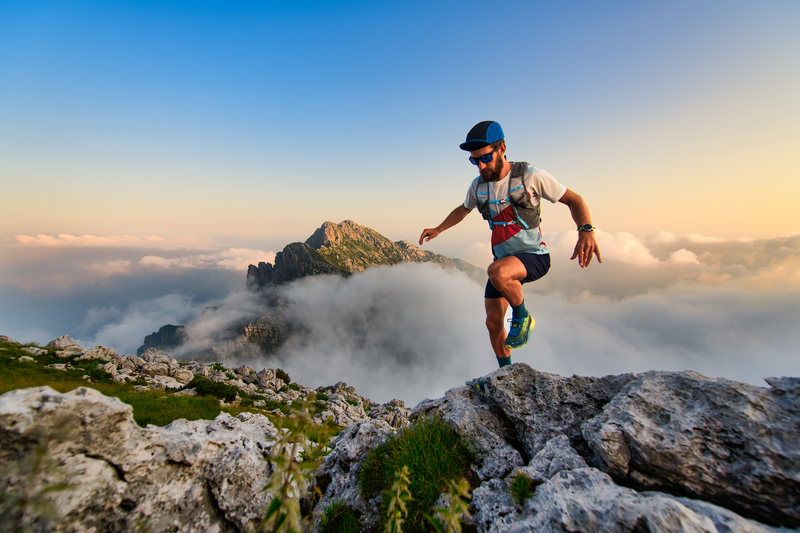
95% of researchers rate our articles as excellent or good
Learn more about the work of our research integrity team to safeguard the quality of each article we publish.
Find out more
ORIGINAL RESEARCH article
Front. Med. , 26 March 2025
Sec. Pulmonary Medicine
Volume 12 - 2025 | https://doi.org/10.3389/fmed.2025.1472295
This article is part of the Research Topic Discerning active TB from latent infection View all 6 articles
Background: A prediction model is hereby developed to identify poor treatment outcomes during the intensive phase in patients with initial treatment of pulmonary tuberculosis (TB).
Methods: The data of inpatients with pulmonary TB were collected from a tertiary hospital located in Southeastern China from July 2019 to December 2023. The included patients were divided into the modeling group and the validation group. The outcome indicator was based on a comparison of pulmonary CT findings before and after the two-month intensive phase of anti-TB treatment. In the modeling group, the independent risk factors of pulmonary TB patients were obtained through logistic regression analysis and then a prediction model was established. The discriminative ability (the area under the curve of the receiver operating characteristic, AUC), its calibration (GiViTI calibration chart), and its clinical applicability (decision curve analysis, DCA) were respectively evaluated. In addition, the prediction effectiveness was compared with that of the machine learning model.
Results: A total of 1,625 patients were included in this study, and 343 patients had poor treatment outcomes in the intensive phase of anti-TB treatment. Logistic regression analysis identified several independent risk factors for poor treatment outcomes, including diabetes, cavities in the lungs, tracheobronchial TB, increased C-reactive protein, and decreased hemoglobin. The AUC values were 0.815 for the modeling group and 0.851 for the validation group. In the machine learning models, the AUC values of the random forest model and the integrated model were 0.821 and 0.835, respectively.
Conclusion: The prediction model established in this study presents good performance in predicting poor treatment outcomes during the intensive phase in patients with pulmonary TB.
In 2022, there were 10.6 million new tuberculosis (TB) cases worldwide, with an incidence rate of 133 per 100,000 people. China ranks third among the 30 high-burden TB countries, accounting for 7.1% of the global incidence. At present, the success rate of initial treatment of pulmonary TB is about 85% (1). Pulmonary TB treatment can be divided into the intensive phase and the continuous phase. Treatment interruptions frequently occur during the intensive phase (2, 3). Additionally, most adverse treatment outcomes in pulmonary TB inpatients also occur during this phase (4–6). Therefore, the intensive phase of treatment is critical for the final prognosis for pulmonary TB patients, resulting into the urgent requirement of earlier detection of treatment outcomes.
