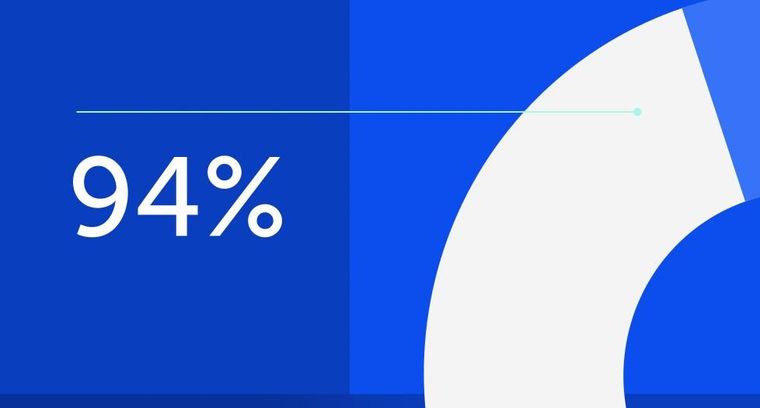
94% of researchers rate our articles as excellent or good
Learn more about the work of our research integrity team to safeguard the quality of each article we publish.
Find out more
REVIEW article
Front. Med., 27 November 2023
Sec. Regulatory Science
Volume 10 - 2023 | https://doi.org/10.3389/fmed.2023.1305756
This article is part of the Research TopicHealthcare in the age of sapient machines: physician decision-making autonomy faced with artificial intelligence. Ethical, deontological and compensatory aspectsView all 7 articles
Artificial intelligence (AI) in medicine is an increasingly studied and widespread phenomenon, applied in multiple clinical settings. Alongside its many potential advantages, such as easing clinicians’ workload and improving diagnostic accuracy, the use of AI raises ethical and legal concerns, to which there is still no unanimous response. A systematic literature review on medical professional liability related to the use of AI-based diagnostic algorithms was conducted using the public electronic database PubMed selecting studies published from 2020 to 2023. The systematic review was performed according to 2020 PRISMA guidelines. The literature review highlights how the issue of liability in case of AI-related error and patient’s damage has received growing attention in recent years. The application of AI and diagnostic algorithm moreover raises questions about the risks of using unrepresentative populations during the development and about the completeness of information given to the patient. Concerns about the impact on the fiduciary relationship between physician and patient and on the subject of empathy have also been raised. The use of AI in medical field and the application of diagnostic algorithms introduced a revolution in the doctor–patient relationship resulting in multiple possible medico-legal consequences. The regulatory framework on medical liability when AI is applied is therefore inadequate and requires urgent intervention, as there is no single and specific regulation governing the liability of various parties involved in the AI supply chain, nor on end-users. Greater attention should be paid to inherent risk in AI and the consequent need for regulations regarding product safety as well as the maintenance of minimum safety standards through appropriate updates.
The application of artificial intelligence (AI) in medical field represents an emerging reality and the use of machinery that assist the professional operators both in the executive and in the diagnostic phases is increasingly frequent in hospitals (1). AI systems are currently destined to intervene on the treatment process both in the diagnostic and therapeutic phase, allowing the healthcare professional to reach, on the one hand, more certain and precise diagnoses, and on the other, surgical therapies, more effective and less invasive (2). The usefulness of AI has been described in radiology, because of its potential to recognize complex patterns and to provide quantitative assessments, and in oncology, as improving the prediction of outcomes, and nowadays its application outspreads to a large part of clinical settings (3, 4). Beside clinical advantages, the use of AI through risk stratification models can be useful to optimize resource plan and allocation (5). In the context of COVID-19 pandemic, opportunities to the AI research community emerged, as evidenced by the speed of production and dissemination of scientific works on this issue (6). Currently, the advantages of AI systems in the patient’s healthcare are widely affirmed, whereas the legal implications are still discussed (7). Since operators are not allowed to understand and verify the logical processes which drive the machine to the results, human–machine interaction in actions and omissions, especially when autonomous choices are taken by AI systems, poses relevant issues of liability in the event of damage to third parties. New technological approaches introduce new realities that might be unlikely to fit within the solid edges of the current law.
In this context, a comprehensive trace of present views about critical issues and in particular about liability related to the application of AI algorithms is essential in order to consider possible solutions.
In May 2023, one of the authors (CC) performed a review of the literature using the public electronic database PubMed. To ensure that no studies were missed, initially no temporal limits were set. The following search phrase was used: “medical liability diagnostic algorithm.” The research returned 96 articles. The same author repeated the review in June 2023, using the same search phrase, and found 97 articles.
Inclusion criteria: articles published between 2020 and 2023, dealing with critical issues, in particular liability, related to the use of AI and AI algorithms in medicine were included.
Exclusion criteria: articles not written in English were excluded.
