- Shenzhen Eye Hospital, Shenzhen, China
Objectives: To explore machine-learning applications in myopia prediction and analyze the influencing factors of myopia.
Methods: Stratified cluster random sampling was used to select elementary school students in Shenzhen, China for inclusion in this case-control study. Myopia screening, ocular biological parameter measurements, and questionnaires were conducted. Random forest (RF), decision tree (DT), extreme gradient boosting trees (XGBoost), support vector machine (SVM), and logistic regression (LR) algorithms were used to construct five myopia prediction models using R software (version 4.3.0). These myopia prediction models were used to investigate the relationship between ocular biological parameters, environmental factors, behavioral factors, genetic factors, and myopia.
Results: This study included 2,947 elementary school students, with a myopia prevalence rate of 47.2%. All five prediction models had an area under the receiver operating characteristic curve (AUC) above 0.75, with prediction accuracy and precision exceeding 0.70. The AUCs in the testing set were 0.846, 0.837, 0.833, and 0.815 for SVM, LR, RF, and XGBoost, respectively, indicating their superior predictive performance to that of DT (0.791). In the RF model, the five most important variables were axial length, age, sex, maternal myopia, and feeding pattern. LR identified axial length was the most significant risk factor for myopia [odds ratio (OR) =8.203], followed by sex (OR = 2.349), maternal myopia (OR = 1.437), Reading and writing posture (OR = 1.270), infant feeding pattern (OR = 1.207), and age (OR = 1.168); corneal radius (OR = 0.034) and anterior chamber depth (OR = 0.516) served as protective factors.
Conclusion: Myopia prediction models based on machine learning demonstrated favorable predictive performance and accurately identified myopia risk factors, and may therefore aid in the implementation of myopia prevention and control measures among high-risk individuals.
Introduction
Myopia is a global public health concern (1) that affected 1.4 billion individuals worldwide in 2020 with a prevalence of 22.9%. It has been projected that this number will increase to 4.7 billion individuals by 2050, resulting in a prevalence of 49.8% (2). In 2020, 52.7% of children and adolescents in China were myopic (3), which contributed to the position of China as the country with the highest number of individuals with myopia (4). Myopia often develops during childhood and adolescence, and myopia development during this period is more likely to progress to high myopia, increasing the risk of various eye diseases such as glaucoma, cataracts, macular degeneration, and retinal detachment. High or pathological myopia significantly increases the risk of blindness (5) and is a major cause of blindness (6). Myopia imposes an economic burden on society (7). In Singapore, an average of $148 is spent on myopia-related costs per adolescent with myopia per year (8). Globally, uncorrected refractive errors result in an estimated annual economic loss of approximately $202 billion (9).
Currently, the etiology of myopia remains unclear, although its development in children and adolescents is thought to be influenced by a combination of environmental, behavioral, and genetic factors (10). Myopia has been associated with sex (10), age (10), near work (11, 12), time spent outdoors (10), duration of electronic device use (10), sleep (13), and parental myopia (14). Exploring influencing factors of myopia occurrence and development and conducting myopia risk assessments are of significant importance for improving the ocular health of children and adolescents. Owing to potential interactions among various influencing factors, such as the co-occurrence of increased screen time, long periods of near work, and reduced time spent outdoors, conventional statistical methods may fail to identify covariance and potential confounding factors (15). Therefore, novel analytical methods are required to mitigate the influence of confounding factors, identify covariates, and measure the magnitude and significance of interactions between variables.
Continuous advancements in computer technology have increased the use of artificial intelligence (AI) in many fields of medicine, including ophthalmology (16). The application of AI in the study of ophthalmic diseases has evolved from its initial focus on diabetic retinopathy (17), age-related macular degeneration (18), and glaucoma (19) to encompass anterior segment disorders such as refractive errors (20). Machine learning, a core component of AI, is increasingly used in the diagnosis, treatment, and prognostic assessment of various diseases (21) and has recently been applied to myopia research, including in the prediction of axial length and identification of influencing factors (21–25). However, the use of machine learning for the prediction of myopia risk remains relatively rare. Previous myopia prediction studies based on machine learning have often failed to comprehensively include feature variables and targeted indicators (26). This study therefore constructed myopia prediction models using multiple indicators, including behavioral habits, dietary habits, genetic factors, and ocular biological parameters, and five machine-learning algorithms: random forest (RF), decision tree (DT), extreme gradient boosting trees (XGBoost), support vector machine (SVM), and logistic regression (LR). The aims of the study were to explore machine-learning applications in myopia prediction, analyze the factors influencing myopia, and provide scientific evidence for targeted myopia prevention, control measures, and policy recommendations.
Methods
Sample size
Sample size was estimated in accordance with the methodology outlined by Riley et al. (27) using the pmsampsize() function in R software (The R Foundation for Statistical Computing, Vienna, Austria). This calculation determined that a minimum of 2,060 study participants were required, with the non-response rate set at 20%.
Study participants
A stratified cluster sampling method was used to select the study population of 2,947 primary school students (744, 528, 527, 360, 459, and 329 in grades 1, 2, 3, 4, 5, and 6, respectively) from Shenzhen in June 2022. The study was approved by the Ethics Committee of Shenzhen Eye Hospital (Approval No. 20201230-06), and informed consent was obtained from the legal guardians of all the participants.
