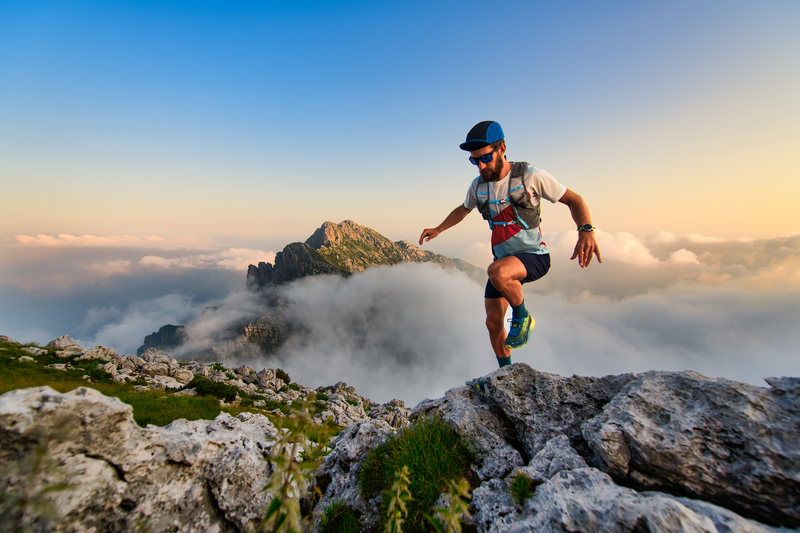
95% of researchers rate our articles as excellent or good
Learn more about the work of our research integrity team to safeguard the quality of each article we publish.
Find out more
SYSTEMATIC REVIEW article
Front. Med. , 08 January 2025
Sec. Regulatory Science
Volume 11 - 2024 | https://doi.org/10.3389/fmed.2024.1522554
This article is part of the Research Topic Errors and Biases in Modern Healthcare: Public Health, Medico-legal and Risk Management Aspects View all 6 articles
Introduction: Adverse events in hospitals significantly compromise patient safety and trust in healthcare systems, with medical errors being a leading cause of death globally. Despite efforts to reduce these errors, reporting remains low, and effective system changes are rare. This systematic review explores the potential of artificial intelligence (AI) in clinical risk management.
Methods: The systematic review was conducted using the PRISMA Statement 2020 guidelines to ensure a comprehensive and transparent approach. We utilized the online tool Rayyan for efficient screening and selection of relevant studies from three different online bibliographic.
Results: AI systems, including machine learning and natural language processing, show promise in detecting adverse events, predicting medication errors, assessing fall risks, and preventing pressure injuries. Studies reveal that AI can improve incident reporting accuracy, identify high-risk incidents, and automate classification processes. However, challenges such as socio-technical issues, implementation barriers, and the need for standardization persist.
Discussion: The review highlights the effectiveness of AI in various applications but underscores the necessity for further research to ensure safe and consistent integration into clinical practices. Future directions involve refining AI tools through continuous feedback and addressing regulatory standards to enhance patient safety and care quality.
Adverse events in hospitals pose a serious threat to patient care quality and safety globally, contributing to patient distrust and impacting healthcare facility reputations (1). A significant report estimated 45,000–98,000 annual deaths in the U.S. due to medical errors (2). Despite widespread reporting systems, < 10% of errors are reported, and only 15% of hospital responses prevent future incidents (3). Overcoming structural and cultural barriers is crucial for improving patient safety (4). Medical errors, defined as actions leading to unintended results, affect patients, families, healthcare providers, and communities (5). They include drug side effects, misdiagnoses, surgical errors, and falls (7), occurring across care processes from medication to post-operative care. Healthcare risk management combines reactive systems like incident reporting with proactive methods such as Failure Mode and Effects Analysis (FMECA) (8), aiming to learn from past errors and prevent future ones through continuous improvement. Artificial Intelligence (AI) offers potential in healthcare by enhancing diagnostics, optimizing care, and predicting outcomes (9, 10). AI can detect clinical data anomalies, improving diagnostic accuracy, though integrating AI requires addressing new and existing risks (11, 12). This review provides an overview of AI applications in clinical risk management, assessing their benefits, reproducibility, and integration challenges in healthcare settings.
The methodology of this systematic review was developed following the guidelines of The Preferred Reporting Items for a Systematic Review and Meta-Analysis of Diagnostic Test Accuracy Studies (PRISMA-DTA) (13).
The keywords for the search (Table 1) were selected using terms related to the phrases “clinical risk management” and “artificial intelligence.” The search string used is provided in Table 1.
The search of the scientific literature was conducted in February 2024. Three online bibliographic databases were examined, which are as follows:
• Pubmed
• Scopus
• Web of Science
The first phase of the literature review was carried out using the Rayyan® tool.
This systematic review includes studies that simultaneously meet all of the following criteria: (1) Use of artificial intelligence systems, defined as any system capable of replicating complex mental processes through the use of a computer. (2)Application of the artificial intelligence system in the healthcare context. (3) Employment of the artificial intelligence system in areas of interest to clinical risk management. (4) Presence of results derived from the active experimentation of the system. (5) Prevention of an adverse event, defined as an unintentional incident resulting in harm to the patient's health that is not directly related to the natural progression of the patient's disease or health condition (14).
Exclusion criteria were primarily used to remove studies that, although involving the use of artificial intelligence systems to enhance care safety, addressed areas not pertinent to the concept of medical error (e.g., risk of cardiac arrest, risk of re-infarction, etc.).
