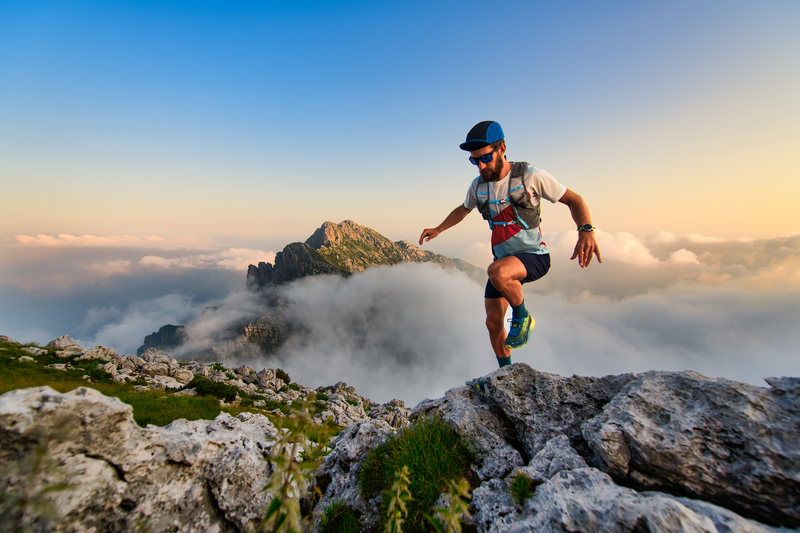
94% of researchers rate our articles as excellent or good
Learn more about the work of our research integrity team to safeguard the quality of each article we publish.
Find out more
ORIGINAL RESEARCH article
Front. Med. , 13 June 2024
Sec. Precision Medicine
Volume 11 - 2024 | https://doi.org/10.3389/fmed.2024.1381031
This article is part of the Research Topic Molecular Informatics in Precision Medicine View all 10 articles
Introduction: Sarcoidosis is a multi-system inflammatory disease of unknown origin with heterogeneous clinical manifestations varying from a single organ non-caseating granuloma site to chronic systemic inflammation and fibrosis. Gene expression studies have suggested several genes and pathways implicated in the pathogenesis of sarcoidosis, however, due to differences in study design and variable statistical approaches, results were frequently not reproducible or concordant. Therefore, meta-analysis of sarcoidosis gene-expression datasets is of great importance to robustly establish differentially expressed genes and signalling pathways.
Methods: We performed meta-analysis on 22 published gene-expression studies on sarcoidosis. Datasets were analysed systematically using same statistical cut-offs. Differentially expressed genes were identified by pooling of p-values using Edgington’s method and analysed for pathways using Ingenuity Pathway Analysis software.
Results: A consistent and significant signature of novel and well-known genes was identified, those collectively implicated both type I and type II interferon mediated signalling pathways in sarcoidosis. In silico functional analysis showed consistent downregulation of eukaryotic initiation factor 2 signalling, whereas cytokines like interferons and transcription factor STAT1 were upregulated. Furthermore, we analysed affected tissues to detect differentially expressed genes likely to be involved in granuloma biology. This revealed that matrix metallopeptidase 12 was exclusively upregulated in affected tissues, suggesting a crucial role in disease pathogenesis.
Discussion: Our analysis provides a concise gene signature in sarcoidosis and expands our knowledge about the pathogenesis. Our results are of importance to improve current diagnostic approaches and monitoring strategies as well as in the development of targeted therapeutics.
Sarcoidosis is an inflammatory disorder characterized by the formation of non-caseating epithelioid granulomas in various organs. However, the aetiology and pathogenesis of sarcoidosis are not fully understood. The lungs and hilar lymph nodes are most often affected, but almost all organs can be involved (1). The clinical presentation of sarcoidosis is therefore heterogeneous and can range from small benign skin lesions to chronic systemic inflammation. This variability in disease presentation makes it challenging to diagnose sarcoidosis. This diagnosis is mainly based on clinical and radiographic presentation, pathological evidence of non-caseating granulomas and the exclusion of other granulomatous diseases (2, 3). Even though, the lesions resolve spontaneously in a large portion of patients, irreversible tissue damage, like pulmonary fibrosis occurs in up to 20% of cases leading to increased morbidity and mortality (4, 5). More knowledge about the molecular mechanisms involved in the pathophysiology of sarcoidosis is warranted to develop better and more adequate monitoring strategies as well as treatment options.
There is a consensus that complex interactions between genetic and environmental triggers culminate into an aberrant immune response to unidentified antigens including infectious agents (6). Moreover, it is proposed that sarcoidosis encompasses both autoinflammatory and autoimmune features (7). Particularly the similarities of sarcoidosis with Blau syndrome (early-onset sarcoidosis), caused by mutations in nucleotide-binding oligomerization domain containing 2 (NOD2), supports an autoinflammatory hypothesis. Meanwhile, the association with HLA-DRB1 genotypes provides more evidence in the direction of autoimmunity (8). In patients with sarcoidosis, an altered T helper 1 (Th1) immune response is observed partly through activation of signal transducer and activator of transcription 1 (STAT1) and production of interferons (IFNs) (9, 10). This has led to the targeting of the Janus kinase (JAK)-STAT signalling pathway in sarcoidosis using inhibitors like baricitinib (11) and tofacitinib (12) for patients with refractory symptoms.
Gene expression studies, also referred to as transcriptomic studies, have been very promising and widely used to identify disease-associated differentially expressed genes (DEGs). Such studies can provide candidate targets for therapy as well as disease biomarkers. However, a concern about transcriptomic studies is their reproducibility and generalizability mainly due to differences in study design, data analysis strategies and limited sample size. With the increasing awareness of open data, more and more datasets are becoming available allowing to identify specific disease associated genes and pathways suitable for therapeutic intervention. Systematic meta-analysis of transcriptomic data for sarcoidosis provides a powerful tool to identify robust gene signatures. Therefore, we systematically analysed sarcoidosis transcriptome by performing meta-analysis on 22 gene expression datasets obtained from various tissues, bronchoalveolar lavage fluid (BALF) and peripheral blood comparing sarcoidosis patients with healthy controls taking into account both the blood and target tissue samples.
The genome expression omnibus (GEO) database (13) was queried for expression profiling by array or high-throughput sequencing using the following string: “Sarcoidosis [All Fields] AND GSE [All Fields].” Datasets containing human RNA expression were selected and further explored with original papers for study design. We excluded single-cell RNA sequencing experiments as well as those with unclear study design or sample annotation. Only datasets with more than four sarcoidosis patients and healthy controls were included in this comparative study. Raw data from these selected studies were downloaded from the GEO database and further processed.