The study selection was performed basing on titles and on abstracts. Overall, 29 articles were sought for retrieval. A full-text reading of retrieved articles was performed. After excluding articles whose full texts did not fulfill the abovementioned criteria, 23 articles were finally included in the review.
The number of articles included and excluded was registered in a PRISMA flow chart (Figure 1).
Figure 1. PRISMA flow-chart adapted from Page et al. (45).
Data concerning advantages of AI, critical issues and liability issue were collected and summarized in Table 1 and Figures 2, 3.
Figure 2. Graphical representation of the pros and cons of using artificial intelligence in diagnostic pathways (created with BioRender.com).
Figure 3. Possible approaches to liability issue (created with BioRender.com).
Most of included articles consist in perspectives articles and reviews, whose construction in most of cases do not meet the criteria of systematic review (n = 1 systematic review, n = 1 scoping review). N = 2 surveys are included in the study.
Improvements in diagnostic accuracy and in personalized medicine represent frequently reported advantages of AI use in medicine. With regard to accuracy, in cardiovascular field, Chung et al. (8) report that “the use of AI-ECG algorithms for rhythm identification and ECG interpretation can be more accurate in interpretation” than current ECG software, and also affirm that AI-based ECG analysis has been proposed as an accurate screening tool in valvular disease field. In breast disease and cancer care area, the use of AI is thought to lead to rapid diagnosis and more detailed evaluation (9). It is important to note, with regard to computer-aided detection (CAD) software that, according to Mezrich (10, 11), these can increase radiologists’ accuracy and fastness, but they act as a “second set of eyes.” Consistently, Vedantham et al. (12) affirm that it is more likely that AI will be used as a second reader, and Harvey and Gowda (13) reports the greater effectiveness of algorithms when subject to human oversight. AI technologies should indeed be considered as supplements to radiologist practice, increasing physicians’ performance and reducing the scope for human errors (13); clinical decision support applications represent tools which help in diagnosis instead of substitutes of clinicians (14).
In addition to the clinical setting, the use of AI could assist surgeons during intervention (15) and lead to more precise and minimally invasive surgical techniques (16).
The reduction of health professionals’ workload represents another potential advantage of AI (12, 17) and, in particular, in radiology field, Vedantham et al. (12) highlight the potential of AI to improve the timeliness of care through its application in triage phase and in prioritizing the reading list.
The topic of time emerges also from the surveys of Pecqueux et al. (16), in terms of reduction of time spent by specialists on monotonous tasks, and of Emiroglu et al. (9), in terms of possibility to get to a rapid diagnosis and to examine a larger number of patients. Moreover, AI may filter out non-complex cases letting a greater focus of specialists on more challenging cases (18).
AI advantages include also the better epidemiological risk assessment of diseases (19) and the early identification of at-risk patients (20). Beside patients care, AI use is supposed to lower costs (21).
Algorithms’ biases and privacy represent important critical issues of AI use in medicine.
With reference to algorithms using supervised training, Vedantham et al. (12) observe that results may be unreliable when the training phase of the algorithm did not include the encountered data (so-called out of distribution data); related to this aspect, López et al. (17) note that inequalities in provision of healthcare services and low health insurance coverage in low-middle income countries result in a lack of data of certain patients group. The development of predictive AI models which were trained with data that do not reflect the context of use of the algorithm represent the so-called “contextual bias” (17). As consequence, despite the potential to improve the access to care (21, 22), AI algorithms may amplify or create health disparities among marginalized groups (23), augment racial and demographic disparities (13), and exacerbate inequities in health outcomes (18).
Other critical issues deal with the impact on traditional doctor–patient relationship, which dynamic based on a fiduciary model will be challenged (8): patients’ care do not only include the diagnosis but also a “holistic approach with human touch” (22), and the use of AI may result in a low ability to empathize with patients (16). Moreover, the use of AI could result in a less rely on history and medical examination, with a possible decrease in quality of medical care: this also raises questions about legal liability in case of diagnostic errors due to the lack of physical examination (24). Bhardwaj (1) excludes that AI and machine learning will ever substitute “an astute and empathetic bedside clinician.” The topic of substitution relates with another critical issue, consisting in the replacement of doctors’ job and job losses (9–11, 21, 22). The use of AI could moreover affect young doctors’ motivation to try a career as radiologist, with a consequent risk of specialists’ lack, and cause a decrease of training opportunities (25). Further, less experienced clinicians may completely trust the diagnosis of AI algorithms (8).
Another main critical issue is the “black box” phenomenon, that is the “inability to fully understand an AI’s decision-making process and the inability to predict the AI’s decisions or outputs” (26): opacity in decision-making is described (27), and the provision of sufficient evidence about the design, the testing and evaluation can be difficult also for software developers (28). The use of black box systems also raises questions about patients with referral to the principle of autonomy and informed consent (26).
