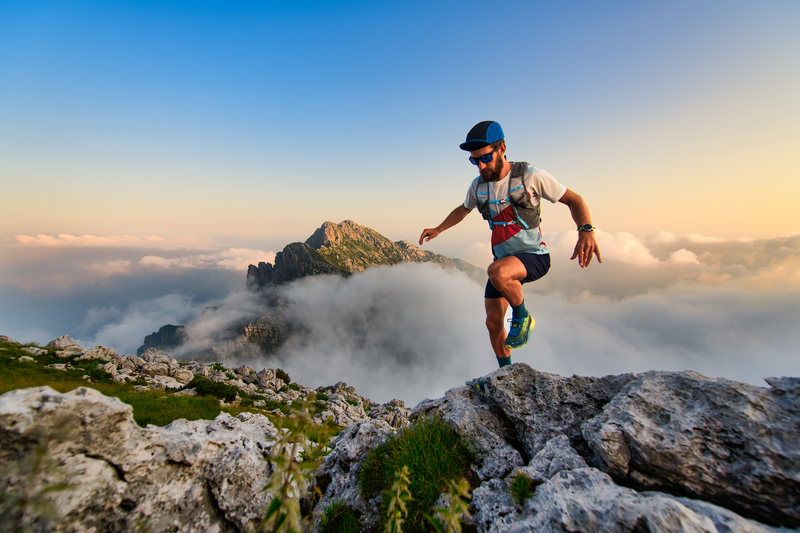
95% of researchers rate our articles as excellent or good
Learn more about the work of our research integrity team to safeguard the quality of each article we publish.
Find out more
REVIEW article
Front. Med. , 19 November 2024
Sec. Precision Medicine
Volume 11 - 2024 | https://doi.org/10.3389/fmed.2024.1452298
Acute and requiring attention problem of oncotheranostics is a necessity for the urgent development of operative and precise diagnostics methods, followed by efficient therapy, to significantly reduce disability and mortality of citizens. A perspective way to achieve efficient personalized treatment is to use methods for operative evaluation of the individual drug load, properties of specific tumors and the effectiveness of selected therapy, and other actual features of pathology. Among the vast diversity of tumor types—brain tumors are the most invasive and malignant in humans with poor survival after diagnosis. Among brain tumors glioblastoma shows exceptionally high mortality. More studies are urgently needed to understand the risk factors and improve therapy approaches. One of the actively developing approaches is the tumor-on-a-chip (ToC) concept. This review examines the achievements of recent years in the field of ToC system developments. The basics of microfluidic chips technologies are considered in the context of their applications in solving oncological problems. Then the basic principles of tumors cultivation are considered to evaluate the main challengers in implementation of microfluidic devices, for growing cell cultures and possibilities of their treatment and observation. The main achievements in the culture types diversity approaches and their advantages are being analyzed. The modeling of angiogenesis and blood-brain barrier (BBB) on a chip, being a principally important elements of the life system, were considered in detail. The most interesting examples and achievements in the field of tumor-on-a-chip developments have been presented.
Cancer continues to occupy a leading position in disease-associated mortality, which is inferior only to cardiovascular diseases (1). The World Cancer Research Fund (WCRF) urges to prevent millions of deaths each year by raising awareness and education about cancer, and pressing governments and individuals across the world to take an active role in the fight against the disease (1). There exists a large variety of cancer types, among which brain cancers have proven to be the most fatal and responsible for substantial morbidity and mortality (2). They are invasive and malignant in humans with poor survival after diagnosis (3). Brain tumors include more than 120 different types, ranging from the least malignant, characterized by not invasive growth, such as meningioma (4), or common in children—medulloblastoma (5), and on the other hand, the most malignant, giving only months of survival for patients and showing extremely high mortality—glioblastoma (6).
Thus, at present time cancer, and brain tumors in particular, still significantly affect the life quality of patients, and it is necessary to admit that current treatment tactics achieve only limited effect (7). The new experimental, ex vivo, models are being extensively developed to deepen understanding of tumor growth, as well as to develop new versions of treatment since in vivo models demand high costs and labor resources while at the same time are considered ethically unacceptable by society (7). They also often fail to mimic crucial features of tumors. It is getting obvious that more studies are urgently needed to understand the risk factors and improve therapy approaches. It is also stressed by WHO, that many of these deaths can be avoided, since many types of tumors can potentially be detected early, treated, and cured (8). But at present time, screening methods for malignant brain tumors, unlike breast cancer or lung cancer, have not yet been developed, so there are still few opportunities for their early diagnosis.
Brain tumors are complex and heterogeneous structures consisting of various types of cells, such as tumoral, neuronal, glial, endothelial, microglial, astrocytic, oligodendroglia, etc. (9–11), which interact with each other and with the environment, forming the tumor microenvironment. The tumor microenvironment includes various factors, such as blood and lymphatic vessels, extracellular matrix, gradients of oxygen, glucose, pH, etc. (12–14). These factors influence tumor growth, metabolism, migration, invasion, angiogenesis, immune response, and resistance to therapy. To adequately model brain tumors in vitro, it is necessary to consider all these aspects and create organ-on-a-chip that maximally mimic the anatomy, physiology and pathophysiology of the brain and its tumor formations.
One of the promising areas of development (after the absolute priority area of screening and early diagnosis approaches) is the creation of means for studying dynamics, interactions in heterogeneous cellular media, therapy and many other aspects of growth and treatment of malignant tumors. This may be achieved by modeling of tumor tissues, particularly using new generation hybrid-integrated devices, such as organs-on-a-chip (OoC), which can be specifically designed for growing tumor cultures and observing their interactions with environment, including cells, anticancer medicines, liquids, etc. (15).
In general, OoC is a relatively new direction in modeling biological systems to study aspects of human pathophysiology and disease (16). OoC are miniaturized hybrid devices mimicking the structure and function of organs or organ parts in vitro that contain living cells and tissues. OoCs make it possible to reproduce structure and function of target fragments of human body, the processes that are developing in them, as well as to study the influence of various factors, such as drugs, toxins, stress conditions, infections, etc., on these processes. It appeared that multidisciplinary approach based on OoC shows quite a few advantages over traditional biological models of tumor research, such as single cells, spheroids, animal models, etc. For example:
- High level of authenticity, due to cultivation of living cells and tissues, which could be personalized, possibility to maintain as well as ability to maintain specified cultivation conditions, including temperature, pressure, pH, oxygen, nutrient media, mechanical forces, etc.
- High level of integration, achieved through the combination of different types of cells and tissues, as well as the inclusion of sensory and actuator elements, allowing to monitor and control the processes occurring in the OoC, as well as regulate various parameters of the microenvironment, such as fluid flow, pressure, temperature, concentration of oxygen, and nutrients.
- A high level of standard microtechnologies participation, allowing the use of a wide range of materials and the creation of functional units adaptively depending on the object being studied and the characteristics required for analysis.
- High level of personalization, achieved by the use of individual cells and tissues of patients, as well as adaptation of OoC parameters and conditions to specific needs and research goals.
- The opportunity of parallel testing of multiple samples and conditions on a single platform.
Current review analyzes the recent progress achieved in the field of, so-called, brain tumor-on-a-chip (ToC) developments. The basics of microfluidic chips technologies are considered in the context of their applications in solving oncological problems. Then the basic principles of tumors cultivation are considered to evaluate the main challengers in implementation of microfluidic devices, for growing cell cultures and possibilities of their treatment and observation. The main achievements in the culture diversity approaches and their advantages are being highlighted. The modeling of angiogenesis process and blood-brain barrier-on-a-chip, as principally important elements of the life system, have been considered in detail. The most interesting examples and achievements in the field of ToC developments have been considered.
The hybrid-integrated devices for mimicking malignant brain tumors, tumors-on-a-chip (ToC), considered in this review are used for modeling and studying brain tumors. In this section the design, materials and technologies of ToCs, as well as the biosensor elements integrated into them are considered.
ToCs for modeling brain tumors can be classified according to various criteria, such as the type of cell structure being mimicked, the spatial organization of the culture, the number of microfluidic channels, the presence of vascularization, and the type of extracellular matrix. Figure 1 shows the classification of ToCs for mimicking brain tumors according to various criteria.
Figure 1. Classification of organ-on-a-chip models for mimicking brain tumors according to various criteria.
Depending on the type of structure being mimicked, the following main designs can be distinguished:
Tumor-on-a-chip (ToC) is modeling the microenvironment and structure of a brain tumor, including tumor cells, extracellular matrix, vessels, immune cells, and other components (25, 26). In relation to neuro-oncology, some authors may also use the term “brain-on-a-chip” (17, 18), but we believe that in this subject area it is more informative to use a term focused specifically on tumors. Such systems usually contain one or more channels filled with neuronal cells or tissues that can undergo electrical or chemical stimulation (19, 20). ToCs are convenient for studying growth mechanisms of tumors, including vascularization (21), invasion, metastasis, angiogenesis, immune response, and drug sensitivity (22–24, 27, 28). Tumor cells in Toc also could be exposed to gradients of oxygen, glucose, pH, mechanical forces, anticancer drugs, etc. (29–31).
Barrier-on-a-chip (BoC) models barriers formed between different tissues or organs, such as the blood-brain barrier (BBB) (32), blood-tumor barrier (blood -tumor barrier, BTB) (33), blood-cerebrospinal fluid barrier (BCSFB) (34), etc. BoCs make it possible to study the structure, function, permeability, transport, regulation, and disruption of barriers under normal and pathological conditions (35, 36). BoCs can also be used to screen and test drug candidates that must overcome barriers to reach the target tissues or organs (37). BoCs typically consist of two or more channels separated by a semipermeable membrane on which the different cell types that form the barrier are grown, such as endothelial, epithelial, astrocytes, pericytes, etc. (38–40). The use of epithelial cells in brain barrier models may seem incomplete or not entirely relevant from a physiological point of view, but there are several reasons for their use. Such cells are relatively easy to culture [e.g., MDCK (canine renal epithelial cells) or Caco-2 (human intestinal epithelial cells)] and they exhibit good barrier properties, making them suitable for basic research (41). The well-established culture protocols make them an attractive experimental target, especially in the initial stages of research. Epithelial cells, although not identical to BBB endothelial cells, can be used to assess basic barrier functions such as transport of substances across the cell layer, active and passive transport. In addition, primary BBB endothelial cells or specific brain cells may be difficult to obtain or expensive to obtain and culture. Epithelial cells, being more readily available, may be used as surrogate models in resource-limited conditions.
Depending on the type of culture, ToCs can be divided into the following categories:
• ToC with 2D cultures. These are the simplest and cheapest models in which cells are grown on a flat surface such as glass, plastic or polymer (42, 43). These planar models are easy to manipulate and analyze, but they do not reflect the realistic and complex structure and function of the tumor microenvironment.
• ToC with 3D cultures. These are more complex and expensive models in which cells are grown in a three-dimensional environment such as a hydrogel, matrix, or scaffold (44, 45). These models better mimic the complex structure and function of the tumor microenvironment, such as three-dimensional organization and function of cells, extracellular matrix, vascularization, barriers, gradients, forces, and other factors (46, 47). Based on three-dimensional cultures, it is possible to work with cell spheroids and organoids, which can consist of various types of cells (48). Such models are more realistic and informative. However, they are also more difficult to manipulate and analyze, and require high control over the microenvironment and integration with biosensors.
Based on the configuration of microfluidic channels, the following designs can be distinguished:
• Single-channel ToCs have a single microchannel in which tumor cells are directly examined (49) or can contain a culture chamber to maintain the necessary conditions (50). Single-channel ToCs allow mimicking a homogeneous environment and provide a constant flow of fluid and nutrients. This is important when studying the movement of anticancer drugs, especially those incorporated with magnetic nanocarriers. In addition, when trying to mathematically describe such complex systems, constant flow conditions allow us to form a more convenient approximation for calculation.
• Multichannel ToCs are those that consist of several channels connected to each other or to a common reservoir (51, 52). Such systems enable culturing of different types of cells and tissues to simulate more complex and realistic tumor microenvironments. Multichannel ToCs allow the simulation of heterogeneous environment and different regimes of fluid and nutrient flow to be provided.
Vascularization plays an important role in the development, growth, invasion, angiogenesis, and metastasis of brain tumors, as well as in the transport and drug toxicity testing. Depending on the presence of vascularization, the following main types of ToCs can be distinguished:
• Non-vascularized ToCs are those that do not have vasculature within or around cells or tissues. Non-vascularized ToCs allow to model avascular brain tumors or individual parts of them, as well as to study the effects of hypoxia, acidity, and metabolic stress on tumor cells (53–55).
• Vascularized ToCs (VToCs) are those that have vasculature within or around cells or tissues. Vascularized ToCs allow to model vascular brain tumors and study the influence of angiogenesis, perfusion, trans endothelial migration and drug delivery on tumor cells (56, 57). It has been shown that VToCs provide higher level of mimicry of the physiological conditions, particularly of blood and lymph circulation, which ensures the more authentic simulation and study of mechanisms of angiogenesis, metastasis, and drug delivery through the BBB (58).
Extracellular matrices (ECM), in the tumor microenvironment and, its influence on the behavior and live prospects of cells (59). The extracellular matrix consists of various proteins, polysaccharides, glycoproteins and glycosaminoglycans, which can form various structures, such as collagen fibers, fibronectin networks, basement membranes, etc. (60). The ECM can influence the morphology, proliferation, differentiation, migration, adhesion, invasion, and apoptosis of cells, as well as their signaling pathways and gene expression (60).
Depending on the type of ECM, the following main types can be distinguished:
• Synthetic ECM consists of synthetic materials such as polymers, gels, or membranes (61). Synthetic materials allow the control of the chemical composition, mechanical properties, and topography of the ECM, as well as ensuring its good biocompatibility and stability. However, synthetic ECM cannot reproduce the biological activity and heterogeneity of life tissues, nor does it reflect the physiological conditions of human ECM. Polyethylene glycol (PEG), polylactide-co-glycolide (PLGA), polydimethylsiloxane (PDMS), or alginate can be used as materials for creating synthetic ECMs. Such systems need to be modified with proteins, peptides, or other bioactive molecules to improve their ability to support cell adhesion and other functions important for cell cultures.
• Biomimetic ECM is composed of biological materials such as collagen, fibronectin, laminin, hyaluronic acid, or decellularized tissue (62, 63). Biomimetic ECM can reproduce the biological activity and heterogeneity of the natural tissues, and reflects the physiological conditions of the human ECM. However, biological ECM requires more complex preparation and sterilization techniques and has limited ECM controllability and variability.
Since ECMs are typically derived from natural sources (e.g., animal or human tissue), their sterilization is an important preparation step before use in biomedical applications. However, sterilization of ECMs is challenging because methods that effectively kill microorganisms (temperature, UV, radiation) can also damage or alter the structure and biological activity of the matrix. Therefore, an important challenge is to integrate this process into the overall workflow of ECM creation and to create effective sterilization protocols, taking into account the use of microfluidic systems.
Specific examples of various organ-on-a-chip designs and topologies for modeling brain tumors will be presented in the following sections.
The materials used to fabricate ToCs are selected according to their structure, characteristics and properties, such as:
• Biocompatibility—the ability of a material not to cause unwanted biological reactions such as inflammation, infection, and immune response or toxicity.
• Optical transparency is the ability of a material to transmit light, allowing cells or tissues within the OoC to be visualized and measured using optical techniques such as microscopy, spectroscopy, or fluorescence.
• Gas permeability—the ability of a material to allow gases, such as oxygen and carbon dioxide, to pass through, which allows to maintain the required level of gas exchange and metabolism of cells or tissues inside the OoC.
• Mechanical strength—the ability of a material to withstand mechanical loads, such as pressure, shear or tension, that may occur during the manufacture, storage or operation of the OoC.
• Chemical resistance—the ability of a material not to enter into chemical reactions with cells, tissues, fluids, or drugs that are inside or in contact with the ToC, thereby avoiding unwanted chemical changes or damage to the ToC.
• Thermal stability—the ability of a material not to change its properties or shape when the temperature changes, which may occur during the manufacture, storage, or operation of ToCs, which allows avoiding of unwanted thermal deformations or destruction.
Depending on the chemical composition, the following are the most common types of materials used for the manufacture of ToCs for mimicking brain tumors:
• Elastomers are polymeric materials with highly elastic properties—exhibit reversible extension with low hysteresis and minimal permanent set. The most widely used elastomer in forming microfluidics structures is polydimethylsiloxane (PDMS). It has the advantages of gas permeability, mechanical compatibility, chemical compatibility, low cost, and ease of processing (64). The main disadvantages of PDMS are hydrophobicity and low mechanical strength (65). In addition, the properties of PDMS may be degraded by thermal sterilization.
• Thermoplastics are materials that can be melted and molded at high temperatures and harden when the temperature is lowered. Thermoplastics such as polycarbonate (PC), polymethyl methacrylate (PMMA), polystyrene (PS), polyethylene (PE), polypropylene (PP), etc. are widely used for OoC fabrication due to their advantages such as high optical transparency, mechanical strength, chemical compatibility, low cost, and ease of processing (66, 67). However, thermoplastics have disadvantages, such as high thermal deformation, rather weak gas permeability, low electrical compatibility, possible autofluorescence and technological difficulties in integration with sensors and actuators (68, 69). In addition, joining and bonding thermoplastics to other materials or their own parts can be difficult. When creating thermoplastic chips, it may be technologically necessary to ensure sufficient hermeticity, especially under high pressure conditions or when long-term flow experiments are required. This can lead to leaks, poor compatibility with sealing materials, and problems with the durability of the device. Thermoplastics can also be coated with various materials such as silicone, polyethylene glycol (PEG), hydrogels, etc. to improve their biocompatibility, permeability, and antibacterial properties (70).
• Glass is an inorganic material that is formed when a molten mixture of silica and metal oxides is rapidly cooled. Glass is also widely used for the fabrication of OoC and ToC, as it has several advantages, such as high strength, sufficient biocompatibility, excellent optical transparency, good chemical stability, ease of microfabrication, and the ability to integrate sensors and actuators (71–73). Some of the most common types of glass that are used to make ToC are borosilicate glass, quartz glass, sapphire glass, pyrex, etc. However, glass has disadvantages such as poor gas permeability, low mechanical compatibility, high fragility, and possible difficulties in processing and heterogeneous integration with other materials.
• Hydrogels are materials that consist of networked polymer structures that can absorb and retain large amounts of water. Some of the most common types of hydrogels that are used to fabricate ToC are agarose, alginate, collagen, hyaluronic acid, gelatin, polyacrylamide, etc. (74–77). Hydrogels are quite often used for the fabrication of ECM in ToC due to their advantages such as high biocompatibility, mechanical compatibility, chemical compatibility, biological activity and variability. However, hydrogels have disadvantages such as low transparency, mechanical strength, thermal stability, and electrical compatibility.
