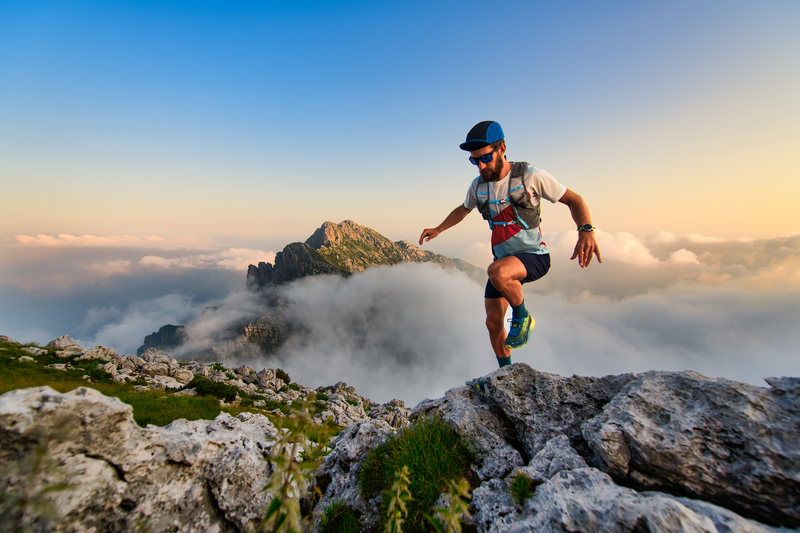
94% of researchers rate our articles as excellent or good
Learn more about the work of our research integrity team to safeguard the quality of each article we publish.
Find out more
ORIGINAL RESEARCH article
Front. Med.
Sec. Nephrology
Volume 12 - 2025 | doi: 10.3389/fmed.2025.1502189
The final, formatted version of the article will be published soon.
You have multiple emails registered with Frontiers:
Please enter your email address:
If you already have an account, please login
You don't have a Frontiers account ? You can register here
Background: Sepsis-associated kidney injury (SAKI) is a prevalent complication in intensive care unit (ICU) patients with sepsis. Diagnosis currently relies on clinical assessment, urine output, and serum creatinine levels, yet effective clinical treatments remain scarce. Our objectives are to explore prospective, targeted medications for the treatment of septic kidney injury and to employ bioinformatics to identify key genes and pathways that may be implicated in the pathogenesis of SAKI.Methods: We utilized the GEO database for differential gene screening. Related genes of septic kidney injury were identified through Pubmed2Ensembl, followed by annotation and visualization of gene ontology biological processes and KEGG pathways using DAVID. Protein-protein interactions were analyzed with the STRING database, and hub genes were identified using Cytoscape software. Candidate genes were further validated through Metascape. The CTD database was employed to uncover the relationship between hub genes and SAKI. CIBERSORT was applied to evaluate the infiltration of immune cells and their association with hub genes. Hub genes were experimentally verified through PCR detection. Lastly, the Drug-Gene Interaction Database (DGIdb) was utilized to identify drug-gene interactions.Results: Six genes, including TNF, CXCL8, IL-6, IL-1β, IL-2 and IL-10, were associated with three major signaling pathways: the COVID-19 adverse outcome pathway, an overview of proinflammatory and profibrotic mediators, and the interleukin-10 signaling pathway. Additionally, 12 targeted drugs were identified as potential therapeutic agents.
Keywords: Septic kidney injury, text mining, Drug Discovery, mRNA-miRNA coexpression networks, Immune Cell Infiltration
Received: 26 Sep 2024; Accepted: 13 Feb 2025.
Copyright: © 2025 Sun, Ding, Yang, Shen, Zhang, Song, Chen, Lin and Chen. This is an open-access article distributed under the terms of the Creative Commons Attribution License (CC BY). The use, distribution or reproduction in other forums is permitted, provided the original author(s) or licensor are credited and that the original publication in this journal is cited, in accordance with accepted academic practice. No use, distribution or reproduction is permitted which does not comply with these terms.
* Correspondence:
Rui Chen, Huazhong University of Science and Technology, Wuhan, China
Disclaimer: All claims expressed in this article are solely those of the authors and do not necessarily represent those of their affiliated organizations, or those of the publisher, the editors and the reviewers. Any product that may be evaluated in this article or claim that may be made by its manufacturer is not guaranteed or endorsed by the publisher.
Research integrity at Frontiers
Learn more about the work of our research integrity team to safeguard the quality of each article we publish.