The need to obtain the informed consent before incorporating AI use into patients’ care is highlighted by Mezrich (11), who also highlights the importance to disclose the findings of computer aided detection applications in reports, explaining the reasons of an eventual disagreement with them. According to Jobson et al. (28), clinicians should inform patients of their intention to use AI, and patients should be sufficiently informed to understand risk, benefits and limitations of AI software and give consent to their use. The providing of inaccurate information to patients and clinicians about risks of AI algorithms may indeed represent a breach of the duty of care (28), and adequacy of information provided to users is crucial in judgments (15). However, referring to information, Harvey and Gowda (14) wonders what exactly should be disclosed to patient.
Other critical issues about the use of AI in medicine consist in privacy (1, 8, 11, 13, 17–20, 22, 24, 28, 29) and cybersecurity (18, 29): algorithms’ efficacy is based on large data and some of them may impact on patients’ privacy (22).
Liability in case of AI errors is a main issue and, despite the reviewed articles discuss multiple liability theories in relation to AI use, a unanimous and definitive answer to this issue currently do not exist.
According to Mezrich (11), since AI represents a new technology, the tort law applicable to it is not yet well developed: in case of suit for malpractice, a breach of duty of care and a deviation from the standard of care is required, and, in the context of AI, negligence might derive from a failure in programming, in supervision, from actions of physicians or algorithm itself (11). In case of failure to use or of reliance on AI medical devices, Lang et al. (27) observe that the fault could still be present if best medical practice at that time would have suggested a different approach. Nevertheless, apportioning liability may be difficult especially when using algorithms developed through neural networks, which represent a black box for both the manufacturers and the physicians (24).
Abràmoff et al. (21) note that creators of autonomous AI products should be liable for harms produced by the device if it was used properly and on label, maintaining the responsibility for proper use of the device with the providers; however, in case of assistive AI, in which the clinicians are capable of an independent evaluation of AI outputs, these latter would remain liable. Similarly, Channa et al. (20) state that if autonomous AI is used without specialists through test administration by the primary care physician, who does not have specialized knowledge, this should not be liable for incorrect results and AI creators or the selling company should assume medical liability.
Manufacturing companies should clearly provide the indications and possible adverse effects in using an AI algorithm (8), and, according to Bazoukis et al. (18), if there are potential adverse events/system failures attributable to the use of algorithms, the developers of augmented intelligence should report it and investigate outcomes, narrowing down clinicians’ responsibility to the “labeled” use of algorithms.
Furthermore, defining the degree of control is of primary importance when discussing liability issue: according to Sung and Poon (15), when machines, based on self-learned algorithm, achieve diagnosis without need of clinician’s approval, liability should at least partially be considered of the AI developer; if fully automated surgery is used, events produced by defects in configuration or design would probably be a responsibility of developers in a product liability claim. The same authors, however, affirm that the developers should not be considered liable just because their AI devices are not able to prevent harm in all instances (15). Even Mezrich (10) observes that the legal handling of AI will be based on the degree of autonomy of the software: when AI is used only as a decision support, the radiologist who makes the final determination bears the liability risk. Differently, if AI algorithm acts as a subordinate of the radiologist, the doctrine of vicarious liability could be applied (10). The model of vicarious liability, which provides that the negligence of an assistant is attributed to the supervisor (“respondeat superior”), is discussed by multiple authors (10, 11, 13, 14, 25). According to Mezrich (10, 11), as an AI algorithm which acts autonomously could be considered analogous to an employee of a facility, its negligence could be attributed to its supervising radiologist or to the institution; according to Harvey and Gowda (13) in clinical settings, hospitals, and physician groups would be responsible for the malpractice of their employees’ use of AI programs. Neri et al. (25) hypothesize an AI subjected to a vicarious civil liability, “written in the software and for which the producers must guarantee the users, so that they can use AI reasonably and with a human-controlled automation.”
Another discussed model consists in the product liability, which requires the existence of a defect in the product and provides that the manufacturer of the defective product is presumed to be responsible of this latter (27): according to Mezrich (10, 11) this model could be obstacled since the original developed algorithm may not be identical to the one that causes the harm, as it improves itself over the time. While explaining this model, Mezrich (10, 11) refers also to the learned intermediary exception, according to which a radiologist would be responsible for liability if he/she has had the chance to review the report and detect errors before its releasing and before the patient was injured. The same author observes that additional aspects of the product liability may derive from the development of AI software by an external vendor and its training “in-house” by a radiology department: in this case, enterprise liability would apply, with a consequent possible liability also of the customizing facility. With reference to the learned intermediary doctrine, Nitiéma et al. (24) note that patients are prevented from directly suing medical device manufacturer and the prescriber represent the end user of the device.
Nitiéma et al. (24) highlight that one proposed legal solution to liability issue consist in the conferral of personhood to AI devices, that would result in direct lawsuits against them in case of malpractice. The conferral of personhood to AI algorithms is also considered by Mezrich (10, 11). Moreover, Mezrich (10, 11) refers to the model of the vaccine injury compensation program, as a possible approach. With regard to AI applications in surgery, a cooperative effort of surgeons and assistive AI systems is hypothesized by Pecqueux et al. (16).