The search across the three databases yielded 662 results (Figure 1). After removing duplicates, the number was reduced to 489 studies. We excluded 421 articles as they did not meet the five established inclusion criteria. In most cases, the excluded events pertained to contexts unrelated to clinical risk management, such as complications arising from the natural progression of diseases rather than preventable adverse events. Following an initial review of the articles, 68 studies were included in the database. An additional 16 studies were excluded. One of the articles (15) was excluded because it represents a future development project for a high-performance prediction, detection, and monitoring platform for managing risks against patient safety, without providing any results. Seven of the articles (16–22) were not included as they addressed clinical risk topics but did not reference the use of artificial intelligence. One of the excluded articles (23) was only available as an abstract. Three studies were not included because, although they discussed the use of artificial intelligence in clinical risk management, they only described the software without reporting results (22, 24, 25). One article was excluded as it was a report of a discussion from a roundtable on risk management in the use of medical devices (26). Another article was not included because it was an editorial and did not meet the inclusion criteria (27). Two articles were excluded as they were not relevant to risk management in the hospital environment (28, 29).
The analyzed studies propose diverse methodologies in the field of risk management, offering both proactive and reactive approaches within a heterogeneous application context. The main characteristics of the reviewed articles are presented in Table 2.
The articles under review were published between 2007 and 2024. As expected, the number of publications has seen a steady increase in recent years due to growing interest, particularly in media coverage, and the development of artificial intelligence systems. Specifically, from 2019 to 2024, 36 of the analyzed studies were produced, compared to 16 from 2007 to 2008. As depicted in Figure 2, the countries where the analyzed studies originated include Israel, Denmark, Netherlands, Lebanon, Brazil, United Kingdom, Switzerland, Canada, France, Italy, Spain, United Arab Emirates, South Korea, Taiwan, Japan, China, Australia, and the United States. Figure 2 shows the duration in years of the studies that provided this type of information.
In this systematic review, it emerged that the most frequently discussed topics in the scientific literature related to risk management are related to the detection of adverse events, followed by the risk of falls, and then the development of pressure ulcers.
In the study conducted by Barmaz Y and Ménard T (30), a hierarchical Bayesian model was employed to estimate reporting rates at clinical sites and assess the risk of under-reporting based on anonymized public clinical trial data from Project Data Sphere. This model infers reporting behavior from patient data, enabling the detection of anomalies across clinical sites. This system has proven useful by reducing the need for audits and enhancing clinical quality assurance activities related to safety reporting in clinical trials. Bates et al. (31) conducted a scoping review evaluating the role of AI in improving patient safety through the interpretation of data collected from vital signs monitoring systems, wearables, and pressure sensors. The evidence gathered recognized significant potential in this approach, though continuous efforts are required for implementing these systems in healthcare organizations. Benin et al. (32) developed an electronic system for processing medical event reports to enhance patient safety. This system improved care safety outcomes by categorizing the same event into multiple error categories based on logical correspondences, unlike manual approaches where each error type corresponds to a single category. Elizabeth M. Borycki's work (33) addressed incident reporting related to adverse events induced by healthcare technologies, assessing the associated advantages and disadvantages. The study concluded that this experimental approach is promising. Chen H et al. (34) evaluated the effectiveness of various machine learning-based automatic tools for adverse event classification, proposing an interface integrated with this system. The results highlighted the potential of such a system to achieve efficient and reliable report classification processes. Similarly, S. Fodeh et al. (35) proposed an automatic classification model for adverse events, combining feature detection system operations with a machine learning classifier. This model proved particularly useful for two adverse event categories: patient identification errors and weight-related issues. In contrast, Allan Fong et al. (36) advocated for the use of natural language processing (NLP) in identifying four categories of errors: Pharmacy Delivery Delay, Pharmacy Dispensing Error, Prescriber Error, and Pyxis Discrepancy. The study demonstrated that the tool's accuracy can help reduce the workload of hospital safety committees. Katsuhide Fujita et al. (37) applied NLP in incident reporting to analyze incident report texts, reinterpreting structured incident information and improving incident-related cause management. The article highlighted the tool's effectiveness, particularly for issues related to patient falls and medication management. Gerdes and Hardahl (38) tested an NLP system for reviewing clinical records to identify adverse events. The encouraging results suggest considering the systematic introduction of such automatic monitoring systems. Gupta et al. (39) proposed an automatic clinical incident classification system testing four different algorithms. Among these, the multinomial naive Bayes algorithm demonstrated particular efficiency, requiring a well-structured training phase. In another study, Gupta J et al. (40) introduced an incident reporting system based on the C4.5 decision tree algorithm and random forest, using a taxonomy from a generic system and one proposed by the WHO. The study demonstrated the superiority of the random forest algorithm and introduced a modification to the WHO taxonomy by adding another adverse event class. Hendrickx et al. (41) applied text mining techniques to highlight patient safety issues, indicating that these systems can be useful for prioritizing safety concerns and automatically classifying event severity. Liu et al. (42) proposed a text mining system for retrospective analysis of patient fall reports, reporting highly encouraging results regarding its application. Ménard et al. (43) proposed an under-reporting detection system for adverse events using a machine learning approach. Positive results from clinical trials of this approach led to the extension of this adverse event detection system to all future Roche/Genentech studies. Okamoto et al. (44) employed a machine learning system to detect unreported errors in medical records, identifying 121 incidents, with 34 subsequently selected as serious errors. In their work, Ong et al. (45) explored using Naïve Bayes and SVM text classifiers to detect extreme-risk events in clinical reports from Australian hospitals. The classifiers were evaluated on their accuracy, precision, recall, F-measure, and AUC, showing feasibility for automatic detection of high-risk incidents. Implementing a fall risk prediction tool resulted in a reduction in patient falls and an increase in risk-targeted nursing interventions in intervention units, although there was no significant difference in fall injury rates compared to control units. Saab et al. (46) proposed a machine learning model for predicting adverse events responsible for hospital readmission, aiming to reduce associated costs. The achieved accuracy levels were consistent with previous studies, highlighting the real-time feedback advantage of the tested system. Sun et al. (47) proposed an incident reporting system combining a conversational interface with speech recognition software, concluding that socio-technical issues currently preclude its implementation. Wang et al. (48) evaluated the feasibility of using the Unified Medical Language System (UMLS) for automatically identifying patient safety incident reports by type and severity, showing its superiority over bag-of-words classifiers. In another study by Wang et al. (49), neural networks were used to assess the severity and gravity of adverse event reports. In a third study by Wang et al. (50), a multi-label incident classification system was structured for multiple incident types in individual reports. While not broadly applicable, this method proved useful in multi-label classification using a support vector machine algorithm. In a systematic review by Young et al. (51), NLP was investigated for free-text recognition in incident reporting. The review concluded that NLP can yield significant information from unstructured data in the specific domain of incident and adverse event classification, potentially enhancing adverse event learning in healthcare. Zhou et al. (52) proposed and tested an automated system for analyzing medication dispensing error reports based on machine learning algorithms. The study developed three different classifiers based on two algorithms (support vector machine and random forest), capable of identifying event causes and reorganizing them based on similarities.
