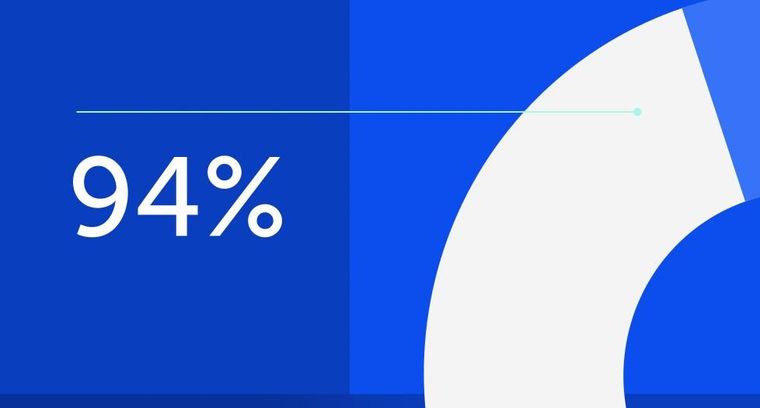
94% of researchers rate our articles as excellent or good
Learn more about the work of our research integrity team to safeguard the quality of each article we publish.
Find out more
ORIGINAL RESEARCH article
Front. Med., 15 January 2024
Sec. Rheumatology
Volume 10 - 2023 | https://doi.org/10.3389/fmed.2023.1301944
This article is part of the Research TopicInterprofessional Approaches for the Management of Chronic DiseasesView all 14 articles
Background: This retrospective study was designed to analyze the prevalence and impact of associated comorbidities on fibromyalgia (FM) outcomes (functionality, pain, depression levels) for patients who participated in an intensive multicomponent clinical program in a tertiary care center.
Methods: Participants included a sample of 411 patients diagnosed with FM at a large tertiary medical center using the 2016 ACR criteria. Patients completed an intensive 2-day cognitive behavioral treatment (CBT) program, filled out the Fibromyalgia Impact Questionnaire Revised (FIQR), the Center for Epidemiologic Studies Depression Scale (CES-D), the Pain Catastrophizing Scale (PCS), and were followed for 6 months after treatment completion. T-tests were performed to analyze differences between the presence or absence of select comorbidities for the three outcomes at follow-up. Statistically significant comorbidities (p < 0.05) were used as predictors in multivariable logistic regression models.
Results: The FM associated comorbidities in this cohort that had significant impact on the measured outcome domains after treatment program completed were Obesity (FIQR p = 0.024), Hypothyroidism (CES-D p = 0.023, PCS p = 0.035), Gastroesophageal reflux disease GERD (PCS p < 0.001), Osteoarthritis (CES-D p = 0.047). Interestingly, Headache, the most frequent FM associated comorbidity in this cohort (33.6%), did not have a significant impact on the outcome domains at follow-up. Obesity (18.2%) was the only FM associated comorbidity significantly impacting all three outcome domains at follow-up.
Conclusion: The present study suggests that addressing obesity may significantly impact outcomes in FM patients.
Fibromyalgia (FM) affects approximately 10 million people in the US alone with estimates as high as 8% of the population world-wide (1, 2). The prevalence rate of FM in the general population is estimated to be 2–4% (3). It is a chronic pain syndrome with features that includes widespread musculoskeletal pain, extreme fatigue, sleep disturbance, and cognitive complaints (4–7). FM can result in significant incapacity with 35% of patients with FM in the United States on government disability assistance (8). Patients with FM struggle with depression with numerous studies documenting a high prevalence of depression in this population (9). Further, pain catastrophizing has been linked to important outcomes in chronic pain patients such as higher opioid use, longer hospital stay and increased likelihood of being on disability (10). FM is associated with other medical and/or psychiatry conditions that affect its clinical presentation, and result in complications that require specific treatments, and may potentially impact outcome (11).
FM is considered a complex condition that affects the way that the brain processes pain signal, leading to high sensitivity and discomfort. Along with pain, individual with FM experience sleep disturbances, cognitive difficulties (sometimes refer to as a “fibro fog”), mood disorders. The most widely used criteria for diagnosing FM is the 2016 American College of Rheumatology (ACR) criteria. According to this criteria, widespread pain, tender trigger points,
and the updated comprehensive evaluation symptoms and their impact on a person quality of life are the main elements to make a FM diagnosis currently (3, 5, 6, 12).
Sex and age can play a role in the prevalence, symptoms, and management of FM, though individual experiences can vary. FM is more commonly diagnosed in women than in men. Studies suggest that women have a higher likelihood of developing FM (70–90%). FM can affect individuals of all ages, but it is more commonly diagnosed in middle aged adults (30–50-year-old) (1, 2).