Figure 2 provides a comparison of the key properties of main materials used in the formation of ToC.
Some other materials as well as the most widely used once described earlier, can be useful in fabricating ToCs. These are: photopolymers (78, 79), silicon (80, 81) (including its use for creating master forms for soft lithography), metals for integrating sensor-actuator elements (82, 83), and membranes made of various materials (84, 85).
The technologies for brain tumor modeling must provide the ability to create microstructures with high precision, complexity and functionality, as well as the ability to integrate various materials, cells, tissues and sensor-actuator elements. Depending on the physical principle, the following are the most common types of technologies for mimicking brain tumors on ToC.
Photolithography is a technology that uses light waves to create micro- and nanostructures on the material coated with a photosensitive layer (photoresist) (86). Photolithography allows you to create ToC structures with high precision, speed, and flexibility, and is also compatible with various materials, such as thermoplastics, glass, silicon, etc. (87, 88). However, photolithography requires expensive and complex equipment, highly qualified personnel, and is also sensitive to contaminants and temperature.
Soft lithography is a technology that uses elastic templates, called masters, to create surface micro- and nanostructures in the layer called a replicant (89, 90). Soft lithography allows you to create ToCs with high accuracy, speed and flexibility, and is also compatible with various materials, such as PDMS, hydrogels, etc. The disadvantages of soft lithography may include the difficulty of controlling the structure and properties, as well as deformation and degradation of the template and material.
Laser ablation is a technology that uses laser beams to remove or modify material with high energy and precision (91). Laser technologies are characterized by high precision, flexibility, repeatability and a wide choice of materials. Laser ablation allows high precision manufacturing process due to the possibility of software control after automated design of the required structure (92). This group of technologies may have disadvantages such as increased channel surface roughness compared to other microfabrication methods (93), which can be solved through additional chemical surface treatment, and may also cause thermal damage or chemical changes to the material.
3D printing is a technology that uses digital models to create three-dimensional objects by depositing sequential layers of material (94). This group of technologies can use extrusion, injection and photopolymerization methods for the formation of microstructures (95). 3D printing allows you to create ToC of a more complex structure; it allows you to form using various materials not only microfluidic systems, reaction chambers, but also various auxiliary elements for the sustainable functioning of a hybrid device (96). However, 3D printing has rather low accuracy, speed, and mechanical strength. Fabrication of complex systems using this technology requires expensive and complex equipment.
Bioprinting is a technology that uses living cells and biomaterials to create three-dimensional tissues and organs by sequentially depositing layers of material (97, 98). Bioprinting makes it possible to ensure high biocompatibility and biological activity of manufactured structures, and is also compatible with various materials, such as hydrogels, collagen, alginate, etc. (99). Using this technology, it is possible to create complex geometries from living elements, simulate micro vessels and cultivate tumor cells in a vascularized system (100, 101). However, bioprinting has low speed and compatibility with integrated sensor-actuator elements, requires expensive and complex equipment, as well as strict control of cell sterility and viability.
To connect layers in microfluidic systems for preparation of ToCs, thermal bonding, connection with glue, photopolymerization, etc. are used (102).
Figure 3 provides a comparison of common technologies for ToC fabrication.
Thus, the diversity of materials and technologies allows researchers to create ToC systems of varying complexity to address a wide range of tasks. ToCs have become an important tool in studying the tumor microenvironment, invasion, angiogenesis, and drug response. Today, the key goal of creating such systems is to achieve the highest degree of biological similarity to ensure high experimental accuracy. Modern microtechnologies, such as photolithography, 3D printing, and bioprinting, enable the creation of complex microstructures and the integration of biological elements, although they sometimes require expensive equipment and high-tech processes. ToC systems are a promising tool for studying brain tumors. They allow for more accurate reproduction of physiological conditions, thereby increasing the efficiency of research into new therapeutic approaches and drugs.
Traditional two-dimensional (2D) brain tumor models cannot fully recapitulate the functionality and life stages of a brain tumor, nor do they reflect the physiological conditions of the human brain. Therefore, the development of more realistic and predictive models of brain tumors is an important task to improve the understanding of the functioning of brain tumors and the development of new therapeutic strategies.
Growing cultures of brain tumors to improve understanding of tumor development and explore new therapies, is a labor intensive and multistage process, when exploring traditional classical approaches (103–105). In human body tumors develop under complex and dynamic conditions which influence their growth, invasion, and metastasis. In the life systems components are under communication and influence on each other. Such communications include cell-cell contacts as well as the mediators enabling these contacts. The mediators include molecules as extracellular vehicles (EVs), providing horizontal transfer of genetic information (Figure 4) (106).
Figure 4. Tumor microenvironment. Tumor cells hijack different cellular and non-cellular non-malignant components of TME to promote their own growth and survival under hostile conditions. Meanwhile, the mediators for such contacts can be soluble factors (chemokines/cytokines/growth factors, etc.), or those that enable horizontal genetic/biomaterial transfer including cfDNA, apoptotic bodies, CTCs, and exosomes. Reprinted from Baghban et al. (106), license CC BY 4.0.
Scaling of operations and integrating them into hybrid microfluidic devices—ToCs, may offer a new technical level of performing these tasks of tumor culturing and investigation. The implementation of this approach requires a detailed analysis of all stages of the process of culturing the malignant tumor cells. A variety of approaches used in this process have been discussed elsewhere (107). The traditional process of creating an organotypic culture is complex and multi-stage (108), and implementation of ToCs for culture growth makes the procedure less labor intensive and more accessible (109–111).
Several cultivation techniques in vitro as noted earlier is applied for tumor modeling to test different therapeutic approaches (112) including 2D cell culture, 3D spheroids of various composition and morphology, 3D grown or printed heterogeneous co-culture (113), etc., which is schematically generalized in Figure 5.
Figure 5. Schematic cross-sectional view of main tumor cultivation chambers integrated in microfluidic systems for cultivation of 2D culture (A), spheroids (B), and 3D heterogeneous co-culture (C). 1—substrate (polymer, glass, silicon, etc.), 2—profile for nutrient flow arrangement, 3—porous support, 4—protein layer, 5—gas delivery channel, 6—casing, 7—tumor cells, 8—growth medium, 9—gas inlet, 10—pharmaceutical compounds inlet, and 11—liquid nutrient inlet.
The implementation of 2D culturing could be completed on a solid substrate, covered with special underlayers, such as collagen-based gels, Matrigel, etc. The 2D cell cultures could be seeded by injection, or by the capillary flow of suspended cells. The process is generally less labor intensive and expensive than 3D culturing. However, planar 2D cell cultures are well-known to have major limitations because the cancer cell behavior is restricted via the lack of biological and mechanical cues, they would naturally experience in vivo. Saydé et al. provide a comparison of 2D and 3D cultures (119). They note that when comparing the morphology and cell differentiation in 2D cultures, the cells lose their natural shape and polarization, while in 3D cultures they retain their real shape and polarization, which more accurately reflects their behavior in the body. In 3D cultures, spontaneous differentiation, and morphogenesis are observed through cell contacts or soluble factors, as well as a better representation of growth factors and genes associated with angiogenesis. In 2D cultures, these processes are modified and less physiological. 3D cultures allow the study of the functional heterogeneity of tumors and offer a more accurate representation of cell proliferation and response to drugs, while in 2D cultures this is limited to observation. However, 2D cultures are better at modeling the immune response, but 3D cultures provide better geometry and structure-function relationships, although complex co-cultures can complicate modeling. 2D cultures are more affordable, while 3D cultures, especially those using complex techniques, are more expensive.
Scaling down the most traditional 2D culturing onto a microfluidic chip needs preparation of the bio substrate at the bottom layer of microfluidic device cultivation chamber, which could be a solid material. However, cell viability may depend on the interactions with neighboring cells, as well as with the ECM (114), insufficient cell breathing due to the lack of vascularity, incorrect values of power and shear stress, which altogether or separately cause changes in cell phenotype during in vitro culturing (115–117), etc.
A variety of 3D culturing methods which can be applied for cultivation of spheroids includes anchorage-independent, or anchorage-dependent platforms (44, 118). Anchorage-dependent platforms provide cells with structural support and require attachment to a substrate for growth, which is important for tissue modeling and differentiation studies. Anchorage-independent platforms allow cells to grow without the need for attachment, making them useful for modeling tumor aggregation, metastasis, and other processes characteristic of cancer cells. Anchorage-dependent platforms could be in due course classified into hydrogel or scaffold-based types, porosity, density, and mechanical strength (119). Such technologies are usually applied for preparation of 3D spheroids, including basic models of tumors and multicellular tumor spheroids. The tumor spheroids are formed as solid spherical 3D cultures formed via proliferation of a single tumor stem/progenitor cell which are capable of self-organization after penetration into sphere-like particles (120, 121). Classification of basic principles for formation of anchorage-independent and anchorage-dependent cultures presented in Wanigasekara et al. (44).
Growing cells under serum-free, non-adherent conditions enables the enrichment of the cancer stem/progenitor cell population to be achieved since only this type of cells can survive and proliferate in this environment (121). It has been shown that 3-D culture methods are able to preserve the natural characteristics of tumors much better than conventional 2-D monolayer cultures, which applies to tumor-derived organoids, tumor-derived spheroids, organotypic multicellular spheroids, and multicellular tumor spheroids (122). Spheroids are unique in that they contain cancer stem cells (CSCs). They have been used as surrogate systems, derived as floating spheres, to evaluate the CSC-related characteristics of solid tumors in vitro, and have been established from numerous cancer types including glioma (122). They are applied as a high-throughput screening platform or for the cultivation of CSC-related tumor cells (122). A three-dimensional (3D) multicellular tumor spheroid to quantify chemotherapeutic and nanoparticle penetration properties in vitro have been described in Ma et al. (123). It is important to mention that multicellular tumor spheroids (MCTS) are able to capture some aspects of the dimensionality, cell-cell contact, and cell-matrix interactions seen in vivo which makes them attractive tool for tissue modeling. Many approaches exist to create MCTS from cell lines, and they have been used to study tumor cell invasion, growth, and how cells respond to drugs in physiologically relevant 3D microenvironments (124). However, there is often a problem of size and shape uniformity in generating tumor organoids. Lee et al. facilitated in vitro uptake of the NPs into the tight 3D tumor spheroids by the semi-spherical shape of the NPs with a proper size and surface charge (125).
An important problem in modeling cancer tissues is that the process is time consuming and have limitations, such as a lack of reproducibility and a wide distribution of spheroid sizes. Therefore, many researchers have used engineering methods, such as microfluidic devices, to culture 3D cells in dynamic conditions. Thus, a simple and low-cost microfluidic device fabricated from polymethyl methacrylate (PMMA) was proposed for dynamic and static cell culture examinations and in vitro monitoring of 3D cells growing. The liposome-based nanocarriers were used as the drug to test cell viability in 3D (126, 127). The approach is expected to improve in vitro testing models, reduce, and eliminate unsuitable compounds, and select more accurate combinations for in vivo testing (126–128).
At the same time, despite organoid techniques attractive features, such as possibility of multichannel testing of drugs and viability of tumor cells, comparative cooperativeness, there are still several disadvantages, and first the specific morphology of spheroids, which is not always perfectly consistent with the native tumor tissue and its environment. That is why the tendency of development tumor-on-a-chip systems for 3D tissue growing under special conditions more closely resembling the life tissues. Such new approaches enable the architecture of cells to be preserved in an extent allowing prediction of toxicity and antitumor drug resistance. Another achievement in 3D tissue engineering is the development of tools for mimicking the tumor microenvironment, enabling the evaluation of metastatic progression and vascularization. However, the costs and reproducibility problems are preventing the vast implementation of such approaches (129, 130). While standardized 3D culturing procedures could both reduce data variability and enhance biological relevance (129–133).
Micro methods could considerably improve the performance and quality of the testing using tumor cultures of various structures. Thus, microwell arrays can provide a facile method to produce uniform-sized spheroids in a high-throughput manner (134). Hydrogel microwell arrays were fabricated using a PDMS stamp as previously described (135, 136).
It has been demonstrated that the method of generating uniform-sized multicellular 3D tumor spheroids using PDMS microwell arrays could be a powerful tool for in vitro drug screening applications. Thus, the co-culture spheroids may have a homogeneous size of < 5% standard deviation, which is comparable to mono-culture spheroids of the same size (137). Such uniform-sized multicellular tumor spheroids are helpful in studying the effect of anticancer drug-loaded nanoparticles on their size or viability. In addition, the homogeneous and uniform-sized 3D tumor spheroids generated in the hydrogel microwells show tight cell-cell junction compared to heterogeneous models.
In creating organoid structures of tumors, it is perspective to use more advanced approaches of organogenesis, such as the one presented for the stem cells growing into organoids. This process requires the biochemical stimulus from exogenous signals, also called morphogens, such as proto-oncogene protein (WNT3), and Noggin (138). Brain organoids are valuable models for the study of human brain diseases and researchers are now in need of improved culturing and imaging tools to capture the in vitro dynamics of development processes in the brain. Khan et al. described the design of a microfluidic chip and bioreactor, to enable the in situ tracking and imaging of brain organoids on-chip under morphogenesis process (139). The low-cost 3D printed microfluidic bioreactor supports organoid growth and provides an optimal imaging chamber for live-organoid imaging, with drug delivery support. The fully isolated design of a live-cell imaging and culturing platform enables long-term live-imaging of the intact live brain organoids as it grows and to analyze thus their self-organization in a controlled environment with high temporal and spatial resolution. Also, spheroids- and organoids-on-a-chip technology could control the spatial distribution of the morphogens, magnifying the response and revealing their functions, using various strategies, such as gradient-concentration model, etc. (139).
Further developments in organoids engineering technologies have enabled to overcome the limitations in reconstituting the perfusable microvascular system of large-scale tumors conserving their key functional features, via the reconstruction of micro vascularized organoid models using technologies of microfluidic systems (Figure 6).
Figure 6. ToC consists of channels that can be loaded with media and cells and can support perfusion culture. Reprinted from Jeong et al. (142), license CC BY 4.0.
Existing 3D tumor models have enabled in vitro simulation of the tumor environment (140–143), and some progress has been made in vascularization using different models (144). Shoval et al. prepared spheroids by mixing cancer cells of different origins with endothelial cells in different ratios, hoping to obtain 3D tumor models with vascular networks. Authors demonstrated the formation of capillary-like structures which were formed upon assembly and growth of mixed spheroids and that spheroids' shape and surface texture may be an indication of spatial invasiveness of cells in the extra-cellular matrix. Biogenic solutions for vascularization of tumor cultures will be considered in the next section.
Although spheroids are considered an ideal model mimicking some important tumor features, such as structural organization and the gradients of oxygen, pH, and nutrients (145), several issues with applying this model at a preclinical level remain, particularly reproducibility and high-throughput application (146). Recently, 3D bioprinting has been recognized as a promising technology for creating a tissue-based platform with high reproducibility and scalability (147–149). Obviously, a key feature in developing a 3D bioprint model useful in pharmaceutical applications in oncology is the choice of an appropriate printable biomaterial, commonly referred to as bioink, essential for determining cancer cell phenotypes and biophysical properties of the tissue. One of the main advantages of proposed 3D in vitro model is that it offers over the conventional 2D culture plates the ability to model and quantify cell invasion in a 3D cell culture setting (150). One advantage of the described design is its ability to create tumoroids in a compartmentalized array of microcavities that are connected to a microchannel (151, 152).
Thus, the 3D tissue cultures represent an experimental model able to potentially mimic in vivo growth more closely. They show distinct characteristics in terms of cellular phenotype, mass transport, and cell–cell interactions as compared with conventional 2D cell cultures. At the same time among 3D cultures organoids and printed tissues have certain limitations, namely some models of well-matrices generate spheroids of a wide distribution of sizes, due to variations inside the same well (153–155). At the same time vascularization, while being a critical value for tumor development and drug delivery, is still missing in 3D models (156, 157). While large-scale investigations and high-throughput tests are expensive and time consuming (158), and variability in biological matrices can lead to unpredicted experimental results (159–162).
More appropriate are the heterogeneous 3D models comprised environmental cells and tumor cells (163). For preparation of this type of cultures, it is suitable to use hybrid microstructures, enabling the growth conditions to be provided. In Figure 12 the universal microfluidic device for cell culturing is presented. When preparing a conventional both 2D or 3D culture it is important to provide an appropriate substrate surface. In case of 2D cultures it could be a macroporous polymeric layer, impregnated with the nutrient, while in the case of 3D culture growth it is important to enlarge the specific surface area of the interface between the surface of the cell structures and the liquid nutrient, gas medium, etc. A universal solution could be the porous substrate (such as nanoporous anodic alumina) layer delivering the nutrient from the lower chamber (Figure 7) (164). At the same time such microsystem could be equipped with autonomous life supporting system.
Figure 7. Setup of microfluidic cartridge and miniaturized incubator microscope platform. (a) SEM image of microcavity with micro hole array membrane (1.6 mm x 1.6 mm). (b) SEM image of micro hole array with hole distance 10 μm. (c) Microfluidic cartridge with connected tubing and cables for impedance measurement. This cartridge is integrated in the miniaturized incubator microscope. (d) Incubator microscope with inserted microfluidic cartridge and lid, for bright field imaging and cell cultivation under controlled temperature. (e) Schematic illustration of the microfluidic cartridge. The electrodes (yellow) are positioned in the two fluidic channels. The electric current flows between the electrodes through the pores in the membrane (dashed line). (f) Schematics of experimental setup with two platform modules for parallel or serial operation. Reprinted from Kohl et al. (164), license CC BY 4.0.
The volume with nutrient has inlet and outlet openings with valves and ferrules, enabling a control over the content of the nutrient. The top chamber contains two or more inlet/outlet openings, enabling the delivery of cells, pharmaceuticals, markers, etc. On the outer circle of the top surface of the chamber, the inlets for air delivery are installed. The camera is covered with a glass window for monitoring of the culture state. The design enables various types of cultures to be grown, including the heterogeneous 3D cultures, containing TME.
Chong et al. described the intact brain samples sliced and seeded in the microfluidic device (Figure 8), thus demonstrating that tumor slices provide a broadly useful platform for studying the tumor microenvironment and evaluating the preclinical efficacy of cancer therapeutics (108).
Figure 8. Samples sliced and seeded in the microfluidic device; (A, B) top view. (C) Side view. The bar is 100 μm. Reprinted from Chong et al. (108), license CC BY 4.0.
