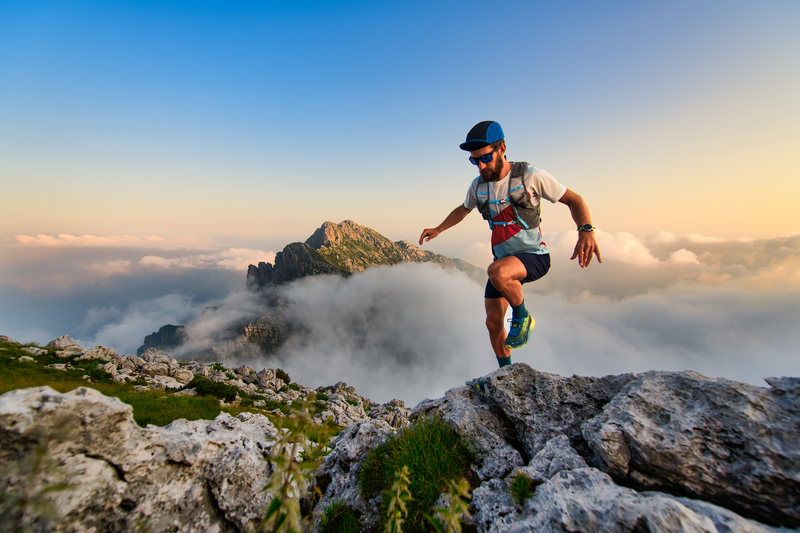
94% of researchers rate our articles as excellent or good
Learn more about the work of our research integrity team to safeguard the quality of each article we publish.
Find out more
ORIGINAL RESEARCH article
Front. Med.
Sec. Intensive Care Medicine and Anesthesiology
Volume 12 - 2025 | doi: 10.3389/fmed.2025.1501025
This article is part of the Research Topic Clinical Application of Artificial Intelligence in Emergency and Critical Care Medicine, Volume V View all 13 articles
The final, formatted version of the article will be published soon.
You have multiple emails registered with Frontiers:
Please enter your email address:
If you already have an account, please login
You don't have a Frontiers account ? You can register here
The aim of this study is to construct and validate new machine learning models to predict pneumonia events in intensive care unit (ICU) patients with acute brain injury.: Acute brain injury patients in ICU of hospitals from January 1, 2020, to December 31, 2021 were retrospective reviewed. Patients were divided into training, and validation sets. The primary outcome was nosocomial pneumonia infection during ICU stay. Machine learning models including XGBoost, DecisionTree, Random Forest, Light GBM, Adaptive Boost, BP, and TabNet were used for model derivation. The predictive value of each model was evaluated using accuracy, precision, recall, F1-score, and area under the curve (AUC), and internal and external validation was performed. Results: A total of 280 ICU patients with acute brain injury were included. Five independent variables for nosocomial pneumonia infection were identified and selected for machine learning model derivations and validations, including tracheotomy time, antibiotic use days, blood glucose, ventilator-assisted ventilation time, and C-reactive protein. The training set revealed the superior and robust performance of the XGBoost with the highest AUC value (0.956), while the Random Forest and Adaptive Boost had the highest AUC value (0.883) in validation set.Machine learning models can effectively predict the risk of nosocomial pneumonia infection in patients with acute brain injury in the ICU. Despite differences in populations and algorithms, the models we constructed demonstrated reliable predictive performance.
Keywords: Intensive Care Unit, Area under the curve, Acute brain injury, nosocomial pneumonia, Machine-learning models
Received: 24 Sep 2024; Accepted: 14 Feb 2025.
Copyright: © 2025 Pan, Bi, Yue, Ji, You, Liu, Xiong, Gu, Chen and Zhang. This is an open-access article distributed under the terms of the Creative Commons Attribution License (CC BY). The use, distribution or reproduction in other forums is permitted, provided the original author(s) or licensor are credited and that the original publication in this journal is cited, in accordance with accepted academic practice. No use, distribution or reproduction is permitted which does not comply with these terms.
* Correspondence:
Yongping You, Department of Neurosurgery, First Affiliated Hospital, Nanjing Medical University, Nanjing, China
Disclaimer: All claims expressed in this article are solely those of the authors and do not necessarily represent those of their affiliated organizations, or those of the publisher, the editors and the reviewers. Any product that may be evaluated in this article or claim that may be made by its manufacturer is not guaranteed or endorsed by the publisher.
Research integrity at Frontiers
Learn more about the work of our research integrity team to safeguard the quality of each article we publish.