In a study by Corny et al. (53), a hybrid clinical decision support system was tested to reduce errors in the medication prescribing phase. Implementing this system demonstrated higher accuracy compared to existing techniques, intercepting 74% of all prescription orders requiring pharmacist intervention, with a precision of 74%. King et al. (54) used machine learning models to predict medication ordering errors and identify contributing factors. Decision trees using gradient boost achieved the highest AUROC (0.7968) and AUPRC (0.0647) among all models, showing promise for error surveillance, patient safety improvement, and targeted clinical review. Wong et al. (55) proposed a wound dressing rights detection system using NLP and deep neural networks. This system automated the identification of dressing incidents, highlighting the potential of deep learning for exploring textual reports on dressing incidents. Zheng et al. (56) focused on medication dispensing errors, reporting the development of an AI system through collaboration with pharmacists. They improved various features such as the interpretability of AI systems by adding gradual check marks, probability scores, and details on medications confused by the AI model. They also emphasized the need to build a simple and accessible system.
Stein et al. (57) evaluated the impact of a fall risk prediction system, assessing its outcomes in terms of patient outcomes and nurse feedback. The results highlighted a slight immediate reduction in the number of falls without consistent long-term effects, but the tool demonstrated intrinsic utility. Cho et al. (58) evaluated the usability of a predictive algorithm for detecting individual fall risk factors. Although a reduction in fall rates was observed, particularly in those over 65 years old, the intervention was not associated with a significant reduction in this rate. Huang et al. (59) employed a machine learning approach to study a 14-month fall event database aimed at developing a predictive fall risk system. This approach demonstrated particular accuracy and is used daily in one of Taiwan's medical centers. Ladios-Martin et al. (60) developed a machine learning tool for fall risk prediction through the evaluation of a series of variables in a retrospective cohort. The Two-Class Bayes Point Machine algorithm was chosen, showing a reduction in fall events compared to the control group. Lee et al. (61) used a different approach to falls, employing data mining on hospital information system data. An artificial neural network was used to develop a predictive model that demonstrated high predictivity with a higher ROC compared to a logistic regression model. Liu et al. (62) proposed a system aimed at improving and automating severity classification models of incidents. The tool proved useful in identifying and classifying fall events, with the top two algorithms being random forest and random oversampling. Shim et al. (63) developed and validated a machine learning model for fall prediction that is integrable into an electronic medical record system. This system, whose effectiveness was confirmed during the study, was subsequently officially integrated into the clinical record system. Silva et al. (64) proposed a machine learning model based on the Naive Bayes algorithm for developing a predictive tool for patient fall risk related to prescribed drug therapy. The Naive Bayes algorithm demonstrated superior values compared to other algorithms, particularly with an AUC of 0.678, sensitivity of 0.546, and specificity of 0.744. Wang et al. (65) proposed a tool to predict the severity of damage following a patient fall. Several machine learning algorithms were used, with the random forest algorithm proving the best with an accuracy of 0.844 and precision of 0.839. Therefore, an online severity prediction system was built using the RF algorithm and Flask package. By leveraging this predictive system, healthcare facilities can enhance patient safety and better allocate limited resources. Wang et al. (66) proposed a model to evaluate the predictability of fall events among hospitalized patients through a retrospective cohort study. A predictive classifier developed using multi-view ensemble learning with missing values demonstrated superior predictive power compared to random forest and support vector machine, two other comparison algorithms.
Barghouthi et al. (67) conducted a systematic review of prediction models for the development of pressure ulcers. The study highlighted that the most commonly employed algorithm is logistic regression. However, it also noted that none of the reviewed studies successfully used the pressure ulcer prediction model in real-world settings. Borlawsky and Hripcsak (68) proposed a similar model based on the C4.5 decision tree induction algorithm. Results showed limited application of this naive classification algorithm to automate the assessment of pressure ulcer risk. Do et al. (69) assessed the impact of an electronic predictive tool on fall risk using EHR data compared to a standard assessment tool. Conducted over 2 years in 12 nursing units, the primary outcome measured was the rate of patient falls, with secondary outcomes including injury rates and nursing interventions. The most accurate model achieved a 99.7% area under the receiver operating characteristic curve, with ten-fold cross-validation ensuring generalizability. Random forest and decision tree models had the highest prediction accuracy rates at 98%, consistent in the validation cohort. Ladios-Martin et al. (70) proposed another predictive model for the risk of developing pressure ulcers using a logistic regression algorithm. The model demonstrated a sensitivity of 0.90, specificity of 0.74, and an area under the curve of 0.89. The model performed well 1 year later in a real-world setting. Song et al. (71) employed a random forest-type predictive algorithm applied to a case study of hospital-acquired and non-hospital-acquired pressure ulcers, showing AUCs of 0.92 and 0.94 in two test sets. The study concluded that the tool could also be employed in real-world settings. Toffaha et al. (72) reviewed the literature on the prediction of pressure ulcer development, highlighting the existence of numerous predictive models, none of which have been applied in real healthcare settings but were rather trained on previous cases.
