- 1OrphanDEV FCRIN Reference Network, Aix Marseille Univ, APHM, INSERM, Inst Neurosci Syst, CHU Timone, Marseille, France
- 2Thelonius Mind, Marseille, France
- 3MyDisease2Ez, Fresnes, France
Drug repurposing, the process of identifying new uses for existing drugs beyond their original indications, offers significant advantages in terms of reduced development time and costs, particularly in addressing unmet medical needs in rare diseases. Artificial intelligence (AI) has emerged as a transformative force in healthcare, and by leveraging AI technologies, researchers aim to overcome some of the challenges associated with rare diseases. This review presents concrete case studies, as well as pre-existing platforms, initiatives, and companies that demonstrate the application of AI for drug repurposing in rare diseases. Despite representing a modest part of the literature compared to other diseases such as COVID-19 or cancer, the growing interest, and investment in AI for drug repurposing in rare diseases underscore its potential to accelerate treatment availability for patients with unmet medical needs.
1 Introduction
Drug repurposing is defined as the process of identifying a new use for an existing drug or active substance in an indication outside the scope of the original indication. It represents important advantages, including reduced development time and costs and potentially large societal healthcare cost savings. Drug repurposing represents a significant innovative option for therapies for rare diseases. Indeed, among the over 7,000 identified rare diseases affecting 4% of the worldwide population, less than 6% of them have an approved treatment option (1, 2). Because of the factors inherent to rare diseases (i.e., limited patient populations, disease complexity, and lack of understanding), the development of new therapies is challenging. In rare diseases, the drug repurposing process results in a reduction of the risk of drug development, and, ultimately, in the acceleration of the available treatment options for patients (3).
Concurrently, artificial intelligence (AI)—including techniques such as machine learning (ML), deep learning (DL), or even natural language processing (NLP) (4)1, (5)2—has emerged as a transformative force in the domain of healthcare, offering unprecedented opportunities for drug discovery, virtual clinical consultation, disease diagnosis, prognosis, medication management, health monitoring, genomic medicine, or even patient care (6, 7). In the context of rare diseases, where data are often scarce and heterogenous, the ability of AI technologies to integrate and analyze data from diverse sources, including electronic health records, genomic data, biomedical literature, or patient registries, can be used to overcome the challenges associated with rare diseases (8).
Ultimately, the synergy between AI and drug repurposing has therefore been defined as holding promises for addressing the unmet medical needs in rare diseases (9, 10). In this review, we provide an overview of the current landscape of AI drug repurposing in rare diseases, showcasing concrete case studies from the literature and highlighting existing platforms, libraries, funded initiatives, and companies at the forefront of this emerging field.
2 Methods
2.1 Literature research
Literature research was performed on the MEDLINE database, using a combination of MeSH terms designed to maximize the retrieval of relevant results aligned with the objectives of the review. The following combination was used: [(artificial intelligence) OR (machine learning) OR (deep learning) OR (natural language processing)] AND [(drug repurposing) OR (drug repositioning)].
All the literature references were then extracted (11 March 2024) under the PubMed format. This format, which replaced the RIS format, allows saving citations as a text or.nbib file matching the MEDLINE format (11).
For further categorization of the retrieved literature, particularly regarding the diseases addressed by the references, we established a list of keywords (as presented in Table 1) for each disease detected in the references. These keywords were selected based on (1) their relevance to the disease category and on (2) their ability to categorize a high proportion of relevant references. Subsequently, a custom Python script was used to match these specified keywords with the abstracts of the literature references retrieved from the initial search. This approach allowed us to categorize the references according to the diseases they address.
2.2 Exploring AI-driven initiatives: beyond scientific literature
In tandem with our literature review, we investigated the existent initiatives leveraging AI tools for drug repurposing in rare diseases. This exploration encompassed two integrated and more general platforms: Google and LinkedIn. We selected all the existent initiatives (companies, open-source or private platforms, libraries, etc.) that explicitly mention using AI tools for drug repurposing in rare diseases.
3 Results
Through the literature research, we have been able to collect a total of 1,090 bibliographic references relating to the use of AI for drug repurposing. Among them, we were able to categorize a total of 677 references according to the diseases they address (62.11%). A total of 18 disease categories were identified (Table 1). The distribution of the different categories is presented in Figures 1A,B.