Normalization of Affymetrix and Illumina BeadChip array data was performed with robust microarray average (RMA) within the R package affy (14) and with neqc within the R package limma (15), respectively. Quantile normalization on the gProcessedSignal and subsequent log-transformation, was used for Agilent datasets. RNAseq count data was normalized within the R package DESeq2 (16). Principal component analysis was performed to assess batch effects and if that was suspected, the ComBat function within the SVA R package (17) was used for batch correction of the gene expression dataset. Differential expression was calculated for every dataset using the limma and DESeq2 R packages for array and sequencing data, respectively. If a dataset contained multiple cell-types or tissues, it was analysed separately based on the cell-types. A paired analysis was performed on datasets containing multiple samples from a single individual. We did not adjust for confounding factors like age, gender or ethnicity, due to the scarcity of data in the datasets, whereas we aimed at analysing each dataset systematically and uniformly.
First, we investigated the sarcoidosis datasets for commonly DEGs. In this analysis, we did not differentiate datasets based on cell type or tissue. A list of DEGs was obtained per dataset by setting the significance level of the adjusted p-value (padj) to less than 0.05 and subsequently annotated the acquired gene lists with HUGO gene symbols. Because the threshold put on the log fold change (FC) depends on the gene and experimental context (18), we did not use the log FC to determine DEGs as a standardized threshold would be too lenient for some datasets and too stringent for others. Ingenuity Pathway Analysis (IPA) (19) was used for functional core analysis of all acquired gene lists. A comparative analysis was performed to investigate the pathways and upstream regulators involved across datasets. Second, the gene lists of DEGs were analysed for overlapping genes. After the individual differential expression analyses, a meta-analysis was performed to test the robustness of our findings. The p-values from the individual analyses were combined using Edgington’s method (20) within the R package metap (21). The calculated pmeta-analysis was subsequently Bonferroni corrected to adjust for multiple testing. Pattern of differential expression was investigated for each gene in the individual datasets through the log FC, where a positive and negative log FC were categorized as upregulation and downregulation of the gene, respectively. If the pattern differed in more than three datasets, the gene was not considered consistently differentially expressed and was excluded from further analysis. Thus an acquired gene list was loaded in IPA to build an integrated gene network. Furthermore, to examine these genes in the context of JAK–STAT pathway, IFN signalling was investigated in a separate analysis. These genes were loaded in a dataset (GSE110549) generated by the Immunological Genome Project exploring in vivo effects of IFN-α and IFN-γ stimulation on murine macrophages (22).
Furthermore, we investigated whether there are cell type or tissue-specific gene expression profiles in sarcoidosis to determine biological mechanisms of granulomatous inflammation and find specific targets for therapy. Because the majority of datasets contained blood samples, we made a distinction between blood and other cell types. We investigated the acquired gene lists for genes exclusively differentially expressed in the affected sarcoidosis tissues. Finally, identified genes that were exclusively up- or downregulated in tissues were uploaded in IPA to investigate possible tissue-specific pathways, upstream regulators and gene networks.
A systematic search was conducted in the GEO database for sarcoidosis specific datasets. Up until January 2024, our search strategy retrieved 80 datasets worldwide, of which 22 datasets (23–41) were selected and subjected to further analysis after rigorous exclusion criteria (Table 1 and Figure 1). In these 22 datasets, a total of 461 sarcoidosis and 497 healthy control samples across multiple tissues and cell types were analysed. Thirteen datasets used blood derived cells for their analysis, whereas 8 datasets were derived from lung, BALF, nasal brush, lymph node and skin tissue. One dataset contained both blood and BALF samples, which were analysed as separate datasets. Besides diagnostic parameters, clinical details were often not included or reported for individual samples. According to the original manuscripts, 11 out of 22 datasets contained at least one sample that used immunosuppressive therapy for sarcoidosis. Analysis of individual datasets for DEGs (padj < 0.05) revealed altered expression of a large number of genes. Thus derived lists of DEGs per dataset ranged from zero to thousands of genes, including two out of 22 datasets that did not show any DEGs (Figure 1).
Figure 1. Flowchart of dataset selection and analysis. *One dataset contained both blood and BALF samples.
In order to allow cross-platform comparison of the data sets, we annotated the lists of DEGs using the platform identifier with HUGO gene nomenclature. Highly consistent DEGs were identified: 30 genes were differentially expressed in at least 13 datasets, of which 20 showed very consistent pattern of differential expression (if the pattern of differential expression differed in more than three datasets, the gene was not considered as a DEG) across the datasets (Table 2). These genes were differentially expressed in both blood-derived datasets as well as in datasets derived from affected tissues. Guanlytate-binding protein 1 (GBP1), STAT1 and tryptophayl-tRNA synthetase 1 (WARS1) showed most consistent differential expression in 16 out of 22 datasets and expression levels were found predominantly upregulated in sarcoidosis. Differential expression of genes high-affinity gamma FC receptor I (FCGR1A also referred as CD64), GBP2, and vesicle-associated membrane protein 5 (VAMP5) was observed in 15 datasets. Interestingly, STAT1, GBP1, GBP2 and GBP5 were upregulated in all datasets (Table 2).
Next, we integrated all the datasets to perform meta-analysis on the p-values to identify additional consistent DEGs as some genes could not be investigated on all transcriptomic platforms due to their varied design. Pooling of p-values with Edgington’s method and subsequent Bonferroni correction resulted in 36 significantly expressed genes. Of these 36 genes, 12 displayed variable pattern of up or down regulation and hence, they were excluded from further analysis (Table 3). To investigate the relationship between the remaining 24 genes, a connectivity plot was generated in IPA (Figure 2). Remarkably, more than half (14 out of the 24) of the genes were well connected in the connectivity network. STAT1, IL-12, and IFN signalling pathways appeared centrally positioned within the network and were predicted to be activated. Also insulin was positioned in the network. Both type I IFN (IFN-α and IFN-β) and type II IFN (IFN-γ) were predicted to be upregulated within the connectivity network. Therefore, we explored this further in a murine dataset specifically on IFN signalling (GSE110549) where we verified that half of the genes identified in the meta-analysis were upregulated upon IFN stimulation, advocating for a pivotal role of altered IFN signalling in sarcoidosis (Supplementary Figure S1).
Figure 2. Connectivity network generated on 16 of the 25 significant genes from the meta-analysis. Centrally positioned in the network are STAT1 and interferon alpha, which suggests these play an important role in sarcoidosis. Red and green molecules are up- and downregulated in the genelist respectively, whilst orange and blue are predicted to be up- and downregulated based on the input genelist by the molecule activity predictor (MAP) function within IPA.
