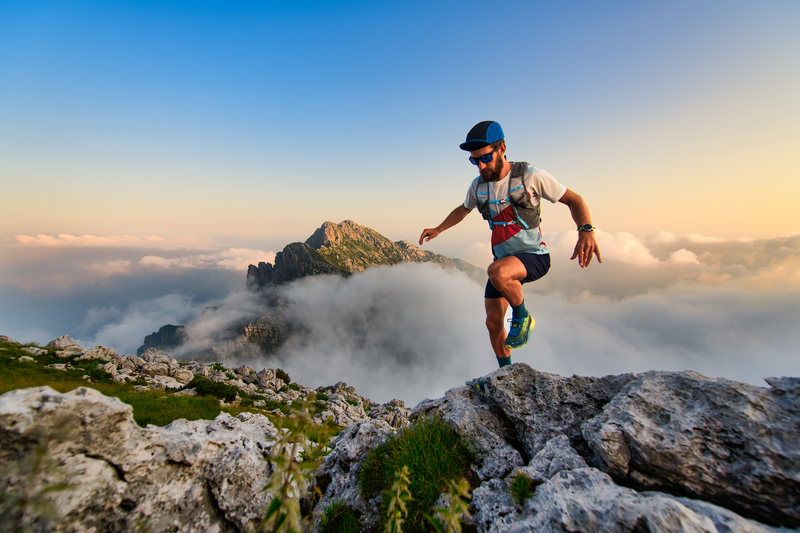
95% of researchers rate our articles as excellent or good
Learn more about the work of our research integrity team to safeguard the quality of each article we publish.
Find out more
ORIGINAL RESEARCH article
Front. Med.
Sec. Translational Medicine
Volume 12 - 2025 | doi: 10.3389/fmed.2025.1475362
The final, formatted version of the article will be published soon.
You have multiple emails registered with Frontiers:
Please enter your email address:
If you already have an account, please login
You don't have a Frontiers account ? You can register here
Decalcification is crucial in enhancing the diagnostic accuracy and interpretability of cardiac CT images, particularly in cardiovascular imaging. Calcification in the coronary arteries and cardiac structures can significantly impact the quality of the images and hinder precise diagnostics. This study introduces a novel approach, Hybrid Models for Decalcify Cardiac CT (HMDC), aimed at enhancing the clarity of cardiac CT images through effective decalcification. Decalcification is critical in medical imaging, especially in cardiac CT scans, where calcification can hinder accurate diagnostics. The proposed HMDC leverages advanced deep-learning techniques and traditional image-processing methods for efficient and robust decalcification. The experimental results demonstrate the superior performance of HMDC, achieving an outstanding accuracy of 97.22%, surpassing existing decalcification methods. The hybrid nature of the model harnesses the strengths of both deep learning and traditional approaches, leading to more transparent and more diagnostically valuable cardiac CT images. The study underscores the potential impact of HMDC in improving the precision and reliability of cardiac CT diagnostics, contributing to advancements in cardiovascular healthcare. This research introduces a cutting-edge solution for decalcifying cardiac CT images and sets the stage for further exploration and refinement of hybrid models in medical imaging applications. The implications of HMDC extend beyond decalcification, opening avenues for innovation and improvement in cardiac imaging modalities, ultimately benefiting patient care and diagnostic accuracy.
Keywords: deep learning, machine learning, Cardiac Classification, Decalcification, Deep convolutional neural networks (CNN), Densenet, Ensemble models
Received: 03 Aug 2024; Accepted: 03 Apr 2025.
Copyright: © 2025 N, R, N, Kaliappan, Yahya, Samikannu, Badruddin, Kamangar and Hussien. This is an open-access article distributed under the terms of the Creative Commons Attribution License (CC BY). The use, distribution or reproduction in other forums is permitted, provided the original author(s) or licensor are credited and that the original publication in this journal is cited, in accordance with accepted academic practice. No use, distribution or reproduction is permitted which does not comply with these terms.
* Correspondence:
Vishnu Kumar Kaliappan, KPR Institute of Engineering and Technology, Coimbatore, Coimbatore, India
Disclaimer: All claims expressed in this article are solely those of the authors and do not necessarily represent those of their affiliated organizations, or those of the publisher, the editors and the reviewers. Any product that may be evaluated in this article or claim that may be made by its manufacturer is not guaranteed or endorsed by the publisher.
Research integrity at Frontiers
Learn more about the work of our research integrity team to safeguard the quality of each article we publish.