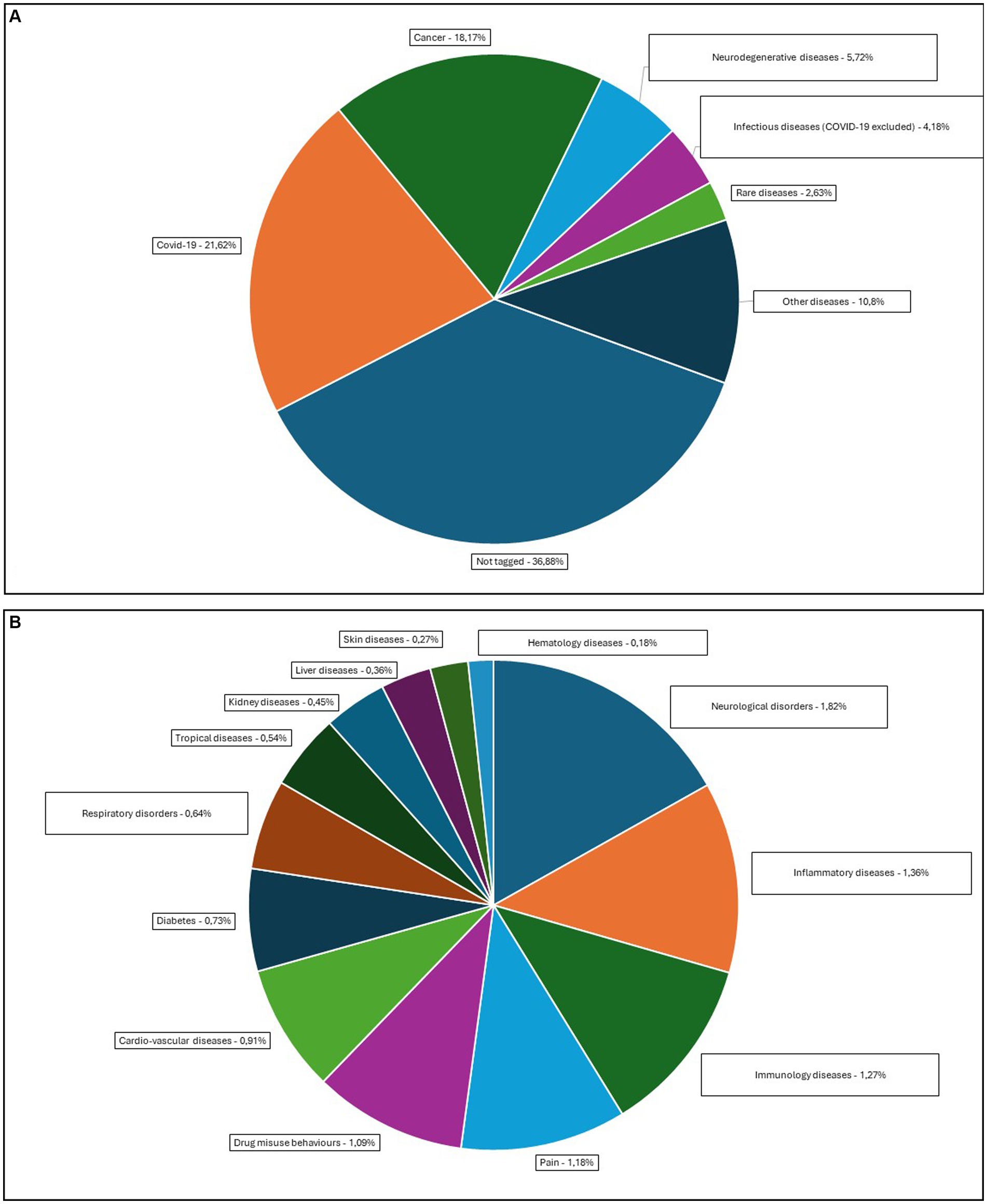
Figure 1. Overview of the diseases targeted by AI applications in drug repurposing. (A) Overview of the diseases representing more than 2% of the retrieved references: COVID-19, cancer, neurodegenerative diseases, infectious diseases, rare diseases, and other diseases (diseases representing less than 2% of the retrieved references) and not tagged. (B) Overview of the diseases representing less than 2% of the retrieved references: neurological disorders, inflammatory diseases, immunology diseases, pain, drug misuse behaviors, cardiovascular diseases, diabetes, respiratory disorders, tropical diseases, kidney diseases, liver diseases, and skin diseases.