At present, the treatment effect of TB patients can be evaluated if they have turned negative on sputum smear and culture results at the end of intensive treatment phase. However, the above methods cannot be used as indicators for assessing the treatment effect of patients with bacillus-negative pulmonary TB in clinical practice (7). Additionally, for some patients with smear-positive pulmonary TB, false-negative results may exist in sputum smear for acid-fast bacilli at the end of the intensive treatment phase. This can occur due to quality problems with the randomly inspected sputum (8). Therefore, it is impossible to comprehensively evaluate the effect in the intensive treatment phase only via the sputum smear. Bacterial culture has the disadvantage of a long incubation time (negative results in liquid culture require more than 42 days) and may not yield results in cases of culture contamination (9). Consequently, the effectiveness of anti-tuberculosis treatment monitoring through direct pathogenetic testing remains challenging.
On the other hand, pulmonary imaging is fast and feasible to visualize the treatment effect. It can be used in the diagnosis and follow-up of pulmonary TB, especially in the continuous monitoring of the prognosis of patients with bacterial-negative pulmonary TB (10). Pulmonary imaging examination mainly includes chest X-ray (CXR) and lung computed tomography (CT). CXR is helpful for the rapid and cost-effective early diagnosis of TB, but using CXR to monitor the prognosis of TB is difficult (11). Because of its high resolution, pulmonary CT is significantly better than CXR in observing the absorption of pulmonary lesions during the entire treatment process of pulmonary TB. It is a highly sensitive tool for tracking the treatment efficacy among pulmonary TB patients (12), especially in the early intensive treatment phase (13).
Few prediction models use pulmonary CT as an outcome indicator to predict the effect of early anti-TB treatment in the intensive phase. Nijiati et al. developed several machine learning prediction models, but the prediction efficiency is not adequate based on the area under the curve of the receiver operating characteristic (AUC) values (13, 14). Moreover, there are fewer clinical indicators included in these prospectives studies, and machine-learning models show deficiencies in the clinical practice (15).
Thereby, it is essential to quickly screen pulmonary TB patients who have poor treatment outcomes during the intensive phase. The changes of pulmonary CT results before and after the intensive treatment phase were adopted in this study as the outcome indicators. Thus, developing such a model enables clinicians to find pulmonary TB patients with poor treatment efficacy as early as possible, enabling timely intervention.
Data from all inpatients in this study were extracted from the medical record information mining database of the affiliated Dongyang Hospital of Wenzhou Medical University. This database was constructed with the technical support of Le 9 Health Science and Technology Co. Ltd. All personal identification information was removed from the medical records.
Inclusion criteria: 1. Patients clinically diagnosed with pulmonary tuberculosis according to the Chinese Diagnostic Criteria for Pulmonary Tuberculosis (WS 288-2017). 2. The patients receiving anti-TB treatment using isoniazid, rifampicin, pyrazinamide and ethambutol (HRZE) for a two-month intensive phase.
Exclusion criteria: 1. Patients under 18 years old; 2. Patients with incomplete data; 3. Pregnant patients; 4. Patients with AIDS; 5. Patients who did not complete the intensive treatment phase (i.e., anti-TB treatment duration less than two months).
According to the above inclusion and exclusion criteria, patients who were first diagnosed with pulmonary TB in our hospital between July 2019 and December 2023 were finally included in this retrospective study, and all variables involved in the prediction model were collected at the time of the patients’ initial admission to the hospital.
The variables included gender, age and the levels of the following indicators in the first examination after admission: creatinine, C-reactive protein (CRP), white blood cells, hemoglobin, platelet, total bilirubin, albumin, alanine aminotransferase, aspartate aminotransferase, total cholesterol, triglyceride, high-density lipoprotein cholesterol (HDL) and low-density lipoprotein cholesterol (LDL). Moreover, the information on smoking history, alcohol consumption history, medical history of diabetes, tumor, hypertension, liver disease and chronic obstructive pulmonary disease were enrolled. The presences of pulmonary cavity or tracheobronchial tuberculosis (TBTB) were identified in pulmonary CT images. The primary outcome indicator was the treatment efficacy by pulmonary CT examination after two months of intensive anti-TB therapy. If the pulmonary CT shows an increase in lung lesions compared to the pre-treatment examination results, it indicates the poor treatment effect.
The statistical analysis in this study was done using R (version 4.2.2). The continuous variables conforming to the normal distribution were expressed as Mean ± SD and analyzed with Student’s t-test. The continuous variables conforming to non-normal distribution were expressed as the median and quartile ranges and analyzed by Mann–Whitney U-test. The categorical variable was expressed in number (percentage) and analyzed by chi square test. p < 0.05 indicated statistical significance.