All individual gene lists of DEGs were loaded into IPA for functional pathway analyses. Eukaryoitc initation factor 2 (EIF2) signalling pathway was consistently predicted to be downregulated across datasets in sarcoidosis patients as compared to healthy controls. On the other hand, pro-inflammatory pathways like IFN-signalling, neuro-inflammation and hypercytokinaemia/hyperchemokinaemia were predicted to be activated in sarcoidosis patients (Figure 3A). This was further indicated by the upstream regulators found within IPA analysis. Pro-inflammatory transcription factors and cytokines (i.e., STAT1, TNF, IL-6 and IL-1β) were consistently predicted to be activated (Figure 3B). Also type I and type II IFNs were consistently predicted to be upregulated, strongly suggesting activation of the IFN-STAT1 pathway. Only a few upstream regulators were consistently downregulated after stringent filtering within IPA. Inhibitors like, PD98059 U0126 and LY294002 (Figure 3B) target the MAPK/ERK and PI3K/AKT/mTOR pathways, suggesting that these chemical compounds could inhibit these activated signalling pathways in sarcoidosis. These pathways often run in parallel and converge to regulate important cellular processes.
Figure 3. In silico functional analysis of the individual differentially expressed gene lists. (A) Heatmap of the comparison analysis within IPA that shows the canonical pathways involved in each individual dataset. Across the datasets, similar canonical pathways were predicted to be involved. (B) Heatmap that shows the upstream regulators predicted to be involved across the functional analyses of the individual datasets.
Finally, we investigated tissue-specific gene signatures in sarcoidosis. We hypothesized that DEGs in affected tissues may play a crucial role in granuloma formation and their maintenance. Nine datasets were retrieved from tissues, of which 8 showed DEGs. A disintegrin and metalloprotease like decysin 1 (ADAMDEC1) and WD repeat and SOCS box-containing protein 1 (WSB1) were differentially expressed (upregulated) in seven datasets, however, these were also differentially expressed in a few blood datasets. The most consistent tissue-specific DEG was matrix metallopeptidase 12 (MMP12), which was highly upregulated in 6 tissue-derived datasets (two BALF, two lung, one lacrimal gland and one lymph node derived dataset) and in no blood-derived dataset. In five of the tissue-specific datasets C-X-C motif chemokine receptor 6 (CXCR6) and syntrophin beta 2 (SNTB2), whereas in four datasets colony stimulating factor 2 (CSF2), fatty acid desaturase 1 (FADS1), interleukin 18 binding protein (IL18BP), acyl-CoA synthetase family member 2 (ACSF2), cystatin B (CSTB), C-C motif chemokine ligand 4 (CCL4), adenosine deaminase (ADA), JAK3, malic enzyme 1 (ME1), muscle RAS Oncogene Homolog (MRAS) and RAS guanyl releasing protein 3 (RASGRP3) were differentially expressed. Most of these genes are involved in the activation of processes such as leukocyte recruitment and migration and form a concise network around PI3K and MAPK/ERK signalling (Figure 4).
Figure 4. Connectivity network generated on 9 of the 14 genes with high tissue specificity. The PI3K-pathway and MAPK/ERK signalling are centrally positioned in the network. Red and green molecules are up- and downregulated in the genelist respectively, whilst orange and blue are predicted to be up- and downregulated based on the input genelist by the molecule activity predictor (MAP) function within IPA.
Using public datasets in the GEO database, we studied 22 sarcoidosis datasets and performed a meta-analysis to identify genes and pathways those are common across these studies and differentiate sarcoidosis patients from healthy controls. We found that there are clear differences in gene expression profiles for GBP1, STAT1 and WARS1 among others between sarcoidosis patients and healthy controls. Furthermore, the integration of datasets provided a comprehensive view for certain genes (STAT1, WARS1, GBP1, VAMP5, and PSTPIP2) being consistently expressed in the majority of datasets advocating for their role in the pathogenesis of sarcoidosis. These genes could potentially be used to develop meaningful genomic-derived biomarkers for sarcoidosis. In analogy to several other transcriptomic studies, we identified both type I and type II IFN signalling as important pathways (42), but in this meta-analysis also poorly studied pathways became apparent in the context of sarcoidosis such as EIF2 signalling and neuro-inflammation. Finally, we explored datasets with samples from sarcoidosis-affected tissues and identified tissue-specific DEGs (MMP12, CXCR6, and SNTB2) those likely to play important respective roles, specifically in granulomatous inflammation. Hence, it can be proposed that these specific genes and their respective translated proteins could be targeted for precise therapy of sarcoidosis lesions.
Whether a gene is considered differentially expressed in transcriptomic studies revolves around the chosen cut-offs in (adjusted) p-value and fold change. The results between transcriptomic studies often differ due to differences in study design, analysis strategies and sample size. Therefore, to circumvent these issues, a meta-analysis on several datasets of particular disease, becomes an important tool for analysis and inferences increasing the strength of such studies to establish true signals (43). We assessed the differential expression pattern by integrating these datasets based on the p-values thus creating a robust analysis to identify consistently DEGs. In this study, to the best of our knowledge, the largest systematic meta-analysis of transcriptomic data in sarcoidosis is being presented.
Sarcoidosis research so far has predominantly focused on the IFN-STAT1 pathway linked to T cells and macrophages. In fact, there have been numerous reports on the development and exacerbation of sarcoidosis after IFN therapy (44, 45). Our study highlights the importance of this pathway as several of the most consistently DEGs are associated with IFN signalling. Furthermore, in IPA analysis JAK1/2 was found in the connectivity network, strongly suggesting the rationale to target the JAK-STAT pathway with JAK inhibitors in sarcoidosis patients. Interestingly, we identified multiple members of GBP gene family to be consistently differentially expressed (GBP1, GBP2, GBP4 and GBP5). These genes are a group of seven IFN-inducible GTPases implicated in the host defence against intracellular pathogens by targeting and inducing lysis of pathogen-containing vacuoles (46). Differential expression of GBPs as found in our study, is not only attributed to the pulmonary sarcoidosis, but aberrant expression of GBP1 has also been demonstrated earlier in acute respiratory distress syndrome (47).
One of the most consistently differentially expressed genes was FCGR1A (CD64), which was differentially expressed in 15 out of 22 datasets. This gene is strongly induced by IFN-γ and plays a central role in antibody-dependent cytotoxicity and FCγ receptor-mediated phagocytosis (48). Phagosome and phagocytosis has been reported to be upregulated in monocytes of sarcoidosis patients (49). In proteomic studies, FCγ receptor-mediated phagocytosis is upregulated in sarcoidosis (50, 51). Several genes including FCGR1A, ubiquitin conjugating enzyme E2 L6 (UBE2L6) and VAMP5, found statistically significant in our meta-analysis have also been described in the context of tuberculosis (TB) (52). In both sarcoidosis and TB, granulomas are the hallmark, but the granulomas in sarcoidosis are non-caseating, whereas the granulomas in TB frequently contain a necrotic core. Most likely these diseases, despite their differences, may share common inflammatory pathways and mechanisms corroborated by overlapping gene expression profiles (25, 31, 33).