The inclusion criteria were as follows: (1) current enrollment in a primary school in Shenzhen, (2) ability to cooperate with ophthalmic examinations, and (3) guardians able to complete questionnaires. The exclusion criteria were as follows: (1) presence of organic eye diseases, and (2) inability to complete ophthalmic examinations.
Data collection
Myopia screening
Myopia screening was performed using visual acuity tests and dioptric detection, and conducted by ophthalmologists following the Chinese Health Standard (WS/T 663-2020) (28). Visual acuity was assessed using visual acuity charts, and non-cycloplegic refraction was performed using an autorefractometer. Autorefraction was performed three times per eye, and the average value was calculated to obtain a reliable refractive error reading for each participant.
Ocular biometric measurements
The ocular biometric parameters of the study participants were measured using an optical biometer. The measured parameters included axial length, central corneal thickness, corneal radius, anterior chamber depth, and lens thickness.
Questionnaire
A questionnaire was developed to include the following content: (1) sociodemographic characteristics, including sex, age, height, and weight; (2) parental myopia status; (3) dietary habits, including the frequency of consumption of sugary foods, sugary beverages, fried foods, vegetables, and fruits; and (4) behavioral factors, including reading and writing posture, duration of continuous reading and writing, sleep duration, and time spent outdoors. Teachers distributed the questionnaires to the guardians of the study participants, who completed them online.
The questionnaire included items assessing adherence to the traditional Chinese guideline known as “yi quan, yi chi, yi cun,” which translates to “one fist, one foot, one inch.” This principle relates to specific distances that should be maintained during reading and writing to promote proper posture and reduce the risk of myopia among schoolchildren. One fist: a distance of one fist (approximately 10 cm) is advised between the chest of a student and the edge of their desk. One foot: a distance of one foot (approximately 33 cm) should be maintained between the eyes of the student and their reading material. One inch: pens or pencils should be held at a distance of one inch (approximately 3.3 cm) from the tip.
Diagnostic criteria for myopia
Participants with an uncorrected visual acuity <5.0 and spherical equivalent refraction < −0.50 diopter and Participants those wore orthokeratology lenses were defined as myopic (28). Myopia was classified based on the spherical equivalent refraction of the right eye. Mild myopia is defined as −3.00 diopter ≤spherical equivalent refraction <−0.50 diopter, moderate myopia is defined as −6.00 diopter ≤spherical equivalent refraction <−3.00 diopter, and high myopia is defined as spherical equivalent refraction <−6.00 diopter.
Construction and evaluation of prediction models
Feature variables
Multivariate logistic regression was used to identify the statistically significant influencing factors of myopia, namely feature variables.
Data splitting
Stratified random sampling (according to myopia status) was used to classify the 2,947 study participants into training (2,064 students) and testing sets (883 students) at a ratio of 7:3.
Data modeling
Feature variables were incorporated into the machine-learning model. The five machine-learning algorithms RF, DT, XGBoost, SVM, and LR were used to construct myopia prediction models from the training data set. The machine-learning modeling process is illustrated in Figure 1.
Model evaluation
The testing set was used to determine model performance metrics such as accuracy, precision, recall, and F1-score. The receiver operating characteristic (ROC) curves were plotted and used to calculate the area under the curve (AUC) and 95% confidence interval. The DeLong test (29) was conducted to assess and compare the performance of the models.
Analysis of feature variable importance
The importance of independent variables reflects their relationship with the dependent variable and explains their contribution to the predictive performance of the model. Mean decrease accuracy and odds ratios (ORs) were used to assess the importance of feature variables to the prediction models. The OR represents the odds of an event in group 1 compared to those in group 2, where odds means the event over non-event.
Statistical analysis
Machine-learning prediction models were constructed, and statistical analyses were performed using R software (version 4.3.0). was used to describe for normally distributed continuous data; intergroup comparisons were performed using independent samples t-tests. Non-normally distributed continuous data are expressed as P50 (P25, P75), and intergroup comparisons were conducted using the Wilcoxon rank-sum test. Count data are presented as frequencies, and intergroup comparisons were performed using the chi-squared test. The significance level (α) for all tests was set at 0.05, with p < 0.05 indicating statistical significance.
Results
Basic information
The study included 2,947 primary school students (1,617 boys and 1,330 girls). The minimum age of the participants was 6 years, and their maximum age was 12 years, with a 50th percentile of 9 years. The prevalence of myopia among the participants was 47.2%. The prevalence of myopia increased with age, and was 24.1, 33.3, 52.6, 55.6, 67.8, and 75.7% in grades 1, 2, 3, 4, 5, and 6, respectively (χ2 = 436.475, p < 0.001). The prevalence of myopia was significantly higher in girls (50.5%) than in boys (44.5%) (χ2 = 10.539, p < 0.001). Myopia became more severe with age, with the prevalence of moderate myopia increasing significantly from 0.7% at the age of 6 years to 26.3% at the age of 12 years (Figure 2).
Feature variables
Logistic regression analysis identified eight statistically significant influencing factors of myopia: age (OR = 1.168), sex (OR = 2.349), maternal myopia (OR = 1.437), infant feeding pattern (OR = 1.207), reading and writing posture (OR = 1.270), axial length (OR = 8.203), anterior chamber depth (OR = 0.516), and corneal radius (OR = 0.034) (Figure 3).