The enrolled patients were divided into the modeling group and validation group at a ratio of 7:3 using “createDataPartition” function in the “caret” package. The “twogrps” function in the “CBCgrps” package was employed to detect significant differences of baseline characteristics between the modeling group and the validation group. Univariate analysis was conducted in the modeling group to screen the risk factors associated with poor treatment outcomes. The “boxTidwell” function in the “car” package was adopted to determine whether the continuous variables were linearly associated using logitp (p > 0.05). The “VIF” function was used for multicollinearity test. If the value of variance inflation factors (VIFs) was less than 5, no significant collinearity was considered. Regarding variables meeting the requirements of linear relation to logitP and no multicollinearity between included variables, a multivariable logistic regression was conducted to obtain independent risk factors for modeling. Finally, the “regplot” package was utilized to draw a nomogram to display the model. The model was evaluated from three aspects: discrimination, calibration and clinical applicability. The discrimination ability of the prediction model refers to its ability to effectively distinguish the poor treatment outcomes of pulmonary TB patients in the intensive phase, which is evaluated by AUC. Higher AUC values indicate better discrimination ability of the model. The calibration of this model was performed using the calibration chart, and the high degree of overlap between the fitting curve and the standard curve indicated the high goodness of fit. The DCA curve was applied to evaluate the clinical applicability of the model. The farther the established DCA curve is away from the two extreme curves (All curve and None curve), the better clinical applicability it indicates.
Finally, the logistic regression model established in this study was compared with the machine learning model. In the machine learning model, the methods of random forest (“randomForest” package), support vector machine (SVM, “kernlab” package), extreme gradient boosting (Xgboost, “xgboost” package) and decision tree (“rpart” package) were set by default parameters to build models. Subsequently, these four machine learning methods were integrated through stacking to establish the ensemble model (16–18), and the DeLong test was used to compare the discrimination ability between the logistic regression model and different machine learning models. A p value less than 0.05 indicated a significant difference in the comparison.
During the period from July 2019 to December 2023, a total of 2,182 inpatients with pulmonary TB received first line anti-TB protocol. Out of these patients, 557 were excluded, including 59 patients younger than 18 years old, 15 HIV patients, 8 pregnant patients, 381 patients with incomplete data, and 94 patients who did not finalize treatment. Finally, 1,625 patients were included in the study (Figure 1). Among the subjects included, 343 patients (21%) had poor treatment outcomes in the intensive phase. There were 1,138 cases in the modeling group (253 cases with poor treatment outcome) and 487 cases in the validation group (90 cases with poor treatment outcome). No significant difference in baseline characteristics between the two groups was detected (p > 0.05, Table 1). In the modeling group, the univariate analysis showed that the ten variables (CRP, white blood cells, hemoglobin, platelet, albumin, HDL, diabetes, tumor, pulmonary disease, and TBTB) were correlated with poor prognosis (p < 0.05, Table 2). The included variables were linear to the logitP (p > 0.05, Supplementary Table S1). No multicollinearity existed as the VIF values of all variables were less than 5 (Supplementary Table S2).
Table 2. Univariate analysis between successful treatment and unsuccessful treatment in modeling groupa.
The final logistic regression analysis results demonstrated increased CRP (OR 1.014), declined hemoglobin (OR 0.979), having diabetes mellitus (OR 2.159), having pulmonary cavity (OR 2.707), and having TBTB (OR 2.628) were independent risk factors related to poor treatment outcomes in the intensive phase of pulmonary TB treatment (Table 3), and were included in the model.
Table 3. Logistic regression analysis of independent risk factors for unsuccessful treatment in patients with pulmonary tuberculosis.
This model was visualized as a personalized nomogram (Figure 2). To use the nomogram, a vertical line was drawn from each variable upwards to the top scoring line and the corresponding points were recorded. Then the scores of the corresponding points of each variable were summed up to calculate the total score, based on which the prediction probability of corresponding poor treatment outcomes at the bottom of the nomogram was finally obtained. For example, the variables of one pulmonary TB patient at admission were as follows: CRP 128.1 (mg/L), hemoglobin 96 (g/L), accompanied by diabetes, lung cavities and TBTB. The patient’s total score was 4.8, and the corresponding predicted probability of poor treatment outcome was 0.93 (Figure 2).