Our analysis revealed novel genes, such as WARS1 and VAMP5 those were never implicated in sarcoidosis. WARS1 is an essential enzyme called tryptophayl-tRNA synthetase 1 that charges tryptophane to its cognate tRNA and also plays a role in the innate immune system. WARS1 is upregulated upon infection and can act as a ligand of toll-like receptor (TLR) 2 and TLR4. This leads to secretion of cytokines and activation of various immune pathways (53). VAMP5 is part of the SNARE protein family, which is involved in vesicle fusion and recycling (54). VAMP5 is involved in intracellular transport including exocytosis, endocytosis and recycling of endosomes (55). These processes are closely related to autophagic pathways, which have been implicated in the pathogenesis of sarcoidosis (56). Identification of these genes adds to the knowledge about the genetics and pathogenesis of sarcoidosis and opens avenues for further research into these proteins.
IPA analysis of datasets analysed in this study predicted EIF2 signalling to be downregulated. The EIF2 signalling cascade is involved in autophagy, protein translation and cell survival as well as the mammalian target of rapamycin (mTOR) pathway (57, 58). Recently, the mTOR pathway gained much attention in sarcoidosis (59–61), after the finding that constitutive activity of mTORC1 causes formation of granulomas (62). In this regard, Gupta and colleagues successfully treated a patient with pulmonary sarcoidosis with mTOR inhibitor sirolimus (63). Moreover, our tissue-specific analysis showed PI3K in the connectivity network, suggesting that the PI3K/mTOR pathway is involved only in affected tissues. Another interesting finding in IPA analysis was the upregulation of neuro-inflammation signalling pathway. Small fiber neuropathy is observed in about 30% of patients with systemic sarcoidosis (64), in which circulating inflammatory and neurotoxic cytokines may be involved (65). Recently, the upregulation of the neuro-inflammatory response was found by another group studying the sarcoidosis transcriptome and proteome (66). This interesting study is a meta-analysis as well, however, their strategy differed significantly from ours as they performed meta-analysis on the common pathways rather than DEGs. Moreover, recently two other meta-analyses on the transcriptome of sarcoidosis have been published (67, 68). These studies used only 11 and 13 studies respectively, excluding many relevant studies in their analyses. Therefore, our study is the largest systematic meta-analysis to identify robust DEGs which can be of value as biomarkers for sarcoidosis.
Interestingly, insulin was another hub in the connectivity network generated by IPA. Sarcoidosis patients are at increased risk for developing type 2 diabetes (T2D) (69). Much is unknown about this association, however, chronic inflammation and increased secretion of cytokines might predispose sarcoidosis patients to develop T2D (70). The IFN-γ/STAT1 upregulation, as found in our study, could be linked to insulin resistance in adipocytes through multiple mechanisms, including downregulation of the insulin receptor and glucose transporter type 4 (71). Additionally, STAT1 expression in white adipose tissue is elevated in prediabetic patients and STAT1 levels are positively correlated with plasma glucose (72). Together, these data suggest an important role for IFN-γ/STAT1 signalling in T2D observed in sarcoidosis patients, that is further exacerbated by steroid treatment and warrants attention from clinicians.
Finally, we looked for a tissue-specific gene signature in sarcoidosis and found MMP12 upregulated in most tissue-specific datasets. MMP12 is an elastase enzyme predominately produced by M2 tissue macrophages (73), those aggregate and form the characteristic multinucleated giant cells as seen in granulomas (74). Involvement of MMP12 in sarcoidosis specially in granuloma progression has been reported previously (27, 75). In our study, MMP12 was only differentially expressed at tissue sites, which is highly suggestive for its crucial role in granuloma formation. CXCR6 was also tissue-specific in our analysis, and this gene has been found to be expressed in Th1 cells surrounding the central core of sarcoidosis granulomas (76). Therefore, tissue-specific DEGs like MMP12 and CXCR6, or potentially its ligand CXCL16, could be interesting therapeutic targets for sarcoidosis lesions.
Among 22 datasets included in this meta-analysis, we were limited with respect to sample size, variable tissues and cell types as well as different technological platforms. Additionally, sarcoidosis patients can differ substantially in clinical presentation, disease progression and treatment response. Studies have shown distinct gene expression profiles between self-limiting sarcoidosis and progressive sarcoidosis (37, 77). Unfortunately, clinical information such as age, sex, ethnicity, treatment regimen, and disease activity were poorly reported by most studies. To appropriately circumvent these limitations, we opted for an iterative and systematic approach, through which we observed highly consistent DEGs and pathways in sarcoidosis despite this variability among studies. Furthermore, we were not able to adjust for other confounding factors such as lymphopenia, which is often observed in sarcoidosis patients and associated with disease activity (78). Attention should be paid to treatment regime and disease activity. Eleven of the 22 included datasets contained at least one sample that used immunosuppressive therapy, whereas three datasets excluded patients who were on immunosuppression. We did not observe major differences in DEGs between these datasets, but it is known that immunosuppression can alter gene expression in disease (79). Appropriate studies with disease endotyping are needed to study and identify potential biomarkers stratifying sarcoidosis subgroups leading to a precision-medicine approach (80). Finally, only eight of the 22 studies investigated here were derived from target tissues, highlighting the need for more studies investigating tissue-specific signatures to gain more insight in the genes and pathways involved in granuloma formation. Whether the described genes in this study represent activation or perpetuation of the disease needs further exploration.
In this meta-analysis study, 22 sarcoidosis gene expression datasets were systematically and uniformly assessed to identify DEGs and their signalling pathways. Integration of the results from individual datasets revealed a number of novel candidate genes (i.e., GBPs, VAMP5 and WARS1) and pathways in addition to previously described DEGs in sarcoidosis. Meta-analysis identified a robust and compact gene signature that points towards altered IFN-JAK-STAT1 signalling in sarcoidosis. Our findings add to the emerging evidence to employ JAK inhibitors as a targeted treatment in sarcoidosis patients. More strikingly, the DEGs found in our meta-analysis can further be explored to develop genomic-derived biomarkers for sarcoidosis. We found tissue-specific signature of genes like MMP12, CXCR6, and SNTB2 suggesting their pathways are likely to be involved in granuloma formation and progression and could eventually be potential therapeutic targets for sarcoidosis. Clinical manifestation still remains a challenge with respect to disease activity and progression, which warrants the need for further transcriptomic studies with endotyping investigating pulmonary phenotypes and immune responses.
The original contributions presented in the study are included in the article/Supplementary material, further inquiries can be directed to the corresponding author.
