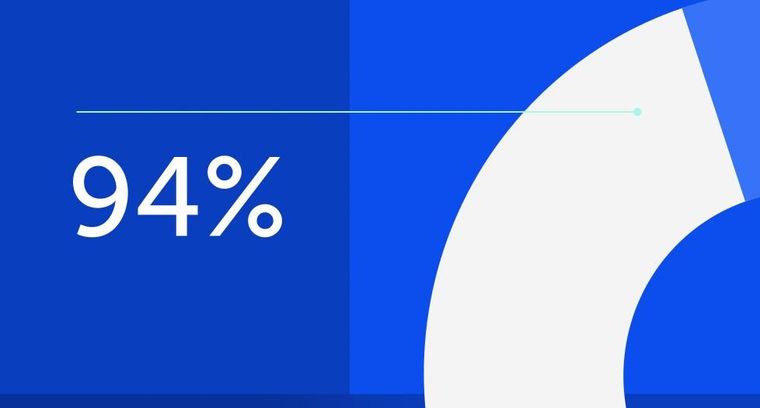
94% of researchers rate our articles as excellent or good
Learn more about the work of our research integrity team to safeguard the quality of each article we publish.
Find out more
EDITORIAL article
Front. Med., 05 April 2024
Sec. Rheumatology
Volume 11 - 2024 | https://doi.org/10.3389/fmed.2024.1402871
This article is part of the Research TopicArtificial Intelligence in Rheumatology and Musculoskeletal DiseasesView all 8 articles
Editorial on the Research Topic
Artificial intelligence in rheumatology and musculoskeletal diseases
The field of artificial intelligence (AI) in medicine has evolved dramatically over the past decade, paving the way for ground-breaking innovation. Recently, the potentiality of AI in rheumatology and musculoskeletal diseases has drawn the attention of the research community. In this Research Topic, various advancements, and applications of AI in the field are showcased, shedding light on this relatively unexplored domain.
Traditionally, the role of a physician in diagnosing and treating health issues has relied heavily on human intelligence, intuition, and experience. However, the process of formulating a diagnosis, the crucial first step in medical intervention, often proves to be challenging and subjective. It involves gathering information through patient history, physical examination, laboratory tests, and imaging studies, followed by the interpretation of this data. Herein lies the opportunity for AI to augment human intelligence in solving health-related problems by managing big data in medicine to support clinicians in the actual clinical practice.
AI could boost clinicians' knowledge and support their decision-making to create an "artificially enhanced rheumatologist.” AI algorithms, particularly data-driven ones such as machine and deep learning, leverage vast amounts of patient data to predict outcomes and facilitate informed decision-making. These algorithms can analyse physician notes (1), laboratory results (2), and imaging studies (3, 4) to aid in the management of rheumatic diseases (5) and to predict patients' prognosis (6). For instance, rule-based natural language processing and text extraction from large datasets of unstructured information have provided insights into treatment patterns and outcomes for rheumatic diseases. Motta et al. have shown that patients with rheumatoid arthritis (RA), psoriatic arthritis (PsA), and psoriasis treated with a multidisciplinary approach are more likely to receive innovative treatments or glucocorticoids, possibly reflecting more complex cases.
Machine-learning algorithms show promise for processing advanced imaging techniques, such as fluorescence optical imaging (FOI), which enables the visualization and analysis of inflammation in rheumatic diseases. In the paper authored by Rothe et al., machine-learning algorithms were applied to reveal the importance ranking of imaging features in FOI and find the most informative findings for accurately diagnosing different rheumatic diseases. Furthermore, deep-learning models can segment relevant structures in ultrasound images and help in educating young sonographers (7) and in the acquisition and interpretation (8, 9) of ultrasound images. Overgaard et al. have developed an AI model which can identify osteophytes and score them using a validated semiquantitative system for ultrasound imaging. The agreement performance between an expert reader and the AI algorithm was found to be slightly higher than previously found agreements between experts when assessing osteophytes on ultrasound images of the hand joints, with potential applications in the diagnosis and monitoring of hand osteoarthritis.
Besides, AI can assist clinicians during remote consultations and telemedicine. Phatak et al. have evaluated the performance of a convolutional neural network on standardized smartphone photographs in detecting inflammation in three hand joints (i.e., wrist, PIP2 and PIP3) of patients with various rheumatic diseases and compared it to a rheumatologist's diagnosis. The good-to-excellent diagnostic accuracy of AI suggests a potential use of computer vision in screening and follow-up of inflammatory arthritis involving the hands.
Additionally, the collection contains three insightful reviews on the integration of AI in rheumatology. The first review authored by Nicoara et al. emphasizes AI's role in the early diagnosis of conditions like RA and axial spondyloarthritis through magnetic resonance imaging. The second review by Gilvaz and Reginato highlights AI's potential in leveraging patient data for optimized management strategies in RA from diagnosis to treatment, while the third review by Dinescu et al. explores AI's applications in musculoskeletal ultrasonography, offering automated solutions for disease monitoring. Together, these reviews underscore the transformative impact of AI in various facets of rheumatology, from diagnosis to treatment optimization and imaging standardization.
The use of AI in rheumatology offers several advantages, including increased efficiency, reduced variability, and improved accessibility to healthcare services. It has the potential to streamline medical care, assist clinicians in decision-making processes, and enhance patient outcomes. However, challenges such as technical limitations, regulatory hurdles, small study size, and risk of overfitting must be addressed before AI can be integrated into routine clinical practice.