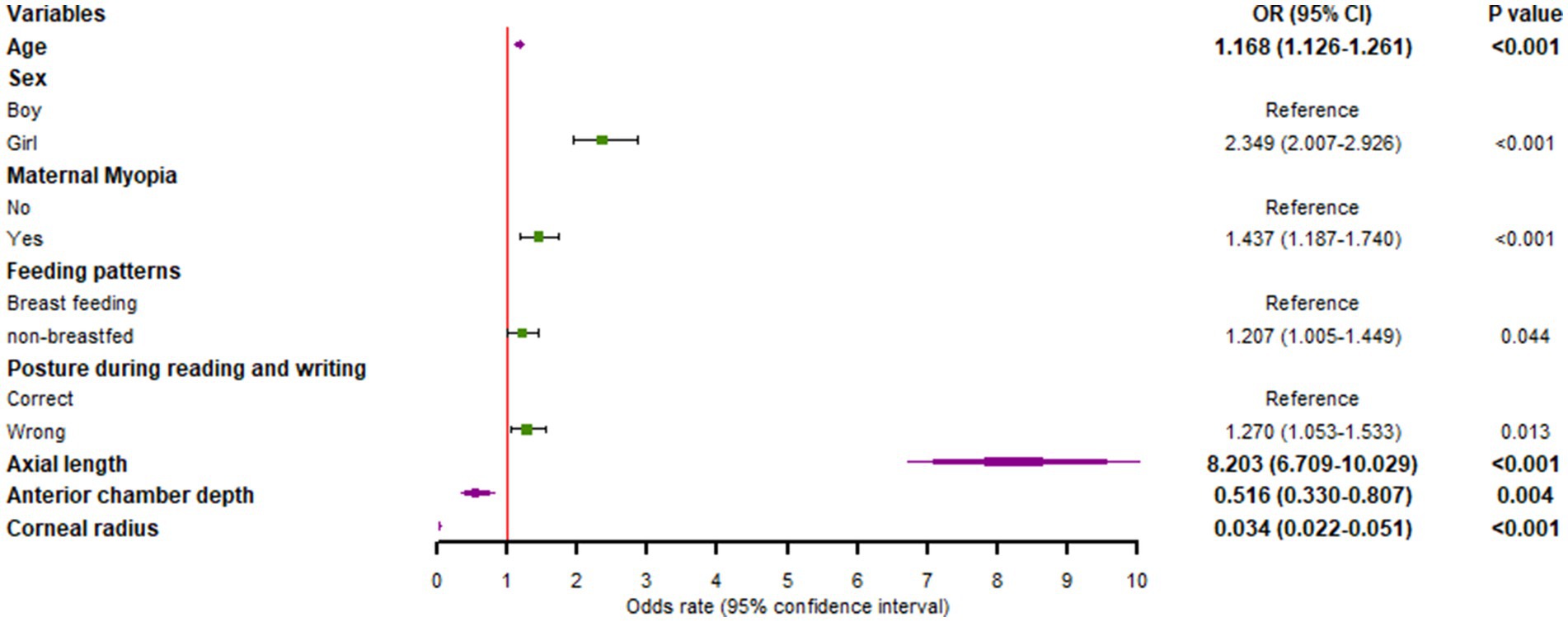
Figure 3. Odds ratios of feature variables in the myopia prediction model based on logistic regression.
Comparison of training and testing sets
The quantitative data were not normally distributed and are presented as P50 (P25, P75) (Figure 4). The prevalence of myopia and feature variables did not differ significantly between the training and testing sets (p < 0.05), indicating a balanced allocation between the two datasets (Table 1).
Evaluation of prediction models
The RF, DT, XGBoost, SVM, and LR prediction models were constructed using the training set and evaluated using testing set. The AUCs of the ROC curves were greater than 0.8 for all the models except the DT. Among the models, the SVM exhibited the highest accuracy, precision, recall, and F1-score, indicating a superior predictive performance for myopia (Table 2 and Figure 5).