Ethical approval was not required for the studies involving humans because the current study uses existing publicly available data from gene expression studies, which obtained consent and ethical approval. No new data was generated in this study. Ethical approval was therefore not required. The studies were conducted in accordance with the local legislation and institutional requirements. The human samples used in this study were acquired from published publicly available datasets. Written informed consent to participate in this study was not required from the participants or the participants’ legal guardians/next of kin in accordance with the national legislation and the institutional requirements.
RW: Writing – review & editing, Writing – original draft, Methodology, Investigation, Formal analysis, Data curation, Conceptualization. HS: Writing – review & editing, Writing – original draft, Investigation. SS: Writing – review & editing, Writing – original draft, Investigation, Formal analysis, Data curation. WD: Writing – review & editing, Writing – original draft, Investigation. HI: Writing – review & editing, Writing – original draft, Investigation. VD: Writing – review & editing, Writing – original draft, Investigation. PD: Writing – review & editing, Writing – original draft, Investigation. PH: Writing – review & editing, Writing – original draft, Investigation, Conceptualization. PS: Writing – review & editing, Writing – original draft, Investigation, Formal analysis, Data curation, Conceptualization.
The author(s) declare financial support was received for the research, authorship, and/or publication of this article. The current study was supported by the European Union’s Horizon 2020 research and innovation program under grant agreement no. 779295 (ImmunAID—Immunome project consortium for AutoInflammatory Disorders).
The authors declare that the research was conducted in the absence of any commercial or financial relationships that could be construed as a potential conflict of interest.
All claims expressed in this article are solely those of the authors and do not necessarily represent those of their affiliated organizations, or those of the publisher, the editors and the reviewers. Any product that may be evaluated in this article, or claim that may be made by its manufacturer, is not guaranteed or endorsed by the publisher.
The Supplementary material for this article can be found online at: https://www.frontiersin.org/articles/10.3389/fmed.2024.1381031/full#supplementary-material
1. Drent, M, Crouser, ED, and Grunewald, J. Challenges of sarcoidosis and its management. N Engl J Med. (2021) 385:1018–32. doi: 10.1056/NEJMra2101555
2. Hunninghake, GW, Costabel, U, Ando, M, Baughman, R, Cordier, JF, du Bois, R, et al. ATS/ERS/WASOG statement on sarcoidosis. American Thoracic Society/European Respiratory Society/world Association of Sarcoidosis and other granulomatous disorders. Sarcoidosis Vasc Diffuse Lung Dis. (1999) 16:149–73.
3. Crouser, ED, Maier, LA, Wilson, KC, Bonham, CA, Morgenthau, AS, Patterson, KC, et al. Diagnosis and detection of sarcoidosis. An official American Thoracic Society clinical practice guideline. Am J Respir Crit Care Med. (2020) 201:e26–51. doi: 10.1164/rccm.202002-0251ST
4. Iannuzzi, MC, Rybicki, BA, and Teirstein, AS. Sarcoidosis. N Engl J Med. (2007) 357:2153–65. doi: 10.1056/NEJMra071714
5. Gupta, R, Judson, MA, and Baughman, RP. Management of Advanced Pulmonary Sarcoidosis. Am J Respir Crit Care Med. (2022) 205:495–506. doi: 10.1164/rccm.202106-1366CI
6. Chen, ES, and Moller, DR. Etiologies of sarcoidosis. Clin Rev Allergy Immunol. (2015) 49:6–18. doi: 10.1007/s12016-015-8481-z
7. McGonagle, D, and McDermott, MF. A proposed classification of the immunological diseases. PLoS Med. (2006) 3:e297. doi: 10.1371/journal.pmed.0030297
8. Levin, AM, Adrianto, I, Datta, I, Iannuzzi, MC, Trudeau, S, Li, J, et al. Association of HLA-DRB1 with sarcoidosis susceptibility and progression in African Americans. Am J Respir Cell Mol Biol. (2015) 53:206–16. doi: 10.1165/rcmb.2014-0227OC
9. Prasse, A, Georges, CG, Biller, H, Hamm, H, Matthys, H, Luttmann, W, et al. Th1 cytokine pattern in sarcoidosis is expressed by bronchoalveolar CD4+ and CD8+ T cells. Clin Exp Immunol. (2000) 122:241–8. doi: 10.1046/j.1365-2249.2000.01365.x
10. Grunewald, J, Grutters, JC, Arkema, EV, Saketkoo, LA, Moller, DR, and Muller-Quernheim, J. Sarcoidosis. Nat Rev Dis Primers. (2019) 5:45. doi: 10.1038/s41572-019-0096-x
11. Scheinberg, M, Maluf, F, and Wagner, J. Steroid-resistant sarcoidosis treated with baricitinib. Ann Rheum Dis. (2020) 79:1259–60. doi: 10.1136/annrheumdis-2020-217271
12. Friedman, MA, Le, B, Stevens, J, Desmarais, J, Seifer, D, Ogle, K, et al. Tofacitinib as a steroid-sparing therapy in pulmonary sarcoidosis, an open-label prospective proof-of-concept study. Lung. (2021) 199:147–53. doi: 10.1007/s00408-021-00436-8
13. Edgar, R, Domrachev, M, and Lash, AE. Gene expression omnibus: NCBI gene expression and hybridization array data repository. Nucleic Acids Res. (2002) 30:207–10. doi: 10.1093/nar/30.1.207
14. Gautier, L, Cope, L, Bolstad, BM, and Irizarry, RA. Affy—analysis of Affymetrix GeneChip data at the probe level. Bioinformatics. (2004) 20:307–15. doi: 10.1093/bioinformatics/btg405