The most common disease categories in which AI has been applied and defined as a promising asset for drug repurposing are COVID-19 (21.62%), cancer (18.17%), neurodegenerative diseases, Parkinson’s and Alzheimer’s disease being the most targeted diseases (5.72%), and infectious diseases, with COVID-19 being excluded (4.18%). Rare diseases are ranked as the fifth most cited diseases, accounting for 2.63% of the literature references.
3.1 Concrete applications of AI in drug repurposing for rare diseases
The categorization of the literature references allowed us to retrieve a total of 28 references related to rare diseases. Among these references, we identified six references describing the identification of candidates for drug repurposing in various rare diseases using different AI technologies. The other 22 articles that are not described in this review were journal articles, reviews, or research supports that did not describe concrete applications of AI in drug repurposing.
• In 2019, Ekins et al. (12) described the application of ML models for 180 approved drugs to identify potential drug repurposing for the treatment of Pitt–Hopkins syndrome (PTHS). Ekins et al. (12) first performed a high-throughput screen (HTS) of the Prestwick chemical library that allowed the identification of 55 inhibitors of Kv7.1 and 93 inhibitors of Nav1.8 as leads for the treatment of PTHS. They then aimed to compare their results to that of the Bayesian ML model in Assay Central. This model allowed the prediction of 35 Kv7.1 and 64 Nav1.8, suggesting that the combination of HTS and ML represents a strength for drug repurposing in rare diseases (12).
• In 2020, Sosa et al. (13) implemented a literature-based graph embedding method for drug repurposing that explicitly models the confidence relationship in the Global Network of Biomedical Relationships (GNBRs) based on their evidence in the literature. GNBR is a heterogeneous knowledge graph (KG) that contains the synthesis and summary of drugs, genes/proteins, and disease relationships described by 28.6 million PubMed references. By matching the information contained in the GNBR with rare disease information extracted from Orphanet—including MeSH, OMIM, and UMLS ID—Sosa et al. (13) retrieved 2,779 rare diseases. The method developed allows to compile a score for the top 30 drug repurposing candidates, in which the validity of the prediction is categorized into six categories: (1) published treatment, meaning that there is literature evidence indicating the use of the drug to treat human subjects; (2) symptom management, meaning that the drug has been used to address symptoms of the disease; (3) co-morbidity treatment, meaning that the drug treats a comorbid or closely related disease; (4) potentially feasible treatment, meaning that there is pre-clinical and/or biologically tractable evidence for the drug targeting the rare disease; (5) possible contraindication, meaning that the drug may produce a physiological effect opposite of the desired one; and (6) unknown/no-effect, where there is little or no literature evidence to support the drug-disease combination. In addition to the correct identification of treatments that have been published in the literature but not retrieved in the GNBR (i.e., cortisone for myelodysplastic syndrome or famotidine for leishmaniasis), the method demonstrates an additional inductive capacity of the model developed. Indeed, two drug candidates were identified as promising candidates for further clinical study: Trifluoperazine as a treatment for Wilms tumor and mTOR inhibitors (Everolimus) as a treatment for sarcoidosis (13).
• In 2021, Esmail and Danter (14) were a to identify new potential therapeutic options for the treatment of metachromatic leukodystrophy (MLD) using artificially generated whole-brain organoid (aiWBO) simulations of MLD. These simulations were generated using the NEUBOrg (artificially induced whole-brain organoid platform), a stem cell simulation platform with a literature-validated hybrid deep-ML system with elements of fully connected recurrent neural networks, cognitive maps, support vector machines, and evolutionary systems. Applied to MLD, NEUBOrg was used to simulate a whole-brain organoid affected by arylsulfatase A (ARSA) deficiency, resulting in the outcome of the disease modeling simulations (aiWBO-MLD). MLD drug targets were identified based on AI WBO-MLD disease profile simulations, and a list of drugs was generated. Specifically, a list of 861 single-drug and double-drug combinations was generated and evaluated by the aiWBO-MLD simulations. The drugs were then ranked according to their effects compared to placebo, and the top 12 options were obtained. All of the options were double drug combinations—regorafenib + olaparib; pembrolizumab + lenvatinib; sunitinib + lenvatinib; lenvatinib + capmatinib; rapamycin + lenvatinib; regorafenib + lenvatinib; regorafenib + calpain inhibitor; sunitinib + nutlin3; sunitinib + olaparib; olaparib + abemaciclib; palbociclib + olaparib; and ribociclib + olaparib—and display an ameliorating effect on the nine factors of MLD disease (14).