Chan (26), after deeply analyzing different theories of liability, proposes that physician, manufacturers and hospitals are considered a common enterprise for the purposes of liability: such approach “shift away from individualistic notions of responsibility, embodied by negligence and products liability, toward a more distributed conception.” The same author affirms that “a common enterprise strict liability approach would create strong incentives for the relevant actors to take care.”
Artificial intelligence refers to software or programs able to perform operations similar to the human activity of learning and decision-making through the use of technologies based on processes of machine learning, deep learning, and neural networks (30). The use of AI in medicine today represents an emerging reality with increasingly clear and secure lines of development. If the advantages that the contribution of automated medicine triggers in the patient’s healthcare and protection system are certain, the implications on a legal and liability level that derive from AI tools are less certain (7). At the root of the problem there are first of all the characteristics of the tool, which does not allow the operator to access the processes through which it achieves the desired result (31). When analyzing the nature of the AI background, it is necessary to keep in mind a gradual transition that it is already making in many areas of its use, that from automation to autonomy: while the automaton performs controlled and controlled by humans (so-called machine learning), the autonomous AI system, or with an increasingly greater degree of autonomy, is able to process the data it collects in a completely independent way, and to act in the context that surrounds it by system that data. The result is a behavior that, net of a desired result achieved, is not knowable by man, not even by the one who produced it (so-called deep learning). The processes that the machine puts in place, in fact, are difficult to identify ex post, as well as ex ante, in the sense that the operator can count on the relative certainty of the result that the machine will complete, but will not be able to identify the “how” of that procedure. The result is a system characterized by complexity, incompleteness, opacity, unpredictability, openness and vulnerability. These characteristics undermine the current models of responsibility, in particular, those that are based on the criterion of imputation of fault and on its identification for the purposes of liability and compensation.
Literature review highlights that issue of liability in case of AI-related error has received growing attention in recent years, nevertheless this issue is mainly discussed in position papers and non-systematic reviews, and more in-depth analysis are scarce. In line with the above, it has emerged that difficulties in apportioning liability are present especially when algorithms developed through neural networks are used, as they cannot be fully understandable both for manufacturer and clinicians, constituting a black box (24). Since AI algorithms’ lack of explainability, it could be difficult for doctors to assess whether the diagnosis or recommendations of an AI system are sound in relation to their own knowledge (26). Moreover, unexperienced physicians may blindly trust diagnosis of AI algorithms so that, according to Chung et al. (8), “a complication or medical malpractice may be further perplexed, since both healthcare professionals and AI developers are involved.”
Another issue related to this aspect consists in the possible presence of biases during the development phase of AI algorithms, which may lead to the incorrect identification of an ill person as healthy (underdiagnosis) and result in a delayed access to care (32). Automatic machine learning requires indeed “big data” to instruct algorithms, yet sometimes these latter are affected by unsatisfactory training samples with repercussions on generalization (33). Alongside clinical consequences, similar situations may raise claims difficult to resolve. The impossibility to identify the exact moment in which the error has occurred, would indeed prevent the finding of the link between the error and the damage, and preclude the compensation. One could, as an evaluation criterion, refer to the degree of autonomy of AI to identify the professional’s contribution: when it is minimal and therefore AI is completely autonomous even in its updates or when human intervention is essential. According to Sung et al. (15), when clinicians or developers are capable to prevent an accident, legal liability is likely to be defined, whereas in case of diagnosis and treatment achieved basing on self-learned algorithms which do not require clinicians’ approval, responsibility should be at least partially attributed to the AI developer. Usually, however, AI as a calculation program used in health systems and hospitals needs a human contribution through which the definitive result is reached, and therefore it is important to analyze the responsibility separating the various profiles.
The use of AI algorithms in medicine indeed implies multiple actors, consisting in developers, hospitals, physicians. Negligence could be expression of a failure of programming, supervision, clinicians’ actions or of the algorithm (11). Therefore, it would be important to differentiate when the error can be traced back to the doctor for the improper use of these systems or when it is only and exclusively attributable to the hospital that manages and sets the instrument that does not require additional human aids. It would also be important to trace the consent of patients to the use of new technologies in clinical practice (33). The information given to patients about risks, benefits and limits of AI is a step of paramount importance, and it should be aimed at ensuring full awareness of choices and eventual alternative pathways in case of resistance to new technologies (34). In this context, however, the difficulty of explaining how AI systems transform inputs into outputs represent a challenge for the informed consent, and sometimes patients’ level of awareness could be not sufficient to make this latter possible. Clinicians could be considered as mediators between patients and AI systems, being the recipients of an explanation from the AI system and acting toward the patient as a translator into a meaningful and easily understood format (35). As pointed out in other medical contexts, doctors have a professional duty to explain and discuss risks, benefits and possible alternatives to a procedure: the information process should take into account the fact that patients may not be able to assess the risks in abstract terms and they may therefore need to rely on a comparison to achieve an informed decision (36). Moreover, the informed consent is supposed to be achieved through an appropriate legal framework for data protection: the machine learning processes require large amounts of data whose right of use is often differently regulated among countries, especially with regard to the degree of de-identification of the patient’s identity (37). The issue of consent is also crucial in view of the progression toward personalized medicine, which is an area in which some fundamental problems of the use of AI in medicine re-emerge, namely privacy, stratification and discrimination of sub-populations on the basis of ethnicity, equality of access and fair allocation of resources (38).