Simsekler et al. (73) employed three different machine learning algorithms to identify potential associations between organizational factors and errors affecting patient and staff safety. The results suggested that “health and wellbeing” is the main theme influencing patient and staff safety errors, with “workplace stress” being the most important factor associated with adverse outcomes for both patients and staff. Murphy et al. (74) proposed a system known as Safer Dx Trigger Tools, capable of identifying real-time and retrospective errors in the care pathway through analysis of electronic clinical data. The study concluded with the potential future application of this type of tool in daily hospital practice. Miniati et al. (75) provided a decision support system for analyzing the safety level associated with the use of technologies for both patients and staff. The experimental tool proved useful in predicting outcomes in specific scenarios, with the authors concluding that this could be extended to other areas. Levis et al. (76) analyzed the suicide risk factor through retrospective analysis of psychiatric notes using a predictive model based on NLP. Specifically, an 8% increase in predictability was observed in the 12-month study cohort compared to more advanced available methods. Hui Jun Si et al. (77) proposed a risk management model related to the disinfection process of hospital environments using AI systems. Using a k-nearest neighbor algorithm, the results highlighted that levels of job satisfaction and work standardization achieved by nursing staff managed by an AI algorithm were significantly higher than those achieved by nurses working in traditionally managed disinfection centers. Huang et al. (78) proposed a system known as Artificial Intelligence Clinical Assistant Decision Support System (AI-CDSS) for preventing thromboembolic events; however, the tool was found to be ineffective. Choundhury et al. (79) conducted a literature review on the role of AI in ensuring patient safety, focusing on subcategories such as clinical alarms, clinical reports, and medication safety issues. Several software analyzed in this study have been designed and developed with features that can be considered medical devices, however, according to the literature reviewed, none of them have reached an official approval stage according to the EU MDR 2017/745 regulation or the US FDA. According to EU MDR 2017/745, among other aspects, software can be considered a medical device if it is intended to provide information for diagnostic and therapeutic purposes, as well as to help prevent, monitor, diagnose or even treat disease or injury (80). Based on this, the model developed by Corny et al. (53), which identifies prescriptions with a high risk of error, could fall into this category, as could the one proposed by Ladios-Martin et al. (6) for fall prevention. User acceptance and specific training are central aspects for the successful implementation of artificial intelligence (AI) systems in clinical settings. Barriers such as resistance to change, technological complexity, and lack of specific expertise can be overcome with targeted strategies such as user-centered design and dedicated training programs. Many studies included in the review highlight the importance of involving end users (physicians, nurses, pharmacists) early in development to ensure that systems meet their operational needs. Targeted training, often supported by pilot testing and simulations, has proven crucial in familiarizing users with new technologies and improving their confidence in daily use. For example, Sun et al. designed a speech recognition-based reporting system, the use of which was tested through a pilot project. The feedback highlighted the need for more detailed instructions to overcome the socio-technical difficulties encountered (47). Silva et al. developed a predictive model for fall risk and accompanied its implementation with specific training sessions. Users evaluated the approach positively, emphasizing the usefulness of ongoing support (64). Similarly, Huang et al. highlighted how practical training sessions improved the adoption of a predictive system for falls risk, facilitating the integration of the software into clinical practice and gathering suggestions for further technical improvements (59). Zheng et al. developed a system to prevent medication dispensing errors using focus groups with pharmacists. This approach allowed them to iterate on the interface and instructions for use, significantly improving end-user satisfaction (56). According to the review, most studies did not highlight significant issues with AI, such as the lack of standards and evaluation metrics. Further research and involvement of FDA and NIST are needed to create standards that ensure patient safety.
The reviewed studies primarily focus on incident reporting in healthcare, with two prominent approaches: automatic incident classification systems and event detection through healthcare documentation analysis. Machine learning algorithms have proven effective in automating incident classification, enhancing accuracy through past case training. Natural language processing and text mining techniques have enabled automated adverse event detection and anomaly identification in clinical data, improving care quality and reducing manual audits. Continuous implementation and system refinement are crucial for maximizing these benefits and addressing socio-technical challenges in healthcare settings. In managing medication errors, AI and machine learning have shown promise in decision support for prescription accuracy and error prevention during medication ordering. Hybrid clinical decision support systems and gradient boosting decision trees demonstrate significant accuracy in intercepting prescription errors. Deep learning techniques improve medication incident identification, emphasizing collaboration with pharmacists for system interpretability and usability in clinical practice. Regarding falls management, AI applications focus on predictive models for fall risk and severity classification systems. While predictive algorithms enhance risk assessment, their impact on reducing falls varies across age groups and implementation settings within electronic health records. Ongoing refinement is necessary to optimize predictive accuracy and practical integration into clinical workflows. Studies on predictive models for pressure ulcer development reveal varied efficacy, with machine learning algorithms like random forest showing promising predictive capability. However, the application of these models in real healthcare environments requires further validation and standardization to ensure practical clinical utility. AI and machine learning also play pivotal roles in enhancing patient and healthcare staff safety. They identify organizational factors influencing safety outcomes, support real-time error detection through tools like Safer Dx Trigger Tools, and improve predictive accuracy for technology-related risks and suicide risk. Despite successes, challenges remain, including the need for standardized evaluation metrics and regulatory oversight to ensure the efficacy and safety of AI applications in patient care. A crucial issue remains the proper and safe implementation of AI in clinical risk management practices. First, it is crucial to assess the specific needs of the clinical setting by going out and identifying all the areas where AI can provide the greatest positive impact, such as adverse event detection, falls prevention, or medication error management. This type of analysis should, in any case, involve end users so that the system is designed and designed based on their operational needs. This should be followed by a controlled pilot phase to test the technology in a protected environment to highlight possible problems related to its use; at this juncture, safety measures such as automated monitoring and audit systems should be implemented to reduce bias and errors (81). At a second stage, user education with training programs to understand the technical operation of the system but also its limitations should be crucial. Once the system is validated, its large-scale implementation should be accompanied by continuous monitoring with periodic audits and user reporting systems. Finally, the AI system should be designed to work in perfect synergy with existing tools such as hospital information systems and electronic health records. Such a holistic approach could not only improve the safety and quality of care but could also optimize the allocation of healthcare resources.