Robertson-Tessi et al. developed a hybrid multiscale computer model of tumor growth within a normal, homeostatic tissue, and demonstrated the mechanisms by which phenotypic, temporal, and spatial heterogeneity affect growth of a tumor and the outcomes of treatments (165). This work highlighted the importance of the phenotypic heterogeneity of tumor cells. A key prediction of this model is that early stages of tumor development (either primary or metastatic) maintain a phenotypically spatially structured population, where less aggressive clones spatially suppress more aggressive counterparts and that standard treatment modalities may selectively destroy this structured population and facilitate subsequent progression. Controlling tumor progression by maintaining rather than destroying this suppressive tumor layer appears to be more effective that conventional high-dose density therapy that aims to kill the maximum possible number of tumor cells (165).
A promising approach to creating tumors-on-a-chip are organotypic models of brain tumors, which attempt to reproduce the complex architecture and microenvironment of brain tissue as accurately as possible. Such models are created from fragments of brain tissue (usually from animals or humans) and preserve natural cellular interactions, including connections between neurons, glial cells, and the extracellular matrix. For example, Sivakumar et al. demonstrated rapid tumor cell expansion and invasion into a brain tissue substrate over relatively short periods of time (166). The monitoring was carried out using GFP fluorescence demonstrated the appearance of aggressively invasive tumor cell characteristics of the SMA-560 (spontaneous murine astrocytoma) cell line (166). The technique is a perspective alternative to in vivo models of brain tumor growth applicable in ToC technologies.
A simple and less resource-intensive, high throughput platform for further tumors studies is presented by Rodriguez et al. (167). Authors prepared a digitally manufactured microfluidic device in a biocompatible thermoplastic material by laser-cutting and solvent bonding. The fluidic performance of the device is described and demonstrates efficient on-device drug-response testing with tumor slices (167). The reported microfluidic platform is intended for functional drug testing of live tumor.
Surendran et al. investigated how the tumor spheroids responded to neutrophil extracellular traps (NETs) produced in the microfluidic channel (168). They leveraged the advantage of magnetic hybrid integration technique for on-demand attachment and detachment of the ensembles, as schematically shown in Figure 9A. The neutrophils we cultured separately in the microfluidic channel and stimulated for NETs with 500 nM PMA for 6 h. The conditioned media that seeped out of the porous channel was collected and added back to the collagen layer on top of the spheroids and incubated for 1 h, before attaching the channel with the spheroid-collagen assembly. More than 60% of the neutrophils NETosed within the channel upon PMA stimulation (Figure 9B). The resultant spheroid distortion observed after 24 h was negligible. No significant difference was observed in the distortion of spheroids due to either direct stimulation with PMA or by stimulation of neutrophils in the channel with PMA (Figure 9C). Thus, the data presented clearly suggested that stromal NETs, and not the tumor-contacted NETs or the vascular NETs, play a significant role in mediating the collective invasion of cancer cells from an aggregated state.
Figure 9. Testing of how NETs production outside the collagen region induces tumor invasion within a TIME, by separate stimulated of the neutrophils in the microchannel with 500 nM PMA. (A) Schematic illustration of the different steps shows that the TIME-on-Chip easily enables on-demand attachment and detachment of the components to initially induce the neutrophils in the microchannel to produce NETs, add the conditioned media to the spheroids, and re-attach the device to enable NETs-spheroid co-culture for analysis. (B) More than 60% of the neutrophils in the microchannel NETosed upon PMA stimulation for 6 h. But even after 24 h of co-culture, the spheroids do not appear significantly distorted as seen in the brightfield images (scale bars represent 150 μm). (C) No significant difference was observed in the distortion of the spheroids with the PMA-stimulated NETs present in the channel or with direct stimulation of the spheroids with PMA (data collected for n = 3 spheroids, mean ± SEM, t-test, ns, not significant). Reprinted from Surendran et al. (168), license CC BY 4.0.
Amereh et al. investigated the influence of matrix stiffness on the growth and invasion of human glioblastoma tumoroids using a PEGDA-printed ToC platform (Figure 10) (169). Collagenase was used to create a heterogeneous collagen cellular matrix. The study allowed to observe a clear dependence of tumor behavior on changes in the rigidity of the extracellular matrix environment. The results showed that tumoroids exhibited higher growth rates and invasion lengths in response to higher concentrations of collagenase. These findings highlight the potential of investigating the impact of various matrix characteristics on tumor growth and invasion. Such investigations may unveil novel therapeutic targets and strategies for combatting glioblastoma's aggressive behavior in a more physiologically relevant context.
Figure 10. In vitro tumoroid invasion platform. (A) Single cell suspension seeded through the loading zone of a self-filling microwell array. (B) Tumoroids were formed after 4 days of culture and were transferred into the tumor-on-a-chip platform. (C) The platform was capable of growing tumoroids in four different chambers, each addressed separately, with an inlet and outlet for collagenase treatment. (D) Tumoroids embedded in bovine fibril collagen hydrogel were loaded into the open surface tumoroid-on-a-chip platform and their growth and invasion were monitored over time. Reprinted from Amereh et al. (169), license CC BY 4.0.
Dorrigiv et al. presented a hybrid modeling technique that combined a continuum reaction–diffusion model and a discrete model to accurately capture the growth and invasion in an inhomogeneous environment (170). The agreement between the experimental results and the model predictions further confirmed the validity and potential of this approach. Extending the ToC platform to incorporate other components of the tumor microenvironment could offer a more comprehensive representation of the complex tumor–stroma interactions. This advancement would enable the study of how different cellular and extracellular components contribute to glioblastoma progression in response to varying matrix characteristics (168–172).
The integration of the sensors with brain ToC platforms to provide real time spatiotemporal information of the tumor microenvironment provides critical insights of cancer progression and understanding of responses to cancer treatments (173). New generation ToC devices show typical characteristics of brain complexity, including the presence of different cell types, separation in different compartments, tissue-like three-dimensionality, and inclusion of the extracellular matrix components (45, 174). Moreover, the incorporation of a vascular system and mimicking the blood-brain barrier (BBB) makes brain ToC particularly attractive, since they can be exploited to test the brain delivery of different drugs and nanoformulations (174).
For real time monitoring of ToCs the best solution would be the integration of sensors into the device to detect physical parameters: temperature, pressure, density, conductivity, electrical impedance, magnetic field, absorbance, fluorescence, etc.; chemical parameters: pH, presence of particular compounds and drugs; biochemical parameters: target proteins, hormones, peptides, etc.; microphysiological parameters: speed of growth, etc.
At the current stage of ToC development the integration of massive sensors could be of a particular interest and provide a breakthrough in studying tumor cultures and their environment, including the dynamics of the processes and response to physical and chemical interventions. Such sensor massive should include physical sensors for control of culture media parameters (temperature, pressure, pH, etc.), and chemical and biochemical parameters (marker proteins, antibodies, DNA/RNA, methabolites, etc.; Figure 11).
Among physical sensors it is necessary to mention recent achievements in fabrication of miniature planar (MEMS/NEMS) sensors integrable into the ToC systems. While developing microfluidic ToCs it is important to consider the group of sensors, which could help to mimicry the biosystem for culture growth by monitoring and adjusting the conditions of the medium (175). These parameters include pressure, particularly monitoring of narrow pressure ranges, using of a minute differential pressure sensor (MDPS), which have found applications in biomedicine, underscoring their significant engineering and medical value. An MDPS generally refers to a pressure sensor capable of measuring pressure ranges below 10 kPa (176, 177).
There is a growing demand for MDPSs with superior measurement resolution, enhanced frequency response, reduced size, and cost-effectiveness. To satisfy the sensitivity requirements of MDPSs, researchers introduced a variety of sensitive structures for ultrasensitive MEMS sensors (178–181), witnessing progressive enhancements in sensitivity (Figure 12).
Figure 12. A diaphragm structure of a piezoresistive pressure sensor with a combination of a four-petal membrane, four narrow beams and a central boss (PMNBCB) for low-pressure ranges. General outlook (A), and cross-section (B). The finite element method (FEM) was used to estimate the stress distribution and analyze the inherent structure's deflection for different parameters. Reprinted from Tran et al. (178), license CC BY 4.0.
Another important parameter is temperature of medium inside ToC. There is a number of solutions of cost effective, integrated sensors. One of approaches is using surface plasmon polaritons (SPPs), which are transverse electromagnetic (TM) waves that propagate along the metal-dielectric interface and decay exponentially away from the metal-dielectric juncture. SPPs have a swift and sensitive response to changes in the ambient medium, which opens new aspects in RI (refractive index) sensing applications. They can tune light-metal interactions and have a wide range of applications in sensors (182, 183) as well switches (184), and other light-on-chip systems useful for operation of life environment.
Another group of sensors essential for monitoring of tumor cells cultivation are the biosensors. A biosensor is defined as an analytical device that incorporates a biological sensing element connected to a transducer to convert an observed response into a measurable signal, whose magnitude is directly proportional to the concentration of a specific chemical/biochemical or a set of chemicals in the samples. Among biosensors, the most widely used are electrochemical and optical methods of signal detection.
Electrochemical transducers use electrochemical reactions to detect and measure biosensing element (bioreceptor) signals (185). Electrochemical biosensors usually use measurement principles such as amperometry, voltammetry, potentiometry, and impedimetry (186, 187). Electrochemical biosensors have advantages such as high sensitivity, specificity, low cost, compact size, integrability, and real-time monitoring capabilities. However, electrochemical biosensors also have disadvantages, such as the need for a reference electrode and electrolyte, as well as possible technological difficulties in immobilizing a biorecognition element into an organ-on-a-chip system. Using electrochemical biosensors, it is possible to monitor tumor parameters such as molecular biomarkers (proteins, nucleic acids), whole cells, cellular activity, metabolism, etc. (188–190).
In some cases when a very high sensitivity of biosensors is necessary the authors propose a solution described by Ji et al. for implementation of electrochemical-aptamer based (E-AB) sensor incorporating organic FETs for amplification of the signal (ref-OECT-based E-AB sensor) produced by capture of Transforming growth factor beta 1 (TGF-β1) (191). This device can directly amplify the current from the electrochemical aptamer-based sensor via the in-plane current modulation in the counter electrode/transistor channel. The integrated sensor can sense TGF-β1 with 3–4 orders of magnitude enhancement in sensitivity compared to that in an electrochemical aptamer-based sensor (292 μA/dec vs. 85 nA/dec; Figure 13) (191).
Figure 13. Design concept of ref-OECT-based E-AB sensor. (A) Schematic image of the ref-OECT-based E-AB sensor. (B) Sensing mechanism of the ref-OECT-based E-AB sensor for TGF-β1. Without the existence of TGF-β1, the methylene blue (MB) redox reporter is closer to the gate electrode surface, which results in a high gate current (IG) as well as a larger channel current modulation (IDS). In the presence of TGF-β1, a conformational change occurs in the aptamer, and the MB redox reporter moves further from the gate electrode surface, which results in low gate current and smaller channel current modulation. Reprinted from Ji et al. (191), license CC BY 4.0.
A platform named “Digital Tissue-bArrier-CytoKine-counting-on-a-chip (DigiTACK),” proposed by Su et al. integrates digital immunosensors into a tissue chip system and demonstrates on-chip multiplexed, ultrasensitive, longitudinal cytokine secretion profiling of cultured brain endothelial barrier tissues (192). The integrated digital sensors utilize a novel beadless microwell format to perform an ultrafast “digital fingerprinting” of the analytes while achieving a low limit of detection (LoD) around 100–500 fg/mL for mouse MCP1 (CCL2), IL-6, and KC (CXCL1).
Optical biosensors use light radiation to detect and measure bioreceptor signals. Optical biosensors can be based on various principles, such as fluorescence, luminescence, absorption, scattering, interference, and others (193, 194). The key advantage of such biosensors is that there is no need for physical contact between the sensor element and the detector (195). In addition, they have high sensitivity, specificity, resolution, and dynamic range, and allow for multi-channel and multiplex analysis. Disadvantages of optical biosensors include the need for special light sources and detectors, the possible use of tags to increase sensitivity, and sensitivity to interference and contamination. Schematic representation of an integrated optical Mach-Zender interferometer (MZI) for the detection of target spike protein S1 subunit crossing the BBB after coronavirus infection (196) (Figure 14). As a light source a visible red laser diode (658 nm, 100 mW, RLT650-100MGS, Roithner Lasertechnik GmbH, Vienna, Austria) was used. The continuous flow of the samples was provided. The schematic representation of the experimental setup and the integrated optical MZI sensor can be seen in Figure 14.
Figure 14. Schematic representation of the biosensor device: the integrated optical Mach–Zehnder interferometer (MZI) for sensing the analyte (1), the microfluidic apparatus (syringe pump, tubes, PDMS microchannel) for fluid sample providing (2), the signal processing unit, namely a photomultiplier tubes (PMT) detector (3) with an oscilloscope (4), the microheater structure for bias point tuning (5). The working principle of the device is also presented: the evanescent field detection is based on the phase difference in the propagating light of the measuring arm (yellow waves) compared to the ones of the reference arm (red waves) (6). Phase difference can be induced by the binding of the target spike protein S1 subunit to the antibody-covered surface of the measuring arm. Reprinted from Petrovszki et al. (196), license CC BY 4.0.
Optical transducers together with various biorecognition elements permit the real-time monitoring of different biological phenomena and markers, such as the release of growth factors, the expression of specific receptors/biomarkers, the activation of immune cells, cell viability, cell-cell interactions, and BBB crossing of drugs and nanoparticles.
One of the important tools for studying cell barriers are transendothelial electrical resistance (TEER) sensors (197), which are designed to assess their integrity and functionality. Such sensors measure the electrical resistance created by cell layers between two electrodes. This method allows us to estimate the density of intercellular contacts and barrier permeability, which is useful for testing drugs and studying the effect of various factors on cell permeability over time. To measure TEER, multimeters or impedance measurement systems are used, which generate alternating current and measure the voltage created on the cell layer. Modern devices can be integrated with automatic systems for data analysis and real-time monitoring (198, 199).
Other types of sensors and biosensors in addition to the above-mentioned once can be integrated into ToCs for modeling and studying brain tumors. They include magnetic, mechanical, etc. (200–202). These sensors can work either alone or in combination with more common detection systems (203).
Vascularized tumor on a chip (VToC) entails emulating intricate microvascular networks like those observed in tumors through microfluidic devices, which are meticulously designed to offer a faithful representation of cancer in vitro, exploration of tumor biology, evaluation of drug efficacy, and anticipation of patient responses to therapies (204). In comparison with traditional systems VToC demonstrate advantages by creating the environment in which physiological conditions for study of tumor-host interactions are of decisive importance for tumor development and for therapy sustainability.
As mentioned earlier, despite aggressive surgery, radiotherapy and chemotherapy, malignant gliomas remain uniformly fatal (56, 205). To progress, these tumors stimulate the formation of new blood vessels through processes driven primarily by vascular endothelial growth factor (VEGF) (206). But the vessels forms are appeared structurally and functionally abnormal, and are contributing to building of a hostile environment, including low oxygen pressure along with interstitial fluid pressure, which altogether lead to a more malignant phenotype with elevated morbidity and mortality (207). Emerging preclinical and clinical data indicate that anti-VEGF therapies are potentially effective in glioblastoma and can transiently normalize tumor vessels. This creates a window of opportunity for optimally combining chemotherapeutics and radiation.
The inhomogeneous density of tumor vessels causes anomalous blood flow. Local leaks may cause inhomogeneous circulation and consequently oxygen and drug delivery. This effect along with the other morphological anomalies of the system, lead to independence of the erythrocyte velocity on the vessels diameter, and is one-to-three orders of magnitude lower than it is observed in the normal pial vessels in gliomas and mammary carcinomas growing in the mouse brain (208). The velocity of erythrocytes movement cannot be measured in patients, while the blood flow velocity measured using functional MRI in patients with malignant gliomas, is abnormally being increased.
While MRI is typically being used for MPI for patients with brain tumor monitoring, a new method of vascular MRI is used for monitoring of structure and function of vessels in these patients.
Angiogenesis contributes to the progression of various malignant tumors. The primary cause of cancer death results from metastasis, which causes up to 90% of cancer-related mortality (209). Metastasis—a multistep process—is the migration and spread of cancer cells from the initial tumor to distant organs via vessels as well as their uncontrolled growth (210, 211). In the metastasis process, both collaborative and antagonistic interactions between host microenvironmental factors and tumor cells take place (212).
Limited blood supply and rapid tumor metabolism within solid tumors leads to nutrient starvation, waste product accumulation and the generation of pH gradients across the tumor mass. These environmental conditions modify multiple cellular functions, including metabolism, proliferation, and drug response (213). Thus, tumor cells located nearby blood vessels have enough nutrients to keep growing, forming a proliferative outer perimeter. Conversely, those cells located in the innermost region die of nutrient starvation, generating a necrotic core in the center of the tumor (214). Analysis of multiphoton images from tumor and normal tissue showed an average blood vessel diameter of 9.50 ± 0.04 μm for tumor tissue and 6.95 ± 0.36 μm for normal contralateral cortex tissue (214).
The described models recapitulate and systematically simplify the in vitro tumor microenvironment. This enables the study of a metastatic process in unprecedented detail. In modeling angiogenesis various approaches maybe perspective, such as: vascularization, microfabrication, bioprinting, etc. The attempts are made to culture the vascular system in the tumor model via using natural cell diversity. Thus, pericytes are cells present at intervals along the walls of capillaries (and post-capillary venules). In the CNS, they are responsible for blood vessel formation, maintenance of the blood–brain barrier, regulation of immune cell entry to the central nervous system (CNS) and control of brain blood flow (215). A method of development angiogenesis process, a so-called neovascularization (NV) on a microfluidic platform using pericytes is described in Kim et al. (216). NV is a multistep process including initial vascular angiogenic sprouting, followed by migration and association with pericytes and smooth muscle cells (217). Kim et al. engineered physiologically relevant in vitro vascular networks recapitulating the physical interaction between endothelial cells (ECs) and pericyte, as well as the development process of neovascularization (216). They demonstrated that the microfluidic model of angiogenesis can be used as a reliable experimental platform to form a perfusable vessel network derived from the co-culture of multiple cell types (Figure 15).
Figure 15. Schematic of the microfluidic system used to mimic the stepwise endothelial-pericyte interaction. (A) The microfluidic device is composed of a central vessel channel, two adjoining media channels, and the outermost fibroblast channel. The vascular network covered by the pericytes was formed in the central channel with assistance from the lateral fibroblasts. (B) The experimental scheme of the stepwise angiogenic process. ECs and pericytes were mixed and attached to the left side of the vessel channel. ECs sprout through the fibrin gel to establish a blood vessel network, and pericytes follow behind the vessel. (C, D) Confocal images show EC patterning prior to pericyte association during the first 3 days (C), and matured pericytes covered the perfusable EC network on day 6 (D). Scale bars, 100 μm. Reprinted from Kim et al. (216), license CC BY 4.0.