In conclusion, while the full potential of AI in rheumatology has yet to be unlocked, ongoing advancements and successful pilot studies indicate a promising future for AI-driven patient care. With continued research and collaboration, AI has the potential to revolutionize the diagnosis, treatment, and management of rheumatic and musculoskeletal diseases, ultimately improving the lives of patients worldwide.
EC: Conceptualization, Writing – original draft, Writing – review & editing. MF: Writing – original draft, Writing – review & editing. FV: Writing – original draft, Writing – review & editing. SM: Writing – original draft, Writing – review & editing. EF: Writing – original draft, Writing – review & editing.
The author(s) declare that no financial support was received for the research, authorship, and/or publication of this article.
The authors declare that the research was conducted in the absence of any commercial or financial relationships that could be construed as a potential conflict of interest.
All claims expressed in this article are solely those of the authors and do not necessarily represent those of their affiliated organizations, or those of the publisher, the editors and the reviewers. Any product that may be evaluated in this article, or claim that may be made by its manufacturer, is not guaranteed or endorsed by the publisher.
1. Goh KH, Wang L, Yeow AYK, Poh H, Li K, Yeow JJL, et al. Artificial intelligence in sepsis early prediction and diagnosis using unstructured data in healthcare. Nat Commun. (2021) 12:1–10. doi: 10.1038/s41467-021-20910-4
2. Wu YD, Sheu RK, Chung CW, Wu YC, Ou CC, Hsiao CW, et al. Application of supervised machine learning to recognize competent level and mixed antinuclear antibody patterns based on icap international consensus. Diagnostics. (2021) 11:642. doi: 10.3390/diagnostics11040642
3. Moon SJ, Lee S, Hwang J, Lee J, Kang S, Cha HS. Performances of machine learning algorithms in discriminating sacroiliitis features on MRI: a systematic review. RMD Open. (2023) 9:3783. doi: 10.1136/rmdopen-2023-003783
4. Christensen AB, Just SA, Andersen JK, Savarimuthu TR. Applying cascaded convolutional neural network design further enhances automatic scoring of arthritis disease activity on ultrasound images from rheumatoid arthritis patients. Ann Rheum Dis. (2020) 79:1189–93. doi: 10.1136/annrheumdis-2019-216636
5. Norgeot B, Glicksberg BS, Trupin L, Lituiev D, Gianfrancesco M, Oskotsky B, et al. Assessment of a deep learning model based on electronic health record data to forecast clinical outcomes in patients with rheumatoid arthritis. JAMA Netw Open. (2019) 2:e190606. doi: 10.1001/jamanetworkopen.2019.0606
6. Tiulpin A, Klein S, Bierma-Zeinstra SM, Thevenot J, Rahtu E, Meurs JV, et al. Multimodal machine learning-based knee osteoarthritis progression prediction from plain radiographs and clinical data. Sci Rep. (2019) 9:1–12. doi: 10.1038/s41598-019-56527-3
7. Cipolletta E, Fiorentino MC, Moccia S, Guidotti I, Grassi W, Filippucci E, et al. Artificial intelligence for ultrasound informative image selection of metacarpal head cartilage. A pilot study. Front Med. (2021) 8:589197. doi: 10.3389/fmed.2021.589197
8. Fiorentino MC, Cipolletta E, Filippucci E, Grassi W, Frontoni E, Moccia S, et al. A deep-learning framework for metacarpal-head cartilage-thickness estimation in ultrasound rheumatological images. Comput Biol Med. (2022) 141:105117. doi: 10.1016/j.compbiomed.2021.105117
9. Smerilli G, Cipolletta E, Sartini G, Moscioni E, Di Cosmo M, Fiorentino MC, et al. Development of a convolutional neural network for the identification and the measurement of the median nerve on ultrasound images acquired at carpal tunnel level. Arthritis Res Ther. (2022) 24:38. doi: 10.1186/s13075-022-02729-6
Keywords: artificial intelligence, convolutional neural network (CNN), imaging, rheumatology, arthritis, magnetic resonance imaging, ultrasound
Citation: Cipolletta E, Fiorentino MC, Vreju FA, Moccia S and Filippucci E (2024) Editorial: Artificial intelligence in rheumatology and musculoskeletal diseases. Front. Med. 11:1402871. doi: 10.3389/fmed.2024.1402871
Received: 18 March 2024; Accepted: 25 March 2024;
Published: 05 April 2024.
Edited and reviewed by: João Eurico Fonseca, University of Lisbon, Portugal
Copyright © 2024 Cipolletta, Fiorentino, Vreju, Moccia and Filippucci. This is an open-access article distributed under the terms of the Creative Commons Attribution License (CC BY). The use, distribution or reproduction in other forums is permitted, provided the original author(s) and the copyright owner(s) are credited and that the original publication in this journal is cited, in accordance with accepted academic practice. No use, distribution or reproduction is permitted which does not comply with these terms.
*Correspondence: Edoardo Cipolletta, edoardocipolletta@gmail.com
†ORCID: Edoardo Cipolletta orcid.org/0000-0002-6881-8197
Maria Chiara Fiorentino orcid.org/0000-0002-7397-803X
Sara Moccia orcid.org/0000-0002-4494-8907
Emilio Filippucci orcid.org/0000-0002-7251-7784
Disclaimer: All claims expressed in this article are solely those of the authors and do not necessarily represent those of their affiliated organizations, or those of the publisher, the editors and the reviewers. Any product that may be evaluated in this article or claim that may be made by its manufacturer is not guaranteed or endorsed by the publisher.
Research integrity at Frontiers
Learn more about the work of our research integrity team to safeguard the quality of each article we publish.