• In 2022, Cong et al. (15) presented a double-sided approach to drug repurposing by combining the gene expression responses of cell lines caused by diseases with data on drug-induced changes in expression. The authors obtained gene expression datasets from 262 patients and 268 healthy human samples associated with 31 diseases directly from the National Center for Biotechnology Information’s (NCBI) Gene Expression Omnibus (GEO). The 31 diseases were then clustered into several classified groups according to disease similarity, and three diseases were selected—according to the k-means method—for further analysis: inclusion body myositis (IBM), polymyositis (PM), and dermatomyositis (DM). Using L1000CDS2, a search engine allowing the prioritization of small molecules (SMs) and drugs to either reverse or mimic observed changes in gene expression, different drugs were identified as able to reverse the expression pattern changes for each of the three diseases: 10 drugs corresponding to IMB and PM, including gemcitabine, tosedostat, wortmannin or alvocidib; and 10 drugs corresponding to PM and DM, including selumetinib, wiskostatin or perhexiline maleate; and 14 drugs for all diseases, including salermide, wortmannin or reserpine (15).
• In 2022, Foksinka et al. (16) demonstrated that mediKanren, an AI reasoning tool that uses KGs for describing relationships between biomedical concepts, aids in treatment identification. Specifically, in cases where upregulating or downregulating a gene could be beneficial, mediKanren helps identify drugs or compounds that may accomplish the desired outcome. Coupled with literature research, the results can be ranked according to the strength of evidence for the therapeutics, and the top drug candidates are prioritized based on accessibility, bioavailability, and safety. As a case in point, this allowed to identify two FDA-approved drugs, celecoxib and levocarnitine, as respective potential therapeutics for the treatment of patients presenting RHOBTB2 and TMLHE variants (16).
• In 2023, Zhu et al. (17) constructed RDKG-115, a rare disease KG involving 115RDs, gleaned from high-quality literature and 4 biomedical datasets: (1) DRKG, a comprehensive KG for drug repurposing, (2) Pathway Commons, an integrated resource providing information on biological pathways and molecular integrations (gene, SMs, and drug entities), (3) PharmKG, a benchmark KG for biomedical mining (gene, drug, and disease entities), and (4) PMapp, a knowledge base application platform for precision medicine. Five types of entities were retrieved in these datasets: disease (DI), drug (DR), gene and gene product (GP), phenotype (PH), and SM data. RDKG-115 was then constructed through entity linking, relation linking (e.g., DI–DI or DR–GP relationships), and entity frequency screening. For the 115 RDs, Zhu et al. (17) were able to analyze the DI-DR and DI-SM relationships to identify the potential drugs and SMs repurposing. Compared to the drug-related entity pairs gathered from the 932 clinical trial records extracted from ClinicalTrials.gov (CTG) for 115 RDs, the analysis method allowed for the retrieval of approximately half of the clinical trials (48.3%).
Focusing on the most studied rare diseases in CTG, multiple sclerosis (MS), RDKG-115 allowed us to demonstrate that melatonin and amyloid-β were the two drugs exhibiting the most promising association with MS. (17)
3.2 Existent initiatives for drug repurposing in rare diseases
In addition to the concrete case studies demonstrating the application of AI technologies for drug repurposing in rare diseases, we can also identify several platforms and libraries, funded initiatives, and companies dedicated to drug repurposing in rare diseases using AI.
3.2.1 Platforms and libraries
3.2.1.1 Every Cure platform
Every Cure3 is a platform funded by the Advanced Research Projects Agency for Health (ARPA-H) aiming to allow the development of a comprehensive, open-source database of drug repurposing opportunities and to launch clinical trials with the most promising treatments (18). This platform obtains, integrates, and analyses multiple data sources (PubMed, clinicaltrials.gov, medical record data, public data repositories, and drug databases), utilizes NLP and applies an ML algorithm to identify the most promising drug repurposing opportunities. Every Cure approach has previously allowed for the identification of drug repurposing opportunities for Castleman disease (adalimumab), COVID-19 (dexamethasone and tocilizumab), and angiosarcoma (pembrolizumab).
3.2.1.2 REPO4EU
REPO4EU4 is a European Union-funded platform for validated precision drug repurposing open to stakeholders for information, multimedia training, matchmaking, and cooperation (19). REPO4EU proposes a European and global platform for mechanism-based drug repurposing, redefining diseases by applying advanced bioinformatics and AI to real-world big data.