Thus, the microfluidic platform enabled to mimic the in vivo neovessel formation followed by pericyte coverage. The microfluidic device was composed of a central vessel channel for engineering pericyte-covered microvessels in vitro, two adjoining media channels, and the outermost fibroblast channel (Figure 16A). A mixture of ECs and pericytes was attached to one side of the central a cellular fibrin matrix. In response to the directional gradient of biochemical factors secreted by fibroblasts, leading cells of EC sprouts grew toward the opposite end of the central channel and spontaneously formed vacuoles that merged into the intracellular lumen (Figures 16B, C). After 5 or 6 days of culture, when the leading portion of the angiogenic sprouts reached the end of the fibrin matrix, ECs robustly developed interconnecting and perfusable vascular networks with tightly adhered pericytes on the basolateral surface of the blood vessels (Figures 16B, D) (217). Matrix based on gelatin-methacrylate/fibrin was combined with endothelial cells to imitate the microenvironment of the tumor. It has been demonstrated that adipocyte- and induced pluripotent stem cells-derived mesenchymal stem cells can manage angiogenesis.
Figure 16. Endothelial cell coating of conduits to mimic blood-tissue barrier function. For optimized coating of inner channel linings, a programmable 3D orbital shaker was developed that halts rotation at distinct positions for defined times to achieve optimized endothelial cell adhesion (A). Compared to manual flipping of the fluidic chips [(B), top panels], the inner surfaces of shaker-incubated tissues are homogenously coated with green fluorescent endothelial cells (HUVEC/hTERT-EYFP) as imaged by confocal microscopy [(B), bottom panels, scale bar 50 μm]. Tissue chips with fibroblasts (HFF/hTERT-ECFP) in the hydrogel matrix and hollow channels with or without endothelial cells (HUVEC/hTERT-EYFP) were perfused with red fluorescent 70 kDa dextran rhodamine B conjugate (0.25 mg ml−1) to assess diffusion into the hydrogel matrix within 30 min (C). Left panels: fibroblast containing hydrogels with uncoated channels. Right panels: endothelial cell coating using orbital shaker procedure. Size marker 200 μm. Red fluorescence in the matrix after 30 min corresponds to the diffusion distance of dextran beads and thus to the endothelial barrier function. Graph shows comparison in red fluorescence between non-coated channels and channels covered with endothelial cells (D). Shown is the mean of three independent experiments. Reprinted from Nothdurfter et al. (217), license CC BY 4.0. Statistical significance was assessed with student's t-test (**p < 0.01).
Bioprinted channels are coated with ECs post printing to form a dense vessel-tissue barrier. Patient-derived neuroblastoma spheroids are added to the matrix during the printing process and grown for more than 2 weeks. Presented the first bioprinted, micro-vascularized neuroblastoma—tumor-environment model directly printed into fluidic chips and a novel medium-throughput biofabrication platform suitable for studying tumor angiogenesis (Figure 16).
The development of new methods and technologies for modeling brain tumors is essential for improving the accuracy of their vital functions research and developing new therapeutic approaches. Traditional 2D tumor models are unable to fully reproduce the complex architecture and life cycles of tumors, making it difficult to predict their behavior and response to treatment. The use of microfluidic chips in combination with 3D cultivation methods allows for a more accurate recreation of the natural conditions of the tumor environment. 3D cultures, such as spheroids and organoids (218), more accurately reflect the interaction of cells and their behavior, while preserving their natural characteristics. This improves the reproducibility and relevance of experiments and allows for the study of tumor cell growth, metastasis, and invasion processes. In addition, the use of ToC systems opens up new opportunities for studying the tumor microenvironment and the dynamics of their development under conditions close to physiological ones. Despite the progress, difficulties remain, such as the high cost and complexity of reproducing tumor structures. In the future, further developments in the field of micro- and nanotechnology, bioprinting, and organoid technologies can significantly expand the possibilities of testing antitumor drugs and studying their impact on various stages of tumor growth.
Techniques of BBB modeling in vitro date back to 1953, when the original monolayer cell culture transwell system (developed by Corning Inc., Corning, NY) was utilized (219, 220). The late 2000's and early 2010's saw the emergence of dynamic and 3D models such as organoids or barriers on chips using microfluidics (221). Now there is many in vitro methods available that can be used in drug development, disease modeling, neurotoxicity screening, and personalized medicine applications to estimate the permeability of a substance across the BBB (222, 223). Drugs targeting the central nervous system (CNS) are 45% less likely to enter Phase III trials than non-CNS drugs, according to data from 1990 to 2012 (224).
The oldest and best-established method to study the transport of molecules across the BBB is to perform in vivo experiments, having their advantage since it allows for studies of the brain in its natural environment, at the same time such experiments are unethical (220). Since human studies are restricted to post-mortem investigations or imaging techniques such as magnetic resonance imaging (MRI) and positron emission tomography (PET) with limited resolution, most research on the BBB still involves laboratory animals (225). In vitro models are comparatively less complex, and flexible, as per the study design, could generate substantial evidence and help identify suitable in vivo animal model selection (226). All the modern types of in vitro BBB models have their specific advantages, limitations, and recommended areas of application (225). A useful BBB model should meet the following criteria: reproducibility of solute permeability, display of a restrictive paracellular pathway and physiologically realistic architecture, functional expression of transporters, and ease of culture (227). Some important challenges in the BBB models development include: (1) improved fidelity of the spatial arrangement of BBB cell types in a zonation-specific manner, (2) incorporation of blood components known to be important in communication between the vascular system and the brain, (3) developing strategies for multiscale/hierarchical models along cerebrovascular zones, and (4) recapitulating aspects of neurovascular coupling (228). The current BBB models use transwell culture inserts, microfluidic devices, and models based on cell aggregates consisting of a human bone marrow microvascular endothelial cells, astrocytes and pericytes (229).
BBB-on-chip models (microfluidic in vitro BBB models or μBBB) can potentially be used as preclinical drug screening and toxicological assessment tools and are being developed intensively (230, 231), similarly to the other tumor-on-a-chip models including vascularized structures providing cell culture and co-culture and also the cell-free models [e.g., parallel artificial membrane permeability assays (PAMPA)] (222, 232). Brain-on-a-chip models have been developed in an attempt to address many of the limitations found in other models and have been used with human cells and dynamic systems to create microphysiological models exhibiting specific functions and unique brain tissue regions (233). The design of a μBBB must consider two main areas: the “blood side,” mimicking the microvascular lumen of the brain capillary bed; and the “brain side,” mimicking the abluminal side of the capillaries and the brain parenchyma (234). μBBB exhibit several key advantages (235): the chips are easy to design and fabricate; microchannel sizes are similar to microvascular structures in vivo; multi-dimensional network structures resemble the microenvironment in vivo; it is straightforward to combine and integrate various functional units.
One of the common approaches in modeling BBB is to measure the electrical resistance across the barrier with a technique called transendothelial electrical resistance (TEER), which uses electrical resistance between two electrodes placed across the BBB (often used in combination with growing endothelial cells in a transwell model) as a surrogate measure of permeability (75). Since the study of TEER and permeability alone does not offer a great insight on pharmacophysiology of BBB due to its association with a complex communicating module, which makes it important to fabricate the whole cerebrovasculature on a chip (207). In this case, for example, a modular chip housing the 3D culturing of ECs from rat brain with 95% of astrocytes, 1% of microglia and rest of neuronal cells can serve as a potential tool for screening the therapeutic agents developed to cure neurological diseases (207).
In vitro BBB models include cell culture models, brain slice models, fiber-based dynamic in vitro BBB (DIV-BBB) models, and μBBB (235). Organoid-based BBB models have also been developed as cell-based, 3D models in the absence of biomaterials (236). Organoids are self-organized cellular structures that can be derived directly from patient tissue or using developmental biology and exhibit characteristics of several organs, which include brain, along with pancreas, gut, retina, and brain (237). The native patient tissue, instead of the standard cell lines, is used to make organoids-on-chip a real part of pre-clinical and clinical research in the field of effective precision medicine (237).
Despite the quite long period of BBB-models development, this area is still attracting scientific interest (238). The use of μBBB can improve BBB modeling by having more realistic dimensions and geometries, and by exposing the endothelium to physiological fluid flow (36). Some authors additionally introduce “μBBB+” models considering non-malignant disorders (239). Developmental and degenerative brain diseases can be characterized with pathological biomarkers often associated with cerebral flow and vasculature (240). The microfluidic BBB model can provide opportunities to regulate the transport of signaling molecules, nutrients, growth factors, and drugs for biological research without incorporating animal or human models, limiting ethical concerns (241). Fluid flow at the BBB plays an important role in maintaining barrier functions and homeostasis of CNS, which is partially caused by shear stress generated by circulatory blood flow in the brain capillaries ranging from 5 to 23 dyn/cm2 (242). The currently existing systems for creating flow inside the microfluidic chip can be divided into the two main groups: passive (including gravity-induced, capillary action, surface tension, vacuum suction, osmosis, pressure-driven) and active (syringe pumps, micropumps, electromagnetic and magnetic valves, and vacuum pumps). One of the most commonly applied methods to create flow in microfluidic devices is a use of syringe pumps (243).
One of the most common used platforms of in vitro BBB models is created using transwells with 10 μm thick polystyrene or polycarbonate membranes with 400 nm pores with a 108 pores/mm2 pore density (220). This BBB model involves the growth of a monolayer of endothelial cells in a transwell insert, which represents the blood or the luminal side, whereas the well in which it is inserted represents the abluminal or parenchymal side (244). This quite simple BBB model is still intensively used and most capable of high-throughput drug screening due to its low cost and relatively easy use (245, 246). The neurovascular unit (NVU) is the minimal functional unit of the brain. It is composed of vascular cells, glial cells, and neurons (247). BBB models can employ primary and immortalized cells from human, rodent, bovine, and porcine sources, neurovascular unit (NVU) cells derived from pluripotent stem cell or neural stem cell sources.
NVU is the system responsible for maintaining the proper internal environment of the CNS through control and modulation of BBB functions and cerebral blood flow (248). The crosstalk between the cells of the NVU is crucial for the formation and maintenance of a functional BBB (249). Up to date, one of the most complex models of the BBB is NVU-on-a-chip (248). Modulation of stem cells differentiation into BBB phenotypic cells can boost the development of clinically relevant NVU models for basic and translational studies and drug development (249). Stem cells could deliver a breakthrough in BBB modeling allowing for the development of the desired cell cultures in situ within the platform itself (250).
A brief outline of BBB models and their major applications in drug development and pharmaceutical research is provided in Figure 17 (251).
Figure 17. A scheme showing the application of various BBB models in drug development and pharmaceutical research. Reprinted from Augustine et al. (251), license CC BY 4.0.
Models comprising pluripotent stem cells-epithelial cells and primary astrocytes/glioma cells, pericytes (and neurons), were revealed to be the most suitable replacements for current in vivo models for the purpose of assessing permeability (252). 3D methods have been evolved into emergent 3D systems, such as self-assembled microvasculatures via angiogenic sprouting or vasculogenesis and organoids that enable the generation of all brain cell types, in some cases with internal vasculatures (253). The inclusion of human-derived cells, tissues, and patient samples is highly recommended to improve predictive power not only in cancer, but also in non-malignant disorders, including dementia research (254). The development of in vitro 3D models able to emulate the intrinsic features of the NVU in a concrete pathology may be of significant benefit to assess the transport of nanomedicines across the diseased cerebral vasculature and opens new avenues to assess the therapeutic potential of nanomedicines for these pathologies (255). 3D spheroid models are being increasingly developed for a variety of pediatric brain cancers replicating elements of the tumor microenvironment such as a gradient distribution of nutrients, oxygen, pH, cell-cell, and cell-extracellular contact (255). Despite altered BBB functions are observed in several diseases of the CNS, little is known about possible tissue size-dependent effects on barrier function, which could severely limit the reproducibility of current in vitro spheroid models (256).
3D μBBB model can be successfully used to study the effect of physiological flow on BBB integrity, including the role of separated flow in the development of pathologies, such as atherosclerosis and aneurysm (257). A microfluidic system can be used for mimicking cellular blood vessel barriers providing means to study the transport of biomolecules (e.g., lipoprotein particles, extracellular vesicles, but also lipids and hormones) across the barrier (257). 3D μBBB models were shown to be perceptive in parasitology research and therefore can be applied to study various microbial agents with neurotropism (258). The utility of the human NVU chip was shown for the real-time observation and quantification of fungal brain penetration and neurotropism (259). Duong et al. (260) designed and fabricated a microfluidic device based on a sandwiched cellulose fiber membrane, which can be used as an easy and cheap functional 3D in vitro BBB model for short experiments. A conventional engineered transwell BBB model is still being actively used, and Vakilian et al. (261) demonstrated the anti-metastatic effect of β-boswellic acid by deteriorating cancer cells as well as improving barrier integrity. A millifluidic device compatible with standard transwell inserts was developed to examine the impact of primary human pericytes and astrocytes on human brain microvascular endothelial cells barrier integrity (262).
A synergistic engineering approach toward 3D vascularized glioblastoma multiforme (GBM)-on-chip model has been developed based on sealed microfluidic channels, endothelialized to mimic the BBB, together with a 3D bioprinted GBM model (263). The complicated GBM microenvironment and the anatomical features and functionality of the in vivo BBB was developed and the influence of the GBM microenvironment on tumor behavior and drug delivery was demonstrated with the use of a human BBB model based on co-culturing BBB-composing cells within a 3D hydrogel matrix (264). Studies with a use of 3D bioprinting and biomaterials to construct GBM and BBB models have demonstrated physiologically relevant properties and improved features compared to traditional models (265). The capability of the BBB-GBM platform was shown to screen therapeutic nanoparticles (NPs) and predict in vivo efficacy, demonstrating improved efficacy of cisplatin when encapsulated in GBM-targeting layer-by-layer NPs both in vitro and in vivo (266).
Preliminary data was shown to demonstrate how system based on co-culturing of tumor cells in a hydrogel mimicking tumor extracellular matrix along with a cellular barrier can be used to monitor the growth of micro-tumors and their interaction with a cellular barrier and migration into the perfusion channel (267). Hajal et al. (268) fabricated an engineered human μBBB model and measured physiologically relevant molecular permeability, which may be used in academic or industry laboratories to study and predict transport across the BBB. The impact of shear stress was demonstrated to an easy to isolate, user-friendly and highly reproducible BBB model derived from human brain cell line and human primary astrocytes co-cultured under continuous laminar flow which can be used to study the permeation and migration of different cell types through a tightly formed BBB under healthy and inflammatory states (269). In the single- and double-channel devices, Martins et al. (270) developed a 3D GBM model and simulated the direct injection/application of chemotherapy at the tumor site (i.e., in situ); the double-channel microfluidic chip has two parallel channels connected by micropillars to mimic the vascular and parenchymal-cancer compartment and therefor to simulate the systemic administration of chemotherapy.
Lam et al. (271) developed a model of GBM and its accompanying BBB that recapitulates clinically relevant features, such as tumor spatial heterogeneity and GBM-BBB interactions. The model allowed to investigate the role of tumor spatial heterogeneity and the BBB in Temozolomide resistance, and to carry out unlabeled proteomic screening to identify potential proteins involved in tumorigenesis and Temozolomide resistance. A tissue-engineered model was developed that provides new insight into changes in brain-tumor barrier phenotype during metastatic breast cancer, which may motivate new therapeutic approaches (228).
The effects of tumor treating fields (by example of electromagnetic field with a frequency of 100 kHz) were examined on the BBB in a 3D co-culture model consisting of primary human brain microvascular endothelial cells and immortalized human pericytes, temporary increase of BBB permeability in an in vitro model of human origin was proven (272). A BBB-glioma chip model was developed reconstituted with human brain microvascular endothelial cells, astrocytes, and pericytes under dynamic culture and U251 cells culturing in Matrigel as cells cluster to mimic the glioma microenvironment for the evaluation of permeability and drug efficacy of potential anti-glioma components of traditional Chinese medicine (273). A blood–brain niche microfluidic chip was developed to characterize alterations to the brain niche and cancer cell metastatic progression to characterize phenotypic and secretory cues provided by individual cellular residents of the brain niche, strocytes and microglia, which attract metastatic cancer cells (274).
The information summarizing various examples of up-to-date in vitro BBB models for neuro-oncology of the most popular 3D-cell culture microfluidic dynamic and transwell-based static types is given in Table 1.
Summarizing the information on the current state of the in vitro BBB models intended for perspective applications in the field of preclinical study of neuro-oncology drug testing, some main tendencies can be formulated. First refers to the use of the modern techniques including 3D dynamic models based on cell co-culturing in gel matrices. And the second refers to the continuous use of rather simple Transwell-based models despite of the more than 50 years history of their development and application. New techniques for modeling brain tumors on microfluidic platforms represent an important step in overcoming the limitations of traditional approaches and help accelerate the development of more effective and safe treatment.
Tumor-on-a-chip (ToC) models play an important role in studying tumor microenvironment, invasion, angiogenesis, and drug response. Classification of such models is based on the type of simulated structure, the number of microchannels, and the presence of a vascular network, which allows the creation of both simple 2D models and more complex 3D systems to mimic developed vascular networks or i tumor behavior when interacting with the blood-brain barrier. Analyzing various approaches to creating ToCs, we can conclude that the creation of a specific type of system and the integration of various sensor elements into it is determined by the set of parameters that researchers need to obtain using such a system. Now, there is no optimal universal solution that would satisfy all the requirements and research goals. However, some general trends and directions in the development of ToCs can be identified that can improve their performance, functionality, and applicability.
There is a trend toward increasing the complexity and realism of models by reproducing more accurate and complete structure and function of the tumor microenvironment, including various types and ratios of cells, extracellular matrix, vascularization, barriers, gradients, forces, and other factors that influence the behavior and fate of tumor cells. Hybrid integration of modern micro- and nanotechnologies with cellular technologies opens new possibilities for creating high-precision models of brain ToCs. The use of microfluidics and microfabrication can more accurately reproduce the processes of angiogenesis, invasion, and tumor response to therapy. However, as the complexity of such systems increases, their cost also increases. This is due to the need for expensive equipment, complex materials, and high requirements for personnel qualifications. To make tumor-on-a-chip technologies more accessible, modular platforms can be introduced that will allow researchers to adapt the systems to their needs without significant costs. Active partnerships between academia and industry can reduce the cost of developing and distributing such systems. This will also allow the development of standardized solutions that will be easily integrated into research worldwide, providing broad access to advanced technologies.