Already in 2018, the European Commission decided to launch a coordinated plan on AI with European Union Member States (in addition to Switzerland and Norway), and in 2019 it published ethics guidelines for trustworthy AI, which emphasized the importance of AI in healthcare with reference to the ageing of the European population. Similarly, the World Health Organization issued in 2021 a guidance on Artificial Intelligence in Health including principles for its design and use (37). In September 2022, a proposal for a new directive on liability of defective products and a proposal for a directive on adapting non-contractual civil liability rules to AI were published by the European Commission (39, 40). This latter purposes to “improve the functioning of the internal market by laying down uniform requirements for non-contractual civil liability for damage caused with the involvement AI systems,” and it would create a “rebuttable presumption of causality,” in order to simplify the burden of proof for harmed people. Despite the provision of some uniform liability rules, however, according to Duffourc et al. (41) by applying these directives, potential liability gaps would persist when using some black-box medical AI systems.
The reviewed literature analyses the current theories of liability, trying to apply them to AI context, including medical malpractice, vicarious liability, product liability, learned intermediary exception. Briefly, according to malpractice law, the physician could be considered liable in negligence “for harmful medical errors that fall below the standard of care” (26). According to the vicarious liability model instead the faults of subordinates would be in charge of principals, for example the hospitals or physician groups in clinical settings (13). Product liability relates to the existence of a defect in the product, and learned intermediary doctrine identifies the physician as intermediary (10, 11, 26). In the context of AI, giving AI personhood has been discussed as a possible solution, so that harmed patients could directly sue AI devices (10, 11, 24). Chan (26) instead suggests a common enterprise model, which encloses manufacturers, physicians and hospitals, which would let a shift from the individualistic concept of responsibility toward a more distributed one.
In authors’ opinion, it would be interesting to think of the relationship between the hospital and AI as that of an institution that, by making this “diagnostic assistant” available, behaves in the relationship with this instrument as a supervisor, as a custodian, which guarantees in the hospital environment its correct use. It is also interesting to note that the uncertainties in the area of professional liability are not only related to AI algorithms, but have also previously arisen referring to the use of telemedicine, for which some of the hypothesized profiles were similar: production defects of the equipment, omitted/defective/ineffective maintenance, errors in the use of the equipment (42). In that context, not only have multiple normative acts, projects and strategic documents been implemented at European level, but also at national level policies aimed at increasing the diffusion and efficiency of telemedicine services (including between 2014 and 2021, the National Guidelines for Telemedicine, the Digital Growth Strategy, the National Directives for the Delivery of Telemedicine Services, and the National Recovery and Resilience Plan) (43). That said, it is clear that the increasing use of AI in medicine will lead to legal challenges regarding medical negligence and that for this reason on the one hand the legal system should provide clear indications on what entity holds the liability, and on the other hand insurances should make explicit the terms of coverage in the event that healthcare decisions are partially achieved an AI system (44).
In any case, the result is a real revolution in the relationship between the patient and the physician, who was born as a pater and undisputed mentor of the patient in the paternalistic model, further evolved into an ally in the management of the patient’s therapy in the model of reciprocity, and is undergoing a new evolution due to the intervention of a third element in the relationship: the AI. This evolution, which is going to challenge the fiduciary doctor–patients relationship as it is known, opens up new medico-legal scenarios in the area of liability. The consequence of the increasing use of automatic technology in healthcare could therefore be a new conception of the doctor as human in command, which sometimes would only keep the role of controlling the final result of the machine (output) and validating it. In such a context, it is conceivable that in case of default on the part of AI intelligence, of which the hospital act as custodian and owner, it will be the latter that with the professional will have to answer, and the hospital will have to provide for a specific insurance that covers that particular risk with a specific “diagnostic assistant” or “algorithm liability” liability insurance contract.
One of the most well-known critical points of legal regulations consists in the difficulty of adapting to new realities. A regulation is considered evolved the more it manages to capture in its own meshes events that are dissimilar to the typical norm that may occur in the future and which can be subsumed by it: this can only happen if it includes broad definitions and at the same time clear, precise, and broad prescriptions. In the current context, in order to answer to the issue of liability for the use AI algorithms, it is essential in the legislative production a scientific as well as legal preparation, since it engages the law in forms of protection not yet known, prompted by events that only science can foresee.