The reviewed studies demonstrate that artificial intelligence (AI) and machine learning (ML) systems are transforming healthcare safety across various domains, including incident management, medication prescription, and fall prevention. Predictive algorithms and ML models have significantly improved the identification and handling of adverse events, reducing reliance on manual audits and enhancing reporting accuracy. Despite these advancements, the practical application of AI in real healthcare settings remains limited and requires ongoing refinement. Future efforts aim to enhance these systems by integrating feedback from healthcare professionals and optimizing their integration with electronic health records. Establishing uniform standards and evaluation metrics is critical to ensuring the effectiveness and safety of AI-driven solutions. Collaboration with regulatory bodies is essential to develop guidelines that support the safe and efficient use of AI technologies in everyday clinical practice. These advancements are expected to not only enhance care quality but also facilitate more effective management of healthcare resources.
The original contributions presented in the study are included in the article/supplementary material, further inquiries can be directed to the corresponding author.
FD: Conceptualization, Formal analysis, Writing – original draft, Investigation, Visualization. GD: Conceptualization, Formal analysis, Methodology, Project administration, Writing – original draft, Writing – review & editing. DF: Methodology, Project administration, Software, Writing – original draft. AD: Software, Writing – original draft. LT: Software, Writing – original draft. VT: Investigation, Supervision, Writing – review & editing. MC: Investigation, Supervision, Writing – review & editing. RS: Conceptualization, Formal analysis, Investigation, Visualization, Writing – original draft.
The author(s) declare that no financial support was received for the research, authorship, and/or publication of this article.
Gianmarco Di Palma is a Ph.D. student enrolled in the National Ph.D. in Artificial Intelligence, XL cycle, course on Health and Life Sciences, organized by Universitè Campus Bio-Medico di Roma.
The authors declare that the research was conducted in the absence of any commercial or financial relationships that could be construed as a potential conflict of interest.
The author(s) declare that no Gen AI was used in the creation of this manuscript.
All claims expressed in this article are solely those of the authors and do not necessarily represent those of their affiliated organizations, or those of the publisher, the editors and the reviewers. Any product that may be evaluated in this article, or claim that may be made by its manufacturer, is not guaranteed or endorsed by the publisher.
1. Schwendimann R, Blatter C, Dhaini S, Simon M, Ausserhofer D. The occurrence, types, consequences and preventability of in-hospital adverse events - a scoping review. BMC Health Serv Res. (2018) 18:521. doi: 10.1186/s12913-018-3335-z
2. Kohn JM, Corrigan M. Donaldson S. To Err is Human: Building a Safer Health System. Washington DC: National Academy Press (1999).
3. Anderson JG, Abrahamson K. Your health care may kill you: medical errors. Stud Health Technol Inform. (2017) 234:13–7. doi: 10.3233/978-1-61499-742-9-13
4. Anderson JG. Regional patient safety initiatives: the missing element of organizational change. AMIA Annu Symp Proc. (2006) 2006:1163–4.
6. Ellahham S. The Domino Effect of Medical Errors. Am J Med Qual. (2019) 34:412–3. doi: 10.1177/1062860618813735
7. Carver N, Gupta V, Hipskind JE. Medical errors. In: StatPearls [Internet]. Treasure Island (FL): StatPearls Publishing (2024).
8. Bender JA, Kulju S, Soncrant C. Combined proactive risk assessment: unifying proactive and reactive risk assessment techniques in health care. Jt Comm J Qual Patient Saf. (2022) 48:326–34. doi: 10.1016/j.jcjq.2022.02.010
9. Bahl M, Barzilay R, Yedidia AB, Locascio NJ, Yu L, Lehman CD. High-risk breast lesions: a machine learning model to predict pathologic upgrade and reduce unnecessary surgical excision. Radiology. (2018) 286:810–8. doi: 10.1148/radiol.2017170549
10. Peerally MF, Carr S, Waring J, Martin G, Dixon-Woods M. Risk controls identified in action plans following serious incident investigations in secondary care: a qualitative study. J Patient Saf. 2024 Jun 26. doi: 10.1097/PTS.0000000000001238
11. McCarthy J, Hayes P. Some philosophical problems from the standpoint of artificial intelligence. In:Meltzer B, Michie D, , editors. Machine Intelligence. Edinburgh: Edinburgh University Press (1969). p. 463–502.
12. Macrae C. Governing the safety of artificial intelligence in healthcare. BMJ Qual Saf. (2019) 28:495–8. doi: 10.1136/bmjqs-2019-009484
13. Page MJ, McKenzie JE, Bossuyt PM, Boutron I, Hoffmann TC, Mulrow CD, et al. The PRISMA 2020 statement: an updated guideline for reporting systematic reviews. BMJ. (2021) 372:n71. doi: 10.1136/bmj.n71
14. Joint Commission International. Accreditation Standards for Hospitals, 7th Edition. Oak Brook, IL: Joint Commission Resources, Inc. (2021).