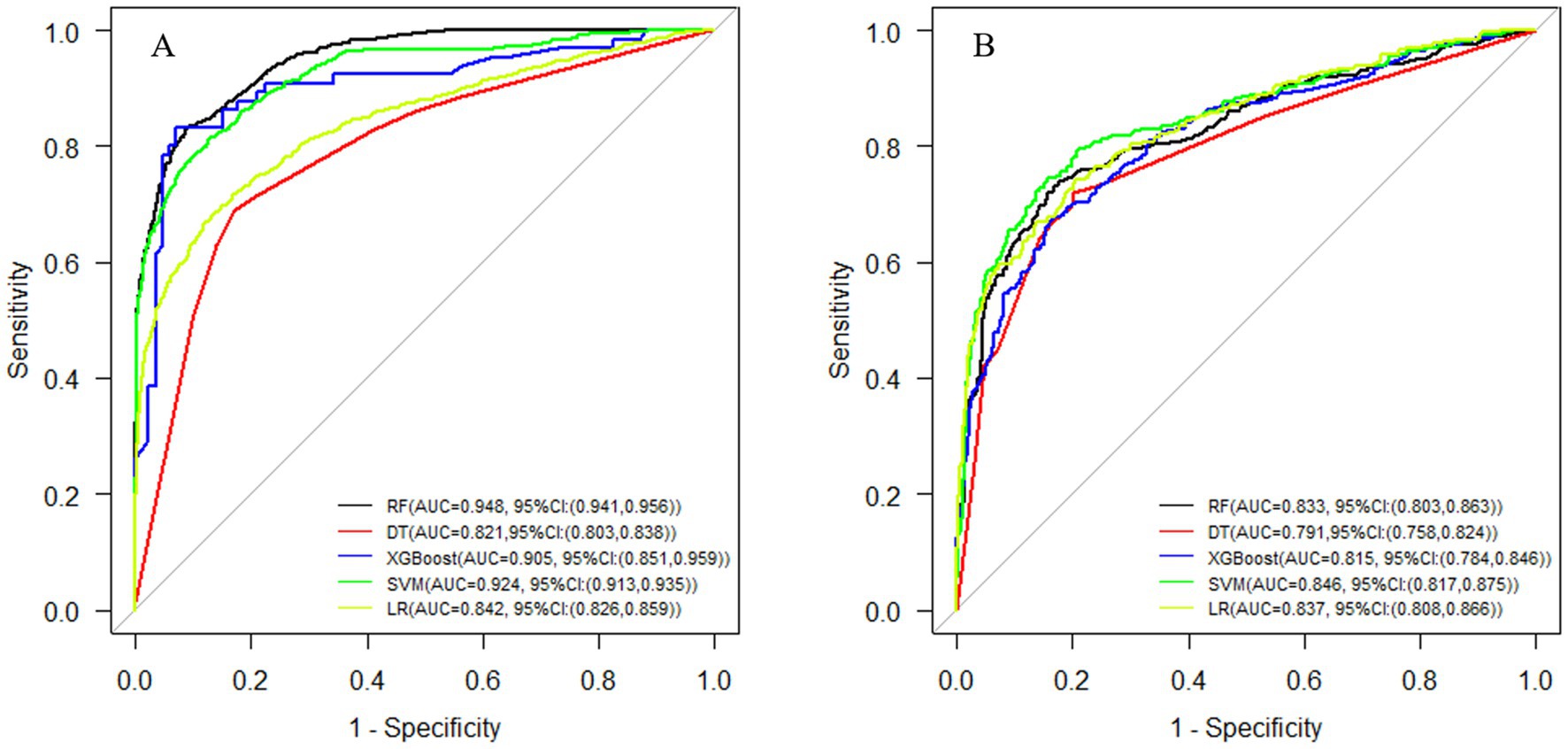
Figure 5. ROC curves of different machine-learning models. (A) Training set. (B) Testing set. ROC, receiver operating characteristic.
Importance of feature variables
As shown in Figure 5, the optimal prediction model in the training set was RF, whereas in the testing set the optimal myopia prediction model was SVM, followed by LR. Analysis of variable importance in the RF model revealed axial length, age, sex, maternal myopia, and infant feeding pattern to be the five most important variables, as shown in Figure 6.
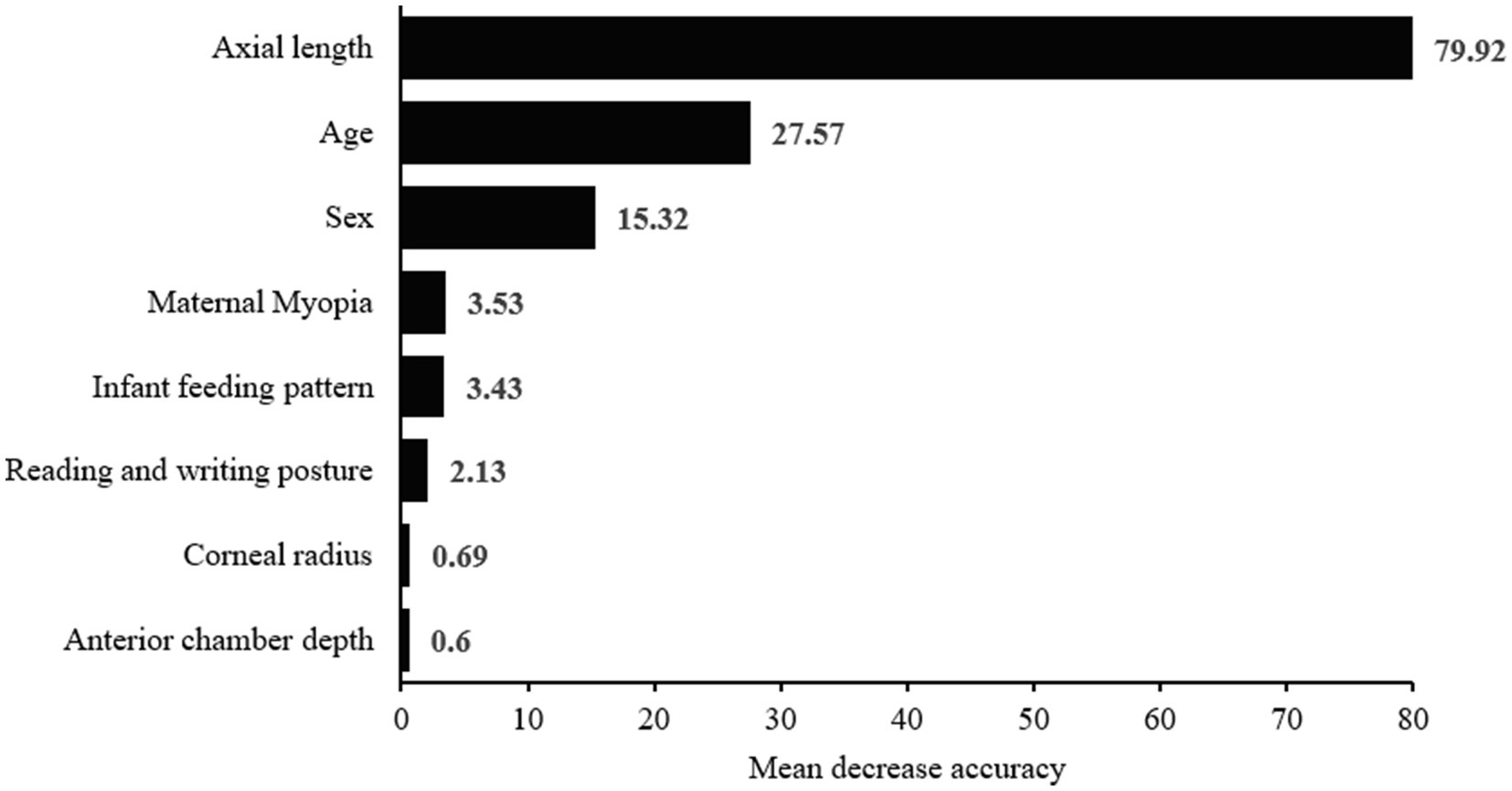
Figure 6. Importance of feature variables for the prediction model constructed using the random forest algorithm.