Figure 2. The nomogram of established model for predicting poor treatment risk in pulmonary tuberculosis patients during hospitalization. The enrolled variables were collected for the first time after admission. A patient was displayed as an example, with detailed enrolled variables labelled by red dots. The variables labelled of asterisk indicated significance in the model, *, p < 0.05, **, p < 0.01, ***, p < 0.001.
The AUC of the logistic regression model in the modeling group was 0.815 (95CI: 0.782–0.849) (Figure 3A), showing good discrimination ability; the p value of the calibration chart was 0.708, with Brier scaled score of 0.118, calibration slope of 1.000, and R2 of 0.373, indicating the good fit (Figure 3B). The DCA curve was far away from the two extreme curves, indicating its good clinical applicability (Figure 3C). The AUC in the validation group was 0.851 (95CI: 0.799–0.904) (Figure 4A); the p value of the calibration chart was 0.568, with Brier scaled score of 0.090, calibration slope of 1.000, R2 of 0.453 (Figure 4B); the DCA curve was far away from the two extreme curves (Figure 4C), suggesting that the prediction model performed well in discrimination, goodness of fit and clinical applicability in the validation group.
Figure 3. Evaluation of logistic model in the modeling group. (A) ROC curves. (B) Calibration curves. (C) Decision-curve analysis.
Figure 4. Evaluation of logistic model in the validation group. (A) ROC curves. (B) Calibration curves. (C) Decision-curve analysis.
The AUC values of machine learning models in the validation group were as follows: random forest (0.821), SVM (0.759), Xgboost (0.795), decision tree (0.690), and integrated machine learning model (0.835) (Figure 5). The discriminative ability of the logistic regression model was significantly higher than that of the models established by SVM, Xgboost and decision tree, but equivalent to that of the models established by random forest and integrated models (Supplementary Table S3).
Figure 5. ROC curves for the logistic model and five machine learning models to predict poor treatment risk. SVM, Support Vector Machine; Xgboost, extreme gradient boosting.
In this study, a clinical prediction model for poor treatment outcomes in the intensive phase in patients with initial pulmonary TB treatment was established. There were five clinical indicators enrolled in this model, including the presence of diabetes, lung cavities, TBTB, declined hemoglobin, and increased CRP. The model established in this study performs well in terms of discrimination, calibration and clinical applicability. This nomogram prediction model could be used as an effective tool for predicting and screening the treatment outcomes in the intensive phase.
The model developed in this study shows that the presence of diabetes in pulmonary TB inpatients is a risk factor for the poor treatment outcomes in the intensive phase. Diabetes mellitus is an important risk factor for TB, which can increase TB incidence and affect patient treatment response (19). Studies have shown that diabetes is associated with an increased risk of adverse outcomes in pulmonary TB. Therefore, there is an urgent need to screen for diabetes in TB patients and to implement interventions to improve the outcomes of pulmonary TB patients combined with diabetes (20–22). Intrapulmonary lesion resorption is better in TB patients with effective glycemic control compared with that in patients without effective glycemic control (23). The presence of lung cavities is an important factor for poor prognosis, diseases recurrence, and drug resistance development in pulmonary TB patients. The presence of lung cavities can hinder the penetration of anti-TB drugs into the lesions due to poor vascularization and necrotic tissue, thereby reducing treatment effectiveness (24, 25). The present study indicated that the presence of pulmonary cavities in baseline information before anti-TB treatment was a risk factor of poor treatment outcomes, which is consistent with previous studies (21, 26). Poor glycemic control further exacerbates the immune dysfunction of pulmonary TB patients and makes them more susceptible to lung cavities, which, in turn, increases the risk of treatment failure (27, 28).
TB is a chronic consumptive infectious disease, which will lead to the decrease of hemoglobin level and anemia. A multicenter cohort study showed that the frequency of adverse outcomes of pulmonary TB treatment increased with the severity of anemia (29). Although hemoglobin levels increase with the success of anti-TB treatment, the clinical recovery of anemic TB patients is slower during the intensive phase of treatment than that of non-anemic patients (30). The present research proved that the decrease in hemoglobin level can be a powerful predictor for treatment failure in the intensive phase of TB patients, and the severity of anemia was proportional to the risk of treatment failure. In addition, a feature of pulmonary TB is systemic inflammation. Previous studies have discovered that CRP can be used as a biomarker for evaluating the severity and treatment effect of TB (31, 32). The change of CRP may assist in evaluating the response of anti-TB treatment in the early stage, and identifying patients with increased risk of adverse outcomes. Compared with that of cured patients, the baseline CRP level in patients with failed outcomes is significantly higher (33, 34). This study showed that elevated CRP is an important predictor for poor treatment outcomes of pulmonary TB patients in the intensive phase.