15. Ritchie, ME, Phipson, B, Wu, D, Hu, Y, Law, CW, Shi, W, et al. Limma powers differential expression analyses for RNA-sequencing and microarray studies. Nucleic Acids Res. (2015) 43:e47. doi: 10.1093/nar/gkv007
16. Love, MI, Huber, W, and Anders, S. Moderated estimation of fold change and dispersion for RNA-seq data with DESeq2. Genome Biol. (2014) 15:550. doi: 10.1186/s13059-014-0550-8
17. Leek, JT, Johnson, WE, Parker, HS, Jaffe, AE, and Storey, JD. The SVA package for removing batch effects and other unwanted variation in high-throughput experiments. Bioinformatics. (2012) 28:882–3. doi: 10.1093/bioinformatics/bts034
18. McCarthy, DJ, and Smyth, GK. Testing significance relative to a fold-change threshold is a TREAT. Bioinformatics. (2009) 25:765–71. doi: 10.1093/bioinformatics/btp053
19. Kramer, A, Green, J, Pollard, J, and Tugendreich, S. Causal analysis approaches in ingenuity pathway analysis. Bioinformatics. (2014) 30:523–30. doi: 10.1093/bioinformatics/btt703
20. Edgington, ES. An additive method for combining probability values from independent experiments. J Psychol. (1972) 80:351–63. doi: 10.1080/00223980.1972.9924813
22. Mostafavi, S, Yoshida, H, Moodley, D, LeBoite, H, Rothamel, K, Raj, T, et al. Parsing the interferon transcriptional Network and its disease associations. Cell. (2016) 164:564–78. doi: 10.1016/j.cell.2015.12.032
23. Bignon, A, Regent, A, Klipfel, L, Desnoyer, A, de la Grange, P, Martinez, V, et al. DUSP4-mediated accelerated T-cell senescence in idiopathic CD4 lymphopenia. Blood. (2015) 125:2507–18. doi: 10.1182/blood-2014-08-598565
24. Blankley, S, Graham, CM, Turner, J, Berry, MP, Bloom, CI, Xu, Z, et al. The transcriptional signature of active tuberculosis reflects symptom status in extra-pulmonary and pulmonary tuberculosis. PLoS One. (2016) 11:e0162220. doi: 10.1371/journal.pone.0162220
25. Bloom, CI, Graham, CM, Berry, MP, Rozakeas, F, Redford, PS, Wang, Y, et al. Transcriptional blood signatures distinguish pulmonary tuberculosis, pulmonary sarcoidosis, pneumonias and lung cancers. PLoS One. (2013) 8:e70630. doi: 10.1371/journal.pone.0070630
26. Chai, Q, Lu, Z, Liu, Z, Zhong, Y, Zhang, F, Qiu, C, et al. Lung gene expression signatures suggest pathogenic links and molecular markers for pulmonary tuberculosis, adenocarcinoma and sarcoidosis. Commun Biol. (2020) 3:604. doi: 10.1038/s42003-020-01318-0
27. Crouser, ED, Culver, DA, Knox, KS, Julian, MW, Shao, G, Abraham, S, et al. Gene expression profiling identifies MMP-12 and ADAMDEC1 as potential pathogenic mediators of pulmonary sarcoidosis. Am J Respir Crit Care Med. (2009) 179:929–38. doi: 10.1164/rccm.200803-490OC
28. Gharib, SA, Malur, A, Huizar, I, Barna, BP, Kavuru, MS, Schnapp, LM, et al. Sarcoidosis activates diverse transcriptional programs in bronchoalveolar lavage cells. Respir Res. (2016) 17:93. doi: 10.1186/s12931-016-0411-y
29. Grayson, PC, Steiling, K, Platt, M, Berman, JS, Zhang, X, Xiao, J, et al. Defining the nasal transcriptome in granulomatosis with polyangiitis (Wegener’s). Arthritis Rheumatol. (2015) 67:2233–9. doi: 10.1002/art.39185
30. Judson, MA, Marchell, RM, Mascelli, M, Piantone, A, Barnathan, ES, Petty, KJ, et al. Molecular profiling and gene expression analysis in cutaneous sarcoidosis: the role of interleukin-12, interleukin-23, and the T-helper 17 pathway. J Am Acad Dermatol. (2012) 66:901–910.e2. doi: 10.1016/j.jaad.2011.06.017
31. Koth, LL, Solberg, OD, Peng, JC, Bhakta, NR, Nguyen, CP, and Woodruff, PG. Sarcoidosis blood transcriptome reflects lung inflammation and overlaps with tuberculosis. Am J Respir Crit Care Med. (2011) 184:1153–63. doi: 10.1164/rccm.201106-1143OC
32. Lepzien, R, Liu, S, Czarnewski, P, Nie, M, Osterberg, B, Baharom, F, et al. Monocytes in sarcoidosis are potent tumour necrosis factor producers and predict disease outcome. Eur Respir J. (2021) 58:2003468. doi: 10.1183/13993003.03468-2020
33. Maertzdorf, J, Weiner, J, Mollenkopf, HJ, Network, TB, Bauer, T, Prasse, A, et al. Common patterns and disease-related signatures in tuberculosis and sarcoidosis. Proc Natl Acad Sci USA. (2012) 109:7853–8. doi: 10.1073/pnas.1121072109
34. Prasse, A, Binder, H, Schupp, JC, Kayser, G, Bargagli, E, Jaeger, B, et al. BAL cell gene expression is indicative of outcome and airway basal cell involvement in idiopathic pulmonary fibrosis. Am J Respir Crit Care Med. (2019) 199:622–30. doi: 10.1164/rccm.201712-2551OC
35. Reichmann, MT, Tezera, LB, Vallejo, AF, Vukmirovic, M, Xiao, R, Reynolds, J, et al. Integrated transcriptomic analysis of human tuberculosis granulomas and a biomimetic model identifies therapeutic targets. J Clin Invest. (2021) 131:148136. doi: 10.1172/JCI148136
36. Rosenbaum, JT, Choi, D, Harrington, CA, Wilson, DJ, Grossniklaus, HE, Sibley, CH, et al. Gene expression profiling and heterogeneity of nonspecific orbital inflammation affecting the lacrimal gland. JAMA Ophthalmol. (2017) 135:1156–62. doi: 10.1001/jamaophthalmol.2017.3458
37. Zhou, T, Zhang, W, Sweiss, NJ, Chen, ES, Moller, DR, Knox, KS, et al. Peripheral blood gene expression as a novel genomic biomarker in complicated sarcoidosis. PLoS One. (2012) 7:e44818. doi: 10.1371/journal.pone.0044818
38. Rutherford, RM, Staedtler, F, Kehren, J, Chibout, SD, Joos, L, Tamm, M, et al. Functional genomics and prognosis in sarcoidosis--the critical role of antigen presentation. Sarcoidosis Vasc Diffuse Lung Dis. (2004) 21:10–8.