Discussion
In this study, the prevalence of myopia among 2,947 primary school students was 47.2%, with rates increasing with age. Five machine-learning algorithms were used to construct myopia risk prediction models that all achieved AUC values over 0.75 and accuracy and precision values over 0.7. The AUCs for prediction models created using the SVM, LR, RF, and XGBoost algorithms were 0.846, 0.837, 0.833, and 0.815, respectively, indicating superior predictive performance compared to that of the prediction models generated using the DT (AUC, 0.791) and XGBoost (AUC, 0.815) algorithms.
The machine-learning models in this study outperformed those constructed in previous studies. A study of primary school students in Jiamusi, China reported AUCs of 0.710, 0.606, 0.682, and 0.620 for RF, DT, XGBoost, and SVM models, respectively (30). A study of primary and secondary school students in Chengdu, China reported AUCs of 0.768, 0.767, and 0.769 for LR, XGBoost, and SVM models, respectively (26). One possible reason for this difference is that the previous studies involved a relatively narrow selection of feature variables, with one relying solely on questionnaires to collect information on participant behaviors (26, 30). The present study collected addition information on the ocular biometric parameters closely associated with myopia.
Analysis of the importance of feature variables in myopia prediction models in the present study and revealed a close association between ocular biometric parameters and myopia. The refractive status of the eye has been shown to be determined by the lens, axial length, and corneal radius (31), with axial length and corneal curvature being the most crucial factors affecting ocular refraction in children and adolescents (32, 33). This is consistent with the findings of the present study, which showed that axial length, anterior chamber depth, and corneal radius were the principal biological parameters influencing myopia. Refractive errors result from a mismatch between the imaging focal length and the axial length. The active mechanism of emmetropization involves axial elongation, whereas the passive mechanism primarily involves the regulation of corneal and lens diopters (34). Axial length gradually increases with age, with a 1-mm increase in axial length corresponding to a myopic shift of 0.69D in spherical equivalent refraction (34). Studies have identified that the axial length/corneal radius ratio as an important parameter for determining myopia in children and adolescents, significantly superior to axial length alone (34). However, the axial length/corneal radius ratio threshold for myopia determination varies among different populations, with reported optimal thresholds of 2.906 for Australian children and adolescents (35) and 3.0 for Singaporean children and adolescents (36). Changes in anterior chamber depth are inversely proportional to lens thickness, with studies indicating that, anterior chamber depth increases by up to 0.18 mm due to lens deformation and displacement following ciliary muscle paralysis (34).
Studies have reported associations between dietary habits (37), sleep (38), near work (39), parental myopia (39), and time spent outdoors (40) and the occurrence and progression of myopia. The present study assessed the influence of environmental and behavioral factors on myopia through questionnaires, and identified sex as a significant factor, with girls at a greater risk of myopia than boys (41). Consistent with this, the probability of myopia in East Asian females has been shown to be twice that in age-matched males, although studies in South Asian and Latin American populations did not find sex to be a significant factor (42). The relationship between myopia and sex may be complicated by factors such as education, time spent outdoors, and economic conditions. The present study also identified parental myopia, specifically maternal myopia, as a significant influencing factor of myopia. Whole-genome studies have associated more than 150 nucleotide polymorphisms with myopia (43).
In the present study, an incorrect reading and writing posture was found to increase the risk of myopia. This is consistent with previous research involving children aged 9–11 years, which showed that children who read and wrote at a distance of less than 30 cm had higher degrees of myopia and greater progression of myopia over a 6-month period (44). This may be the result of a compensatory mechanism, whereby children with existing myopia hold reading materials closer to see more clearly, further exacerbating myopia. The association between breastfeeding and myopia has been receiving increasing attention. The present study identified infant feeding pattern as an influencing factor of myopia. A previous study of children aged 6–12 years found that myopia prevalence was lower among breastfed children (51.8%) than among non-breastfed children (64.7%) (45). Aksoy et al. (46) demonstrated that in first- and second-grade elementary school students, non-breastfed children were more likely to have refractive errors than were children who were exclusively or partially breastfed. The present study additionally found that body mass index (BMI) influenced myopia in the RF model. Large-scale population studies have shown a J-shaped relationship between BMI and myopia, with both low and high BMI associated with myopia (47).