It is reported that about 10–40% of pulmonary TB patients suffer from TBTB, and TBTB lesions often damage the tracheobronchial wall, resulting in its necrosis and tracheobronchial stenosis. Long-term bronchial stenosis, twisting and deformation can trigger local ventilation and blood flow dysfunction, which may lead to intractable TB lowering the effectiveness of the treatment, and even cause death (35, 36). The presence of TBTB in patients was hereby shown as a risk factor for poor treatment outcomes in the intensive phase. Early diagnosis of TBTB through radiological imaging and bronchoscopy, timely anti-TB treatment and interventional treatment under bronchoscope can reduce the risk of further aggravation of bronchial stenosis, and preserve the pulmonary ventilation function as much as possible to improve the prognosis (37).
A previous study indicated that machine learning models could accurately predict the treatment outcome of pulmonary TB patients (14, 16, 38). Therefore, several machine learning models were also hereby established to compare its effectiveness with the logical regression model in this study. The results suggested that the discriminative ability of logistic regression model was significantly higher than that of SVM, Xgboost and decision tree models. However, the efficiency was comparable to that of random forest and integrated models. Nevertheless, machine learning models often face challenges in clinical interpretation, making them difficult to popularize and apply in actual clinical practice (15, 39). The discrimination ability of the developed logistic regression model is not inferior to that of the machine learning model, but it is easier to interpret clinically. Therefore, this model can be better popularized and applied in the clinic.
Limitations of this study: (1) The data included in this study were from a single center. The patients from other regions might have different clinical features, resulting into different enrolled variables in the prediction model and varied prediction efficiency. Therefore, multi-center studies were required to validate our findings in the future. (2) Some patients were not included in this study due to information loss, which might bring a bias in patient population.
In this study, a prediction model was established to evaluate the risk of poor treatment outcomes in pulmonary tuberculosis patients during the intensive phase of treatment. The model performed well and can assist clinicians in implementing more targeted interventions to improve treatment success rates in pulmonary tuberculosis patients.
The original contributions presented in the study are included in the article/Supplementary material, further inquiries can be directed to the corresponding authors.
The studies involving humans were approved by Ethics Committee of Affiliated Dongyang Hospital of Wenzhou Medical University. The studies were conducted in accordance with the local legislation and institutional requirements. Written informed consent for participation was not required from the participants or the participants’ legal guardians/next of kin in accordance with the national legislation and institutional requirements.
BL: Data curation, Funding acquisition, Investigation, Software, Writing – original draft, Writing – review & editing. YS: Conceptualization, Resources, Validation, Writing – original draft, Writing – review & editing. MW: Data curation, Resources, Writing – original draft, Writing – review & editing. CJ: Investigation, Software, Writing – original draft, Writing – review & editing. CL: Data curation, Validation, Writing – original draft, Writing – review & editing. XP: Conceptualization, Writing – original draft, Writing – review & editing. XC: Conceptualization, Writing – original draft, Writing – review & editing.
The author(s) declare that financial support was received for the research and/or publication of this article. This study was supported by the Public Science and Technology Research Project of Jinhua (grant No. 2022-4-262) and the Zhejiang Provincial Medical and Health Science and Technology Plan (grant No. 2024XY083).
The authors thank the Le9 Health platform for the technical support, by which the clinical data of patients with pulmonary tuberculosis in the hospital was collected efficiently and accurately.
The authors declare that the research was conducted in the absence of any commercial or financial relationships that could be construed as a potential conflict of interest.
All claims expressed in this article are solely those of the authors and do not necessarily represent those of their affiliated organizations, or those of the publisher, the editors and the reviewers. Any product that may be evaluated in this article, or claim that may be made by its manufacturer, is not guaranteed or endorsed by the publisher.