39. Sharma, SM, Choi, D, Planck, SR, Harrington, CA, Austin, CR, Lewis, JA, et al. Insights in to the pathogenesis of axial spondyloarthropathy based on gene expression profiles. Arthritis Res Ther. (2009) 11:R168. doi: 10.1186/ar2855
40. Yoshioka, K, Sato, H, Kawasaki, T, Ishii, D, Imamoto, T, Abe, M, et al. Transcriptome analysis of peripheral blood mononuclear cells in pulmonary sarcoidosis. Front Med. (2022) 9:822094. doi: 10.3389/fmed.2022.822094
41. Ascoli, C, Schott, CA, Huang, Y, Turturice, BA, Wang, W, Ecanow, N, et al. Altered transcription factor targeting is associated with differential peripheral blood mononuclear cell proportions in sarcoidosis. Front Immunol. (2022) 13:848759. doi: 10.3389/fimmu.2022.848759
42. Schupp, JC, Vukmirovic, M, Kaminski, N, and Prasse, A. Transcriptome profiles in sarcoidosis and their potential role in disease prediction. Curr Opin Pulm Med. (2017) 23:487–92. doi: 10.1097/MCP.0000000000000403
43. Cahan, P, Rovegno, F, Mooney, D, Newman, JC, St Laurent, G, and McCaffrey, TA. Meta-analysis of microarray results: challenges, opportunities, and recommendations for standardization. Gene. (2007) 401:12–8. doi: 10.1016/j.gene.2007.06.016
44. Chakravarty, SD, Harris, ME, Schreiner, AM, and Crow, MK. Sarcoidosis triggered by interferon-Beta treatment of multiple sclerosis: a case report and focused literature review. Semin Arthritis Rheum. (2012) 42:206–12. doi: 10.1016/j.semarthrit.2012.03.008
45. Kato, A, Ishihara, M, and Mizuki, N. Interferon-induced sarcoidosis with uveitis as the initial symptom: a case report and review of the literature. J Med Case Rep. (2021) 15:568. doi: 10.1186/s13256-021-03181-x
46. Ngo, CC, and Man, SM. Mechanisms and functions of guanylate-binding proteins and related interferon-inducible GTPases: roles in intracellular lysis of pathogens. Cell Microbiol. (2017) 19:e12791. doi: 10.1111/cmi.12791
47. Kong, SL, Chui, P, Lim, B, and Salto-Tellez, M. Elucidating the molecular physiopathology of acute respiratory distress syndrome in severe acute respiratory syndrome patients. Virus Res. (2009) 145:260–9. doi: 10.1016/j.virusres.2009.07.014
48. Dubaniewicz, A, Typiak, M, Wybieralska, M, Szadurska, M, Nowakowski, S, Staniewicz-Panasik, A, et al. Changed phagocytic activity and pattern of Fcgamma and complement receptors on blood monocytes in sarcoidosis. Hum Immunol. (2012) 73:788–94. doi: 10.1016/j.humimm.2012.05.005
49. Talreja, J, Farshi, P, Alazizi, A, Luca, F, Pique-Regi, R, and Samavati, L. RNA-sequencing identifies novel pathways in sarcoidosis monocytes. Sci Rep. (2017) 7:2720. doi: 10.1038/s41598-017-02941-4
50. Kjellin, H, Silva, E, Branca, RM, Eklund, A, Jakobsson, PJ, Grunewald, J, et al. Alterations in the membrane-associated proteome fraction of alveolar macrophages in sarcoidosis. Sarcoidosis Vasc Diffuse Lung Dis. (2016) 33:17–28.
51. Silva, E, Souchelnytskyi, S, Kasuga, K, Eklund, A, Grunewald, J, and Wheelock, AM. Quantitative intact proteomics investigations of alveolar macrophages in sarcoidosis. Eur Respir J. (2013) 41:1331–9. doi: 10.1183/09031936.00178111
52. La Distia, NR, Sitompul, R, Bakker, M, Versnel, MA, Swagemakers, SMA, van der Spek, PJ, et al. Type 1 interferon-inducible gene expression in QuantiFERON gold TB-positive uveitis: a tool to stratify a high versus low risk of active tuberculosis? PLoS One. (2018) 13:e0206073. doi: 10.1371/journal.pone.0206073
53. Lee, HC, Lee, ES, Uddin, MB, Kim, TH, Kim, JH, Chathuranga, K, et al. Released Tryptophanyl-tRNA Synthetase stimulates innate immune responses against viral infection. J Virol. (2019) 93:e18. doi: 10.1128/JVI.01291-18
54. Hong, W. SNAREs and traffic. Biochim Biophys Acta. (2005) 1744:120–44. doi: 10.1016/j.bbamcr.2005.03.014
55. Tajika, Y, Takahashi, M, Khairani, AF, Ueno, H, Murakami, T, and Yorifuji, H. Vesicular transport system in myotubes: ultrastructural study and signposting with vesicle-associated membrane proteins. Histochem Cell Biol. (2014) 141:441–54. doi: 10.1007/s00418-013-1164-z
56. Pacheco, Y, Valeyre, D, El Jammal, T, Vallee, M, Chevalier, F, Lamartine, J, et al. Autophagy and Mitophagy-related pathways at the crossroads of genetic pathways involved in familial sarcoidosis and host-pathogen interactions induced by coronaviruses. Cells. (2021) 10:81995. doi: 10.3390/cells10081995
57. Wengrod, JC, Wang, D, Weiss, S, Zhong, H, Osman, I, and Gardner, LB. Phosphorylation of eIF2alpha triggered by mTORC1 inhibition and PP6C activation is required for autophagy and is aberrant in PP6C-mutated melanoma. Sci Signal. (2015) 8:e899. doi: 10.1126/scisignal.aaa0899
58. Wengrod, JC, and Gardner, LB. Cellular adaptation to nutrient deprivation: crosstalk between the mTORC1 and eIF2alpha signaling pathways and implications for autophagy. Cell Cycle. (2015) 14:2571–7. doi: 10.1080/15384101.2015.1056947
59. Crouser, ED, Locke, LW, Julian, MW, Bicer, S, Sadee, W, White, P, et al. Phagosome-regulated mTOR signalling during sarcoidosis granuloma biogenesis. Eur Respir J. (2021) 57:2002695. doi: 10.1183/13993003.02695-2020
60. Pacheco, Y, Lim, CX, Weichhart, T, Valeyre, D, Bentaher, A, and Calender, A. Sarcoidosis and the mTOR, Rac1, and autophagy triad. Trends Immunol. (2020) 41:286–99. doi: 10.1016/j.it.2020.01.007
61. Rastogi, R, Jiang, Z, Ahmad, N, Rosati, R, Liu, Y, Beuret, L, et al. Rapamycin induces mitogen-activated protein (MAP) kinase phosphatase-1 (MKP-1) expression through activation of protein kinase B and mitogen-activated protein kinase kinase pathways. J Biol Chem. (2013) 288:33966–77. doi: 10.1074/jbc.M113.492702
62. Linke, M, Pham, HT, Katholnig, K, Schnoller, T, Miller, A, Demel, F, et al. Chronic signaling via the metabolic checkpoint kinase mTORC1 induces macrophage granuloma formation and marks sarcoidosis progression. Nat Immunol. (2017) 18:293–302. doi: 10.1038/ni.3655