In addition, there is a need to increase the degree of automation and standardization of models using more universal and interoperable platforms, protocols, interfaces, and software. This will make it easier and faster to create, configure, manage, and analyze tumor cultures using ToCs. In addition, the implementation of modern solutions based on information technology and artificial intelligence will simplify the management of complex experiments and the analysis of large volumes of data, which will make the research process not only faster but also cheaper. This will also lead to more efficient use of resources and a reduction in errors associated with manual operations. As a result, increasing the level of automation and standardization will be an important step toward the development of more accessible and scalable models in biomedical research. A separate challenge is to improve the sensitivity, resolution, and integration of models using more advanced and miniaturized sensors that can detect and measure a wider range of parameters related to brain tumors or their treatment, as well as transmit and process these signals in real time and with high accuracy and reliability.
Thus, ToCs for brain tumor mimicking represent a promising and innovative technology that can significantly improve the understanding of the mechanisms and processes associated with brain tumors, as well as contribute to the development of new and effective strategies for the early diagnostics, therapy, and prevention of this diseases.
TZ: Conceptualization, Supervision, Writing – original draft, Writing – review & editing. NS: Conceptualization, Formal analysis, Funding acquisition, Methodology, Project administration, Software, Supervision, Visualization, Writing – original draft, Writing – review & editing. KG: Conceptualization, Methodology, Project administration, Supervision, Validation, Writing – original draft, Writing – review & editing. NM: Writing – review & editing. SC: Writing – review & editing. MS: Funding acquisition, Project administration, Supervision, Writing – original draft, Writing – review & editing.
The author(s) declare financial support was received for the research, authorship, and/or publication of this article. This work was financially supported by the Ministry of Science and Higher Education of the Russian Federation (Agreement No. 075-15-2022-301), Technische Universität München (TUM) within the DFG funding programme Open Access Publishing.
The authors declare that the research was conducted in the absence of any commercial or financial relationships that could be construed as a potential conflict of interest.
All claims expressed in this article are solely those of the authors and do not necessarily represent those of their affiliated organizations, or those of the publisher, the editors and the reviewers. Any product that may be evaluated in this article, or claim that may be made by its manufacturer, is not guaranteed or endorsed by the publisher.
1. Cancer Prevention Science Communication. WCRF (2023). Available at: https://www.wcrf.org/world-cancer-day-2023-working-to-prevent-cancer-worldwide/ (accessed February 13, 2024).
2. Miller KD, Ostrom QT, Kruchko C, Patil N, Tihan T, Cioffi G, et al. Brain and other central nervous system tumor statistics, 2021. CA Cancer J Clin. (2021) 71:21693. doi: 10.3322/caac.21693
3. Louis DN, Perry A, Wesseling P, Brat DJ, Cree IA, Figarella-Branger D, et al. The 2021 WHO classification of tumors of the central nervous system: a summary. Neuro Oncol. (2021) 23:1231–51. doi: 10.1093/neuonc/noab106
4. Gousias K, Trakolis L, Simon M. Meningiomas with CNS invasion. Front Neurosci. (2023) 17:1189606. doi: 10.3389/fnins.2023.1189606
5. Choi JY. Medulloblastoma: current perspectives and recent advances. Brain Tumor Res Treat. (2023) 11:28. doi: 10.14791/btrt.2022.0046
6. Mohammed A, Hamdan A, Homoud A. Histopathological profile of brain tumors: a 12-year retrospective study from Madinah, Saudi Arabia. Asian J Neurosurg. (2019) 14:1106–11. doi: 10.4103/ajns.AJNS_185_19
7. Pertz M, Schlegel U, Thoma P. Sociocognitive functioning and psychosocial burden in patients with brain tumors. Cancers. (2022) 14:30767. doi: 10.3390/cancers14030767
8. Global Cancer Observatory. Cancer Today. Lyon: International Agency for Research on Cancer. Available at: https://gco.iarc.fr/today (accessed February 01, 2024).
9. Andersen BM, Faust Akl C, Wheeler MA, Chiocca EA, Reardon DA, Quintana FJ. Glial and myeloid heterogeneity in the brain tumour microenvironment. Nat Rev Cancer. (2021) 21:786–802. doi: 10.1038/s41568-021-00397-3
10. Nafe R, Hattingen E. Cellular components of the tumor environment in gliomas-what do we know today? Biomedicines. (2024) 12:10014. doi: 10.3390/biomedicines12010014
11. Sai Krishna AVS, Ramu A, Hariharan S, Sinha S, Donakonda S. Characterization of tumor microenvironment in glioblastoma multiforme identifies ITGB2 as a key immune and stromal related regulator in glial cell types. Comput Biol Med. (2023) 165:107433. doi: 10.1016/j.compbiomed.2023.107433
12. Demicco M, Liu XZ, Leithner K, Fendt SM. Metabolic heterogeneity in cancer. Nat Metab. (2024) 6:18–38. doi: 10.1038/s42255-023-00963-z
13. Goenka A, Khan F, Verma B, Sinha P, Dmello CC, Jogalekar MP, et al. Tumor microenvironment signaling and therapeutics in cancer progression. Cancer Commun. (2023) 43:525–61. doi: 10.1002/cac2.12416
14. Seaman K, Sun Y, You L. Recent advances in cancer-on-a-chip tissue models to dissect the tumour microenvironment. Med-X. (2023) 1:11. doi: 10.1007/s44258-023-00011-1
15. Cancer. Key Facts (2022). Available at: https://www.who.int/news-room/fact-sheets/detail/cancer (accessed February 01, 2024).
16. Leung CM, de Haan P, Ronaldson-Bouchard K, Kim G-A, Ko J, Rho HS, et al. A guide to the organ-on-a-chip. Nat Rev Methods Prim. (2022) 2:1–29. doi: 10.1038/s43586-022-00118-6
17. Wang Z, Zhang Y, Li Z, Wang H, Li N, Deng Y. Microfluidic brain-on-a-chip: from key technology to system integration and application. Small. (2023) 19:4427. doi: 10.1002/smll.202304427
18. Zhao C, Wang Z, Tang X, Qin J, Jiang Z. Recent advances in sensor-integrated brain-on-a-chip devices for real-time brain monitoring. Coll Surf B Biointerf. (2023) 229:113431. doi: 10.1016/j.colsurfb.2023.113431
19. Li Z, Zhao Y, Lv X, Deng Y. Integrated brain on a chip and automated organ-on-chips systems. Interdiscip Med. (2023) 1:2 doi: 10.1002/INMD.20220002
20. Andolfi A, Arnaldi P, Donatella Di L, Pepe S, Frega M, Fassio A, et al. A micropatterned thermoplasmonic substrate for neuromodulation of in vitro neuronal networks. Acta Biomater. (2023) 158:281–91. doi: 10.1016/j.actbio.2022.12.036
21. Barbato MG, Pereira RC, Mollica H, Palange AL, Ferreira M, Decuzzi P, et al. A permeable on-chip microvasculature for assessing the transport of macromolecules and polymeric nanoconstructs. J Coll Interface Sci. (2021) 594:409–23. doi: 10.1016/j.jcis.2021.03.053
22. Lei Y, Li J, Wang N, Yang X, Hamada Y, Li Q, et al. An on-chip model for investigating the interaction between neurons and cancer cells. Integr Biol. (2016) 8:359–67. doi: 10.1039/c5ib00309a
23. Liu X, Zhang W, Zheng W, Jiang X. Micropatterned coculture platform for screening nerve-related anticancer drugs. ACS Nano. (2021) 15:637–49. doi: 10.1021/acsnano.0c06416
24. Brooks A, Zhang Y, Chen J, Zhao CX. Cancer metastasis-on-a-chip for modeling metastatic cascade and drug screening. Adv Healthc Mater. (2024) 2023:2436. doi: 10.1002/adhm.202302436
25. Jouybar M, de Winde CM, Wolf K, Friedl P, Mebius RE, den Toonder JMJ. Cancer-on-chip models for metastasis: importance of the tumor microenvironment. Trends Biotechnol. (2024) 42:431–48. doi: 10.1016/j.tibtech.2023.10.001
26. Papp D, Korcsmaros T, Hautefort I. Revolutionizing immune research with organoid-based co-culture and chip systems. Clin Exp Immunol. (2024) 2024:uxae004. doi: 10.1093/cei/uxae004
27. Ustun M, Dabbagh SR, Ilci IS, Bagci-Onder T, Tasoglu S. Glioma-on-a-chip models. Micromachines. (2021) 12:50490. doi: 10.3390/mi12050490
28. Alves AH, Nucci MP, Mamani JB, Valle NME, Ribeiro EF, Rego GNA, et al. The advances in glioblastoma on-a-chip for therapy approaches. Cancers. (2022) 14:40869. doi: 10.3390/cancers14040869
29. Khan ZS, Vanapalli SA. Probing the mechanical properties of brain cancer cells using a microfluidic cell squeezer device. Biomicrofluidics. (2013) 7:4774310. doi: 10.1063/1.4774310
30. Kim H, Sa JK, Kim J, Cho HJ, Oh HJ, Choi DH, et al. Recapitulated crosstalk between cerebral metastatic lung cancer cells and brain perivascular tumor microenvironment in a microfluidic co-culture chip. Adv Sci. (2022) 9:1785. doi: 10.1002/advs.202201785
31. Tsai HF, Trubelja A, Shen AQ, Bao G. Tumour-on-a-chip: microfluidic models of tumour morphology, growth and microenvironment. J R Soc Interface. (2017) 14:137. doi: 10.1098/rsif.2017.0137
32. Kawakita S, Mandal K, Mou L, Mecwan MM, Zhu Y, Li S, et al. Organ-on-a-chip models of the blood-brain barrier: recent advances and future prospects. Small. (2022) 18:1401. doi: 10.1002/smll.202201401
33. Terrell-Hall TB, Ammer AG, Griffith JIG, Lockman PR. Permeability across a novel microfluidic blood-tumor barrier model. Fluids Barriers CNS. (2017) 14:9. doi: 10.1186/s12987-017-0050-9
34. Erb U, Schwerk C, Schroten H, Karremann M. Review of functional in vitro models of the blood-cerebrospinal fluid barrier in leukaemia research. J Neurosci Methods. (2020) 329:108478. doi: 10.1016/j.jneumeth.2019.108478
35. Yu F, Selva Kumar ND, Choudhury D, Foo LC, Ng SH. Microfluidic platforms for modeling biological barriers in the circulatory system. Drug Discov Today. (2018) 23:815–29. doi: 10.1016/j.drudis.2018.01.036
36. van der Helm MW, van der Meer AD, Eijkel JCT, van den Berg A, Segerink LI. Microfluidic organ-on-chip technology for blood-brain barrier research. Tissue Barriers. (2016) 4:1142493. doi: 10.1080/21688370.2016.1142493
37. Fan Y, Xu C, Deng N, Gao Z, Jiang Z, Li X, et al. Understanding drug nanocarrier and blood-brain barrier interaction based on a microfluidic microphysiological model. Lab Chip. (2023) 23:1935–44. doi: 10.1039/D2LC01077A
38. Lin L, Wang X, Niu M, Wu Q, Wang H, Zu Y, et al. Biomimetic epithelium/endothelium on chips. Eng Regen. (2022) 3:201–16. doi: 10.1016/j.engreg.2022.05.001
39. Yu F, Nivasini SK, Foo LC, Ng SH, Hunziker W, Choudhury D, et al. pump-free tricellular blood-brain barrier on-a-chip model to understand barrier property and evaluate drug response. Biotechnol Bioeng. (2020) 117:1127–36. doi: 10.1002/bit.27260
40. Carton F, Malatesta M. In vitro models of biological barriers for nanomedical research. Int J Mol Sci. (2022) 23:168910. doi: 10.3390/ijms23168910
41. Eltanameli B, Piñeiro-Llanes J, Cristofoletti R. Recent advances in cell-based in vitro models for predicting drug permeability across brain, intestinal, and pulmonary barriers. Expert Opin Drug Metab Toxicol. (2024) 20:439–58. doi: 10.1080/17425255.2024.2366390
42. Driver R, Mishra S. Organ-on-a-chip technology: an in-depth review of recent advancements and future of whole body-on-chip. Biochip J. (2023) 17:1–23. doi: 10.1007/s13206-022-00087-8
43. Keshavarz M, Ahmadi Nasab N. Cell culture techniques in microfluidic chips. In: Principles of Human Organs-on-Chips. Sawston, CA: Woodhead Publishing (2023). p. 89–121.
44. Wanigasekara J, Cullen PJ, Bourke P, Tiwari B, Curtin JF. Advances in 3D culture systems for therapeutic discovery and development in brain cancer. Drug Discov Today. (2023) 28:103426. doi: 10.1016/j.drudis.2022.103426
45. Marino A, Battaglini M, Carmignani A, Pignatelli F, De Pasquale D, Tricinci O, et al. Magnetic self-assembly of 3D multicellular microscaffolds: a biomimetic brain tumor-on-a-chip for drug delivery and selectivity testing. APL Bioeng. (2023) 7:155037. doi: 10.1063/5.0155037
46. Cauli E, Polidoro MA, Marzorati S, Bernardi C, Rasponi M, Lleo A. Cancer-on-chip: a 3D model for the study of the tumor microenvironment. J Biol Eng. (2023) 17:6. doi: 10.1186/s13036-023-00372-6
47. Sorhun DT, Ozturk E. The effect of culture dimensionality and brain extracellular matrix in neuronal differentiation. Eur J Biol. (2023) 82:142–53. doi: 10.26650/EurJBiol.2023.1317681
48. Hwangbo H, Chae SJ, Kim W, Jo S, Kim GH. Tumor-on-a-chip models combined with mini-tissues or organoids for engineering tumor tissues. Theranostics. (2024) 14:33–55. doi: 10.7150/thno.90093
49. Islam M, Motasim Bellah M, Sajid A, Raziul Hasan M, Kim YT, Iqbal SM. Effects of nanotexture on electrical profiling of single tumor cell and detection of cancer from blood in microfluidic channels. Sci Rep. (2015) 5:13031. doi: 10.1038/srep13031
50. Salman MM, Marsh G, Kusters I, Delincé M, Di Caprio G, Upadhyayula S, et al. Design and validation of a human brain endothelial microvessel-on-a-chip open microfluidic model enabling advanced optical imaging. Front Bioeng Biotechnol. (2020) 8:573775. doi: 10.3389/fbioe.2020.573775
51. Song Y, Shou X, Sheng B, Mei J, Shi K, Shang L, et al. Cell membranes from tumor-tropic MSCs screened by a microfluidic chip for drug nanoparticles encapsulation and cancer targeted therapy. Adv Healthc Mater. (2023) 12:2904. doi: 10.1002/adhm.202202904
52. Geng X, Zhou ZA, Mi Y, Wang C, Wang M, Guo C, et al. Glioma single-cell biomechanical analysis by cyclic conical constricted microfluidics. Anal Chem. (2023) 95:15585–94. doi: 10.1021/acs.analchem.3c02434
53. Hachey SJ, Hughes CCW. Applications of tumor chip technology. Lab Chip. (2018) 18:2893–912. doi: 10.1039/C8LC00330K
54. Li W, Zhou Z, Zhou X, Khoo BL, Gunawan R, Chin YR, et al. 3D biomimetic models to reconstitute tumor microenvironment in vitro: spheroids, organoids, and tumor-on-a-chip. Adv Healthc Mater. (2023) 12:2609. doi: 10.1002/adhm.202202609
55. Kwee BJ, Li X, Nguyen XX, Campagna C, Lam J, Sung KE. Modeling immunity in microphysiological systems. Exp Biol Med. (2023) 248:2001–19. doi: 10.1177/15353702231215897
56. Villasante A, Lopez-Martinez MJ, Quiñonero G, Garcia-Lizarribar A, Peng X, Samitier J. Microfluidic model of the alternative vasculature in neuroblastoma. Vitr Model. (2024) 3:49–63. doi: 10.1007/s44164-023-00064-x
57. Bae J, Kim MH, Han S, Park S. Development of tumor-vasculature interaction on chip mimicking vessel co-option of glioblastoma. Biochip J. (2023) 17:77–84. doi: 10.1007/s13206-022-00090-z
58. Rama Varma A, Fathi P. Vascularized microfluidic models of major organ structures and cancerous tissues. Biomicrofluidics. (2023) 17:159800. doi: 10.1063/5.0159800
59. Dzobo K, Dandara C. The extracellular matrix: its composition, function, remodeling, and role in tumorigenesis. Biomimetics. (2023) 8:v1. doi: 10.20944/preprints202301.0555.v1
60. Afshar K, Sanaei MJ, Ravari MS, Pourbagheri-Sigaroodi A, Bashash D. An overview of extracellular matrix and its remodeling in the development of cancer and metastasis with a glance at therapeutic approaches. Cell Biochem Funct. (2023) 41:930–52. doi: 10.1002/cbf.3846
61. Aazmi A, Zhang D, Mazzaglia C, Yu M, Wang Z, Yang H, et al. Biofabrication methods for reconstructing extracellular matrix mimetics. Bioact Mater. (2024) 31:475–96. doi: 10.1016/j.bioactmat.2023.08.018
62. Tamayo-Angorrilla M, López de Andrés J, Jiménez G, Marchal JA. The biomimetic extracellular matrix: a therapeutic tool for breast cancer research. Transl Res. (2022) 247:117–36. doi: 10.1016/j.trsl.2021.11.008
63. Kutluk H, Bastounis EE, Constantinou I. Integration of extracellular matrices into organ-on-chip systems. Adv Healthc Mater. (2023) 12:3256. doi: 10.1002/adhm.202203256
64. Ariati R, Sales F, Souza A, Lima RA, Ribeiro J. Polydimethylsiloxane composites characterization and its applications: a review. Polymers. (2021) 13:234258. doi: 10.3390/polym13234258
65. Miranda I, Souza A, Sousa P, Ribeiro J, Castanheira EMS, Lima R, et al. Properties and applications of PDMS for biomedical engineering: a review. J Funct Biomater. (2022) 13:1002. doi: 10.3390/jfb13010002
66. Campbell SB, Wu Q, Yazbeck J, Liu C, Okhovatian S, Radisic M. Beyond polydimethylsiloxane: alternative materials for fabrication of organ-on-a-chip devices and microphysiological systems. ACS Biomater Sci Eng. (2021) 7:2880–99. doi: 10.1021/acsbiomaterials.0c00640
67. Luo Y, Li X, Zhao Y, Zhong W, Xing M, Lyu G. Development of organs-on-chips and their impact on precision medicine and advanced system simulation. Pharmaceutics. (2023) 15:82094. doi: 10.3390/pharmaceutics15082094
68. Chong H, Majerus SJA, Bogie KM, Zorman CA. Non-hermetic packaging of biomedical microsystems from a materials perspective: a review. Med Devices Sensors. (2020) 3:10082. doi: 10.1002/mds3.10082