The present study has some limitations. First, the diagnosis of myopia was based on non-cycloplegic refraction. Non-cycloplegic refraction has been shown to have high sensitivity and specificity in myopia screening among students (48), and myopia prevalence may have been overestimated to some extent. Second, the use of a questionnaire introduces the possibility of recall bias. Finally, the case-control design of the study limited its ability to determine causal relationships. Future prospective cohort studies are required to further elucidate the etiology of myopia using machine-learning algorithms.
In conclusion, the present study identified axial elongation, female sex, thickening of the lens, maternal myopia, incorrect reading and writing posture, and increased age as risk factors for myopia; corneal radius was a protective factor against myopia. Machine-learning models constructed using these factors accurately predicted myopia and may be used to identify high-risk populations. Cohort studies will be conducted to validate the identified myopia risk factors and elucidate their causal relationships.
Data availability statement
The original contributions presented in the study are included in the article/supplementary material, further inquiries can be directed to the corresponding author.
Ethics statement
The studies involving humans were approved by the Ethics Committee of the Shenzhen Eye Hospital (No. 20201230-06). The studies were conducted in accordance with the local legislation and institutional requirements. Written informed consent for participation in this study was provided by the participants’ legal guardians/next of kin.
Author contributions
JM: Writing – review & editing, Writing – original draft, Supervision, Methodology, Investigation. HZ: Writing – original draft, Investigation, Formal analysis. MJ: Writing – original draft, Investigation, Data curation.
Funding
The author(s) declare that financial support was received for the research, authorship, and/or publication of this article. This work was supported by Shenzhen Science and Technology Program (JCYJ20230807114608016).
Conflict of interest
The authors declare that the research was conducted in the absence of any commercial or financial relationships that could be construed as a potential conflict of interest.
Publisher’s note
All claims expressed in this article are solely those of the authors and do not necessarily represent those of their affiliated organizations, or those of the publisher, the editors and the reviewers. Any product that may be evaluated in this article, or claim that may be made by its manufacturer, is not guaranteed or endorsed by the publisher.
References
1. Morgan, IG, French, AN, Ashby, RS, Guo, X, Ding, X, He, M, et al. The epidemics of myopia: aetiology and prevention. Prog Retin Eye Res. (2018) 62:134–49. doi: 10.1016/j.preteyeres.2017.09.004
2. Holden, BA, Fricke, TR, Wilson, DA, Jong, M, Naidoo, KS, Sankaridurg, P, et al. Global prevalence of myopia and high myopia and temporal trends from 2000 through 2050. Ophthalmology. (2016) 123:1036–42. doi: 10.1016/j.ophtha.2016.01.006
3. Mu, J, Zhong, H, Liu, M, Jiang, M, Shuai, X, Chen, Y, et al. Trends in myopia development among primary and secondary school students during the COVID-19 pandemic: a large-scale cross-sectional study. Front Public Health. (2022) 10:859285. doi: 10.3389/fpubh.2022.859285
4. Sun, HP, Li, A, Xu, Y, and Pan, CW. Secular trends of reduced visual acuity from 1985 to 2010 and disease burden projection for 2020 and 2030 among primary and secondary school students in China. JAMA Ophthalmol. (2015) 133:262–8. doi: 10.1001/jamaophthalmol.2014.4899
5. Morgan, IG, Ohno-Matsui, K, and Saw, SM. Myopia. Lancet. (2012) 379:1739–48. doi: 10.1016/S0140-6736(12)60272-4
6. Wu, PC, Huang, HM, Yu, HJ, Fang, PC, and Chen, CT. Epidemiology of myopia. Asia Pac J Ophthalmol. (2016) 5:386–93. doi: 10.1097/APO.0000000000000236
7. Rahi, JS, Cumberland, PM, and Peckham, CS. Myopia over the lifecourse: prevalence and early life influences in the 1958 British birth cohort. Ophthalmology. (2011) 118:797–804. doi: 10.1016/j.ophtha.2010.09.025
8. Lim, MC, Gazzard, G, Sim, EL, Tong, L, and Saw, SM. Direct costs of myopia in Singapore. Eye. (2009) 23:1086–9. doi: 10.1038/eye.2008.225
9. Fricke, TR, Holden, BA, Wilson, DA, Schlenther, G, Naidoo, KS, Resnikoff, S, et al. Global cost of correcting vision impairment from uncorrected refractive error. Bull World Health Organ. (2012) 90:728–38. doi: 10.2471/BLT.12.104034