The increasingly widespread use of intelligent systems, capable of learning and making decisions, opens up new exciting frontiers, but at the same time, it radically changes the relationship between humans and technology. Over the last 3 years, the interest about advantages and critical issues related to the application of AI in medicine has significantly grown, with an increasing attention to the potential liability consequences in case of error. Alongside its potentials, the wide use of AI still raises concerns regarding possible development bias, disparities, informed consent, privacy, doctor–patient relationship, and liability. Since the use of AI in healthcare involves multiple actors, consisting in manufacturer, hospitals, physicians, different solutions to the liability issue have been analyzed, which however do not identify a definitive answer. The application of AI is rapidly extending to all healthcare setting, and an unequivocal answer on the allocation of responsibility in case of errors is becoming increasingly necessary. The danger of over-reliance and excessive dependency on such systems, which could lead to significant effects of de-skilling and desensitization of doctors to the clinical context, should also be highlighted and studied. It is also necessary for AI systems to explain to operators how they arrived at their conclusion and decision, providing the evidence that underlies their reasoning. This way, they can potentially choose to refuse to follow the suggestion if they believe any errors might have been made. In order to outline a reference regulatory framework to which address issues arising from the use of AI algorithms, broad definitions but at the same time clear and precise prescriptions should be provided based on scientific evidences.
CC: Data curation, Formal analysis, Investigation, Methodology, Writing – original draft. AD: Data curation, Software, Writing – review and editing. BM: Data curation, Software, Writing – review and editing. LC: Conceptualization, Writing – review and editing. PT: Conceptualization, Supervision, Writing – original draft.
The author(s) declare that no financial support was received for the research, authorship, and/or publication of this article.
The authors declare that the research was conducted in the absence of any commercial or financial relationships that could be construed as a potential conflict of interest.
All claims expressed in this article are solely those of the authors and do not necessarily represent those of their affiliated organizations, or those of the publisher, the editors and the reviewers. Any product that may be evaluated in this article, or claim that may be made by its manufacturer, is not guaranteed or endorsed by the publisher.
1. Bhardwaj A. Promise and provisions of artificial intelligence and machine learning in healthcare. J Healthc Leadersh. (2022) 14:113–8. doi: 10.2147/JHL.S369498
2. Kline A, Wang H, Li Y, Dennis S, Hutch M, Xu Z, et al. Multimodal machine learning in precision health: a scoping review. npj Digit. Med. (2022) 5:171. doi: 10.1038/s41746-022-00712-8
3. Hosny A, Parmar C, Quackenbush J, Schwartz LH, Aerts HJWL. Artificial intelligence in radiology. Nat Rev Cancer. (2018) 18:500–10. doi: 10.1038/s41568-018-0016-5
4. Farina E, Nabhen JJ, Dacoregio MI, Batalini F, Moraes FY. An overview of artificial intelligence in oncology. Future Sci OA. (2022) 8:FSO787. doi: 10.2144/fsoa-2021-0074
5. Kamran F, Tang S, Otles E, McEvoy DS, Saleh SN, Gong J, et al. Early identification of patients admitted to hospital for covid-19 at risk of clinical deterioration: model development and multisite external validation study. BMJ (Clinical Research ed.). (2022) 376:e068576. doi: 10.1136/bmj-2021-068576
6. Rockall AG, Shelmerdine SC, Chen M. AI and ML in radiology: making progress. Clin Radiol. (2023) 78:81–2. doi: 10.1016/j.crad.2022.10.010
7. Aagaard L. Artificial intelligence decision support systems and liability for medical injuries. J Res Pharm Pract. (2020) 9:125–7. doi: 10.4103/jrpp.JRPP_20_65
8. Chung CT, Lee S, King E, Liu T, Armoundas AA, Bazoukis G, et al. Clinical significance, challenges and limitations in using artificial intelligence for electrocardiography-based diagnosis. Int J Arrhythmia. (2022) 23:24. doi: 10.1186/s42444-022-00075-x
9. Emiroglu M, Esin H, Erdogan M, Ugurlu L, Dursun A, Mertoglu S, et al. National study on use of artificial intelligence in breast disease and cancer. Bratisl Lek Listy. (2022) 123:191–6. doi: 10.4149/BLL_2022_032
10. Mezrich JL. Is artificial intelligence (AI) a pipe dream? Why legal issues present significant hurdles to AI autonomy. AJR Am J Roentgenol. (2022) 219:152–6. doi: 10.2214/AJR.21.2722