3.2.1.3 Open Targets
This innovative, large-scale, multi-year, public-private partnership uses human genetics and genomics data for systematic drug target identification and prioritization (20).5 The OpenTargets Platform integrates public domain data to enable target identification and prioritization, whereas OpenTargets Genetics identifies targets based on Genome-Wide Association Studies (GWAS) and functional genomics.
Open Targets is a consortium of partner institutions, including major pharmaceutical companies Bristol Myers Squibb, Genentech, GSK, Pfizer, Sanofi, and Wellcome Sanger Institute, but also the European Bioinformatics Institute (MBL-EBI).
3.2.1.4 Broad Drug Repurposing Hub
The Drug Repurposing Hub is an open-access drug library and information resource repository of more than 6,000 compounds, aiming to accelerate drug development and drug repurposing (21).6
The Drug Repurposing Hub has been developed through (1) the identification of compounds, (2) the comprehensive annotation of their known activities and clinical indications, and (3) the experimental confirmation of the drug’s identity and purity. Ultimately, the Hub proposes to the scientific community a resource containing annotations—name, chemical structure, clinical trial status, mechanism of action, protein targets, disease areas, approved indications, external links, purity, and vendor ID—of more than 6,000 drugs reaching phases 1–3 in clinical development (22).
3.2.1.5 REMEDi4ALL
Launched in September 2022, REMEDi4ALL is a 5-year EU-funded research initiative aiming to drive forward the repurposing of medicines in Europe (23).7 To this end, REMEDi4ALL will build a state-of-the-art platform to provide expertise and services across the complete value chain for patient-centric medicine repurposing, assemble advanced in silico tools for ML and AI, create a global community of practice, train and educate the next generation of stakeholders and favor dialogue and debate to advance policy and fair access to repurposed medicines across the EU. The therapeutic areas that are being covered by the project are pancreatic cancer, COVID-19, rare diseases, and ultra-rare diseases.
3.2.2 Funded initiatives
3.2.2.1 DREAMS
The European Commission has invested €8 million into the Drug REpurposing and Artificial intelligence for Muscular disorders (DREAMS) consortium. Announced on 29 November 2023, this 5-year project has the objective of crafting treatment for five rare neuromuscular diseases—Duchenne muscular dystrophy, centronuclear myopathy, Emery–Dreifuss muscular dystrophy, Pompe disease, and Danon disease—through a groundbreaking methodology combining AI, stem cells, and pharmacological screening (24). This consortium unites a skilled and complementary consortium of European partners, including I-Stem (France), Kantify (Belgium), The Institute of Myology (France), Center for Neuroscience and Cell Biology (Portugal), The Technion (Israel), Samsara Therapeutics (United Kingdom), Assistance Publique—Hopitaux de Paris (France), AFM-Telethon (France), and Zabala Innovation (Spain).
3.2.3 Companies
3.2.3.1 HealX
The UK-based company leverages AI technology to identify promising drugs and compounds already approved or in clinical development that could be repurposed to treat rare diseases (25).8 Through its platform, HealNet and HealX use ML methods to extract disease knowledge from a variety of published sources and predict which drugs and combination therapies are most likely to succeed in the clinic. Since its inception, the company has entered a number of collaborative partnerships with academics, patients, and industry groups aimed at discovering new treatments for a range of rare conditions, such as Friedreich’s ataxia or muscular dystrophy.
At the time of writing (17 March 2024), the HealX pipeline focuses on 15 conditions including oncology disorders (neurofibromatosis type 1: cutaneous neurofibroma and plexiform neurofibroma), neuro-developmental disorders (Fragile X syndrome and Angelman Syndrome), renal/liver disorders (autosomal dominant/recessive polycystic kidney disease and autosomal dominant polycystic liver disease), neuromuscular disorders (myotonic dystrophy type-1, spinocerebellar ataxia and pseudoachondroplasia), ocular disorders (leber hereditary optic neuropathy and autosomal dominant optic atrophy) and other exploratory programs (Alport syndrome, alpha-1 antitrypsin deficiency, facioscapulohumeral muscular dystrophy and pitt hopkins). Noteworthy, HealX received (1) Investigational New Drug (IND) approval from the FDA for the phase 2a clinical trial of their AI-discovered treatment, Sulindac, a non-steroidal anti-inflammatory drug, for the treatment of fragile X syndrome in 2021, as well as an Orphan Drug Designation (ODD) in Europe and in the US for the same compound, and (2) a US ODD for their AI-discovered treatment, Nitroxoline, an antibiotic, for the treatment of Neurofibromatosis type 1 in 2023.