FM patients experience a higher rate of comorbid conditions, specifically rheumatologic and psychiatric conditions as compared with the general population (13–15), that have included coronary artery disease, myocardial infarction (MI), hypertension, stroke (16–18), diabetes mellitus, and irritable bowel syndrome (13, 19). In one study involving a large sample of community-dwelling adults with FM, over 50% were found to have seven or more chronic conditions (20). Most scientific studies that have examined comorbidity in FM has focused on clinical presentation, pain management, and medications (21–24). FM has been associated with various comorbid entities including medical and psychiatric disorders. During the last few decades, the prevalence of chronic illnesses has risen due to multiple factors including but not limited to better understanding of the diseases, increased longevity, improved access to health care, electronic medical record, patient online services, etc. (25, 26). Comorbidities may lead to a delay in the diagnosis of FM, may be mistaken as poor control of the primary disease, may cause leading to incorrect treatment decisions, and may also increase Morbi and mortality in these patients (23, 27).
The aim of this study is to determine the prevalence of comorbidities in a cohort of FM patients (25, 28, 29) who attended a 2-day cognitive behavioral treatment program, and examine the impact of each comorbidity on specific outcomes of level of psychological distress/depression, functional status, and cognitive strategies that specifically impact pain and its management.
Participants were recruited from a large tertiary medical center’s Fibromyalgia Treatment Program. Inclusion criteria for the study included a diagnosis with FM using the 2016 ACR criteria (5) and informed consent to participate in the study. No exclusion criteria were used for the study. The Fibromyalgia Treatment Program is an intensive 2-day cognitive behavioral treatment program. Participants completed follow up surveys approximately 6-months following treatment to assess outcomes. The study was approved by the Mayo Clinic Institutional Review Board (Protocol ID: 19–000495). Baseline data including study measures and demographic information were collected before the patients started the 2-day program. Post-intervention data were collected with mailed surveys at post-treatment. If patients did not respond to the mailed survey a reminder letter was sent out.
Presence of a comorbidity was defined as any diagnosis from a patient’s medical history. Individual comorbidities were grouped into twelve broader categories with patients belonging to a particular category if they had any diagnosed conditions from medical history or current visit (29, 30). Multi-morbidities were defined as low (2 or less), and high (3 or more) comorbidity-associated medical diagnosis documented at any time (medical history/current visit) (26, 31).
The Fibromyalgia Impact Questionnaire-Revised (FIQR) measures functional status and is the most widely used measure of functional impairment in FM patients. Three domains are evaluated with this measure and include function, overall impact, and symptoms. The scale includes twenty-one items that are scored from 0–10 with higher scores reflecting greater functional impairment. Severe functional impairment is indicated by scores of 60 or above. The psychometric properties of this scale have been researched (12).
The Center for Epidemiologic Studies of depression Scale (CES-D) is a 20-item scale that assesses the presence and severity of depressive symptoms (32). Scores range from 0 to 6-with higher scores reflecting a higher degree of depressive symptomatology. The clinical threshold for depression is a score of 16 on this measure. The psychometric properties of the CES-D are well researched (33).
The Pain Catastrophizing Scale (PCS) is a self-report questionnaire designed to measure the tendency of individuals to catastrophize or magnify the significance of pain they experience. It assesses the degree to which a person experiences some negative thoughts and emotions related to pain, such as rumination, magnification, and helplessness. These 3 subscales help researchers and healthcare professionals better understand and evaluate the psychological impact of pain on individuals (34–36).
The Fibromyalgia Treatment Program is a 16-h, cognitive behavioral based, group program that addresses education and evidence-based strategies to decrease central sensitization. Strategies addressed include relaxation training, use of moderation, pacing, exercise, cognitive skills, sleep hygiene, stress management, social support, and the use of pharmacological options to improve symptoms and functioning (37). The effectiveness of this intervention has been described elsewhere (38).
Cohort characteristics, comorbidities, and outcomes were summarized with frequency (percentage) for categorical variables and with mean, standard deviation, and range for continuous variables. Twelve individual conditions selected based on prevalence as well as twelve grouped comorbidities were further used in outcome analysis. T-tests were performed to analyze differences between presence or absence of individual comorbidities as well as grouped comorbidities for the three outcomes (FIQR, CES-D, PCS at follow-up). Additionally, we compared patients with 2 or less vs. 3 or more comorbidities for each outcome. The comorbidities that were statistically significant comorbidities (p < 0.05) in our univariate analyses were selected for inclusion into our multivariable logistic regression models as predictors; all the comorbidities were evaluated under this criterium for each outcome independently. The multivariable logistic regression models were adjusted for patient’s age and sex, where odds ratios (ORs) and 95% confidence intervals (Cis) were estimated; binary outcomes were defined as severe functioning (FIQR >60), severe depression (CES-D ≥ 20), and severe pain catastrophizing (PCS ≥ 30). Analyses were conducted using R Statistical Software (version 4.0.3; R Foundation for Statistical Computing, Vienna, Austria).
