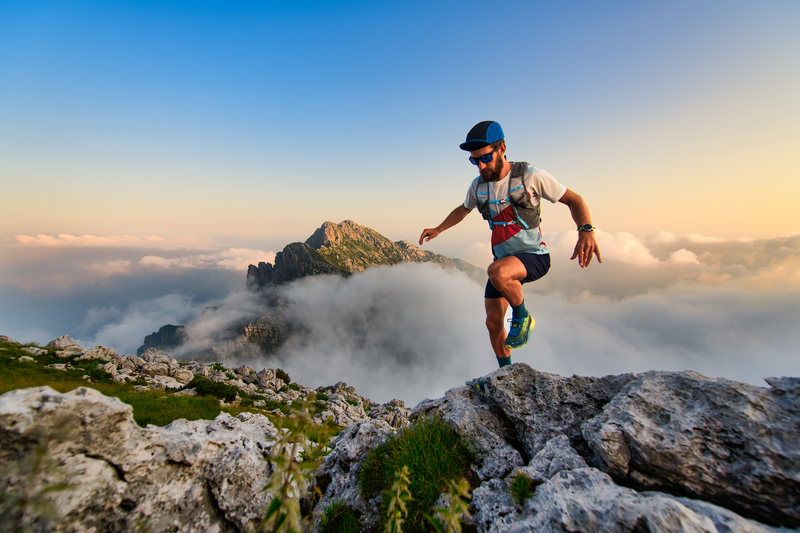
95% of researchers rate our articles as excellent or good
Learn more about the work of our research integrity team to safeguard the quality of each article we publish.
Find out more
EDITORIAL article
Front. Med. , 09 January 2024
Sec. Intensive Care Medicine and Anesthesiology
Volume 10 - 2023 | https://doi.org/10.3389/fmed.2023.1346070
This article is part of the Research Topic Clinical Application of Artificial Intelligence in Emergency and Critical Care Medicine, Volume IV View all 17 articles
Editorial on the Research Topic
Clinical application of artificial intelligence in emergency and critical care medicine, volume IV
Integrating artificial intelligence (AI) into the realm of emergency and critical care medicine marks a transformative stage in healthcare delivery. In Volume IV of the research compilation titled “Clinical application of artificial intelligence in emergency and critical care medicine,” a collection of 16 articles highlights the burgeoning intersection between advanced technology and acute medical interventions. This compilation looks at a spectrum of innovative applications, ranging from diagnostic support systems to predictive analytics, all poised to reshape the dynamics of emergency medical response and critical patient care.
Guiot et al. presented an interesting retrospective study on COVID-19 patients. Over the last few years, there have been millions of COVID-19 cases with many deaths (1). To help manage the load on radiologists, an artificial intelligence (AI) based analysis (CACOVID-CT) was implemented to evaluate the severity of the disease on the basis of CT chests performed on those patients. Progress in machine learning and artificial intelligence has led to the creation of tools that can augment the diagnostic skills of radiologists (2). The area of the lung affected by COVID-19 Affected Area (%AA) and CT severity score (total CT-SS) were quantified to help evaluate outcome and prognosis. It is interesting to note that both %AA and CT-SS had a high correlation with length of stay, risk for invasive ventilation, ICU admission, and death during hospital stay. It alleviated the workload of radiologists by measuring the severity of lung damage.
As the pandemic continued to grow, there was an increased number of COVID-19 patients with acute respiratory distress syndrome (ARDS) in the ICU (3). However, there was limited information about predictive studies of ARDS in those patients. Zhou et al. attempted to create predictive models to establish a correlation between ARDS and COVID-19. One hundred three critically ill COVID patients were included in the study, and the development of ARDS in patients admitted to ICU was the primary outcome. Based on convolutional neural network (CNN) and extreme gradient boosting (XGBoost), two predictive models were established. Out of 104, 23 (22.3%) of patients developed ARDS. In critically ill COVID-19 patients, an integrated deep-learning model can be helpful to predict ARDS.
As the healthcare industry embarks on a paradigm shift toward a more data-driven and technologically enhanced future, this volume is a comprehensive exploration of AI's profound impact in optimizing clinical decision-making, resource allocation, and patient outcomes within the high-stakes environments of emergency and critical care settings. Saqib et al. carried out a comprehensive search in PubMed, Google Scholar, PLOS One, and Scopus to develop an understanding of AI applications in critical illness in a narrative review. They concluded that it is vital to ensure that AI systems are made robust and reliable in the care of critically ill patients. Also, there should be transparent and comprehensible reasoning behind recommendations generated by AI. Quality control measures must be in place to ensure safety and effectiveness.
Nonvariceal upper gastrointestinal bleeding (NVUGIB) in patients with decompensated cirrhosis can be critically ill and has been associated with a higher rate of readmissions and mortality (4). For patients with NVUGIB, Ungureanu et al. developed an artificial neural network with mortality as the primary outcome. Over 1000 NVUGIB patients hospitalized were divided into training and testing groups in this retrospective study. Glasgow Blatchford (GBS), AIM65, and admission Rockall (Rock) are non-endoscopic risk scores used in the past. In the study, four machine learning algorithms, Quadratic Discriminant Analysis (QDA), logistic regression (LR), Linear Discriminant Analysis (LDA), and K-Nearest Neighbor (K-NN) were used with GBS, Rock, AIM65, and others. It was noted that the machine learning models had more accuracy in identifying patients with a higher mortality risk than the current risk scores. An accuracy of 98% was seen with K-NN classifier, proving that there is scope for using machine learning in NVUGIB patients to predict mortality.