10. Mu, J, Zeng, D, Fan, J, Liu, M, Jiang, M, Shuai, X, et al. Epidemiological characteristics and influencing factors of myopia among primary school students in southern China: a longitudinal study. Int J Public Health. (2023) 68:1605424. doi: 10.3389/ijph.2023.1605424
11. Gopalakrishnan, A, Hussaindeen, JR, Sivaraman, V, Swaminathan, M, Wong, YL, Armitage, JA, et al. Myopia and its association with near work, outdoor time, and housing type among schoolchildren in South India. Optom Vis Sci. (2023) 100:105–10. doi: 10.1097/OPX.0000000000001975
12. Philipp, D, Vogel, M, Brandt, M, Rauscher, FG, Hiemisch, A, Wahl, S, et al. The relationship between myopia and near work, time outdoors and socioeconomic status in children and adolescents. BMC Public Health. (2022) 22:2058. doi: 10.1186/s12889-022-14377-1
13. Zhou, Z, Morgan, IG, Chen, Q, Jin, L, He, M, and Congdon, N. Disordered sleep and myopia risk among Chinese children. PLoS One. (2015) 10:e0121796. doi: 10.1371/journal.pone.0121796
14. Ip, JM, Huynh, SC, Robaei, D, Rose, KA, Morgan, IG, Smith, W, et al. Ethnic differences in the impact of parental myopia: findings from a population-based study of 12-year-old Australian children. Invest Ophthalmol Vis Sci. (2007) 48:2520–8. doi: 10.1167/iovs.06-0716
15. Cortina-Borja, M, Smith, AD, Combarros, O, and Lehmann, DJ. The synergy factor: a statistic to measure interactions in complex diseases. BMC Res Notes. (2009) 2:105. doi: 10.1186/1756-0500-2-105
16. Ting, DSW, Peng, L, Varadarajan, AV, Keane, PA, Burlina, PM, Chiang, MF, et al. Deep learning in ophthalmology: the technical and clinical considerations. Prog Retin Eye Res. (2019) 72:100759. doi: 10.1016/j.preteyeres.2019.04.003
17. Abràmoff, MD, Lou, Y, Erginay, A, Clarida, W, Amelon, R, Folk, JC, et al. Improved automated detection of diabetic retinopathy on a publicly available dataset through integration of deep learning. Invest Ophthalmol Vis Sci. (2016) 57:5200–6. doi: 10.1167/iovs.16-19964
18. Schlegl, T, Waldstein, SM, Bogunovic, H, Endstraßer, F, Sadeghipour, A, Philip, AM, et al. Fully automated detection and quantification of macular fluid in OCT using deep learning. Ophthalmology. (2018) 125:549–58. doi: 10.1016/j.ophtha.2017.10.031
19. Asaoka, R, Murata, H, Iwase, A, and Araie, M. Detecting preperimetric glaucoma with standard automated perimetry using a deep learning classifier. Ophthalmology. (2016) 123:1974–80. doi: 10.1016/j.ophtha.2016.05.029
20. Rampat, R, Deshmukh, R, Chen, X, Ting, DSW, Said, DG, Dua, HS, et al. Artificial intelligence in cornea, refractive surgery, and cataract: basic principles, clinical applications, and future directions. Asia Pac J Ophthalmol. (2021) 10:268–81. doi: 10.1097/APO.0000000000000394
21. Bengio, Y, Courville, A, and Vincent, P. Representation learning: a review and new perspectives. IEEE Trans Pattern Anal Mach Intell. (2013) 35:1798–828. doi: 10.1109/TPAMI.2013.50
22. Tang, T, Yu, Z, Xu, Q, Peng, Z, Fan, Y, Wang, K, et al. A machine learning-based algorithm used to estimate the physiological elongation of ocular axial length in myopic children. Eye Vis. (2020) 7:50. doi: 10.1186/s40662-020-00214-2
23. Tu, Y, Hu, X, Zeng, C, Ye, M, Zhang, P, Jin, X, et al. A machine-learning approach to discerning prevalence and causes of myopia among elementary students in Hubei. Int Ophthalmol. (2022) 42:2889–902. doi: 10.1007/s10792-022-02279-5
24. Li, SM, Ren, MY, Gan, J, Zhang, SG, Kang, MT, Li, H, et al. Machine learning to determine risk factors for myopia progression in primary school children: the Anyang Childhood Eye Study. Ophthalmol Ther. (2022) 11:573–85. doi: 10.1007/s40123-021-00450-2
25. Lu, HC, Chen, HY, Huang, CJ, Chu, PH, Wu, LS, and Tsai, CY. Predicting axial length from choroidal thickness on optical coherence tomography images with machine learning based algorithms. Front Med. (2022) 9:850284. doi: 10.3389/fmed.2022.850284
26. Xiao, J, Liu, M, Huang, Q, Sun, Z, Ning, L, Duan, J, et al. Analysis and modeling of myopia-related factors based on questionnaire survey. Comput Biol Med. (2022) 150:106162. doi: 10.1016/j.compbiomed.2022.106162
27. Riley, RD, Ensor, J, Snell, KIE, Harrell, FE Jr, Martin, GP, Reitsma, JB, et al. Calculating the sample size required for developing a clinical prediction model. BMJ. (2020) 368:m441. doi: 10.1136/bmj.m441
28. National Health Commission of the People’s Republic of China . Specification for screening of refractive error in primary and secondary school students (WS/T 663-2020). Beijing: National Health Commission of the People’s Republic of China (2020).