The effect of baseline measures of pain, depression, and function was not considered in the regression modeling. The main aim of this study was to look at associations of comorbidities with our outcomes at follow-up. Another study we published using the same cohort discussed the treatment effectiveness between our baseline and follow-up outcome scores (38).
Participants included 411 patients diagnosed with fibromyalgia at a large tertiary medical center using the 2016 ACR criteria (5). The sample completed an intensive 2-day cognitive behavioral treatment program and were followed after treatment to assess treatment effectiveness. Follow up data was received 4–8 months after treatment with an average for the sample of 6-month follow-up.
In our cohort, women represent 90.3%, and the age mean was 54.7 years for the overall sample (411) with a standard deviation SD of 13.9 years (Table 1). As seen in Figure 1, 1,000 patients were assessed for eligibility from the Fibromyalgia Treatment Program. From them, zero patients were excluded due to ineligibility and all consented to participate. All 1,000 patients completed baseline data collection. Of them, 511 patients were lost to follow up as they did not complete mailed surveys at 6-months post-intervention. A subset of patients (n = 78) were excluded due to inability to locate sufficient medical chart review information. In the present analysis, 411 patients were included.
The results, as contained in Table 1, revealed the following comorbidities: headaches (33.6%), osteoarthritis (23.4%), hypertension (23.1%), insomnia (22.4%), obesity (18.2%), low back pain (18%), obstructive sleep apnea (OSA) (16.5%), irritable bowel syndrome (IBS) (14.8%), abdominal pain (10%), hypothyroidism (7.1%), rheumatoid arthritis (RA) (5.8%), and gastroesophageal reflux (GERD) (4.1%).
Comparisons between patients with vs. patients without a certain comorbidity for the three outcomes are summarized in Table 2. Obesity negatively impacted functioning at follow-up with obese patients having, on average, a poorer functioning score than non-obese patients (mean: 51.0 vs. 45.1, p = 0.024). Patients with hypothyroidism, however, were more likely to have lower depression (mean: 18.2 vs. 21.6, p = 0.023) and pain catastrophizing (mean: 12.1 vs. 16.0, p = 0.035) scores at follow-up than patients without hypothyroidism. Similarity, patients with osteoarthritis had a lower depression score (mean: 19.6 vs. 21.9, p = 0.047) and patients with gastroesophageal reflux (GERD) had a lower pain catastrophizing score (mean: 12.1 vs. 16.0, p = 0.023) six months after the treatment program. Although not statistically significant, abdominal pain, headache, irritable bowel syndrome, and rheumatoid arthritis negatively impacted all three outcomes at follow-up (Table 2).
In Table 3, we examined logistic regressions for significant comorbidities and binary treatment outcomes. In our multivariate analysis, obesity was the only statistically significant predictor of FIQR score greater than 60 after adjusting for patient’s age and sex, showing that obese patients were twice more likely to have poorer functional status after completing the treatment program (OR = 1.77, 95% CI: 1.04–2.98, p = 0.033).
Table 3. Comparison between patients with vs. without select comorbidities by outcomes at follow-up.
Adjusting logistic regression models for age and sex is important for several reasons: confounding variables can distort the observed associations, population characteristics are fundamental demographic variables that reflect important differences in the population, and standardization allows for more meaningful comparisons and reduces the potential impact of demographic differences on the observed associations (3, 6, 8, 39).
Table 4 compares differences between grouped comorbidities for these three outcomes at follow-up. Patients in the psychiatry and gynecology groups tended to have higher depression scores (mean: 22.6 vs. 20.1, p = 0.008; 25.2 vs. 21.1, p = 0.022, respectively). Also, patients in the psychiatry group experienced higher pain catastrophizing score (mean: 17.1 vs. 14.4, p = 0.035).
Comparison was done with those having 2 or fewer comorbidities vs. 3 or more comorbidities to see if the outcome measures were negatively impacted by the number of comorbidities (possibly indicative of worse health which could be confounding or contributing factors to dysfunction, depression, and pain catastrophizing) in addition to the condition of FM. In our cohort, 32.8% of patients had 3 or more comorbidities while 67.2% had 2 or less (Table 1). When we analyzed impact of multi-morbidities on outcomes, we found no statistically significant differences between the two groups in functioning, depression or pain catastrophizing scores (Table 5).
The present study assessed the prevalence and impact of comorbidities on treatment effectiveness in FM patients. We examined comorbidities and treatment outcomes (functional impairment, pain catastrophizing, and depression) using several statistical techniques. The data revealed that in our sample, similar to other published studies with FM populations, the most common comorbid conditions were identified: headaches, hypertension, osteoarthritis, and insomnia. Unlike other published studies with higher rates, Rheumatoid arthritis was only identified in 5.8% of our sample (12, 21–37).