69. Shakeri A, Jarad NA, Khan S, Didar FT. Bio-functionalization of microfluidic platforms made of thermoplastic materials: a review. Anal Chim Acta. (2022) 1209:339283. doi: 10.1016/j.aca.2021.339283
70. Osório LA, Silva E, Mackay RE. A review of biomaterials and scaffold fabrication for organ-on-a-chip (OOAC) systems. Bioengineering. (2021) 8:113. doi: 10.3390/bioengineering8080113
71. Tajeddin A, Mustafaoglu N. Design and fabrication of organ-on-chips: promises and challenges. Micromachines. (2021) 12:1443. doi: 10.3390/mi12121443
72. Schneider S, Brás EJS, Schneider O, Schlünder K, Loskill P. Facile patterning of thermoplastic elastomers and robust bonding to glass and thermoplastics for microfluidic cell culture and organ-on-chip. Micromachines. (2021) 12:575. doi: 10.3390/mi12050575
73. Dsouza VL, Kuthethur R, Kabekkodu SP, Chakrabarty S. Organ-on-chip platforms to study tumor evolution and chemosensitivity. Biochim Biophys Acta Rev Cancer. (2022) 1877:17. doi: 10.1016/j.bbcan.2022.188717
74. Zhao Z, Vizetto-Duarte C, Moay ZK, Setyawati MI, Rakshit M, Kathawala MH, et al. Composite hydrogels in three-dimensional in vitro models. Front Bioeng Biotechnol. (2020) 8:611. doi: 10.3389/fbioe.2020.00611
75. Potjewyd G, Kellett KAB, Hooper NM. 3D hydrogel models of the neurovascular unit to investigate blood-brain barrier dysfunction. Neuronal Signal. (2021) 5:1–24. doi: 10.1042/NS20210027
76. Oh H, Kang M, Bae E, Jung Y, Cho J, Poirier J, et al. Fabrication of hydrogel microchannels using aqueous two-phase printing for 3D blood brain barrier. Biochip J. (2023) 17:369–83. doi: 10.1007/s13206-023-00110-6
77. Ahmed T. Functional biomaterials for biomimetic 3D in vitro tumor microenvironment modeling. Vitr Model. (2023) 2:1–23. doi: 10.1007/s44164-023-00043-2
78. Chen Z, Lee JB. Biocompatibility of su-8 and its biomedical device applications. Micromachines. (2021) 12:70794. doi: 10.3390/mi12070794
79. Wang Y, Zhu J, Chen P, Li Y, Yan S, Wang J, et al. Wound-on-a-chip: high-throughput 3D wound healing assay with a novel SU-8 mesh chip. Sens Actuat B Chem. (2019) 280:86–93. doi: 10.1016/j.snb.2018.10.050
80. da Ponte RM, Gaio N, van Zeijl H, Vollebregt S, Dijkstra P, Dekker R, et al. Monolithic integration of a smart temperature sensor on a modular silicon-based organ-on-a-chip device. Sens Actuat A Phys. (2021) 317:112439. doi: 10.1016/j.sna.2020.112439
81. Huh D, Kim HJ, Fraser JP, Shea DE, Khan M, Bahinski A, et al. Microfabrication of human organs-on-chips. Nat Protoc. (2013) 8:2135–57. doi: 10.1038/nprot.2013.137
82. Marrero D, Guimera A, Maes L, Villa R, Alvarez M, Illa X. Erratum: Organ-on-a-chip with integrated semitransparent organic electrodes for barrier function monitoring (Lab on a Chip (2023). Lab Chip. (2023) 23:1954. doi: 10.1039/D3LC90025H
83. Holzreuter MA, Segerink LI. Innovative electrode and chip designs for transendothelial electrical resistance measurements in organs-on-chips. Lab Chip. (2024) 24:1121–34. doi: 10.1039/D3LC00901G
84. Esch MB, Sung JH, Yang J, Yu C, Yu J, March JC, et al. On chip porous polymer membranes for integration of gastrointestinal tract epithelium with microfluidic “body-on-a-chip” devices. Biomed Microdevices. (2012) 14:895–906. doi: 10.1007/s10544-012-9669-0
85. Rahimnejad M, Rasouli F, Jahangiri S, Ahmadi S, Rabiee N, Ramezani Farani M, et al. Engineered biomimetic membranes for organ-on-a-chip. ACS Biomater Sci Eng. (2022) 8:5038–59. doi: 10.1021/acsbiomaterials.2c00531
86. Stokes K, Clark K, Odetade D, Hardy M, Goldberg Oppenheimer P. Advances in lithographic techniques for precision nanostructure fabrication in biomedical applications. Discov Nano. (2023) 18:3938. doi: 10.1186/s11671-023-03938-x
87. Gimondi S, Ferreira H, Reis RL, Neves NM. Microfluidic devices: a tool for nanoparticle synthesis and performance evaluation. ACS Nano. (2023) 17:14205–28. doi: 10.1021/acsnano.3c01117
88. Puryear JR, Yoon JK, Kim YT. Advanced fabrication techniques of microengineered physiological systems. Micromachines. (2020) 11:80730. doi: 10.3390/mi11080730
89. Scott SM, Ali Z. Fabrication methods for microfluidic devices: an overview. Micromachines. (2021) 12:30319. doi: 10.3390/mi12030319
90. Kodihalli Shivaprakash N, Banerjee PS, Banerjee SS, Barry C, Mead J. Advanced polymer processing technologies for micro- and nanostructured surfaces: a review. Polym Eng Sci. (2023) 63:1057–81. doi: 10.1002/pen.26280
91. Al-Kattan A, Grojo D, Drouet C, Mouskeftaras A, Delaporte P, Casanova A, et al. Short-pulse lasers: a versatile tool in creating novel nano-/micro-structures and compositional analysis for healthcare and wellbeing challenges. Nanomaterials. (2021) 11:1–34. doi: 10.3390/nano11030712
92. Saorin G, Caligiuri I, Rizzolio F. Microfluidic organoids-on-a-chip: the future of human models. Semin Cell Dev Biol. (2023) 144:41–54. doi: 10.1016/j.semcdb.2022.10.001
93. Han X, Zhang Y, Tian J, Wu T, Li Z, Xing F, et al. Polymer-based microfluidic devices: a comprehensive review on preparation and applications. Polym Eng Sci. (2022) 62:3–24. doi: 10.1002/pen.25831
94. Milton LA, Viglione MS, Ong LJY, Nordin GP, Toh YC. Vat photopolymerization 3D printed microfluidic devices for organ-on-a-chip applications. Lab Chip. (2023) 23:3537–60. doi: 10.1039/D3LC00094J
95. Carvalho V, Gonçalves I, Lage T, Rodrigues RO, Minas G, Teixeira SFCF, et al. 3D printing techniques and their applications to organ-on-a-chip platforms: a systematic review. Sensors. (2021) 21:93304. doi: 10.3390/s21093304
96. Mehta V, Rath SN. 3D printed microfluidic devices: a review focused on four fundamental manufacturing approaches and implications on the field of healthcare. Bio-Design Manuf. (2021) 4:311–43. doi: 10.1007/s42242-020-00112-5
97. Rahmani Dabbagh S, Rezapour Sarabi M, Birtek MT, Mustafaoglu N, Zhang YS, Tasoglu S. 3D bioprinted organ-on-chips. Aggregate. (2023) 4:197. doi: 10.1002/agt2.197
98. Moghimi N, Hosseini SA, Dalan AB, Mohammadrezaei D, Goldman A, Kohandel M. Controlled tumor heterogeneity in a co-culture system by 3D bio-printed tumor-on-chip model. Sci Rep. (2023) 13:40680. doi: 10.1038/s41598-023-40680-x
99. Unagolla JM, Jayasuriya AC. Hydrogel-based 3D bioprinting: a comprehensive review on cell-laden hydrogels, bioink formulations, and future perspectives. Appl Mater Today. (2020) 18:100479. doi: 10.1016/j.apmt.2019.100479
100. Zheng F, Xiao Y, Liu H, Fan Y, Dao M. Patient-specific organoid and organ-on-a-chip: 3D cell-culture meets 3D printing and numerical simulation. Adv Biol. (2021) 5:24. doi: 10.1002/adbi.202000024
101. Lee G, Kim SJ, Park JK. Fabrication of a self-assembled and vascularized tumor array via bioprinting on a microfluidic chip. Lab Chip. (2023) 23:4079–91. doi: 10.1039/D3LC00275F
102. Feng J, Neuzil J, Manz A, Iliescu C, Neuzil P. Microfluidic trends in drug screening and drug delivery. Trends Anal Chem. (2023) 158:116821. doi: 10.1016/j.trac.2022.116821
103. Soares C, Lee KFH, Cook D, Béïque JC. A cost-effective method for preparing, maintaining, and transfecting neurons in organotypic slices., Methods Mol Biol. (2014) 13:205–19. doi: 10.1007/978-1-4939-1096-0_13
104. Repossi R, Martín-Ramírez R, Gómez-Bernal F, Medina L, Fariña-Jerónimo H, González-Fernández R, et al. Evaluation of zonulin expression and its potential clinical significance in glioblastoma. Cancers. (2024) 16:20356. doi: 10.3390/cancers16020356
105. Nayernia Z, Turchi L, Cosset E, Peterson H, Dutoit V, Dietrich PY, et al. The relationship between brain tumor cell invasion of engineered neural tissues and in vivo features of glioblastoma. Biomaterials. (2013) 34:8279–90. doi: 10.1016/j.biomaterials.2013.07.006
106. Baghban R, Roshangar L, Jahanban-Esfahlan R, Seidi K, Ebrahimi-Kalan A, Jaymand M, et al. Tumor microenvironment complexity and therapeutic implications at a glance. Cell Commun Signal. (2020) 18:4. doi: 10.1186/s12964-020-0530-4
107. Bouquerel C, Dubrova A, Hofer I, Phan DTT, Bernheim M, Ladaigue S, et al. Bridging the gap between tumor-on-chip and clinics: a systematic review of 15 years of studies. Lab Chip. (2023) 23:3906–35. doi: 10.1039/D3LC00531C
108. Chong HK, Ma Z, Wong KKC, Morokoff A, French C. An in vitro brain tumour model in organotypic slice cultures displaying epileptiform activity. Brain Sci. (2023) 13:1451. doi: 10.3390/brainsci13101451
109. Sadahiro H, Kang KD, Gibson JT, Minata M, Yu H, Shi J, et al. Activation of the receptor tyrosine kinase AXL regulates the immune microenvironment in glioblastoma. Cancer Res. (2018) 78:3002–13. doi: 10.1158/0008-5472.CAN-17-2433
110. Behnan J, Isakson P, Joel M, Cilio C, Langmoen IA, Vik-Mo EO, et al. Recruited brain tumor-derived mesenchymal stem cells contribute to brain tumor progression. Stem Cells. (2014) 32:1110–23. doi: 10.1002/stem.1614
111. Sampson JH, Gunn MD, Fecci PE, Ashley DM. Brain immunology and immunotherapy in brain tumours. Nat Rev Cancer. (2020) 20:12–25. doi: 10.1038/s41568-019-0224-7
112. Barbosa MAG, Xavier CPR, Pereira RF, Petrikaite V, Vasconcelos MH. 3D cell culture models as recapitulators of the tumor microenvironment for the screening of anti-cancer drugs. Cancers. (2022) 14:10190. doi: 10.3390/cancers14010190
113. Samadian H, Jafari S, Sepand MR, Alaei L, Sadegh Malvajerd S, Jaymand M, et al. 3D bioprinting technology to mimic the tumor microenvironment: tumor-on-a-chip concept. Mater Today Adv. (2021) 12:100160. doi: 10.1016/j.mtadv.2021.100160
114. Lee J, Lilly D, Doty C, Podsiadlo P, Kotov N. In vitro toxicity testing of nanoparticles in 3D cell culture. Small. (2009) 5:1213–21. doi: 10.1002/smll.200801788
115. Caballero D, Kaushik S, Correlo VM, Oliveira JM, Reis RL, Kundu SC. Organ-on-chip models of cancer metastasis for future personalized medicine: from chip to the patient. Biomaterials. (2017) 149:98–115. doi: 10.1016/j.biomaterials.2017.10.005
116. Miranda CC, Fernandes TG, Diogo MM, Cabral JMS. Towards multi-organoid systems for drug screening applications. Bioengineering. (2018) 5:49. doi: 10.3390/bioengineering5030049
117. Zarrintaj P, Saeb MR, Stadler FJ, Yazdi MK, Nezhad MN, Mohebbi S, et al. Human organs-on-chips: a review of the state-of-the-art, current prospects, and future challenges. Adv Biol. (2022) 6:526. doi: 10.1002/adbi.202000526
118. Foglietta F, Canaparo R, Muccioli G, Terreno E, Serpe L. Methodological aspects and pharmacological applications of three-dimensional cancer cell cultures and organoids. Life Sci. (2020) 254:117784. doi: 10.1016/j.lfs.2020.117784
119. Saydé T, Hamoui O El, Alies B, Gaudin K, Lespes G, Battu S. Biomaterials for three-dimensional cell culture: from applications in oncology to nanotechnology. Nanomaterials. (2021) 11:1–29. doi: 10.3390/nano11020481
120. Yuan X, Curtin J, Xiong Y, Liu G, Waschsmann-Hogiu S, Farkas DL, et al. Isolation of cancer stem cells from adult glioblastoma multiforme. Oncogene. (2004) 23:9392–400. doi: 10.1038/sj.onc.1208311
121. Yehya A, Bahmad H, Abou-Kheir W. In vitro tumorigenic assay: a tumor sphere assay for cancer stem cells. Methods Mol Biol. (2024) 2777:91–8. doi: 10.1007/978-1-0716-3730-2_7
122. Ishiguro T, Ohata H, Sato A, Yamawaki K, Enomoto T, Okamoto K. Tumor-derived spheroids: relevance to cancer stem cells and clinical applications. Cancer Sci. (2017) 108:283–9. doi: 10.1111/cas.13155
123. Ma HL, Jiang Q, Han S, Wu Y, Tomshine JC, Wang D, et al. Multicellular tumor spheroids as an in vivo-like tumor model for three-dimensional imaging of chemotherapeutic and nano material cellular penetration. Mol Imaging. (2012) 11:487–98. doi: 10.2310/7290.2012.00012
124. Gencoglu MF, Barney LE, Hall CL, Brooks EA, Schwartz AD, Corbett DC, et al. Comparative study of multicellular tumor spheroid formation methods and implications for drug screening. ACS Biomater Sci Eng. (2018) 4:410–20. doi: 10.1021/acsbiomaterials.7b00069
125. Lee JM, Park DY, Yang L, Kim EJ, Ahrberg CD, Lee KB, et al. Generation of uniform-sized multicellular tumor spheroids using hydrogel microwells for advanced drug screening. Sci Rep. (2018) 8:7. doi: 10.1038/s41598-018-35216-7
126. Abolhassani H, Zaer M, Shojaosadati SA, Hashemi-Najafabadi S. Rapid generation of homogenous tumor spheroid microtissues in a scaffold-free platform for high-throughput screening of a novel combination nanomedicine. PLoS ONE. (2023) 18:282064. doi: 10.1371/journal.pone.0282064
127. Do TD, Pham UT, Nguyen LP, Nguyen TM, Bui CN, Oliver S, et al. Fabrication of a low-cost microfluidic device for high-throughput drug testing on static and dynamic cancer spheroid culture models. Diagnostics. (2023) 13:81394. doi: 10.3390/diagnostics13081394
128. Brancato V, Oliveira JM, Correlo VM, Reis RL, Kundu SC. Could 3D models of cancer enhance drug screening? Biomaterials. (2020) 232:119744. doi: 10.1016/j.biomaterials.2019.119744
129. Verjans ET, Doijen J, Luyten W, Landuyt B, Schoofs L. Three-dimensional cell culture models for anticancer drug screening: worth the effort? J Cell Physiol. (2018) 233:2993–3003. doi: 10.1002/jcp.26052
130. Heuer C, Preuß JA, Habib T, Enders A, Bahnemann J. 3D printing in biotechnology-an insight into miniaturized and microfluidic systems for applications from cell culture to bioanalytics. Eng Life Sci. (2022) 22:744–59. doi: 10.1002/elsc.202100081
131. Shree B, Tripathi S, Sharma V. Transforming growth factor-beta-regulated LncRNA-MUF promotes invasion by modulating the miR-34a snail1 axis in glioblastoma multiforme. Front Oncol. (2022) 11:788755. doi: 10.3389/fonc.2021.788755
132. Haisler WL, Timm DM, Gage JA, Tseng H, Killian TC, Souza GR. Three-dimensional cell culturing by magnetic levitation. Nat Protoc. (2013) 8:1940–9. doi: 10.1038/nprot.2013.125
133. Langhans SA. Three-dimensional in vitro cell culture models in drug discovery and drug repositioning. Front Pharmacol. (2018) 9:6. doi: 10.3389/fphar.2018.00006
134. Kim SH, Lee GH, Park JY. Microwell fabrication methods and applications for cellular studies. Biomed Eng Lett. (2013) 3:131–7. doi: 10.1007/s13534-013-0105-z
135. Bae JH, Lee JM, Chung BG. Hydrogel-encapsulated 3D microwell array for neuronal differentiation. Biomed Mater. (2016) 11:15019. doi: 10.1088/1748-6041/11/1/015019
136. Kim JE, Lee JM, Chung BG. Microwell arrays for uniform-sized embryoid body-mediated endothelial cell differentiation. Biomed Microdevices. (2014) 16:559–66. doi: 10.1007/s10544-014-9858-0
137. Kang AR, Seo HI, Chung BG, Lee SH. Concave microwell array-mediated three-dimensional tumor model for screening anticancer drug-loaded nanoparticles. Nanomed Nanotechnol Biol Med. (2015) 11:1153–61. doi: 10.1016/j.nano.2015.02.009
138. Fang G, Chen YC, Lu H, Jin D. Advances in spheroids and organoids on a chip. Adv Funct Mater. (2023) 33:15043. doi: 10.1002/adfm.202215043
139. Khan I, Prabhakar A, Delepine C, Tsang H, Pham V, Sur M. A low-cost 3D printed microfluidic bioreactor and imaging chamber for live-organoid imaging. Biomicrofluidics. (2021) 15:41027. doi: 10.1063/5.0041027
140. Hughes AM, Kolb AD, Shupp AB, Shine KM, Bussard KM. Printing the pathway forward in bone metastatic cancer research: applications of 3d engineered models and bioprinted scaffolds to recapitulate the bone-tumor niche. Cancers. (2021) 13:1–28. doi: 10.3390/cancers13030507
141. Mosquera MJ, Kim S, Bareja R, Fang Z, Cai S, Pan H, et al. Extracellular matrix in synthetic hydrogel-based prostate cancer organoids regulate therapeutic response to EZH2 and DRD2 inhibitors. Adv Mater. (2022) 34:96. doi: 10.1002/adma.202100096
142. Jeong SY, Lee JH, Shin Y, Chung S, Kuh HJ. Co-culture of tumor spheroids and fibroblasts in a collagen matrix-incorporated microfluidic chip mimics reciprocal activation in solid tumor microenvironment. PLoS ONE. (2016) 11:159013. doi: 10.1371/journal.pone.0159013
143. Wang R, Zhang C, Li D, Yao Y. Tumor-on-a-chip: perfusable vascular incorporation brings new approach to tumor metastasis research and drug development. Front Bioeng Biotechnol. (2022) 10:57913. doi: 10.3389/fbioe.2022.1057913
144. Shoval H, Karsch-Bluman A, Brill-Karniely Y, Stern T, Zamir G, Hubert A, et al. Tumor cells and their crosstalk with endothelial cells in 3D spheroids. Sci Rep. (2017) 7:10699. doi: 10.1038/s41598-017-10699-y
145. Thakuri PS, Gupta M, Plaster M, Tavana H. Quantitative size-based analysis of tumor spheroids and responses to therapeutics. Assay Drug Dev Technol. (2019) 17:140–9. doi: 10.1089/adt.2018.895
146. Han SJ, Kwon S, Kim KS. Challenges of applying multicellular tumor spheroids in preclinical phase. Cancer Cell Int. (2021) 21:8. doi: 10.1186/s12935-021-01853-8
