, X, Lu, Y, and Wang, S. Prediction of recurrence of early gastric carcinoma after endoscopic submucosal dissection. Afr Health Sci. (2023) 23:283–9. doi: 10.4314/ahs.v23i2.32
59. Huang, B, Ding, F, and Li, Y. A practical recurrence risk model based on lasso-cox regression for gastric cancer. J Cancer Res Clin Oncol. (2023) 149:15845–54. doi: 10.1007/s00432-023-05346-1
60. Cao, M, Hu, C, Pan, S, Zhang, Y, Yu, P, Zhang, R, et al. Development and validation of nomogram for predicting early recurrence after radical gastrectomy of gastric cancer. World J Surg Oncol. (2024) 22:21. doi: 10.1186/s12957-023-03294-1
61. Wang, Q, Zhang, Q, Zhu, J, Li, L, Zeng, R, Ding, H, et al. Nomogram for predicting overall survival after curative gastrectomy using inflammatory, nutritional and pathological factors. Clin Transl Oncol. (2024) 26:1001–11. doi: 10.1007/s12094-023-03340-0
62. Liu, D, Quan, H, Ma, M, Zhou, H, Yang, X, Wu, Z, et al. Nomogram to predict overall survival of patients receiving radical gastrectomy and incomplete peri-operative adjuvant chemotherapy for stage II/III gastric cancer: a retrospective bi-center cohort study. BMC Cancer. (2024) 24:344. doi: 10.1186/s12885-024-12103-1
63. Jiang, Y, Hu, H, Shao, X, Li, W, Lu, Y, Liang, J, et al. A novel web-based dynamic prognostic nomogram for gastric signet ring cell carcinoma: a multicenter population-based study. Front Immunol. (2024) 15:15. doi: 10.3389/fimmu.2024.1365834
64. Shao, X-X, Li, X-C, Lin, Z-J, Ruan, Y-J, Lu, G-R, Wang, W-Z, et al. A prognostic model for survival in patients with gastric signet ring cell carcinoma. Dig Dis. (2024) 42:221–9. doi: 10.1159/000536454
65. Yue, Q, Han, W, and Ling, LZ. Nine-gene prognostic signature related to gut microflora for predicting the survival in gastric Cancer patients. Turk J Gastroenterol. (2024) 35:102–11. doi: 10.5152/tjg.2024.23063
66. Zhong, J, Pan, R, Gao, M, Mo, Y, Peng, X, Liang, G, et al. Identification and validation of a T cell marker gene-based signature to predict prognosis and immunotherapy response in gastric cancer. Sci Rep. (2023) 13:21357. doi: 10.1038/s41598-023-48930-8
67. Lu, T, Lu, M, Liu, H, Song, D, Wang, Z, Guo, Y, et al. Establishment of a prognostic model for gastric cancer patients who underwent radical gastrectomy using machine learning: a two-center study. Front Oncol. (2023) 13:13. doi: 10.3389/fonc.2023.1282042
68. Lin, J, Su, H, Zhou, Q, Pan, J, and Zhou, L. Predictive value of nomogram based on Kyoto classification of gastritis to diagnosis of gastric cancer. Scand J Gastroenterol. (2022) 57:574–80. doi: 10.1080/00365521.2021.2023626
69. Tong, Y-X, Ye, X, Chen, Y-Q, You, Y-R, Zhang, H-J, Chen, S-X, et al. A nomogram model of spectral CT quantitative parameters and clinical characteristics predicting lymphovascular invasion of gastric cancer. Heliyon. (2024) 10:e29214. doi: 10.1016/j.heliyon.2024.e29214
70. Ge, H-T, Chen, J-W, Wang, L-L, Zou, T-X, Zheng, B, Liu, Y-F, et al. Preoperative prediction of lymphovascular and perineural invasion in gastric cancer using spectral computed tomography imaging and machine learning. World J Gastroenterol. (2024) 30:542–55. doi: 10.3748/wjg.v30.i6.542