29. DeLong, ER, DeLong, DM, and Clarke-Pearson, DL. Comparing the areas under two or more correlated receiver operating characteristic curves: a nonparametric approach. Biometrics. (1988) 44:837–45. doi: 10.2307/2531595
30. Tong, HJ, Huang, ZM, Li, YL, Chen, YM, Tian, B, Ding, LL, et al. Machine learning to analyze the factors influencing myopia in students of different school periods. Front Public Health. (2023) 11:1169128. doi: 10.3389/fpubh.2023.1169128
31. Grosvenor, T, and Goss, DA. Role of the cornea in emmetropia and myopia. Optom Vis Sci. (1998) 75:132–45. doi: 10.1097/00006324-199802000-00017
32. Scheiman, M, Gwiazda, J, Zhang, Q, Deng, L, Fern, K, Manny, RE, et al. Longitudinal changes in corneal curvature and its relationship to axial length in the Correction of Myopia Evaluation Trial (COMET) cohort. J Optom. (2016) 9:13–21. doi: 10.1016/j.optom.2015.10.003
33. Ip, JM, Huynh, SC, Kifley, A, Rose, KA, Morgan, IG, Varma, R, et al. Variation of the contribution from axial length and other oculometric parameters to refraction by age and ethnicity. Invest Ophthalmol Vis Sci. (2007) 48:4846–53. doi: 10.1167/iovs.07-0101
34. Liu, W, Liu, W, and Wang, C. Ocular biometric parameters of mild hyperopia to mild myopia children aged 6–14 years from Wenzhou optometry center: a cross-sectional study. Front Med. (2022) 9:992587. doi: 10.3389/fmed.2022.992587
35. Ojaimi, E, Rose, KA, Morgan, IG, Smith, W, Martin, FJ, Kifley, A, et al. Distribution of ocular biometric parameters and refraction in a population-based study of Australian children. Invest Ophthalmol Vis Sci. (2005) 46:2748–54. doi: 10.1167/iovs.04-1324
36. Saw, SM, Chua, WH, Hong, CY, Wu, HM, Chia, KS, Stone, RA, et al. Height and its relationship to refraction and biometry parameters in Singapore Chinese children. Invest Ophthalmol Vis Sci. (2002) 43:1408–13.
37. Li, M, Tan, CS, Foo, LL, Sugianto, R, Toh, JY, Sun, CH, et al. Dietary intake and associations with myopia in Singapore children. Ophthalmic Physiol Opt. (2022) 42:319–26. doi: 10.1111/opo.12929
38. Li, M, Tan, CS, Xu, L, Foo, LL, Yap, F, Sun, CH, et al. Sleep patterns and myopia among school-aged children in Singapore. Front Public Health. (2022) 10:828298. doi: 10.3389/fpubh.2022.828298
39. Pärssinen, O, and Kauppinen, M. Associations of near work time, watching TV, outdoors time, and parents' myopia with myopia among school children based on 38-year-old historical data. Acta Ophthalmol. (2022) 100:e430–8. doi: 10.1111/aos.14980
40. Gupta, S, Joshi, A, Saxena, H, and Chatterjee, A. Outdoor activity and myopia progression in children: a follow-up study using mixed-effects model. Indian J Ophthalmol. (2021) 69:3446–50. doi: 10.4103/ijo.IJO_3602_20
41. Zhang, Y, Qiu, K, and Zhang, Q. Ametropia prevalence of primary school students in Chinese multi-ethnic regions. Strabismus. (2020) 28:13–6. doi: 10.1080/09273972.2019.1665691
42. Rudnicka, AR, Kapetanakis, VV, Wathern, AK, Logan, NS, Gilmartin, B, Whincup, PH, et al. Global variations and time trends in the prevalence of childhood myopia, a systematic review and quantitative meta-analysis: implications for aetiology and early prevention. Br J Ophthalmol. (2016) 100:882–90. doi: 10.1136/bjophthalmol-2015-307724
43. Morgan, IG, and Rose, KA. Myopia: is the nature-nurture debate finally over? Clin Exp Optom. (2019) 102:3–17. doi: 10.1111/cxo.12845
44. Huang, PC, Hsiao, YC, Tsai, CY, Tsai, DC, Chen, CW, Hsu, CC, et al. Protective behaviours of near work and time outdoors in myopia prevalence and progression in myopic children: a 2-year prospective population study. Br J Ophthalmol. (2020) 104:956–61. doi: 10.1136/bjophthalmol-2019-314101
45. Liu, S, Ye, S, Wang, Q, Cao, Y, and Zhang, X. Breastfeeding and myopia: a cross-sectional study of children aged 6-12 years in Tianjin, China. Sci Rep. (2018) 8:10025. doi: 10.1038/s41598-018-27878-0
46. Aksoy, A, Ozdemir, M, Aslan, L, Aslankurt, M, and Gul, O. Effect of breast feeding on ocular morbidity. Med Sci Monit. (2014) 20:24–7. doi: 10.12659/msm.889879
47. Peled, A, Nitzan, I, Megreli, J, Derazne, E, Tzur, D, Pinhas-Hamiel, O, et al. Myopia and BMI: a nationwide study of 1.3 million adolescents. Obesity. (2022) 30:1691–8. doi: 10.1002/oby.23482
Keywords: machine learning, myopia, influencing factors, children and adolescents, predictive model
Citation: Mu J, Zhong H and Jiang M (2024) Machine-learning models to predict myopia in children and adolescents. Front. Med. 11:1482788. doi: 10.3389/fmed.2024.1482788
Edited by:
Xinyu Liu, Singapore Eye Research Institute (SERI), SingaporeReviewed by:
Zhengcun Pei, Tianjin University, ChinaBhim Bahadur Rai, Australian National University, Australia
Copyright © 2024 Mu, Zhong and Jiang. This is an open-access article distributed under the terms of the Creative Commons Attribution License (CC BY). The use, distribution or reproduction in other forums is permitted, provided the original author(s) and the copyright owner(s) are credited and that the original publication in this journal is cited, in accordance with accepted academic practice. No use, distribution or reproduction is permitted which does not comply with these terms.
*Correspondence: Jingfeng Mu, MTAxNDEyMDMwMEBxcS5jb20=
†These authors have contributed equally to this work