63. Gupta, N, Bleesing, JH, and McCormack, FX. Successful response to treatment with Sirolimus in pulmonary sarcoidosis. Am J Respir Crit Care Med. (2020) 202:e119–20. doi: 10.1164/rccm.202004-0914IM
64. Tavee, JO, Karwa, K, Ahmed, Z, Thompson, N, Parambil, J, and Culver, DA. Sarcoidosis-associated small fiber neuropathy in a large cohort: clinical aspects and response to IVIG and anti-TNF alpha treatment. Respir Med. (2017) 126:135–8. doi: 10.1016/j.rmed.2017.03.011
65. Basantsova, NY, Starshinova, AA, Dori, A, Zinchenko, YS, Yablonskiy, PK, and Shoenfeld, Y. Small-fiber neuropathy definition, diagnosis, and treatment. Neurol Sci. (2019) 40:1343–50. doi: 10.1007/s10072-019-03871-x
66. Bhargava, M, Liao, S-Y, Elliott, ED, Maier, LA, and Leach, SM. The landscape of transcriptomics and proteomics studies in sarcoidosis. ERJ Open Res. (2021) 8:00621–2021. doi: 10.1183/23120541.00621-2021
67. Jiang, Y, Jiang, D, Costabel, U, Dai, H, and Wang, C. A transcriptomics-based meta-analysis identifies a cross-tissue signature for sarcoidosis. Front Med. (2022) 9:960266. doi: 10.3389/fmed.2022.960266
68. Duo, M, Liu, Z, Li, P, Wang, Y, Zhang, Y, Weng, S, et al. Integrative bioinformatics analysis to explore a robust diagnostic signature and landscape of immune cell infiltration in sarcoidosis. Front Med (Lausanne). (2022) 9:942177. doi: 10.3389/fmed.2022.942177
69. Entrop, JP, Kullberg, S, Grunewald, J, Eklund, A, Brismar, K, and Arkema, EV. Type 2 diabetes risk in sarcoidosis patients untreated and treated with corticosteroids. ERJ Open Res. (2021) 7:00028–2021. doi: 10.1183/23120541.00028-2021
70. Donath, MY, and Shoelson, SE. Type 2 diabetes as an inflammatory disease. Nat Rev Immunol. (2011) 11:98–107. doi: 10.1038/nri2925
71. McGillicuddy, FC, Chiquoine, EH, Hinkle, CC, Kim, RJ, Shah, R, Roche, HM, et al. Interferon gamma attenuates insulin signaling, lipid storage, and differentiation in human adipocytes via activation of the JAK/STAT pathway. J Biol Chem. (2009) 284:31936–44. doi: 10.1074/jbc.M109.061655
72. Cox, AR, Chernis, N, Bader, DA, Saha, PK, Masschelin, PM, Felix, JB, et al. STAT1 dissociates adipose tissue inflammation from insulin sensitivity in obesity. Diabetes. (2020) 69:2630–41. doi: 10.2337/db20-0384
73. Nakagomi, D, Suzuki, K, Meguro, K, Hosokawa, J, Tamachi, T, Takatori, H, et al. Matrix metalloproteinase 12 is produced by M2 macrophages and plays important roles in the development of contact hypersensitivity. J Allergy Clin Immunol. (2015) 135:1397–400. doi: 10.1016/j.jaci.2014.10.055
74. Locke, LW, Crouser, ED, White, P, Julian, MW, Caceres, EG, Papp, AC, et al. IL-13-regulated macrophage polarization during granuloma formation in an in vitro human sarcoidosis model. Am J Respir Cell Mol Biol. (2019) 60:84–95. doi: 10.1165/rcmb.2018-0053OC
75. Mohan, A, Neequaye, N, Malur, A, Soliman, E, McPeek, M, Leffler, N, et al. Matrix Metalloproteinase-12 is required for granuloma progression. Front Immunol. (2020) 11:553949. doi: 10.3389/fimmu.2020.553949
76. Agostini, C, Cabrelle, A, Calabrese, F, Bortoli, M, Scquizzato, E, Carraro, S, et al. Role for CXCR6 and its ligand CXCL16 in the pathogenesis of T-cell alveolitis in sarcoidosis. Am J Respir Crit Care Med. (2005) 172:1290–8. doi: 10.1164/rccm.200501-142OC
77. Lockstone, HE, Sanderson, S, Kulakova, N, Baban, D, Leonard, A, Kok, WL, et al. Gene set analysis of lung samples provides insight into pathogenesis of progressive, fibrotic pulmonary sarcoidosis. Am J Respir Crit Care Med. (2010) 181:1367–75. doi: 10.1164/rccm.200912-1855OC
78. Sweiss, NJ, Salloum, R, Gandhi, S, Alegre, ML, Sawaqed, R, Badaracco, M, et al. Significant CD4, CD8, and CD19 lymphopenia in peripheral blood of sarcoidosis patients correlates with severe disease manifestations. PLoS One. (2010) 5:e9088. doi: 10.1371/journal.pone.0009088
79. Northcott, M, Gearing, LJ, Bonin, J, Koelmeyer, R, Hoi, A, Hertzog, PJ, et al. Immunosuppressant exposure confounds gene expression analysis in systemic lupus erythematosus. Front Immunol. (2022) 13:964263. doi: 10.3389/fimmu.2022.964263
80. Karampitsakos, T, Juan-Guardela, BM, Tzouvelekis, A, and Herazo-Maya, JD. Precision medicine advances in idiopathic pulmonary fibrosis. EBioMedicine. (2023) 95:104766. doi: 10.1016/j.ebiom.2023.104766
Keywords: sarcoidosis, granuloma, gene expression, meta-analysis, IFN-JAK-STAT signalling
Citation: van Wijck RTA, Sharma HS, Swagemakers SMA, Dik WA, IJspeert H, Dalm VASH, van Daele PLA, van Hagen PM and van der Spek PJ (2024) Bioinformatic meta-analysis reveals novel differentially expressed genes and pathways in sarcoidosis. Front. Med. 11:1381031. doi: 10.3389/fmed.2024.1381031
Received: 02 February 2024; Accepted: 23 May 2024;
Published: 13 June 2024.
Edited by:
Miriana d’Alessandro, University of Siena, ItalyReviewed by:
Ourania Papaioannou, General University Hospital of Patras, GreeceCopyright © 2024 van Wijck, Sharma, Swagemakers, Dik, IJspeert, Dalm, van Daele, van Hagen and van der Spek. This is an open-access article distributed under the terms of the Creative Commons Attribution License (CC BY). The use, distribution or reproduction in other forums is permitted, provided the original author(s) and the copyright owner(s) are credited and that the original publication in this journal is cited, in accordance with accepted academic practice. No use, distribution or reproduction is permitted which does not comply with these terms.
*Correspondence: Peter J. van der Spek, cC52YW5kZXJzcGVrQGVyYXNtdXNtYy5ubA==
Disclaimer: All claims expressed in this article are solely those of the authors and do not necessarily represent those of their affiliated organizations, or those of the publisher, the editors and the reviewers. Any product that may be evaluated in this article or claim that may be made by its manufacturer is not guaranteed or endorsed by the publisher.
Research integrity at Frontiers
Learn more about the work of our research integrity team to safeguard the quality of each article we publish.