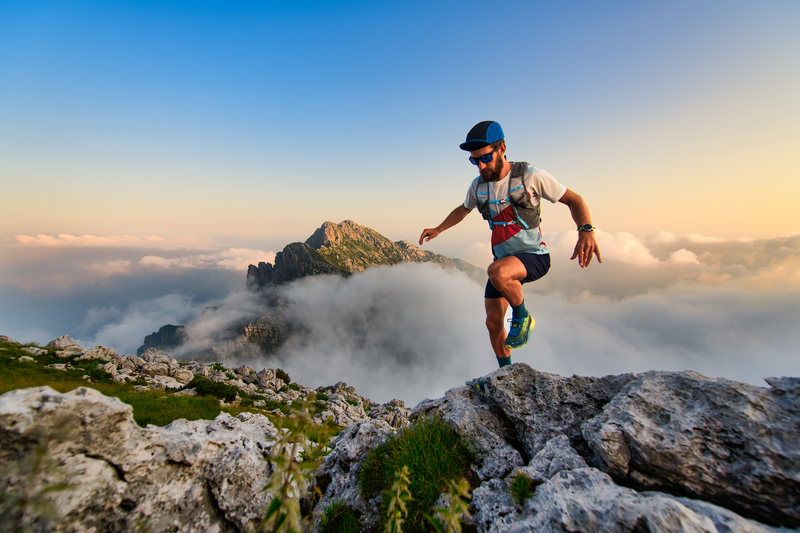
94% of researchers rate our articles as excellent or good
Learn more about the work of our research integrity team to safeguard the quality of each article we publish.
Find out more
ORIGINAL RESEARCH article
Front. Med.
Sec. Obstetrics and Gynecology
Volume 12 - 2025 | doi: 10.3389/fmed.2025.1493771
This article is part of the Research Topic A Lifecourse Perspective on Polycystic Ovary Syndrome (PCOS): Bridging Gaps in Research and Practice View all 12 articles
The final, formatted version of the article will be published soon.
You have multiple emails registered with Frontiers:
Please enter your email address:
If you already have an account, please login
You don't have a Frontiers account ? You can register here
The current study demonstrated that oxidative stress (OS) is closely related to the pathogenesis of polycystic ovary syndrome (PCOS). However, there are numerous factors that lead to OS, therefore, identifying the key genes associated with PCOS that contribute to OS is crucial for elucidating the pathogenesis of PCOS and selecting appropriate treatment strategies. Four datasets (GSE95728, GSE106724, GSE138572, and GSE145296) were downloaded from the Gene Expression Omnibus (GEO) database. GSE95728 and GSE106724 were combined to identify differentially expressed genes (DEGs) in PCOS. Weighted Gene Correlation Network Analysis (WGCNA) was used to screen key module genes associated with PCOS. Differentially expressed OS related genes (DE-OSRGs) associated with PCOS were obtained by overlapping DEGs, key module genes, and OSRGs. Subsequently, the optimal machine model was obtained to identify key genes by comparing the performance of the random forest model (RF), support vector machine model (SVM), and generalized linear model (GLM). The molecular networks were constructed to reveal the non-coding regulatory mechanisms of key genes based on GSE138572 and GSE145296. The Drug-Gene Interaction Database (DGIdb) was used to predict the potential therapeutic agents of key genes for PCOS. Finally, the expression of key OSRGs was validated by RT-PCR. In this study, 8 DE-OSRGs were identified. Based on the residuals and root mean square error of the three models, the best performance of RF was derived and 7 key genes (TNFSF10, CBL, IFNG, CP, CASP8, APOA1, and DDIT3) were identified. The GSEA enrichment analysis revealed that TNFSF10, CP, DDIT3, and INFG are all enriched in the NODlike receptor signaling pathway and natural killer cell-mediated cytotoxicity pathways. The molecular regulatory network uncovered that both TNFSF10 and CBL are regulated by noncoding RNAs. Additionally, 70 potential therapeutic drugs for PCOS were predicted, with ibuprofen associated with DDIT3 and IFNG. RT-qPCR validation confirmed the expression trends of key genes IFNG, DDIT3, and APOA1 were consistent with the dataset, and the observed differences were statistically significant (P < 0.05). The identification of seven key genes and molecular regulatory networks through bioinformatics analysis is of great significance for exploring the pathogenesis and therapeutic strategies of PCOS.
Keywords: Polycystic Ovary Syndrome, oxidative stress-related genes, machine learning, key genes, Drug prediction
Received: 09 Sep 2024; Accepted: 12 Feb 2025.
Copyright: © 2025 Li, Liu, Liu, Zheng, Chen, Du and Ye. This is an open-access article distributed under the terms of the Creative Commons Attribution License (CC BY). The use, distribution or reproduction in other forums is permitted, provided the original author(s) or licensor are credited and that the original publication in this journal is cited, in accordance with accepted academic practice. No use, distribution or reproduction is permitted which does not comply with these terms.
* Correspondence:
Qinhua Li, Yichang Central People's Hospital, Yichang, China
Lei Liu, The First Clinical Medical College of China Three Gorges University, Yichang, 443000, Hubei Province, China
Yuhan Liu, The First Clinical Medical College of China Three Gorges University, Yichang, 443000, Hubei Province, China
Tingting Zheng, The First Clinical Medical College of China Three Gorges University, Yichang, 443000, Hubei Province, China
Ningjing Chen, The First Clinical Medical College of China Three Gorges University, Yichang, 443000, Hubei Province, China
Peiyao Du, The First Clinical Medical College of China Three Gorges University, Yichang, 443000, Hubei Province, China
Hong Ye, The First Clinical Medical College of China Three Gorges University, Yichang, 443000, Hubei Province, China
Disclaimer: All claims expressed in this article are solely those of the authors and do not necessarily represent those of their affiliated organizations, or those of the publisher, the editors and the reviewers. Any product that may be evaluated in this article or claim that may be made by its manufacturer is not guaranteed or endorsed by the publisher.
Research integrity at Frontiers
Learn more about the work of our research integrity team to safeguard the quality of each article we publish.