3.2.3.2 Biovista
Biovista applies its systematic discovery AI platform, Project Prodigy, to develop a pipeline of repositioned drug candidates in different disease areas, including neurodegenerative diseases, epilepsy, oncology, and orphan diseases (26).9 The platform analyzes massive data resources and identifies non-obvious, mechanism of action-based associations between compounds, molecular targets, and diseases. Biovista partners with biopharmaceutical and biotechnology companies (Astellas, Pfizer, Hewlett Packard Enterprise, Novartis, Dart Therapeutics, etc.), as well as public and private organizations and academic institutes.
4 Discussion
Through this mini-review, we have been able to characterize the proportion represented by rare diseases in the literature describing the application of AI for drug repurposing. Compared to the other diseases categorized (e.g., COVID-19, cancer, or neurodegenerative diseases), rare diseases only represent 2.63% of the overall literature, which can also be found regarding the broader use of AI in rare diseases, where, compared to other application domains (e.g., disease diagnosis, gene identification, and drug discovery), drug repurposing is also a relatively less prominent area of focus (27, 28). This low representation of rare diseases in the literature on AI-driven drug repurposing may be attributed to several factors, including the complexity of rare diseases, the small number of patients affected by rare diseases, the limited availability of data, the variability in symptoms, the genetic diversity among patients, or even the lack of funding priorities in rare diseases, making research in rare diseases not only challenging but also economically unappealing.
However, different applications have demonstrated that AI in drug repurposing is promising and that it will benefit patients suffering from rare diseases. Indeed, six concrete applications illustrated diverse strategies regarding drug repurposing in rare diseases: Ekins et al. combined HTS and ML to identify potential treatments for PTHS; Sosa et al. (13) used literature-based graph embedding to categorize drug repurposing candidates in various rare diseases. Esmail and Danter (14) generated artificial brain organoids to identify promising drug combinations for the treatment of MLD. Cong et al. used gene expression data to match diseases with potential drug candidates, while Foksinka et al. (16) used AI reasoning tools to identify therapeutics based on gene regulation, and, finally, Zhu et al. (17) constructed a rare disease KG to identify potential drugs and SMs for repurposing in various rare diseases. This momentum surrounding AI-driven drug repurposing in rare diseases is also reflected in the various current initiatives transcending core research. Scientists have developed algorithms, platforms, and libraries to inform and assist the scientific community. Companies have made it their business to identify and obtain regulatory approvals for promising drugs, while public bodies and big pharma’s involvement is already being manifested.
The landscape of AI-driven drug repurposing in rare diseases presents an intriguing paradox. We observe a scarcity of research, companies, and funding dedicated to leveraging AI for drug repurposing in rare diseases. Given the substantial financial incentives for pharmaceutical companies to pursue drug repurposing compared to the development of new drugs from scratch and given the current AI tools rapidly expanding across various sectors, including health, one can wonder why it is so rare, especially when patients with rare diseases are often treated with off-label drugs. Is the complexity of rare diseases truly hindering drug repurposing efforts? Are current AI tools not yet capable of identifying potential drug repurposing opportunities for rare diseases? Or perhaps these efforts are underway but kept low-profile by industry players to safeguard intellectual property, considering the intricate nature of AI’s intellectual property landscape? Another aspect worth considering is the question of profitability in treating rare diseases. Could concerns about the financial viability of treatments for rare diseases be a factor in the relative lack of investment in AI-driven drug repurposing, notably because it requires expertise that is scarce on the market and therefore inevitably expensive?
In light of these reflections, it would not be surprising to witness a surge of small biotech or tech companies emerging in the coming year, dedicated to the repurposing of therapies, whether polypharmacological compounds or generics, for rare diseases using AI-driven approaches. Such initiatives could hold tremendous promise for patients with rare diseases, offering new treatment options and potentially improving their lives.
Author contributions
LC: Data curation, Writing – original draft. VM: Software, Writing – review & editing. ST: Writing – review & editing. JB: Writing – review & editing. OB: Writing – review & editing.
Funding
The author(s) declare financial support was received for the research, authorship, and/or publication of this article. This material is based on the study supported by the Association nationale de la recherche et de la technologie (ANRT) with a CIFRE fellowship granted to LC.