147. Fatimi A, Okoro OV, Podstawczyk D, Siminska-Stanny J, Shavandi A. Natural hydrogel-based bio-inks for 3D bioprinting in tissue engineering: a review. Gels. (2022) 8:30179. doi: 10.3390/gels8030179
148. Higginbottom SL, Tomaskovic-Crook E, Crook JM. Considerations for modelling diffuse high-grade gliomas and developing clinically relevant therapies. Cancer Metastasis Rev. (2023) 42:507–41. doi: 10.1007/s10555-023-10100-7
149. Bai L, Hao Z, Wang S, Zhou J, Yao S, Pei N, et al. Biomimetic three-dimensional glioma model printed in vitro for the studies of glioma cells and neurons interactions. Int J Bioprinting. (2023) 9:1–14. doi: 10.18063/ijb.715
150. Lee SY, Koo IS, Hwang HJ, Lee DW. In vitro three-dimensional (3D) cell culture tools for spheroid and organoid models. SLAS Discov. (2023) 28:119–37. doi: 10.1016/j.slasd.2023.03.006
151. Yamada KM, Cukierman E. Modeling tissue morphogenesis and cancer in 3D. Cell. (2007) 130:601–10. doi: 10.1016/j.cell.2007.08.006
152. Chehri B, Liu K, Vaseghi G, Seyfoori A, Akbari M. In vitro glioblastoma model on a plate for localized drug release study from a 3D-printed drug-eluted hydrogel mesh. Cells. (2024) 13:40363. doi: 10.3390/cells13040363
153. Kitaeva KV, Rutland CS, Rizvanov AA, Solovyeva VV. Cell culture based in vitro test systems for anticancer drug screening. Front Bioeng Biotechnol. (2020) 8:322. doi: 10.3389/fbioe.2020.00322
154. Poornima K, Francis AP, Hoda M, Eladl MA, Subramanian S, Veeraraghavan VP, et al. Implications of three-dimensional cell culture in cancer therapeutic research. Front Oncol. (2022) 12:891673. doi: 10.3389/fonc.2022.891673
155. Habanjar O, Diab-Assaf M, Caldefie-Chezet F, Delort L. 3D cell culture systems: tumor application, advantages, and disadvantages. Int J Mol Sci. (2021) 22:12200. doi: 10.3390/ijms222212200
156. Colombo E, Cattaneo MG. Multicellular 3D models to study tumour-stroma interactions. Int J Mol Sci. (2021) 22:1–19. doi: 10.3390/ijms22041633
157. Jensen C, Teng Y. Is it time to start transitioning from 2D to 3D cell culture? Front Mol Biosci. (2020) 7:33. doi: 10.3389/fmolb.2020.00033
158. Alzeeb G, Metges JP, Corcos L, Le Jossic-Corcos C. Three-dimensional culture systems in gastric cancer research. Cancers. (2020) 12:1–20. doi: 10.3390/cancers12102800
159. Klein E, Hau AC, Oudin A, Golebiewska A, Niclou SP. Glioblastoma organoids: pre-clinical applications and challenges in the context of immunotherapy. Front Oncol. (2020) 10:4121. doi: 10.3389/fonc.2020.604121
160. Farhat J, Pandey I, Al-wahsh M. Transcending toward advanced 3d-cell culture modalities: a review about an emerging paradigm in translational oncology. Cells. (2021) 10:71657. doi: 10.3390/cells10071657
161. Caragher S, Chalmers AJ, Gomez-Roman N. Glioblastoma's next top model: novel culture systems for brain cancer radiotherapy research. Cancers. (2019) 11:10044. doi: 10.3390/cancers11010044
162. Paolillo M, Comincini S, Schinelli S. In vitro glioblastoma models: a journey into the third dimension. Cancers. (2021) 13:102449. doi: 10.3390/cancers13102449
163. Fong ELS, Harrington DA, Farach-Carson MC, Yu H. Heralding a new paradigm in 3D tumor modeling. Biomaterials. (2016) 108:197–213. doi: 10.1016/j.biomaterials.2016.08.052
164. Kohl Y, Biehl M, Spring S, Hesler M, Ogourtsov V, Todorovic M, et al. Microfluidic in vitro platform for (nano)safety and (nano)drug efficiency screening. Small. (2021) 17:6012. doi: 10.1002/smll.202006012
165. Robertson-Tessi M, Gillies RJ, Gatenby RA, Anderson ARA. Impact of metabolic heterogeneity on tumor growth, invasion, and treatment outcomes. Cancer Res. (2015) 75:1567–79. doi: 10.1158/0008-5472.CAN-14-1428
166. Sivakumar R, Chan M, Shin JS, Nishida-Aoki N, Kenerson HL, Elemento O, et al. Organotypic tumor slice cultures provide a versatile platform for immuno-oncology and drug discovery. Oncoimmunology. (2019) 8:1670019. doi: 10.1080/2162402X.2019.1670019
167. Rodriguez AD, Horowitz LF, Castro K, Kenerson H, Bhattacharjee N, Gandhe G, et al. A microfluidic platform for functional testing of cancer drugs on intact tumor slices. Lab Chip. (2020) 20:1658–75. doi: 10.1039/C9LC00811J
168. Surendran V, Rutledge D, Colmon R, Chandrasekaran A. A novel tumor-immune microenvironment (TIME)-on-Chip mimics three dimensional neutrophil-tumor dynamics and neutrophil extracellular traps (NETs)-mediated collective tumor invasion. Biofabrication. (2021) 13:abe1cf. doi: 10.1088/1758-5090/abe1cf
169. Amereh M, Seyfoori A, Dallinger B, Azimzadeh M, Stefanek E, Akbari M. 3D-printed tumor-on-a-chip model for investigating the effect of matrix stiffness on glioblastoma tumor invasion. Biomimetics. (2023) 8:50421. doi: 10.3390/biomimetics8050421
170. Dorrigiv D, Simeone K, Communal L, Kendall-Dupont J, St-Georges-robillard A, Péant B, et al. Microdissected tissue vs tissue slices-a comparative study of tumor explant models cultured on-chip and off-chip. Cancers. (2021) 13:164208. doi: 10.3390/cancers13164208
171. Mazzocchi AR, Rajan SAP, Votanopoulos KI, Hall AR, Skardal A. In vitro patient-derived 3D mesothelioma tumor organoids facilitate patient-centric therapeutic screening. Sci Rep. (2018) 8:8. doi: 10.1038/s41598-018-21200-8
172. Lopez-Muñoz GA, Mugal S, Ramón-Azcón J. Sensors and biosensors in organs-on-a-chip platforms. Adv Exp Med Biol. (2022) 1379:55–80. doi: 10.1007/978-3-031-04039-9_3
173. Zhou L, Liu L, Chang MA, Ma C, Chen W, Chen P. Spatiotemporal dissection of tumor microenvironment via in situ sensing and monitoring in tumor-on-a-chip. Biosens Bioelectron. (2023) 225:115064. doi: 10.1016/j.bios.2023.115064
174. Kim J, Kim J, Jin Y, Cho SW. In situ biosensing technologies for an organ-on-a-chip. Biofabrication. (2023) 15:aceaae. doi: 10.1088/1758-5090/aceaae
175. Zhang YS, Aleman J, Shin SR, Kilic T, Kim D, Shaegh SAM, et al. Multisensor-integrated organs-on-chips platform for automated and continual in situ monitoring of organoid behaviors. Proc Natl Acad Sci USA. (2017) 114:E2293–302. doi: 10.1073/pnas.1612906114
176. Naresh V, Lee N. A review on biosensors and recent development of nanostructured materials-enabled biosensors. Sensors. (2021) 21:1–35. doi: 10.3390/s21041109
177. Khan MS, Tariq MO, Nawaz M, Ahmed J. MEMS sensors for diagnostics and treatment in the fight against COVID-19 and other pandemics. IEEE Access. (2021) 9:61123–49. doi: 10.1109/ACCESS.2021.3073958
178. Tran AV, Zhang X, Zhu B. Mechanical structural design of a piezoresistive pressure sensor for low-pressure measurement: a computational analysis by increases in the sensor sensitivity. Sensors. (2018) 18:72023. doi: 10.3390/s18072023
179. Yu Z, Zhao Y, Li C, Liu Y, Zhou G, Tian B. A micro-pressure sensor with high sensitivity and overload resistance. Proc IEEE Sensors. (2013) 1–4. doi: 10.1109/ICSENS.2013.6688392
180. Xu T, Zhao L, Jiang Z, Xu Y, Zhao Y. Modeling and analysis of a novel combined peninsula-island structure diaphragm for ultra-low pressure sensing with high sensitivity. J Phys D Appl Phys. (2016) 49:75110. doi: 10.1088/0022-3727/49/7/075110
181. Xu T, Lu D, Zhao L, Jiang Z, Wang H, Guo X, et al. Application and optimization of stiffness abruption structures for pressure sensors with high sensitivity and anti-overload ability. Sensors. (2017) 17:91965. doi: 10.3390/s17091965
182. Rakhshani MR. Refractive index sensor based on dual side-coupled rectangular resonators and nanorods array for medical applications. Opt Quantum Electron. (2021) 53:4. doi: 10.1007/s11082-021-02857-4
183. Khonina SN, Kazanskiy NL, Butt MA, Kazmierczak A, Piramidowicz R. Plasmonic sensor based on metal-insulator-metal waveguide square ring cavity filled with functional material for the detection of CO2 gas. Opt Express. (2021) 29:16584. doi: 10.1364/OE.423141
184. Karimi Y, Kaatuzian H, Tooghi A, Danaie M. All-optical plasmonic switches based on Fano resonance in an X-shaped resonator coupled to parallel stubs for telecommunication applications. Optik. (2021) 243:167424. doi: 10.1016/j.ijleo.2021.167424
185. Wang X, Zhou J, Wang H. Bioreceptors as the key components for electrochemical biosensing in medicine. Cell Reports Phys Sci. (2024) 5:101801. doi: 10.1016/j.xcrp.2024.101801
186. Oliveira M, Conceição P, Kant K, Ainla A, Diéguez L. Electrochemical sensing in 3D cell culture models: new tools for developing better cancer diagnostics and treatments. Cancers. (2021) 13:1–21. doi: 10.3390/cancers13061381
187. Sitkov N, Ryabko A, Moshnikov V, Aleshin A, Kaplun D, Zimina T. Hybrid impedimetric biosensors for express protein markers detection. Micromachines. (2024) 15:20181. doi: 10.3390/mi15020181
188. Lei L, Ma B, Xu C, Liu H. Emerging tumor-on-chips with electrochemical biosensors. Trends Anal Chem. (2022) 153:116640. doi: 10.1016/j.trac.2022.116640
189. Nguyen TNH, Horowitz L, Krilov T, Lockhart E, Kenerson HL, Yeung RS, et al. Label-free, real-time monitoring of cytochrome c responses to drugs in microdissected tumor biopsies with a multi-well aptasensor platform. bioRxiv. (2024) 2024:578278. doi: 10.1101/2024.01.31.578278
190. Shin H, Jeong S, Lee JH, Sun W, Choi N, Cho IJ. 3D high-density microelectrode array with optical stimulation and drug delivery for investigating neural circuit dynamics. Nat Commun. (2021) 12:3. doi: 10.1038/s41467-020-20763-3
191. Ji X, Lin X, Rivnay J. Organic electrochemical transistors as on-site signal amplifiers for electrochemical aptamer-based sensing. Nat Commun. (2023) 14:2. doi: 10.1038/s41467-023-37402-2
192. Su SH, Song Y, Stephens A, Situ M, McCloskey MC, McGrath JL, et al. A tissue chip with integrated digital immunosensors: in situ brain endothelial barrier cytokine secretion monitoring. Biosens Bioelectron. (2023) 224:115030. doi: 10.1016/j.bios.2022.115030
193. Yang W, Li T, Liao S, Zhou J, Huang L. Organ-on-a-chip platforms integrated with biosensors for precise monitoring of the cells and cellular microenvironment. Trends Anal Chem. (2024) 172:117569. doi: 10.1016/j.trac.2024.117569
194. Calejo I, Heinrich MA, Zambito G, Mezzanotte L, Prakash J, Moreira Teixeira L. Advancing tumor microenvironment research by combining organs-on-chips and biosensors. Adv Exp Med Biol. (2022) 1379:171–203. doi: 10.1007/978-3-031-04039-9_7
195. Fuchs S, Johansson S, Tjell A, Werr G, Mayr T, Tenje M. In-line analysis of organ-on-chip systems with sensors: integration, fabrication, challenges, and potential. ACS Biomater Sci Eng. (2021) 7:2926–48. doi: 10.1021/acsbiomaterials.0c01110
196. Petrovszki D, Walter FR, Vigh JP, Kocsis A, Valkai S, Deli MA, et al. Penetration of the SARS-CoV-2 spike protein across the blood-brain barrier, as revealed by a combination of a human cell culture model system and optical biosensing. Biomedicines. (2022) 10:10188. doi: 10.3390/biomedicines10010188
197. Nguyen H-T, Rissanen S-L, Peltokangas N, Laakkonen T, Kettunen J, Barthod L, et al. Highly scalable and standardized organ-on-chip platform with TEER for biological barrier modeling. Tissue Barriers. (2024) 2024:2315702. doi: 10.1080/21688370.2024.2315702
198. Tawade P, Mastrangeli M. Integrated electrochemical and optical biosensing in organs-on-chip. ChemBioChem. (2024) 25:560. doi: 10.1002/cbic.202300560
199. Aydogmus H, Hu M, Ivancevic L, Frimat JP, van den Maagdenberg AMJM, Sarro PM, et al. An organ-on-chip device with integrated charge sensors and recording microelectrodes. Sci Rep. (2023) 13:5. doi: 10.1038/s41598-023-34786-5
200. Zhu Y, Mandal K, Hernandez AL, Kawakita S, Huang W, Bandaru P, et al. State of the art in integrated biosensors for organ-on-a-chip applications. Curr Opin Biomed Eng. (2021) 19:100309. doi: 10.1016/j.cobme.2021.100309
201. Alavijeh AA, Barati M, Barati M, Dehkordi HA. The potential of magnetic nanoparticles for diagnosis and treatment of cancer based on body magnetic field and organ-on-the-chip. Adv Pharm Bull. (2019) 9:360–73. doi: 10.15171/apb.2019.043
202. Öztatli H, Altintas Z, Garipcan B. Biosensors for organs-on-a-chip and organoids. Adv Sens Technol Biomed Environ Constr Appl. (2022) 2022:471–514. doi: 10.1016/B978-0-323-90222-9.00007-8
203. Ferrari E, Palma C, Vesentini S, Occhetta P, Rasponi M. Integrating biosensors in organs-on-chip devices: a perspective on current strategies to monitor microphysiological systems. Biosensors. (2020) 10:110. doi: 10.3390/bios10090110
204. Parihar P, Sunildutt N, Rahim CSA, Samantasinghar A, Lim JH, Choi KH. An overview of advancements and technologies in vascularization strategies for tumor-on-a-chip models. Adv Ther. (2024) 2024:410. doi: 10.1002/adtp.202300410
205. Jiang C, Mogilevsky C, Belal Z, Kurtz G, Alonso-Basanta M. Hypofractionation in glioblastoma: an overview of palliative, definitive, and exploratory uses. Cancers. (2023) 15:235650. doi: 10.3390/cancers15235650
206. Pérez-Gutiérrez L, Ferrara N. Biology and therapeutic targeting of vascular endothelial growth factor A. Nat Rev Mol Cell Biol. (2023) 24:816–34. doi: 10.1038/s41580-023-00631-w
207. Musafargani S, Mishra S, Gulyás M, Mahalakshmi P, Archunan G, Padmanabhan P, et al. Blood brain barrier: a tissue engineered microfluidic chip. J Neurosci Methods. (2020) 331:108525. doi: 10.1016/j.jneumeth.2019.108525
208. Jain RK, Di Tomaso E, Duda DG, Loeffler JS, Sorensen AG, Batchelor TT. Angiogenesis in brain tumours. Nat Rev Neurosci. (2007) 8:610–22. doi: 10.1038/nrn2175
209. Ma YHV, Middleton K, You L, Sun Y. A review of microfluidic approaches for investigating cancer extravasation during metastasis. Microsystems Nanoeng. (2018) 4:104. doi: 10.1038/micronano.2017.104
210. Cho H, Kim J, Song H, Sohn KY, Jeon M, Han KH. Microfluidic technologies for circulating tumor cell isolation. Analyst. (2018) 143:2936–70. doi: 10.1039/C7AN01979C
211. Xia Y, Wan Y, Hao S, Nisic M, Harouaka RA, Chen Y, et al. Nucleus of circulating tumor cell determines its translocation through biomimetic microconstrictions and its physical enrichment by microfiltration. Small. (2018) 14:2899. doi: 10.1002/smll.201802899
212. Chen MB, Hajal C, Benjamin DC, Yu C, Azizgolshani H, Hynes RO, et al. Inflamed neutrophils sequestered at entrapped tumor cells via chemotactic confinement promote tumor cell extravasation. Proc Natl Acad Sci USA. (2018) 115:7022–7. doi: 10.1073/pnas.1715932115
213. Ayuso JM, Virumbrales-Munoz M, McMinn PH, Rehman S, Gomez I, Karim MR, et al. Tumor-on-A-chip: a microfluidic model to study cell response to environmental gradients. Lab Chip. (2019) 19:3461–71. doi: 10.1039/C9LC00270G
214. Farrar CT, Kamoun WS, Ley CD, Kim YR, Kwon SJ Dai G, Rosen BR, et al. In vivo validation of MRI vessel caliber index measurement methods with intravital optical microscopy in a U87 mouse brain tumor model. Neuro Oncol. (2010) 12:341–50. doi: 10.1093/neuonc/nop032
215. Dalkara T, Gursoy-Ozdemir Y, Yemisci M. Brain microvascular pericytes in health and disease. Acta Neuropathol. (2011) 122:1–9. doi: 10.1007/s00401-011-0847-6
216. Kim J, Chung M, Kim S, Jo DH, Kim JH, Jeon NL. Engineering of a biomimetic pericyte-covered 3D microvascular network. PLoS ONE. (2015) 10:133880. doi: 10.1371/journal.pone.0133880
217. Nothdurfter D, Ploner C, Coraça-Huber DC, Wilflingseder D, Müller T, Hermann M, et al. 3D bioprinted, vascularized neuroblastoma tumor environment in fluidic chip devices for precision medicine drug testing. Biofabrication. (2022) 14:acfb7. doi: 10.1088/1758-5090/ac5fb7
218. Vega FM, Colmenero-Repiso A, Gómez-Muñoz MA, Rodríguez-Prieto I, Aguilar-Morante D, Ramírez G, et al. CD44-high neural crest stem-like cells are associated with tumour aggressiveness and poor survival in neuroblastoma tumours. EBioMedicine. (2019) 49:82–95. doi: 10.1016/j.ebiom.2019.10.041
219. Rice O, Surian A, Chen Y. Modeling the blood-brain barrier for treatment of central nervous system (CNS) diseases. J Tissue Eng. (2022) 13:97. doi: 10.1177/20417314221095997
220. Wolff A, Antfolk M, Brodin B, Tenje M. In vitro blood-brain barrier models - an overview of established models and new microfluidic approaches. J Pharm Sci. (2015) 104:2727–46. doi: 10.1002/jps.24329
221. Chaulagain B, Gothwal A, Lamptey RNL, Trivedi R, Mahanta AK, Layek B, et al. Experimental models of in vitro blood-brain barrier for CNS drug delivery: an evolutionary perspective. Int J Mol Sci. (2023) 24:32710. doi: 10.3390/ijms24032710
222. Oddo A, Peng B, Tong Z, Wei Y, Tong WY, Thissen H, et al. Advances in microfluidic blood-brain barrier (BBB) models. Trends Biotechnol. (2019) 37:1295–314. doi: 10.1016/j.tibtech.2019.04.006
223. Neuhaus W. In vitro models of the blood-brain barrier. In:Stone N, , editor. Handbook of Experimental Pharmacology. Berlin: Springer Science and Business Media Deutschland GmbH (2021). p. 75–110.