11. Mezrich JL. Demystifying medico-legal challenges of artificial intelligence applications in molecular imaging and therapy. PET Clin. (2022) 17:41–9. doi: 10.1016/j.cpet.2021.08.002
12. Vedantham S, Shazeeb MS, Chiang A, Vijayaraghavan GR. Artificial intelligence in breast X-ray imaging. Semin Ultrasound CT MR. (2023) 44:2–7. doi: 10.1053/j.sult.2022.12.002
13. Harvey HB, Gowda V. Regulatory issues and challenges to artificial intelligence adoption. Radiol Clin North Am. (2021) 59:1075–83. doi: 10.1016/j.rcl.2021.07.007
14. Harvey HB, Gowda V. Clinical applications of AI in MSK imaging: a liability perspective. Skeletal Radiol. (2022) 51:235–8. doi: 10.1007/s00256-021-03782-z
15. Sung JJ, Poon NC. Artificial intelligence in gastroenterology: where are we heading? Front Med. (2020) 14:511–7. doi: 10.1007/s11684-020-0742-4
16. Pecqueux M, Riediger C, Distler M, Oehme F, Bork U, Kolbinger FR, et al. The use and future perspective of Artificial Intelligence-A survey among German surgeons. Front Public Health. (2022) 10:982335. doi: 10.3389/fpubh.2022.982335
17. López DM, Rico-Olarte C, Blobel B, Hullin C. Challenges and solutions for transforming health ecosystems in low- and middle-income countries through artificial intelligence. Front Med (Lausanne). (2022) 9:958097. doi: 10.3389/fmed.2022.958097
18. Bazoukis G, Hall J, Loscalzo J, Antman EM, Fuster V, Armoundas AA. The inclusion of augmented intelligence in medicine: a framework for successful implementation. Cell Rep Med. (2022) 3:100485. doi: 10.1016/j.xcrm.2021.100485
19. Musacchio N, Giancaterini A, Guaita G, Ozzello A, Pellegrini MA, Ponzani P, et al. Artificial intelligence and big data in diabetes care: a position statement of the italian association of medical diabetologists. J Med Internet Res. (2020) 22:e16922. doi: 10.2196/16922
20. Channa R, Wolf R, Abramoff MD. Autonomous artificial intelligence in diabetic retinopathy: from algorithm to clinical application. J Diabetes Sci Technol. (2021) 15:695–8. doi: 10.1177/1932296820909900
21. Abràmoff MD, Tobey D, Char DS. Lessons learned about autonomous AI: finding a safe, efficacious, and ethical path through the development process. Am J Ophthalmol. (2020) 214:134–42. doi: 10.1016/j.ajo.2020.02.022
22. Pai VV, Pai RB. Artificial intelligence in dermatology and healthcare: an overview. Indian J Dermatol Venereol Leprol. (2021) 87:457–67. doi: 10.25259/IJDVL_518_19
23. Filipow N, Main E, Sebire NJ, Booth J, Taylor AM, Davies G, et al. Implementation of prognostic machine learning algorithms in paediatric chronic respiratory conditions: a scoping review. BMJ Open Respir Res. (2022) 9:e001165. doi: 10.1136/bmjresp-2021-001165
24. Nitiéma P. Artificial intelligence in medicine: text mining of health care workers’ Opinions. J Med Internet Res. (2023) 25:e41138. doi: 10.2196/41138
25. Neri E, Coppola F, Miele V, Bibbolino C, Grassi R. Artificial intelligence: who is responsible for the diagnosis? Radiol Med. (2020) 125:517–21. doi: 10.1007/s11547-020-01135-9
26. Chan B. Applying a common enterprise theory of liability to clinical AI systems. Am J Law Med. (2021) 47:351–85. doi: 10.1017/amj.2022.1
27. Lang M, Bernier A, Knoppers BM. Artificial intelligence in cardiovascular imaging: “unexplainable” legal and ethical challenges? Can J Cardiol. (2022) 38:225–33. doi: 10.1016/j.cjca.2021.10.009
28. Jobson D, Mar V, Freckelton I. Legal and ethical considerations of artificial intelligence in skin cancer diagnosis. Australas J Dermatol. (2022) 63:e1–5. doi: 10.1111/ajd.13690
29. Patcas R, Bornstein MM, Schätzle MA, Timofte R. Artificial intelligence in medico-dental diagnostics of the face: a narrative review of opportunities and challenges. Clin Oral Investig. (2022) 26:6871–9. doi: 10.1007/s00784-022-04724-2
30. Bird & Bird LLP. I Sistemi di Intelligenza Artificiale per la Diagnosi Medica. London: Bird & Bird LLP (2022).
31. Rowland SP, Fitzgerald JE, Lungren M, Lee EH, Harned Z, McGregor AH. Digital health technology-specific risks for medical malpractice liability. NPJ Digit Med. (2022) 5:157. doi: 10.1038/s41746-022-00698-3
32. Seyyed-Kalantari L, Zhang H, McDermott MBA, Chen IY, Ghassemi M. Underdiagnosis bias of artificial intelligence algorithms applied to chest radiographs in under-served patient populations. Nat Med. (2021) 27:2176–82. doi: 10.1038/s41591-021-01595-0
33. O’Sullivan S, Nevejans N, Allen C, Blyth A, Leonard S, Pagallo U, et al. Legal, regulatory, and ethical frameworks for development of standards in artificial intelligence (AI) and autonomous robotic surgery. Int J Med Robot. (2019) 15:e1968. doi: 10.1002/rcs.1968
34. Comitato Nazionale di Bioetica. Intelligenza Artificiale e Medicina. Aspetti Etici. (2020). Rome. Available online at: https://bioetica.governo.it/media/4263/intelligenza-artificiale-parere-congiunto-cnb-cnbbsv-it-en.pdf (accessed November 14, 2023).