Acknowledgments
The authors would like to thank F-CRIN for their support. OrphanDev (https://www.orphan-dev.org/) is a French national network funded by F-CRIN aiming to bring solutions to patients suffering from rare diseases.
Conflict of interest
LC, VM, and JB were employed by Thelonius Mind. ST was employed by MyDisease2Ez.
The remaining author declares that the research was conducted in the absence of any commercial or financial relationships that could be construed as a potential conflict of interest.
Publisher’s note
All claims expressed in this article are solely those of the authors and do not necessarily represent those of their affiliated organizations, or those of the publisher, the editors and the reviewers. Any product that may be evaluated in this article, or claim that may be made by its manufacturer, is not guaranteed or endorsed by the publisher.
Footnotes
1. ^https://www.ncbi.nlm.nih.gov/pmc/articles/PMC7347027/
2. ^https://link-springer-com.proxy.insermbiblio.inist.fr/article/10.1007/s11042-022-13428-4
5. ^https://www.opentargets.org/
References
1. Haendel, M, Vasilevsky, N, Unni, D, Bologa, C, Harris, N, Rehm, H, et al. How many rare diseases are there? Nat Rev Drug Discov. (2020) 19:77–8. doi: 10.1038/d41573-019-00180-y
2. Scherman, D, and Fetro, C. Drug repositioning for rare diseases: knowledge-based success stories. Therapie. (2020) 75:161–7. doi: 10.1016/j.therap.2020.02.007
3. Roessler, HI, Knoers, NVAM, van Haelst, MM, and van Haaften, G. Drug repurposing for rare diseases. Trends Pharmacol Sci. (2021) 42:255–67. doi: 10.1016/j.tips.2021.01.003
4. Choi, RY, Aaron, SC, Jayashree, K-C, Michael, FC, and Peter, JC. “Introduction to Machine Learning, Neural Networks, and Deep Learning.” Translational Vision Science & Technology (n.d.). 9: 14. doi: 10.1167/tvst.9.2.14
5. Khurana, D, Aditya, K, Kiran, K, and Sukhdev, S. “Natural Language Processing: State of the Art, Current Trends and Challenges.” Multimedia Tools and Applications 82: 3713–44. doi: 10.1007/s11042-022-13428-4
6. Alowais, SA, Alghamdi, SS, Alsuhebany, N, Alqahtani, T, Alshaya, AI, Almohareb, SN, et al. Revolutionizing healthcare: the role of artificial intelligence in clinical practice. BMC Med Educ. (2023) 23:689. doi: 10.1186/s12909-023-04698-z
7. Bajwa, J, Munir, U, Nori, A, and Williams, B. Artificial intelligence in healthcare: transforming the practice of medicine. Future Healthc J. (2021) 8:e188–94. doi: 10.7861/fhj.2021-0095
8. Brasil, S, Pascoal, C, Francisco, R, Dos Reis Ferreira, V, Videira, PA, and Valadão, AG. Artificial intelligence (AI) in rare diseases: is the future brighter? Genes. (2019) 10:978. doi: 10.3390/genes10120978
9. Jonker, AH, O’Connor, D, Cavaller-Bellaubi, M, Fetro, C, Gogou, M, ’t Hoen, PAC, et al. Drug repurposing for rare: progress and opportunities for the rare disease community. Front Med. (2024) 11:1352803. doi: 10.3389/fmed.2024.1352803
10. van der Pol Karel, H, Aljofan, M, Blin, O, Cornel, JH, Rongen, GA, Woestelandt, A-G, et al. Drug repurposing of generic drugs: challenges and the potential role for government. Appl Health Econ Health Policy. (2023) 21:831–40. doi: 10.1007/s40258-023-00816-6
11. U.S. National Library of Medicine. (2024). The new PubMed updated: summary display with full author list, send to: Citation Manager, PubMed Format, and more. Available at: https://www.nlm.nih.gov/pubs/techbull/tb.html. (Consulté le avril 25, 2024)
12. Ekins, S, Gerlach, J, Zorn, KM, Antonio, BM, Lin, Z, and Gerlach, A. Repurposing approved drugs as inhibitors of Kv7.1 and Nav1.8 to treat Pitt Hopkins syndrome. Pharm Res. (2019) 36:137. doi: 10.1007/s11095-019-2671-y
13. Sosa, DN, Derry, A, Guo, M, Wei, E, Brinton, C, and Altman, RB. A literature-based knowledge graph embedding method for identifying drug repurposing opportunities in rare diseases. Pac Symp Biocomput. (2020) 25:463–74.