The Supplementary material for this article can be found online at: https://www.frontiersin.org/articles/10.3389/fmed.2025.1472295/full#supplementary-material
1. World Health Organization. Global tuberculosis report 2023. Geneva: World Health Organization (2023).
2. Oh, AL, Makmor-Bakry, M, Islahudin, F, Ting, CY, Chan, SK, and Tie, ST. Characteristics, predictors and consequences of tuberculosis treatment interruption: a multicentre retrospective cohort study. Trop Med Int Health. (2024) 29:434–45. doi: 10.1111/tmi.13987
3. Kimani, E, Muhula, S, Kiptai, T, Orwa, J, Odero, T, and Gachuno, O. Factors influencing TB treatment interruption and treatment outcomes among patients in Kiambu County, 2016-2019. PLoS One. (2021) 16:e0248820. doi: 10.1371/journal.pone.0248820
4. Sariem, CN, Odumosu, P, Dapar, MP, Musa, J, Ibrahim, L, and Aguiyi, J. Tuberculosis treatment outcomes: a fifteen-year retrospective study in Jos-north and Mangu, plateau state, north - Central Nigeria. BMC Public Health. (2020) 20:1224. doi: 10.1186/s12889-020-09289-x
5. Alemu, A, Bitew, ZW, and Worku, T. Poor treatment outcome and its predictors among drug-resistant tuberculosis patients in Ethiopia: a systematic review and meta-analysis. Int J Infect Dis. (2020) 98:420–39. doi: 10.1016/j.ijid.2020.05.087
6. Baumann, R, Kaempfer, S, Chegou, NN, Nene, NF, Veenstra, H, Spallek, R, et al. Serodiagnostic markers for the prediction of the outcome of intensive phase tuberculosis therapy. Tuberculosis (Edinb). (2013) 93:239–45. doi: 10.1016/j.tube.2012.09.003
7. Kwak, SH, Choi, JS, Lee, EH, Lee, SH, Leem, AY, Lee, SH, et al. Characteristics and risk factors associated with missed diagnosis in patients with smear-negative pulmonary tuberculosis. Korean J Intern Med. (2021) 36:S151–s159. doi: 10.3904/kjim.2019.435
8. Sinshaw, W, Kebede, A, Bitew, A, Tesfaye, E, Tadesse, M, Mehamed, Z, et al. Prevalence of tuberculosis, multidrug resistant tuberculosis and associated risk factors among smear negative presumptive pulmonary tuberculosis patients in Addis Ababa, Ethiopia. BMC Infect Dis. (2019) 19:641. doi: 10.1186/s12879-019-4241-7
9. Riou, C, du Bruyn, E, Kim, GHJ, da Costa, I, Lee, J, Sher, A, et al. Derivation of a high-resolution CT-based, semi-automated radiographic score in tuberculosis and its relationship to bacillary load and antitubercular therapy. Eur Respir J. (2023) 62:2300600. doi: 10.1183/13993003.00600-2023
10. Suárez, I, Fünger, SM, Kröger, S, Rademacher, J, Fätkenheuer, G, and Rybniker, J. The diagnosis and treatment of tuberculosis. Dtsch Arztebl Int. (2019) 116:729–35. doi: 10.3238/arztebl.2019.0729
11. Natarajan, S, Sampath, P, Arunachalam, R, Shanmuganathan, V, Dhiman, G, Chakrabarti, P, et al. Early diagnosis and meta-agnostic model visualization of tuberculosis based on radiography images. Sci Rep. (2023) 13:22803. doi: 10.1038/s41598-023-49195-x
12. Skoura, E, Zumla, A, and Bomanji, J. Imaging in tuberculosis. Int J Infect Dis. (2015) 32:87–93. doi: 10.1016/j.ijid.2014.12.007
13. Nijiati, M, Guo, L, Tuersun, A, Damola, M, Abulizi, A, Dong, J, et al. Deep learning on longitudinal CT scans: automated prediction of treatment outcomes in hospitalized tuberculosis patients. iScience. (2023) 26:108326. doi: 10.1016/j.isci.2023.108326
14. Nijiati, M, Guo, L, Abulizi, A, Fan, S, Wubuli, A, Tuersun, A, et al. Deep learning and radiomics of longitudinal CT scans for early prediction of tuberculosis treatment outcomes. Eur J Radiol. (2023) 169:111180. doi: 10.1016/j.ejrad.2023.111180
