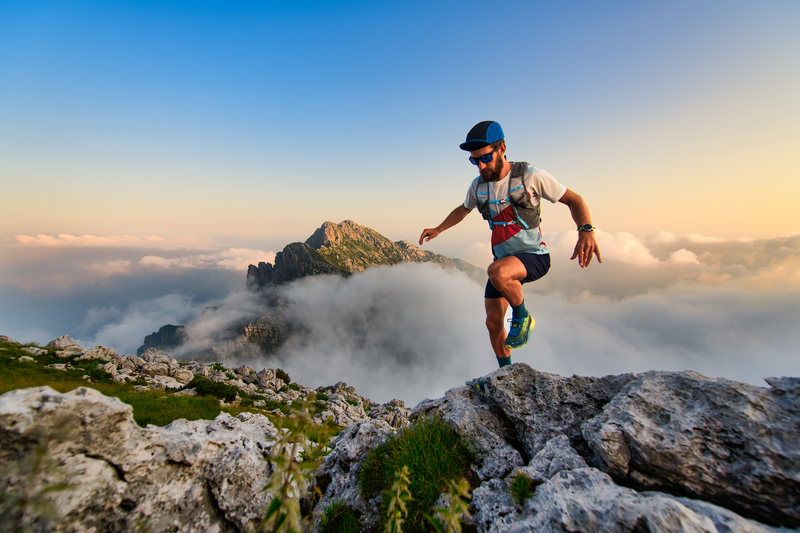
95% of researchers rate our articles as excellent or good
Learn more about the work of our research integrity team to safeguard the quality of each article we publish.
Find out more
ORIGINAL RESEARCH article
Front. Med.
Sec. Pathology
Volume 12 - 2025 | doi: 10.3389/fmed.2025.1502749
This article is part of the Research Topic Artificial Intelligence-Assisted Medical Imaging Solutions for Integrating Pathology and Radiology Automated Systems - Volume II View all 16 articles
The final, formatted version of the article will be published soon.
You have multiple emails registered with Frontiers:
Please enter your email address:
If you already have an account, please login
You don't have a Frontiers account ? You can register here
The liver is one of the vital organs of human body that performs some of the most crucial biological processes such as protein and biochemical synthesis, which is required for digestion and cleansing. A large number of patients are suffering from liver disease and hence it has become a life-threatening issue around the world. Annually, around 2 million people die because of liver disease, this accounts for around 4% of all deaths, due to factors like obesity, undiagnosed hepatitis, and excessive alcohol consumption. These factors accumulate and deteriorate the liver condition. Immediate action is necessary for timely diagnosis of the ailment before irreversible damage is done. The work aims to evaluate some of the prominent machine learning algorithms for diagnosing and predicting chronic liver disease. Also, real-world datasets often have imbalanced class distributions, causing classifiers to perform poorly, leading to low accuracy, precision, recall values and high misclassification. The Indian Patient Liver Disease (ILPD) datasets also face an imbalance issue. This work presents two hybrid models, namely SMOTEENN-KNN and SMOTEENN-AdaBoost, which can robustly handle the problem of imbalance in real-world datasets, in addition to improving the accuracy of liver disease prediction. We have also designed a hybrid model which involves the combination of Recursive Feature Elimination (RFE) for feature selection, SMOTE-ENN to tackle the problem of data imbalance and Ensemble learning for enhanced predictions. This hybrid Ensemble model outperforms the other state of art works done and gives an accuracy score of 93.2%, Brier Score loss of 0.032. The research work highlights the potential of data balancing techniques and Ensemble models to improve predictive accuracy in liver disease diagnosis.
Keywords: imbalanced data, SMOTE, SMOTEENN, SMOTE-Tomek, Logistic regression, SVM, random forest, KNN
Received: 27 Sep 2024; Accepted: 04 Apr 2025.
Copyright: © 2025 Rani, Jaiswal, ., ., Bhushan, Ullah, SINGH and Diwakar. This is an open-access article distributed under the terms of the Creative Commons Attribution License (CC BY). The use, distribution or reproduction in other forums is permitted, provided the original author(s) or licensor are credited and that the original publication in this journal is cited, in accordance with accepted academic practice. No use, distribution or reproduction is permitted which does not comply with these terms.
* Correspondence:
Manoj Diwakar, Graphic Era University, Dehradun, India
Disclaimer: All claims expressed in this article are solely those of the authors and do not necessarily represent those of their affiliated organizations, or those of the publisher, the editors and the reviewers. Any product that may be evaluated in this article or claim that may be made by its manufacturer is not guaranteed or endorsed by the publisher.
Research integrity at Frontiers
Learn more about the work of our research integrity team to safeguard the quality of each article we publish.