14. Esmail, S, and Danter, WR. Artificially induced pluripotent stem cell-derived whole-brain organoid for modelling the pathophysiology of metachromatic leukodystrophy and drug repurposing. Biomedicines. (2021) 9:440. doi: 10.3390/biomedicines9040440
15. Cong, Y, Shintani, M, Imanari, F, Osada, N, and Endo, T. A new approach to drug repurposing with two-stage prediction, machine learning, and unsupervised clustering of gene expression. OMICS. (2022) 26:339–47. doi: 10.1089/omi.2022.0026
16. Foksinska, A, Crowder, CM, Crouse, AB, Henrikson, J, Byrd, WE, Rosenblatt, G, et al. The precision medicine process for treating rare disease using the artificial intelligence tool mediKanren. Front Artif Intell. (2022) 5:910216. doi: 10.3389/frai.2022.910216
17. Zhu, C, Xia, X, Li, N, Zhong, F, Yang, Z, and Liu, L. RDKG-115: assisting drug repurposing and discovery for rare diseases by trimodal knowledge graph embedding. Comput Biol Med. (2023) 164:107262. doi: 10.1016/j.compbiomed.2023.107262
18. Every Cure. (2024). Unlocking the hidden potential of existing drugs to save lives. Available at: https://everycure.org/. (Consulté le mars 17, 2024)
19. REPO4EU. (2024). Euro-global platform for drug repurposing. Available at: https://repo4.eu/. (Consulté le mars 18, 2024)
20. Open Targets. (2024). Available at: https://www.opentargets.org/. (Consulté le 17 mars, 2024)
21. Broad Institute. (2019). Drug Repurposing Hub. Available at: https://www.broadinstitute.org/drug-repurposing-hub
22. Corsello, SM, Joshua, AB, Zihan, L, Joshua, G, Patrick, M, Jodi, EH, et al. “The Drug Repurposing Hub: A next-Generation Drug Library and Information Resource.” Nature Medicine (2017) 23: 405–8. doi: 10.1038/nm.4306
23. EURORDIS. (2024). REMEDi4ALL, an ambitious EU-funded research initiative, launches to drive forward the repurposing of medicines in Europe. Available at: https://www.eurordis.org/press-release-remedi4all/. (Consulté le mars 18, 2024)
24. AFM Téléthon. (2023). I-Stem, coordinateur du consortium de recherche européen DREAMS associant intelligence artificielle, cellules souches et criblage pharmacologique pour traiter des maladies neuromusculaires. Available at: https://www.afm-telethon.fr/fr/communiques-de-presse/i-stem-coordinateur-du-consortium-de-recherche-europeen-dreams-associant
25. HealX. (2024). AI drug discovery. Rare disease treatment. Available at: https://healx.ai/. (Consulté le mars 17, 2024)
26. Biovista. (2024). Drug positioning and prioritization-drug repositioning. Biovista drug positioning and prioritization (blog). Available at: https://www.biovista.com/solutions/drug-repositioning/. (Consulté le mars 17, 2024)
27. Visibelli, A, Bianca, R, Ottavia, S, and Annalisa, S. “The Impact of Artificial Intelligence in the Odyssey of Rare Diseases.” Biomedicines (2023) 1: 887. doi: 10.3390/biomedicines11030887
Keywords: artificial intelligence, machine learning, deep learning, drug repurposing, rare diseases
Citation: Cortial L, Montero V, Tourlet S, Del Bano J and Blin O (2024) Artificial intelligence in drug repurposing for rare diseases: a mini-review. Front. Med. 11:1404338. doi: 10.3389/fmed.2024.1404338
Edited by:
Viviana Giannuzzi, Fondazione per la Ricerca Farmacologica Gianni Benzi onlus, ItalyReviewed by:
Pierre-Axel Monternier, Reventus Pharma, FranceSandra Brasil, NOVA University of Lisbon, Portugal
Copyright © 2024 Cortial, Montero, Tourlet, Del Bano and Blin. This is an open-access article distributed under the terms of the Creative Commons Attribution License (CC BY). The use, distribution or reproduction in other forums is permitted, provided the original author(s) and the copyright owner(s) are credited and that the original publication in this journal is cited, in accordance with accepted academic practice. No use, distribution or reproduction is permitted which does not comply with these terms.
*Correspondence: Lucas Cortial, bHVjYXMuY29ydGlhbEB0aGVsb25pdXNtaW5kLmNvbQ==