15. Kelly, CJ, Karthikesalingam, A, Suleyman, M, Corrado, G, and King, D. Key challenges for delivering clinical impact with artificial intelligence. BMC Med. (2019) 17:195. doi: 10.1186/s12916-019-1426-2
16. Kheirandish, M, Catanzaro, D, Crudu, V, and Zhang, S. Integrating landmark modeling framework and machine learning algorithms for dynamic prediction of tuberculosis treatment outcomes. J Am Med Inform Assoc. (2022) 29:900–8. doi: 10.1093/jamia/ocac003
17. Orjuela-Cañón, AD, Jutinico, AL, Awad, C, Vergara, E, and Palencia, A. Machine learning in the loop for tuberculosis diagnosis support. Front Public Health. (2022) 10:876949. doi: 10.3389/fpubh.2022.876949
18. Zhang, Z, Chen, L, Xu, P, and Hong, Y. Predictive analytics with ensemble modeling in laparoscopic surgery: a technical note. Laparoscopic Endoscopic Robotic Surg. (2022) 5:25–34. doi: 10.1016/j.lers.2021.12.003
19. Dooley, KE, and Chaisson, RE. Tuberculosis and diabetes mellitus: convergence of two epidemics. Lancet Infect Dis. (2009) 9:737–46. doi: 10.1016/S1473-3099(09)70282-8
20. Arriaga, MB, Araújo-Pereira, M, Barreto-Duarte, B, Nogueira, B, Freire, MVCNS, Queiroz, ATL, et al. The effect of diabetes and prediabetes on Antituberculosis treatment outcomes: a multicenter prospective cohort study. J Infect Dis. (2022) 225:617–26. doi: 10.1093/infdis/jiab427
21. Koo, HK, Min, J, Kim, HW, Lee, J, Kim, JS, Park, JS, et al. Prediction of treatment failure and compliance in patients with tuberculosis. BMC Infect Dis. (2020) 20:622. doi: 10.1186/s12879-020-05350-7
22. Ridolfi, F, Peetluk, L, Amorim, G, Turner, M, Figueiredo, M, Cordeiro-Santos, M, et al. Tuberculosis treatment outcomes in Brazil: different predictors for each type of unsuccessful outcome. Clin Infect Dis. (2023) 76:e930–7. doi: 10.1093/cid/ciac541
23. Song, C, Xie, W, Gong, L, Ren, M, Pan, P, and Luo, B. The relationship between HbA1c control levels and antituberculosis treatment effects: a meta-analysis. J Chin Med Assoc. (2019) 82:915–21. doi: 10.1097/JCMA.0000000000000205
24. Urbanowski, ME, Ordonez, AA, Ruiz-Bedoya, CA, Jain, SK, and Bishai, WR. Cavitary tuberculosis: the gateway of disease transmission. Lancet Infect Dis. (2020) 20:e117–28. doi: 10.1016/S1473-3099(20)30148-1
25. Peloquin, CA, and Davies, GR. The treatment of tuberculosis. Clin Pharmacol Ther. (2021) 110:1455–66. doi: 10.1002/cpt.2261
26. Gopalan, N, Srinivasalu, VA, Chinnayan, P, Velayutham, B, Bhaskar, A, Santhanakrishnan, R, et al. Predictors of unfavorable responses to therapy in rifampicin-sensitive pulmonary tuberculosis using an integrated approach of radiological presentation and sputum mycobacterial burden. PLoS One. (2021) 16:e0257647. doi: 10.1371/journal.pone.0257647
27. Wei, R, Li, P, Xue, Y, Liu, Y, Gong, W, and Zhao, W. Impact of diabetes mellitus on the immunity of tuberculosis patients: a retrospective, Cross-Sectional Study. Risk Manag Healthc Policy. (2022) 15:611–27. doi: 10.2147/RMHP.S354377
28. Meng, F, Lan, L, Wu, G, Ren, X, Yuan, X, Yang, M, et al. Impact of diabetes itself and glycemic control status on tuberculosis. Front Endocrinol. (2023) 14:1250001. doi: 10.3389/fendo.2023.1250001
29. Araújo-Pereira, M, Nogueira, BMF, Spener-Gomes, R, Carvalho, ACC, Sant’Anna, FM, Figueiredo, MC, et al. Anemia and anti-tuberculosis treatment outcome in persons with pulmonary tuberculosis: a multi-center prospective cohort study. J Infect Public Health. (2023) 16:974–80. doi: 10.1016/j.jiph.2023.04.009
30. Ashenafi, S, Bekele, A, Aseffa, G, Amogne, W, Kassa, E, Aderaye, G, et al. Anemia is a strong predictor of wasting, disease severity, and progression, in clinical tuberculosis (TB). Nutrients. (2022) 14:3318. doi: 10.3390/nu14163318