15. Carenini M. ReMINE Consortium. ReMINE: an ontology-based risk management platform. Stud Health Technol Inform. (2009) 148:32–42. doi: 10.3233/978-1-60750-043-8-32
16. Di Giovanni R, Cochrane A, Parker J, Lewis J. Adverse events in the digital age and where to find them. Pharmacoepidemiol Drug Saf. (2022) 31:1131–9. doi: 10.1002/pds.5532
17. Katsulis Z, Ergai A, Leung WY, Schenkel L, Rai A, Adelman J, et al. Iterative user centered design for development of a patient-centered fall prevention toolkit. Appl Ergon. (2016) 56:117–26. doi: 10.1016/j.apergo.2016.03.011
18. Padula WV, Pronovost PJ, Makic MBF, Wald HL, Moran D, Mishra MK et al. Value of hospital resources for effective pressure injury prevention: a cost-effectiveness analysis. BMJ Quality Safety. (2019). 28:132–41. doi: 10.1136/bmjqs-2017-007505
19. Shojania KG. Incident reporting systems: what will it take to make them less frustrating and achieve anything useful? Jt Comm J Qual Patient Saf. (2021) 47:755–8. doi: 10.1016/j.jcjq.2021.10.001
20. Novak A, Nyflot MJ, Ermoian RP, Jordan LE, Sponseller PA, Kane GM, et al. Targeting safety improvements through identification of incident origination and detection in a near-miss incident learning system. Med Phys. (2016) 43:2053–62. doi: 10.1118/1.4944739
21. Piscitelli A, Bevilacqua L, Labella B, Parravicini E, Auxilia F. A keyword approach to identify adverse events within narrative documents from 4 Italian institutions. J Patient Saf. (2022) 18:e362–7. doi: 10.1097/PTS.0000000000000783
22. Macrae C. Managing risk and resilience in autonomous and intelligent systems: Exploring safety in the development, deployment, and use of artificial intelligence in healthcare. Risk Analysis. (2024) 44:1999–2025. doi: 10.1111/risa.14273
23. Gupta J, Patrick J. Automated validation of patient safety clinical incident classification: macro analysis. Stud. Health Technol Informat. (2013) 188:52–57. doi: 10.3233/978-1-61499-266-0-52
24. Kleymenova E, Dronov M, Yashina L, Payushchik S, Nigmatkulova M, Otdelenov V. User-configurable decision support system for clinical risk management. Procedia Comp Sci. (2021) 190:463–70. doi: 10.1016/j.procs.2021.06.054
25. Proux D, Segond F, Gerbier S, Metzger MH. Addressing risk assessment for patient safety in hospitals through information extraction in medical reports. In:Shi Z, Mercier-Laurent E, Leake D, , editors. Intelligent Information Processing IV. IFIP - The International Federation for Information Processing, Vol. 288. Boston, MA: Springer (2008). p. 230–9. doi: 10.1007/978-0-387-87685-6_28
26. Stern G, Baird P, Bills E, Busdicker M, Grob A, Krenc T, et al. Roundtable discussion: in medical device risk management, patients take on increased importance. Biomed Instrum Technol. (2020) 54:283–90. doi: 10.2345/0899-8205-54.4.283
27. Patriarca R, Falegnami A, Bilotta F. Embracing simplexity: the role of artificial intelligence in peri-procedural medical safety. Expert Rev Med Devices. (2019) 16:77–9. doi: 10.1080/17434440.2019.1561269
28. Thornton J, Tandon R. Does machine-learning-based prediction of suicide risk actually reduce rates of suicide: a critical examination. Asian J Psychiatr. (2023) 88:103769. doi: 10.1016/j.ajp.2023.103769
29. Tucan P, Gherman B, Major K, Vaida C, Major Z, Plitea N, et al. Fuzzy logic-based risk assessment of a parallel robot for elbow and wrist rehabilitation. Int J Environ Res Public Health. (2020) 17:654. doi: 10.3390/ijerph17020654
30. Barmaz Y, Ménard T. Bayesian modeling for the detection of adverse events underreporting in clinical trials. Drug Safety. (2021) 44:949–55. doi: 10.1007/s40264-021-01094-8
31. Bates DW, Levine DM, Syrowatka A, Kuznetsova M, Craig KJT, Rui A, et al. The potential of artificial intelligence to improve patient safety: a scoping review. NPJ Digit Med. (2021) 4:1. doi: 10.1038/s41746-021-00423-6
32. Benin AL, Fodeh SJ, Lee K, Koss M, Miller P, Brandt C. Electronic approaches to making sense of the text in the adverse event reporting system. J Healthc Risk Manage. (2016) 36:10–20. doi: 10.1002/jhrm.21237
33. Borycki EM, Farghali A, Kushniruk AW. Integrating human patterns of qualitative coding with machine learning: a pilot study involving technology-induced error incident reports. Stud Health Technol Informat. (2022) 295:276–80. doi: 10.3233/SHTI220716
34. Chen HB, Cohen E, Wilson D, Alfred M. A machine learning approach with human-AI collaboration for automated classification of patient safety event reports: algorithm development and validation study. JMIR Human Fact. (2024) 11:53378. doi: 10.2196/53378
35. Fodeh SJ, Miller P, Brandt C, Koss M, Kuenzli E. Laplacian SVM based Feature Selection Improves Medical Event Reports Classification. (2015), 449–54. doi: 10.1109/ICDMW.2015.141
36. Fong A, Harriott N, Walters DM, Gross SA, Brown G, Smith V. Integrating natural language processing expertise with patient safety event review committees to improve the analysis of medication events. Int J Med Inform. (2017) 104:120–25. doi: 10.1016/j.ijmedinf.2017.05.005
37. Fujita K, Akiyama M, Park K, Yamaguchi EN, Furukawa H. Linguistic analysis of large-scale medical incident reports for patient safety. Stud Health Technol Informat. (2012) 180:250–54. doi: 10.3233/978-1-61499-101-4-250
38. Gerdes LU, Hardahl C. Text mining electronic health records to identify hospital adverse events. Stud Health Technol Informat. (2013) 192:1145. doi: 10.3233/978-1-61499-289-9-1145
39. Gupta J, Koprinska I, Patrick J. Automated classification of clinical incident types. Stud Health Technol Informat. (2015) 214:87–93. doi: 10.3233/978-1-61499-558-6-87
40. Gupta J, Patrick J, Poon S, et al. Clinical safety incident taxonomy performance on c4.5 decision tree and random forest. Stud Health Technol Informat. (2019) 266:83–88.