71. Cong, R, Xu, R, Ming, J, and Zhu, Z. Construction of a preoperative nomogram model for predicting perineural invasion in advanced gastric cancer. Front Med (Lausanne). (2024) 11:11. doi: 10.3389/fmed.2024.1344982
72. He, Y, Yang, M, Hou, R, Ai, S, Nie, T, Chen, J, et al. Preoperative prediction of perineural invasion and lymphovascular invasion with CT radiomics in gastric cancer. Eur J Radiol Open. (2024) 12:100550. doi: 10.1016/j.ejro.2024.100550
73. Feng, Q-X, Xu, L-L, Li, Q, Jiang, X-T, Tang, B, Sun, N-N, et al. Development and validation of a dual-energy CT-based model to estimate the malignant probability of distal gastric wall thickening. J Gastrointest Oncol. (2022) 13:539–47. doi: 10.21037/jgo-21-552
74. Chen, K, Wang, Y, Lang, Y, Yang, L, Guo, Z, Wu, W, et al. Machine learning models to predict submucosal invasion in early gastric cancer based on endoscopy features and standardized color metrics. Sci Rep. (2024) 14:10445. doi: 10.1038/s41598-024-61258-1
75. Li, S-Q, Zhang, K-C, Li, J-Y, Liang, W-Q, Gao, Y-H, Qiao, Z, et al. Establishment and validation of a nomogram to predict the risk of ovarian metastasis in gastric cancer: based on a large cohort. World J Clin Cases. (2020) 8:4331–41. doi: 10.12998/wjcc.v8.i19.4331
76. Hu, W, Zhao, Y, Ji, H, Chen, A, Xu, Q, Liu, Y, et al. Nomogram based on dual-energy CT-derived extracellular volume fraction for the prediction of microsatellite instability status in gastric cancer. Front Oncologia. (2024) 14:14. doi: 10.3389/fonc.2024.1370031
77. Pan, B, Zhang, W, Chen, W, Zheng, J, Yang, X, Sun, J, et al. Establishment of the radiologic tumor invasion index based on Radiomics splenic features and clinical factors to predict serous invasion of gastric Cancer. Front Oncologia. (2021) 11:11. doi: 10.3389/fonc.2021.682456
78. Liu, J, Sun, R, Cai, K, Xu, Y, and Yuan, W. A nomogram combining neutrophil to lymphocyte ratio (NLR) and prognostic nutritional index (PNI) to predict distant metastasis in gastric cancer. Sci Rep. (2024) 14:15391. doi: 10.1038/s41598-024-65307-7
79. Chen, W, Zhang, W, Chen, X, Dong, W, Cai, Y, Cheng, J, et al. Computed tomography-based radiomics nomogram for predicting therapeutic response to neoadjuvant chemotherapy in locally advanced gastric cancer: a scale for treatment predicting. Clin Transl Oncol. (2024) 26:1944–55. doi: 10.1007/s12094-024-03417-4
80. Liu, B, Xu, Y-J, Chu, F-R, Sun, G, Zhao, G-D, and Wang, S-Z. Development of a clinical nomogram for prediction of response to neoadjuvant chemotherapy in patients with advanced gastric cancer. World J Gastrointest Surg. (2024) 16:396–408. doi: 10.4240/wjgs.v16.i2.396
81. Zhang, J, Zhang, Q, Zhao, B, and Shi, G. Deep learning nomogram for predicting neoadjuvant chemotherapy response in locally advanced gastric cancer patients. Abdom Radiol (NY). (2024) 49:3780–96. doi: 10.1007/s00261-024-04331-7
82. Cui, Y, Zhang, J, Li, Z, Wei, K, Lei, Y, Ren, J, et al. A CT-based deep learning radiomics nomogram for predicting the response to neoadjuvant chemotherapy in patients with locally advanced gastric cancer: a multicenter cohort study. EClinicalMedicine. (2022) 46:101348. doi: 10.1016/j.eclinm.2022.101348