224. Neves ER, Harley BAC, Pedron S. Microphysiological systems to study tumor-stroma interactions in brain cancer. Brain Res Bull. (2021) 174:220–9. doi: 10.1016/j.brainresbull.2021.06.012
225. Ruck T, Bittner S, Meuth SG. Blood-brain barrier modeling: challenges and perspectives. Neural Regen Res. (2015) 10:889–91. doi: 10.4103/1673-5374.158342
226. Singh S, Agrawal M, Vashist R, Patel RK, Sangave SD, Alexander A. Recent advancements on in vitro blood-brain barrier model: a reliable and efficient screening approach for preclinical and clinical investigation. Expert Opin Drug Deliv. (2023) 20:1839–57. doi: 10.1080/17425247.2023.2295940
227. Wilhelm I, Krizbai IA. In vitro models of the blood-brain barrier for the study of drug delivery to the brain. Mol Pharm. (2014) 11:1949–63. doi: 10.1021/mp500046f
228. Linville RM, Maressa J, Guo Z, Chung TD, Farrell A, Jha R, et al. A tissue-engineered model of the blood-tumor barrier during metastatic breast cancer. Fluids Barriers CNS. (2023) 20:1–17. doi: 10.1186/s12987-023-00482-9
229. Gastfriend BD, Palecek SP, Shusta E V. Modeling the blood-brain barrier: beyond the endothelial cells. Curr Opin Biomed Eng. (2018) 5:6–12. doi: 10.1016/j.cobme.2017.11.002
230. Campisi M, Lim SH, Chiono V, Kamm RD. 3D self-organized human blood-brain barrier in a microfluidic chip. In:Ebrahimkhani MR, Hislop J, , editors. Programmed Morphogenesis. Methods in Molecular Biology, Vol. 2258. New York, NY: Humana (2021). doi: 10.1007/978-1-0716-1174-6_14
231. Piantino M, Figarol A, Matsusaki M. Three-dimensional in vitro models of healthy and tumor brain microvasculature for drug and toxicity screening. Front Toxicol. (2021) 3:1–18. doi: 10.3389/ftox.2021.656254
233. Amirifar L, Shamloo A, Nasiri R, de Barros NR, Wang ZZ, Unluturk BD, et al. Brain-on-a-chip: recent advances in design and techniques for microfluidic models of the brain in health and disease. Biomaterials. (2022) 285:121531. doi: 10.1016/j.biomaterials.2022.121531
234. Guarino V, Zizzari A, Bianco M, Gigli G, Moroni L, Arima V. Advancements in modelling human blood brain-barrier on a chip. Biofabrication. (2023) 15:acb571. doi: 10.1088/1758-5090/acb571
235. Jiang L, Li S, Zheng J, Li Y, Huang H. Recent progress in microfluidic models of the blood-brain barrier. Micromachines. (2019) 10:1–20. doi: 10.3390/mi10060375
236. Wang X, Hou Y, Ai X, Sun J, Xu B, Meng X, et al. Potential applications of microfluidics based blood brain barrier (BBB)-on-chips for in vitro drug development. Biomed Pharmacother. (2020) 132:110822. doi: 10.1016/j.biopha.2020.110822
237. Sharma A, Fernandes DC, Reis RL, Gołubczyk D, Neumann S, Lukomska B, et al. Cutting-edge advances in modeling the blood-brain barrier and tools for its reversible permeabilization for enhanced drug delivery into the brain. Cell Biosci. (2023) 13:1–21. doi: 10.1186/s13578-023-01079-3
238. He Y, Yao Y, Tsirka SE, Cao Y. Cell-culture models of the blood-brain barrier. Stroke. (2014) 45:2514–26. doi: 10.1161/STROKEAHA.114.005427
239. Peng B, Hao S, Tong Z, Bai H, Pan S, Lim KL, et al. Blood-brain barrier (BBB)-on-a-chip: a promising breakthrough in brain disease research. Lab Chip. (2022) 22:3579–602. doi: 10.1039/D2LC00305H
240. Floryanzia SD, Nance E. Applications and considerations for microfluidic systems to model the blood-brain barrier. ACS Appl Bio Mater. (2023) 6:3617–32. doi: 10.1021/acsabm.3c00364
241. Reshma S, Megha KB, Amir S, Rukhiya S, Mohanan PV. Blood brain barrier-on-a-chip to model neurological diseases. J Drug Deliv Sci Technol. (2023) 80:104174. doi: 10.1016/j.jddst.2023.104174
242. Cui B, Cho SW. Blood-brain barrier-on-a-chip for brain disease modeling and drug testing. BMB Rep. (2022) 55:213–9. doi: 10.5483/BMBRep.2022.55.5.043
243. Iakovlev AP, Erofeev AS, Gorelkin PV. Novel pumping methods for microfluidic devices: a comprehensive review. Biosensors. 12:956. doi: 10.3390/bios12110956
244. Jagtiani E, Yeolekar M, Naik S, Patravale V. In vitro blood brain barrier models: an overview. J Control Release. (2022) 343:13–30. doi: 10.1016/j.jconrel.2022.01.011
245. Williams-Medina A, Deblock M, Janigro D. In vitro models of the blood-brain barrier: tools in translational medicine. Front Med Technol. (2020) 2:1–20. doi: 10.3389/fmedt.2020.623950
246. Gonzales-Aloy E, Ahmed-Cox A, Tsoli M, Ziegler DS, Kavallaris M. From cells to organoids: the evolution of blood-brain barrier technology for modelling drug delivery in brain cancer. Adv Drug Deliv Rev. (2023) 196:114777. doi: 10.1016/j.addr.2023.114777
247. Yu X, Ji C, Shao A. Neurovascular unit dysfunction and neurodegenerative disorders. Front Neurosci. (2020) 14:334. doi: 10.3389/fnins.2020.00334
248. Łach A, Wnuk A, Wójtowicz AK. Experimental models to study the functions of the blood-brain barrier. Bioengineering. (2023) 10:50519. doi: 10.3390/bioengineering10050519
249. Kaisar MA, Sajja RK, Prasad S, Abhyankar VV, Liles T, Cucullo L. New experimental models of the blood-brain barrier for CNS drug discovery. Expert Opin Drug Discov. (2017) 12:89–103. doi: 10.1080/17460441.2017.1253676
250. Sivandzade F, Cucullo L. In-vitro blood-brain barrier modeling: a review of modern and fast-advancing technologies. J Cereb Blood Flow Metab. (2018) 38:1667–81. doi: 10.1177/0271678X18788769
251. Augustine R, Aqel AH, Kalva SN, Joshy KS, Nayeem A, Hasan A. Bioengineered microfluidic blood-brain barrier models in oncology research. Transl Oncol. (2021) 14:101087. doi: 10.1016/j.tranon.2021.101087
252. Prashanth A, Donaghy H, Stoner SP, Hudson AL, Wheeler HR, Diakos CI, et al. Are in vitro human blood-brain-tumor-barriers suitable replacements for in vivo models of brain permeability for novel therapeutics? Cancers. (2021) 13:1–20. doi: 10.3390/cancers13050955
253. Hajal C, Offeddu GS, Shin Y, Zhang S, Morozova O, Hickman D, et al. Engineered Human Blood-Brain Barrier Microfluidic Model for Vascular Permeability Analyses. Berlin: Springer US (2022). p. 95–128.
254. Pérez-López A, Torres-Suárez AI, Martín-Sabroso C, Aparicio-Blanco J. An overview of in vitro 3D models of the blood-brain barrier as a tool to predict the in vivo permeability of nanomedicines. Adv Drug Deliv Rev. (2023) 196:114816. doi: 10.1016/j.addr.2023.114816
255. Morris EK, Daignault-Mill S, Stehbens SJ, Genovesi LA, Lagendijk AK. Addressing blood-brain-tumor-barrier heterogeneity in pediatric brain tumors with innovative preclinical models. Front Oncol. (2023) 13:1–9. doi: 10.3389/fonc.2023.1101522
256. Eilenberger C, Rothbauer M, Selinger F, Gerhartl A, Jordan C, Harasek M, et al. A microfluidic multisize spheroid array for multiparametric screening of anticancer drugs and blood-brain barrier transport properties. Adv Sci. (2021) 8:1–20. doi: 10.1002/advs.202004856
257. Jacak J, Buchroithner B, Mayr S, Hauser F, Priglinger E, Stangl H, et al. Dual channel microfluidics for mimicking the blood-brain barrier. ACS Nano. (2021) 15:2984–93. doi: 10.1021/acsnano.0c09263
258. Long RKM, Piatti L, Korbmacher F, Bernabeu M. Understanding parasite-brain microvascular interactions with engineered 3D blood-brain barrier models. Mol Microbiol. (2022) 117:693–704. doi: 10.1111/mmi.14852
259. Kim J, Lee KT, Lee JS, Shin J, Cui B, Yang K, et al. Fungal brain infection modelled in a human-neurovascular-unit-on-a-chip with a functional blood-brain barrier. Nat Biomed Eng. (2021) 5:830–46. doi: 10.1038/s41551-021-00743-8
260. Duong DD, Kwak J, Song HS, Lee NY. Construction of microfluidic blood-brain barrier model assisted by 3D coculture on cellulose fiber. Microsyst Technol. (2021) 27:3917–26. doi: 10.1007/s00542-020-05197-7
261. Vakilian S, Alam K, Al-Kindi J, Jamshidi-Adegani F, Rehman NU, Tavakoli R, et al. An engineered microfluidic blood-brain barrier model to evaluate the anti-metastatic activity of β-boswellic acid. Biotechnol J. (2021) 16:44. doi: 10.1002/biot.202100044
262. Harding IC, O'Hare NR, Vigliotti M, Caraballo A, Lee CI, Millican K, et al. Developing a transwell millifluidic device for studying blood-brain barrier endothelium. Lab Chip. (2022) 18:657J. doi: 10.1039/D2LC00657J
263. Silvani G, Basirun C, Wu H, Mehner C, Poole K, Bradbury P, et al. A 3D-bioprinted vascularized glioblastoma-on-a-chip for studying the impact of simulated microgravity as a novel pre-clinical approach in brain tumor therapy. Adv Ther. (2021) 4:106. doi: 10.1002/adtp.202100106
264. Seo S, Nah SY, Lee K, Choi N, Kim HN. Triculture model of in vitro BBB and its application to study BBB-associated chemosensitivity and drug delivery in glioblastoma. Adv Funct Mater. (2022) 32:6860. doi: 10.1002/adfm.202106860
265. Tang M, Rich JN, Chen S. Biomaterials and 3D bioprinting strategies to model glioblastoma and the blood-brain barrier. Adv Mater. (2021) 33:1–25. doi: 10.1002/adma.202004776
266. Straehla JP, Hajal C, Safford HC, Offeddu GS, Boehnke N, Dacoba TG, et al. A predictive microfluidic model of human glioblastoma to assess trafficking of blood-brain barrier-penetrant nanoparticles. Proc Natl Acad Sci USA. (2022) 119:2118697. doi: 10.1073/pnas.2118697119
267. Nair AL, Mesch L, Schulz I, Becker H, Raible J, Kiessling H, et al. Parallelizable microfluidic platform to model and assess in vitro cellular barriers: technology and application to study the interaction of 3D tumor spheroids with cellular barriers. Biosensors. (2021) 11:90314. doi: 10.3390/bios11090314
268. Hajal C, Le Roi B, Kamm RD, Maoz BM. Biology and models of the blood-brain barrier. Annu Rev Biomed Eng. (2021) 23:359–84. doi: 10.1146/annurev-bioeng-082120-042814
269. Meena M, Vandormael R, De Laere M, Pintelon I, Berneman Z, Watts R, et al. A microfluidic in vitro three-dimensional dynamic model of the blood-brain barrier to study the transmigration of immune cells. Brain Sci. (2022) 12:101293. doi: 10.3390/brainsci12101293
270. Martins AM, Brito A, Barbato MG, Felici A, Reis RL, Pires RA, et al. Efficacy of molecular and nano-therapies on brain tumor models in microfluidic devices. Biomater Adv. (2023) 144:213227. doi: 10.1016/j.bioadv.2022.213227
271. Lam MSY, Aw JJY, Tan D, Vijayakumar R, Lim HYG, Yada S, et al. Unveiling the influence of tumor microenvironment and spatial heterogeneity on temozolomide resistance in glioblastoma using an advanced human in vitro model of the blood-brain barrier and glioblastoma. Small. (2023) 2302280:1–16. doi: 10.1002/smll.202302280
272. Salvador E, Köppl T, Hörmann J, Schönhärl S, Bugaeva P, Kessler AF, et al. Tumor treating fields (TTFields) induce cell junction alterations in a human 3D in vitro model of the blood-brain barrier. Pharmaceutics. (2023) 15:10185. doi: 10.3390/pharmaceutics15010185
273. Shi Y, He X, Wang H, Dai J, Fang J, He Y, et al. Construction of a novel blood brain barrier-glioma microfluidic chip model: applications in the evaluation of permeability and anti-glioma activity of traditional Chinese medicine components. Talanta. (2023) 253:123971. doi: 10.1016/j.talanta.2022.123971
274. Westerhof TM, Yang BA, Merrill NM, Yates JA, Altemus M, Russell L, et al. Blood-brain barrier remodeling in an organ-on-a-chip device showing Dkk1 to be a regulator of early metastasis. Adv NanoBiomed Res. (2023) 3:1–18. doi: 10.1002/anbr.202200036
275. Janjua TI, Cao Y, Ahmed-Cox A, Raza A, Moniruzzaman M, Akhter DT, et al. Efficient delivery of Temozolomide using ultrasmall large-pore silica nanoparticles for glioblastoma. J Control Release. (2023) 357:161–74. doi: 10.1016/j.jconrel.2023.03.040
276. Santillán-cortez D, Castell-rodríguez AE, González-arenas A. Optimizing cell inoculation in a microfluidic system in order to mimic the hemato-encephalic barrier and tumor dynamic cell interaction. Preprints. (2024) 2024: 2024010547. doi: 10.20944/preprints202401.0547.v1
Keywords: brain tumor, tumor-on-a-chip, blood-brain barrier, organ-on-a-chip, microfluidic devices
Citation: Zimina TM, Sitkov NO, Gareev KG, Mikhailova NV, Combs SE and Shevtsov MA (2024) Hybrid-integrated devices for mimicking malignant brain tumors (“tumor-on-a-chip”) for in vitro development of targeted drug delivery and personalized therapy approaches. Front. Med. 11:1452298. doi: 10.3389/fmed.2024.1452298
Received: 20 June 2024; Accepted: 14 October 2024;
Published: 19 November 2024.
Edited by:
Udhaya Kumar, Baylor College of Medicine, United StatesReviewed by:
Chenguang Zhang, Sanofi Genzyme, United StatesCopyright © 2024 Zimina, Sitkov, Gareev, Mikhailova, Combs and Shevtsov. This is an open-access article distributed under the terms of the Creative Commons Attribution License (CC BY). The use, distribution or reproduction in other forums is permitted, provided the original author(s) and the copyright owner(s) are credited and that the original publication in this journal is cited, in accordance with accepted academic practice. No use, distribution or reproduction is permitted which does not comply with these terms.
*Correspondence: Nikita O. Sitkov, c2l0a292OTNAeWFuZGV4LnJ1
Disclaimer: All claims expressed in this article are solely those of the authors and do not necessarily represent those of their affiliated organizations, or those of the publisher, the editors and the reviewers. Any product that may be evaluated in this article or claim that may be made by its manufacturer is not guaranteed or endorsed by the publisher.
Research integrity at Frontiers
Learn more about the work of our research integrity team to safeguard the quality of each article we publish.