41. Hendrickx I, Voets T, van Dyk P, Kool RB. Using text mining techniques to identify health care providers with patient safety problems: exploratory study. J Med Internet Res. (2021) 23:7. doi: 10.2196/19064
42. Liu J, Wong ZS, Tsui KL, So HY, Kwok A. Exploring hidden in-hospital fall clusters from incident reports using text analytics. Stud Health Technol Inform. (2019) 264:1526–27. doi: 10.3233/SHTI190517
43. Ménard T, Barmaz Y, Koneswarakantha B, Bowling R, Popko L. Enabling data-driven clinical quality assurance: predicting adverse event reporting in clinical trials using machine learning. Drug Safety. (2019) 42:1045–53. doi: 10.1007/s40264-019-00831-4
44. Okamoto K, Yamamoto T, Hiragi S, Ohtera S, Sugiyama O, Yamamoto G, et al. Detecting severe incidents from electronic medical records using machine learning methods. Stud Health Technol Inform. (2020) 270:1247–48. doi: 10.3233/SHTI200385
45. Ong M, Magrabi F, Coiera E. Automated identification of extreme-risk events in clinical incident reports. J Am Med Inform Assoc. (2012) 19:e110–18. doi: 10.1136/amiajnl-2011-000562
46. Saab A, Saikali M, Lamy JB. Comparison of machine learning algorithms for classifying adverse-event related 30-day hospital readmissions: potential implications for patient safety. Stud Health Technol Inform. (2020) 272:51–54. doi: 10.3233/SHTI200491
47. Sun O, Chen J, Magrabi F. Using voice-activated conversational interfaces for reporting patient safety incidents: a technical feasibility and pilot usability study. Stud Health Technol Inform. (2018) 252:139–44. doi: 10.3233/978-1-61499-890-7-139
48. Wang Y, Coiera E, Magrabi F. Can unified medical language system-based semantic representation improve automated identification of patient safety incident reports by type and severity? J Am Med Inform Assoc. (2020) 27:1502–9. doi: 10.1093/jamia/ocaa082
49. Wang Y, Coiera E, Magrabi F. Using convolutional neural networks to identify patient safety incident reports by type and severity. J Am Med Inform Assoc. (2019) 26:1600–8. doi: 10.1093/jamia/ocz146
50. Wang Y, Coiera E, Runciman W, Magrabi F. Automating the identification of patient safety incident reports using multi-label classification. Stud Health Technol Inform. (2017) 245:609–13. doi: 10.3233/978-1-61499-830-3-609
51. Young IJB, Luz S, Lone N. A systematic review of natural language processing for classification tasks in the field of incident reporting and adverse event analysis. Int J Med Inform. (2019) 132: 103971. doi: 10.1016/j.ijmedinf.2019.103971
52. Zhou S, Kang H, Yao B, Gong Y. An automated pipeline for analyzing medication event reports in clinical settings. BMC Med Inform Decis Mak. (2018) 18:113. doi: 10.1186/s12911-018-0687-6
53. Corny J, Rajkumar A, Martin O, Dode X, Lajonchère JP, Billuart O, et al. A machine learning-based clinical decision support system to identify prescriptions with a high risk of medication error. Am Med Inform Assoc. (2020) 27:1688–94. doi: 10.1093/jamia/ocaa154
54. King CR, Abraham J, Fritz BA, Cui Z, Galanter W, Chen Y, et al. Predicting self-intercepted medication ordering errors using machine learning. PLoS ONE. (2021) 16:7. doi: 10.1371/journal.pone.0254358
55. Wong Z, So HY, Kwok BS, Lai MW, Sun DT. Medication-rights detection using incident reports: a natural language processing and deep neural network approach. Health Informatics J. (2020) 26:1777–94. doi: 10.1177/1460458219889798
56. Zheng Y, Rowell B, Chen Q, Kim JY, Kontar RA, Yang XJ, et al. Designing human-centered ai to prevent medication dispensing errors: focus group study with pharmacists. JMIR Format Res. (2023) 7:e51921. doi: 10.2196/51921
57. Cho I, Jin IS, Park H, Dykes PC. Clinical impact of an analytic tool for predicting the fall risk in inpatients: controlled interrupted time series. JMIR Med Informat. (2021) 9:11. doi: 10.2196/26456
58. Cho I, Kim M, Song MR, Dykes PC. Evaluation of an approach to clinical decision support for preventing inpatient falls: a pragmatic trial. JAMIA Open. (2023) 6:2. doi: 10.1093/jamiaopen/ooad019
59. Huang WR, Tseng RC, Chu WC. Establishing a prediction model by machine learning for accident-related patient safety. Wireless Commun Mobile Comput. 2022:1869252. (2022). doi: 10.1155/2022/1869252
60. Ladios-Martin M, Cabañero-Martínez MJ, Fernández-de-Maya J, Ballesta-López FJ, Belso-Garzas A, Zamora-Aznar FM, et al. Development of a predictive inpatient falls risk model using machine learning. J Nurs Manag. (2022) 30:3777–86. doi: 10.1111/jonm.13760
61. Lee T-T, Liu C-Y, Kuo Y-H, Mills ME, Fong J-G, Hung C. Application of data mining to the identification of critical factors in patient falls using a web-based reporting system. Int J Med Informat. (2011) 80:141–50. doi: 10.1016/j.ijmedinf.2010.10.009
62. Liu J, Wong ZSY, So HY, Tsui L. Evaluating resampling methods and structured features to improve fall incident report identification by the severity level. J Am Med Inform Assoc. (2021) 28:1756–64. doi: 10.1093/jamia/ocab048
63. Shim S, Yu JY, Jekal S, Song YJ, Moon KT, Lee JH, et al. Development and validation of interpretable machine learning models for inpatient fall events and electronic medical record integration. Clini Exp Emerg Med. (2022) 9:345–53. doi: 10.15441/ceem.22.354
64. Silva AP, Santos HDPD, Rotta ALO, Baiocco GG, Vieira R, Urbanetto JS. Drug-related fall risk in hospitals: a machine learning approach. Acta Paulista De Enfermagem. (2023) 36:771. doi: 10.37689/acta-ape/2023AO00771