83. Li, J, Yin, H, Wang, Y, Zhang, H, Ma, F, Li, H, et al. Multiparametric MRI-based radiomics nomogram for early prediction of pathological response to neoadjuvant chemotherapy in locally advanced gastric cancer. Eur Radiol. (2023) 33:2746–56. doi: 10.1007/s00330-022-09219-y
84. Zhong, H, Wang, T, Hou, M, Liu, X, Tian, Y, Cao, S, et al. Deep learning Radiomics nomogram based on enhanced CT to predict the response of metastatic lymph nodes to neoadjuvant chemotherapy in locally advanced gastric Cancer. Ann Surg Oncol. (2024) 31:421–32. doi: 10.1245/s10434-023-14424-0
85. Li, J, Chen, X, Xu, S, Wang, Y, Ma, F, Wu, Y, et al. Predicting pathologic response to neoadjuvant chemotherapy in locally advanced gastric cancer: the establishment of a spectral CT-based nomogram from prospective datasets. Eur J Surg Oncol. (2024) 50:108020. doi: 10.1016/j.ejso.2024.108020
86. Tan, X, Yang, X, Hu, S, Chen, X, and Sun, Z. A nomogram for predicting postoperative complications based on tumor spectral CT parameters and visceral fat area in gastric cancer patients. Eur J Radiol. (2023) 167:111072. doi: 10.1016/j.ejrad.2023.111072
87. Ma, X, Lu, X, Jiang, X, Wang, J, Wang, T, and Zhang, L. A nomogram combining prognostic nutritional index and platelet lymphocyte ratio predicts postoperative pulmonary infection following D2 radical gastrectomy for gastric cancer. Nutr Hosp. (2024) 41:602–11. doi: 10.20960/nh.05079
88. Zhou, L, Li, Y, Ni, Y, and Liu, C. Analysis of postoperative pulmonary complications after gastrectomy for gastric cancer: development and validation of a nomogram. Front Surg. (2023) 10:10. doi: 10.3389/fsurg.2023.1308591
89. Zhang, B, Zhu, Q, and Ji, Z-P. Nomogram for predicting early complications after distal gastrectomy. World J Gastrointest Surg. (2023) 15:2500–12. doi: 10.4240/wjgs.v15.i11.2500
90. Xu, B, Zhang, F, Peng, Y, and Tong, S. Predicting esophagojejunal anastomotic leakage in gastric cancer patients after total gastrectomy: development and assessment of a new predictive nomogram. Asian J Surg. (2024) 47:528–30. doi: 10.1016/j.asjsur.2023.09.093
91. Zhou, H, Lei, H, Zhao, H, Huang, K, Wang, Y, Hong, R, et al. Development and validation of a multi-parameter nomogram for venous thromboembolism in gastric cancer patients: a retrospective analysis. PeerJ. (2024) 12:e17527. doi: 10.7717/peerj.17527
92. Yu, W, Zhang, Q, Ali, M, Chen, B, Yang, Y, Wang, L, et al. A nomogram for predicting the recurrence of small bowel obstruction after gastrectomy in patients with gastric cancer. World J Surg Oncol. (2023) 21:351. doi: 10.1186/s12957-023-03197-1
93. Huang, M-B, Xu, C, Chen, H, Lin, J-X, Zheng, C-H, Chen, Q-X, et al. Development and validation of a prognostic model for postoperative anastomotic recurrence in Siewert II or III adenocarcinomas without neoadjuvant therapy in an east Asian population. J Gastrointest Cancer. (2024) 55:702–13. doi: 10.1007/s12029-023-01002-6
94. Wang, Q, Shen, K, Fei, B, Wei, M, Ge, X, and Xie, Z. Development and validation of a nomogram to predict cancer-specific survival of elderly patients with unresected gastric cancer who received chemotherapy. Sci Rep. (2024) 14:9008. doi: 10.1038/s41598-024-59516-3
95. Zuo, L, Lin, J, Ge, S, Wu, R, Liu, B, Cheng, Y, et al. Preoperative visceral fat index predicts the survival outcomes of patients with gastric cancer after surgery. Oncol Lett. (2024) 27:99. doi: 10.3892/ol.2024.14233