35. Council of Europe. The impact of artificial intelligence on the doctor-patient relationship. (2021). Available online at: https://rm.coe.int/inf-2022-5-report-impact-of-ai-on-doctor-patient-relations-e/1680a68859. (accessed November 14, 2023).
36. Gullo G, Scaglione M, Buzzaccarini G, Laganà AS, Basile G, Chiantera V, et al. Cell-free fetal DNA and non-invasive prenatal diagnosis of chromosomopathies and pediatric monogenic diseases: a critical appraisal and medicolegal remarks. J Pers Med. (2022) 13:1.
37. Medenica S, Zivanovic D, Batkoska L, Marinelli S, Basile G, Perino A, et al. The future is coming: artificial intelligence in the treatment of infertility could improve assisted reproduction outcomes-the value of regulatory frameworks. Diagnostics (Basel, Switzerland). (2022) 12:2979.
38. Piergentili R, Basile G, Nocella C, Carnevale R, Marinelli E, Patrone R, et al. Using ncRNAs as tools in cancer diagnosis and treatment-the way towards personalized medicine to improve patients’ health. Int J Mol Sci. (2022) 23:9353. doi: 10.3390/ijms23169353
39. European Parliament. EU Legislation in Progress. New Product Liability Directive. (2023). Available online at: https://www.europarl.europa.eu/RegData/etudes/BRIE/2023/739341/EPRS_BRI(2023)739341_EN.pdf (accessed November 14, 2023).
40. European Parliament. EU Legislation in Progress. Artificial Intelligence Liability Directive. (2023). Available online at: https://www.europarl.europa.eu/RegData/etudes/BRIE/2023/739342/EPRS_BRI(2023)739342_EN.pdf (accessed November 14, 2023).
41. Duffourc MN, Gerke S. The proposed EU Directives for AI liability leave worrying gaps likely to impact medical AI. NPJ Digit Med. (2023) 6:77. doi: 10.1038/s41746-023-00823-w
42. Basile G, Accetta R, Marinelli S, D’Ambrosi R, Petrucci QA, Giorgetti A, et al. Traumatology: adoption of the Sm@rtEven application for the remote evaluation of patients and possible medico-legal implications. Journal of clinical medicine. (2022) 11:3644. doi: 10.3390/jcm11133644
43. Ricci G, Caraffa AM, Gibelli F. Telemedicine as a strategic tool to enhance the effectiveness of care processes: technological and regulatory evolution over the past two decades. Healthcare (Basel, Switzerland). (2023) 11:734.
44. Yu KH, Beam AL, Kohane IS. Artificial intelligence in healthcare. Nat Biomed Eng. (2018) 2:719–31. doi: 10.1038/s41551-018-0305-z
Keywords: artificial intelligence, diagnostic algorithm, medical liability, regulation, systematic review
Citation: Cestonaro C, Delicati A, Marcante B, Caenazzo L and Tozzo P (2023) Defining medical liability when artificial intelligence is applied on diagnostic algorithms: a systematic review. Front. Med. 10:1305756. doi: 10.3389/fmed.2023.1305756
Received: 02 October 2023; Accepted: 08 November 2023;
Published: 27 November 2023.
Edited by:
Giovanna Ricci, University of Camerino, ItalyReviewed by:
Giuseppe Basile, IRCCS Istituto Ortopedico Galeazzi, ItalyCopyright © 2023 Cestonaro, Delicati, Marcante, Caenazzo and Tozzo. This is an open-access article distributed under the terms of the Creative Commons Attribution License (CC BY). The use, distribution or reproduction in other forums is permitted, provided the original author(s) and the copyright owner(s) are credited and that the original publication in this journal is cited, in accordance with accepted academic practice. No use, distribution or reproduction is permitted which does not comply with these terms.
*Correspondence: Pamela Tozzo, cGFtZWxhLnRvenpvQHVuaXBkLml0
Disclaimer: All claims expressed in this article are solely those of the authors and do not necessarily represent those of their affiliated organizations, or those of the publisher, the editors and the reviewers. Any product that may be evaluated in this article or claim that may be made by its manufacturer is not guaranteed or endorsed by the publisher.
Research integrity at Frontiers
Learn more about the work of our research integrity team to safeguard the quality of each article we publish.