65. Wang H-H, Huang C-C, Talley PC, Kuo K-M. Using healthcare resources wisely: a predictive support system regarding the severity of patient falls. J Healthc Eng. (2022) (2022) 2022:3100618. doi: 10.1155/2022/3100618
66. Wang L, Xue Z, Ezeana CF, Puppala M, Chen S, Danforth RL, et al. Preventing inpatient falls with injuries using integrative machine learning prediction: a cohort study. NPJ Digit Med. (2019) 2:3. doi: 10.1038/s41746-019-0200-3
67. Barghouthi ED, Owda AY, Asia M, Owda M. Systematic review for risks of pressure injury and prediction models using machine learning algorithms. Diagnostics. (2023) 13:17. doi: 10.3390/diagnostics13172739
68. Borlawsky T, Hripcsak G. Evaluation of an automated pressure ulcer risk assessment model. Home Health Care Managem Pract. (2007) 19: 272–84. doi: 10.1177/1084822307303566
69. Do Q, Lipatov K, Ramar K, Rasmusson J, Rasmusson J, Pickering BW, Herasevich V. Pressure injury prediction model using advanced analytics for at-risk hospitalized patients. J Patient Saf. (2022) 18:e1083–9. doi: 10.1097/PTS.0000000000001013
70. Ladios-Martin M, Fernández-de-Maya J, Ballesta-López F-J, Belso-Garzas A, Mas-Asencio M, Cabañero-Martínez MJ. Predictive modeling of pressure injury risk in patients admitted to an intensive care unit. Am J Critical Care. (2020) 29:e70–80. doi: 10.4037/ajcc2020237
71. Song W, Kang M-J, Zhang L, Jung W, Song J, Bates DW, et al. Predicting pressure injury using nursing assessment phenotypes and machine learning methods. J Am Med Inform Assoc. (2021) 28:759–65. doi: 10.1093/jamia/ocaa336
72. Toffaha KM, Simsekler MCE, Omar MA. Leveraging artificial intelligence and decision support systems in hospital-acquired pressure injuries prediction: a comprehensive review. Artif Intellig Med. (2023) 141:102560. doi: 10.1016/j.artmed.2023.102560
73. Simsekler MCE, Rodrigues C, Qazi A, Ellahham S, Ozonoff A. A comparative study of patient and staff safety evaluation using tree-based machine learning algorithms. Reliabil Eng Syst Safety. (2021) 208:107416. doi: 10.1016/j.ress.2020.107416
74. Murphy DR, Meyer AN, Sittig DF, Meeks DW, Thomas EJ, Singh H. Application of electronic trigger tools to identify targets for improving diagnostic safety. BMJ Qual Saf. (2019) 28:151–59. doi: 10.1136/bmjqs-2018-008086
75. Miniati R, Dori F, Iadanza E, et al. A methodology for technolgy risk assessment in hospitals. In IFMBE Proc. (2013) 39:736–39. doi: 10.1007/978-3-642-29305-4_193
76. Levis M, Westgate CL, Gui J, Watts BV, Shiner B. Natural language processing of clinical mental health notes may add predictive value to existing suicide risk models. Psychol Med. (2021) 51:1382–91. doi: 10.1017/S0033291720000173
77. Si HJ, Xu LR, Chen LY, Wang Q, Zhou R, Zhu AQ. Research on risk management optimization of artificial intelligence technology-assisted disinfection supply center. Arch Clin Psychiatry. (2023) 50:269–76. doi: 10.15761/0101-60830000000739
78. Huang X, Zhou S, Ma X, Jiang S, Xu Y, You Y, et al. Effectiveness of an artificial intelligence clinical assistant decision support system to improve the incidence of hospital-associated venous thromboembolism: a prospective, randomised controlled study. BMJ Open Quality. (2023) 12:4. doi: 10.1136/bmjoq-2023-002267
79. Choudhury A, Asan O. Role of artificial intelligence in patient safety outcomes: systematic literature review. JMIR Med Inform. (2020) 8:e18599. doi: 10.2196/18599
80. Regulation Regulation (EU) 2017/745 of the European Parliament and of the Council of 5 April 2017 on medical devices amending Directive 2001/83/EC Regulation (EC) No 178/2002 and Regulation (EC) No 1223/2009 and repealing Council Directives 90/385/EEC and 93/42/EEC. (2017). Available at: https://eur-lex.europa.eu/eli/reg/2017/745/oj (accessed 10 December, 2024).
Keywords: artificial intelligence, patient safety, healthcare, intelligent systems, machine learning
Citation: De Micco F, Di Palma G, Ferorelli D, De Benedictis A, Tomassini L, Tambone V, Cingolani M and Scendoni R (2025) Artificial intelligence in healthcare: transforming patient safety with intelligent systems—A systematic review. Front. Med. 11:1522554. doi: 10.3389/fmed.2024.1522554
Received: 04 November 2024; Accepted: 13 December 2024;
Published: 08 January 2025.
Edited by:
Cristiana Sessa, Oncology Institute of Southern Switzerland (IOSI), SwitzerlandReviewed by:
Ioannis Alagkiozidis, Maimonides Medical Center, United StatesCopyright © 2025 De Micco, Di Palma, Ferorelli, De Benedictis, Tomassini, Tambone, Cingolani and Scendoni. This is an open-access article distributed under the terms of the Creative Commons Attribution License (CC BY). The use, distribution or reproduction in other forums is permitted, provided the original author(s) and the copyright owner(s) are credited and that the original publication in this journal is cited, in accordance with accepted academic practice. No use, distribution or reproduction is permitted which does not comply with these terms.
*Correspondence: Gianmarco Di Palma, Zy5kaXBhbG1hQHBvbGljbGluaWNvY2FtcHVzLml0
Disclaimer: All claims expressed in this article are solely those of the authors and do not necessarily represent those of their affiliated organizations, or those of the publisher, the editors and the reviewers. Any product that may be evaluated in this article or claim that may be made by its manufacturer is not guaranteed or endorsed by the publisher.
Research integrity at Frontiers
Learn more about the work of our research integrity team to safeguard the quality of each article we publish.