96. Chen, Z-R, Yang, M-F, Xie, Z-Y, Wang, P-A, Zhang, L, Huang, Z-H, et al. Risk stratification in gastric cancer lung metastasis: utilizing an overall survival nomogram and comparing it with previous staging. World J Gastrointest Surg. (2024) 16:357–81. doi: 10.4240/wjgs.v16.i2.357
97. Meng, N, Niu, X, Wu, J, Wu, H, Li, T, Yang, J, et al. Development and validation of nomogram models for predicting overall survival and cancer-specific survival in gastric cancer patients with liver metastases: a cohort study based on the SEER database. Am J Cancer Res. (2024) 14:2272–86. doi: 10.62347/ZPPK5664
98. Huang, W, Xiong, W, Tang, L, Chen, C, Yuan, Q, Zhang, C, et al. Non-invasive CT imaging biomarker to predict immunotherapy response in gastric cancer: a multicenter study. J Immunother Cancer. (2023) 11:e007807. doi: 10.1136/jitc-2023-007807
99. Deng, G, Zhu, D, Du, Z, Xue, Y, Song, H, and Li, Y. Body composition change indices combined with prognostic nutritional index predicts the clinical outcomes of patients with gastric cancer treated with immune checkpoint inhibitor. Cancer Med. (2024) 13:e7110. doi: 10.1002/cam4.7110
100. Liu, B, and Zhang, L. Geriatric nutritional risk index predicts the prognosis of gastric cancer patients treated with immune checkpoint inhibitors. Medicine (Baltimore). (2024) 103:e37863. doi: 10.1097/MD.0000000000037863
101. Li, H, Sun, Y, Wang, C, and Xue, Y. Prognostic value of fibrinogen to Prealbumin ratio (FPR) in Resectable gastric Cancer. J Inflamm Res. (2024) 17:1325–35. doi: 10.2147/JIR.S440832
102. Tian, H, Liu, Z, Zhang, Z, Zhang, L, Zong, Z, Liu, J, et al. Clinical significance of fibrinogen and platelet to pre-albumin ratio in predicting the prognosis of advanced gastric Cancer. J Inflamm Res. (2023) 16:4373–88. doi: 10.2147/JIR.S412033
103. Zhang, S, Xu, H, Li, W, Cui, J, Zhao, Q, Guo, Z, et al. Development and validation of an inflammatory biomarkers model to predict gastric cancer prognosis: a multi-center cohort study in China. BMC Cancer. (2024) 24:1. doi: 10.1186/s12885-024-12483-4
104. Sun, J-R, Kong, C-F, Ye, Y-X, Wang, Q, Qu, X-K, Jia, L-Q, et al. Integrated analysis of single-cell and bulk RNA-sequencing reveals a novel signature based on NK cell marker genes to predict prognosis and immunotherapy response in gastric cancer. Sci Rep. (2024) 14:7648. doi: 10.1038/s41598-024-57714-7
105. Ba, Z-C, Zhu, X-Q, Li, Z-G, and Li, Y-Z. Development and validation of a prognostic immunoinflammatory index for patients with gastric cancer. World J Gastroenterol. (2024) 30:3059–75. doi: 10.3748/wjg.v30.i24.3059
106. Gao, X, Li, G, Deng, J, Zhao, L, Han, W, Zhang, N, et al. Association of survival with adjuvant chemotherapy in patients with stage IB gastric cancer: a multicentre, observational, cohort study. Lancet Reg Health West Pac. (2024) 45:101031. doi: 10.1016/j.lanwpc.2024.101031
107. Dong, Q, Zhao, F, Li, Y, Song, F, Li, E, Gao, L, et al. The correlation between systemic inflammatory markers and efficiency for advanced gastric cancer patients treated with ICIs combined with chemotherapy. Immunology. (2024) 172:77–90. doi: 10.1111/imm.13759