In hospitals, length of stay (LOS) indicates the efficiency of management (5). Zeleke et al. attempted to compare and develop various models to estimate LOS and prolonged LOS in patients admitted through the emergency room. Six algorithms (Random Forest (RF), Support Vector Machines (SVM), Gradient Boosting (GB), AdaBoost, K-Nearest Neighbors (KNN), and logistic regression (LR) were used, and they analyzed a total of 12,858 patients. Out of them, 61% had a prolonged LOS. The models were evaluated using the Brier score with the area under the curve, sensitivity, accuracy, precision, specificity, and F1 score.GB algorithm best predicted the accuracy of prolonged LOS, and there was tremendous potential seen in the machine learning-based methods to assess for LOS. They also give insights to help understand the risks behind increased LOS. If combined with provider expertise, they can be used to make informed decisions.
In conclusion, examining “Clinical application of artificial intelligence in emergency and critical care medicine,” Volume IV, has provided a nuanced insight into the transformative potential of artificial intelligence within the critical domains of emergency and critical care medicine. It encapsulates a diverse array of AI applications, ranging from real-time diagnostics to prognostic modeling, each contributing to an evolving landscape where technology complements and enhances the capabilities of healthcare professionals. Although most topics have been covered in the collection of articles, we should be cognizant of the fact that technological advancement with AI does bring to light an acute need to address ethical considerations. Healthcare industry needs to protect the values of medicine and follow fundamental ethical principles. The future calls for papers for this special topic may consider including it. As we move forward, a collaborative effort between clinicians, technologists, and policymakers is crucial to harness the full potential of artificial intelligence for improving patient care in these critical settings.
GD: Conceptualization, Methodology, Visualization, Writing – original draft. ZZ: Conceptualization, Supervision, Writing – review & editing. HG: Conceptualization, Methodology, Visualization, Writing – review & editing. RK: Conceptualization, Project administration, Resources, Supervision, Writing – review & editing.
The author(s) declare that financial support was received for the research, authorship, and/or publication of this article. ZZ received funding from the China National Key Research and Development Program (Nos. 2023YFC3603104 and 2022YFC2504500), the Huadong Medicine Joint Funds of the Zhejiang Provincial Natural Science Foundation of China under Grant No. LHDMD24H150001, the Fundamental Research Funds for the Central Universities (226-2022-00148), National Natural Science Foundation of China (82272180), and the Project of Drug Clinical Evaluate Research of Chinese Pharmaceutical Association No. CPA-Z06-ZC-2021-004.
The authors declare that the research was conducted in the absence of any commercial or financial relationships that could be construed as a potential conflict of interest.
The author(s) declared that they were an editorial board member of Frontiers, at the time of submission. This had no impact on the peer review process and the final decision.
All claims expressed in this article are solely those of the authors and do not necessarily represent those of their affiliated organizations, or those of the publisher, the editors and the reviewers. Any product that may be evaluated in this article, or claim that may be made by its manufacturer, is not guaranteed or endorsed by the publisher.
1. Naik R, Avula S, Palleti SK, Gummadi J, Ramachandran R, Chandramohan D, et al. From emergence to endemicity: a comprehensive review of COVID-19. Cureus. (2023) 15:e48046. doi: 10.7759/cureus.48046
2. Grewal H, Dhillon G, Monga V, Sharma P, Buddhavarapu VS, Sidhu G, et al. Radiology gets chatty: the ChatGPT saga unfolds. Cureus. (2023) 15:e40135. doi: 10.7759/cureus.40135
3. Fan E, Beitler JR, Brochard L, Calfee CS, Ferguson ND, Slutsky AS, et al. COVID-19-associated acute respiratory distress syndrome: is a different approach to management warranted? Lancet Respir Med. (2020) 8:816–21. doi: 10.1016/S2213-2600(20)30304-0
4. Kruger AJ, Abougergi MS, Jalil S, Sobotka LA, Wellner MR, Porter KM, et al. Outcomes of nonvariceal upper gastrointestinal bleeding in patients with cirrhosis: a national analysis. J Clin Gastroenterol. (2023) 57:848–53. doi: 10.1097/MCG.0000000000001746
Keywords: artificial intelligence, prediction, critical care, machine learning, intensive care unit
Citation: Dhillon G, Zhang Z, Grewal H and Kashyap R (2024) Editorial: Clinical application of artificial intelligence in emergency and critical care medicine, volume IV. Front. Med. 10:1346070. doi: 10.3389/fmed.2023.1346070
Received: 28 November 2023; Accepted: 22 December 2023;
Published: 09 January 2024.
Edited by:
Gulzar H. Shah, Georgia Southern University, United StatesReviewed by:
Athanasios Chalkias, University of Pennsylvania, United StatesCopyright © 2024 Dhillon, Zhang, Grewal and Kashyap. This is an open-access article distributed under the terms of the Creative Commons Attribution License (CC BY). The use, distribution or reproduction in other forums is permitted, provided the original author(s) and the copyright owner(s) are credited and that the original publication in this journal is cited, in accordance with accepted academic practice. No use, distribution or reproduction is permitted which does not comply with these terms.
*Correspondence: Rahul Kashyap, a2FzaHlhcG1kQGdtYWlsLmNvbQ==
Disclaimer: All claims expressed in this article are solely those of the authors and do not necessarily represent those of their affiliated organizations, or those of the publisher, the editors and the reviewers. Any product that may be evaluated in this article or claim that may be made by its manufacturer is not guaranteed or endorsed by the publisher.
Research integrity at Frontiers
Learn more about the work of our research integrity team to safeguard the quality of each article we publish.