31. Sigal, GB, Segal, MR, Mathew, A, Jarlsberg, L, Wang, M, Barbero, S, et al. Biomarkers of tuberculosis severity and treatment effect: a directed screen of 70 host markers in a randomized clinical trial. EBioMedicine. (2017) 25:112–21. doi: 10.1016/j.ebiom.2017.10.018
32. Zimmer, AJ, Lainati, F, Aguilera Vasquez, N, Chedid, C, McGrath, S, Benedetti, A, et al. Biomarkers that correlate with active pulmonary tuberculosis treatment response: a systematic review and Meta-analysis. J Clin Microbiol. (2022) 60:e0185921. doi: 10.1128/jcm.01859-21
33. Wilson, D, Moosa, MYS, Cohen, T, Cudahy, P, Aldous, C, and Maartens, G. Evaluation of tuberculosis treatment response with serial C-reactive protein measurements. Open Forum Infect Dis. (2018) 5:ofy253. doi: 10.1093/ofid/ofy253
34. Kumar, NP, Moideen, K, Nancy, A, Viswanathan, V, Thiruvengadam, K, Sivakumar, S, et al. Acute phase proteins are baseline predictors of tuberculosis treatment failure. Front Immunol. (2021) 12:731878. doi: 10.3389/fimmu.2021.731878
35. Xue, Q, Wang, N, Xue, X, and Wang, J. Endobronchial tuberculosis: an overview. Eur J Clin Microbiol Infect Dis. (2011) 30:1039–44. doi: 10.1007/s10096-011-1205-2
36. Jung, SS, Park, HS, Kim, JO, and Kim, SY. Incidence and clinical predictors of endobronchial tuberculosis in patients with pulmonary tuberculosis. Respirology. (2015) 20:488–95. doi: 10.1111/resp.12474
37. Hu, T, Li, Y, Wang, X, Chen, Y, Nie, X, Zhuang, R, et al. Early and regular bronchoscopy examination on effect of diagnosis and prognosis for patients with tracheobronchial tuberculosis. Front Med. (2022) 9:825736. doi: 10.3389/fmed.2022.825736
38. Asad, M, Mahmood, A, and Usman, M. A machine learning-based framework for predicting treatment failure in tuberculosis: a case study of six countries. Tuberculosis. (2020) 123:101944. doi: 10.1016/j.tube.2020.101944
Keywords: pulmonary tuberculosis, pulmonary CT, intensive phase, poor treatment outcome, prediction model, machine learning model
Citation: Lu B, Shi Y, Wang M, Jin C, Liu C, Pan X and Chen X (2025) Development of a clinical prediction model for poor treatment outcomes in the intensive phase in patients with initial treatment of pulmonary tuberculosis. Front. Med. 12:1472295. doi: 10.3389/fmed.2025.1472295
Received: 01 August 2024; Accepted: 11 March 2025;
Published: 26 March 2025.
Edited by:
Synne Jenum, Oslo University Hospital, NorwayReviewed by:
Wenhui Guo, Stanford University, United StatesCopyright © 2025 Lu, Shi, Wang, Jin, Liu, Pan and Chen. This is an open-access article distributed under the terms of the Creative Commons Attribution License (CC BY). The use, distribution or reproduction in other forums is permitted, provided the original author(s) and the copyright owner(s) are credited and that the original publication in this journal is cited, in accordance with accepted academic practice. No use, distribution or reproduction is permitted which does not comply with these terms.
*Correspondence: Xinling Pan, cGFuZmVuZ3l1d3V6dUAxNjMuY29t; Xiang Chen, Y2hlbnhpYW5nMzEzMEAxNjMuY29t
Disclaimer: All claims expressed in this article are solely those of the authors and do not necessarily represent those of their affiliated organizations, or those of the publisher, the editors and the reviewers. Any product that may be evaluated in this article or claim that may be made by its manufacturer is not guaranteed or endorsed by the publisher.
Research integrity at Frontiers
Learn more about the work of our research integrity team to safeguard the quality of each article we publish.