108. Maimaiti, A, Zhou, Y, Wang, D, Zhou, Z, Pei, H, and Li, Y. Comprehensive survival nomograms for locally advanced gastric cancer: a large population-based real-world study. Transl Cancer Res. (2023) 12:2989–3006. doi: 10.21037/tcr-22-1255
109. Xu, Y, Zhang, P, Luo, Z, Cen, G, Zhang, S, Zhang, Y, et al. A predictive nomogram developed and validated for gastric cancer patients with triple-negative tumor markers. Future Oncol. (2024) 20:919–34. doi: 10.2217/fon-2023-0626
110. Zhou, D, Zeng, C, Zhang, L, Gao, X, Li, G, and Wang, X. Serum ferritin is associated with sarcopenia and predicts long-term survival for gastric cancer undergoing radical gastrectomy. Eur J Gastroenterol Hepatol. (2023) 35:1341–8. doi: 10.1097/MEG.0000000000002659
111. Zhang, J, Yin, X, Wang, H, Fang, T, Gao, J, Zhu, Z, et al. Development and validation of tumor marker indices in advanced gastric Cancer patients. Cancer Control. (2023) 30:30. doi: 10.1177/10732748231202466
112. Sun, M, Yang, Y, and Zhao, J. Establishment of a novel survival assessment and prediction model for advanced gastric cancer patients receiving immunotherapy. Oncol Lett. (2023) 26:451. doi: 10.3892/ol.2023.14038
113. Ma, Y, Wang, Y, Hu, C, Zi, M, Chen, J, Cao, M, et al. The percentages of signet-ring cells (SRCs) affects the prognosis after radical gastrectomy for advanced gastric cancer. Langenbeck's Arch Surg. (2023) 408:1. doi: 10.1007/s00423-023-03114-w
114. Zhang, S, Zheng, L, Zhang, Y, Gao, Y, Liu, L, Jiang, Z, et al. A web-based prediction model for long-term cancer-specific survival of middle-aged patients with early-stage gastric cancer: a multi-institutional retrospective study. J Cancer Res Clin Oncol. (2023) 149:16551–61. doi: 10.1007/s00432-023-05405-7
Keywords: gastric cancer, nomogram, risk prediction, disease assessment, treatment response, prognosis evaluation
Citation: Wang H, Ding Y, Zhuang M, Li K, Zhao S and Li D (2025) Application and progress of nomograms in gastric cancer. Front. Med. 12:1510742. doi: 10.3389/fmed.2025.1510742
Received: 13 October 2024; Accepted: 14 January 2025;
Published: 29 January 2025.
Edited by:
Wenlin Yang, University of Florida, United StatesReviewed by:
Shuai Chen, Affiliated Hospital of Jining Medical University, ChinaCopyright © 2025 Wang, Ding, Zhuang, Li, Zhao and Li. This is an open-access article distributed under the terms of the Creative Commons Attribution License (CC BY). The use, distribution or reproduction in other forums is permitted, provided the original author(s) and the copyright owner(s) are credited and that the original publication in this journal is cited, in accordance with accepted academic practice. No use, distribution or reproduction is permitted which does not comply with these terms.
*Correspondence: Dehong Li, bGRoODEwMTA5QDEyNi5jb20=
Disclaimer: All claims expressed in this article are solely those of the authors and do not necessarily represent those of their affiliated organizations, or those of the publisher, the editors and the reviewers. Any product that may be evaluated in this article or claim that may be made by its manufacturer is not guaranteed or endorsed by the publisher.
Research integrity at Frontiers
Learn more about the work of our research integrity team to safeguard the quality of each article we publish.