Treatment outcomes were then compared for FM patients with and without each comorbidity. This analysis indicated that FM patients with obesity had significantly poorer treatment outcomes in functional impairment. Other comorbidities, including Osteoarthritis, GERD, and Hypothyroidism appeared to impact treatment outcomes in a positive direction. These positive findings may be artificial, as the comorbidity occurred in a very small subset of patients (e.g., Osteoarthritis n = 96; GERD n = 17; Hypothyroidism n = 29).
Taking into account age and sex, Odds Ratios (ORs) were analyzed for Obesity, Osteoarthritis, and Hypothyroidism. These comorbidities were selected based on their significance in the previous analysis (40, 41). ORs were calculated to predict dichotomous treatment outcomes (FIQR >60 = significantly impaired; CES-D > 20 = clinically significant depression; PCS > 30 = clinically significant pain catastrophizing). Of these OR’s, only Obesity indicated a significantly increased risk of functional impairment (OR = 1.77). Put differently, FM patients with Obesity had a 77% higher risk of being significantly impaired at 6-months follow-up, compared to FM patients without Obesity. Obesity adversely affected functioning in our sample at follow-up and needs further investigation. Obesity may impact ability to exercise leading to a deconditioned state that further intensifies FM symptoms (Table 3). A previous systematic review and meta-analysis showed that obesity may impact FM in several ways in addition to potentially limiting exercise or activity level which included level of pain, number of tender trigger points used in previous diagnostic criteria, level of disability, degree of fatigue, and sleep disturbance as well as quality of life (42).
We then grouped comorbidities into different specialty domains (e.g., Rheumatological, Psychiatry, Pain). These analyses revealed Psychiatric comorbidities yielded associations with increased depression and pain catastrophizing scores at follow-up. As these outcomes are expected to correlate with psychological functioning, this finding is expected. The prevalence of psychiatric disorders found in our study was 48.2% which is significantly higher than a previous report where only 25.3% were found to have a psychiatric disorder (27). However, Kleycamp et al. (43) completed a systematic overview of psychiatric and chronic pain comorbidities among patients diagnosed with FM and noted the most prevalent comorbidity was depression that was found in over 50% of patients similar to our findings. Depression (38) is a significant comorbidity associated with FM and prevalence was consistent with results of another cross-sectional studies (44) In addition, Gynecological comorbidities were associated with worse depressive symptoms at follow-up. Gynecological comorbidities were less frequently observed in this study sample (n = 21). This finding is consistent with other studies with depression and Gynecological conditions.
Finally, we examined the impact of multimorbidities on treatment outcomes. Data revealed that the Fibromyalgia Treatment Program was equally efficacious for patients with 2 or less comorbidities as 3 or more comorbidities. To clarify the possible confusion between what constitutes a FM symptom versus an associated comorbidity, we based this not only in terms of the scientific society criteria, but also in the consideration that pain syndrome is not exclusive of FM, it is one of its main characteristics. Abdominal pain, headache could be part of the FM diagnosis, however there are other areas to be considered such as overlapping chronic pain syndromes that make them sometimes independent conditions (19–23). We included abdominal pain, headache since they were documented in the electronic medical record prior to the diagnosis of FM, and specific treatments and follow ups were given previously.
Insomnia and sleep disorder are related but have distinct differences. FM symptoms could include sleep disturbance; however, this is a broader term that encompasses various conditions that disrupt normal sleep pattern. Sleep disorders can include insomnia, as well as other conditions like obstructive sleep apnea (OSA), narcolepsy, restless leg syndrome, and parasomnias. Each sleep disorder has his own specific characteristics diagnostic criteria (42,44,45). We have included insomnia as an associated comorbidity in this study since prior to the diagnosis of FM, and documented in medical history, it was a diagnosis (22.38%), not a FM symptom and a specific treatment and follow ups were offered in some of the cohort patients.
Limitations of this study include the setting of a tertiary medical care clinic that results in patients with a higher degree of symptom severity and potentially comorbidity. Additionally, conducting multiple comparisons for hypothesis-generation purposes increases the risk of false positive results. This possibility should be considered when interpreting the findings. Moreover, correction procedures for multiple comparisons were not applied in our study, potentially influencing the significance of certain findings. Future research should address this limitation by employing appropriate correction methods. Further replication studies are needed to validate the significant finding related to obesity and establish the reliability and generalizability of our results.
One of the major limitations of this study is that the comorbidities were collected from medical records and relied on a condition having been diagnosed/documented in the patient notes to be considered a comorbidity The process of collecting data from the electronic medical record was done through an electronic search and by hand in order to ensure accuracy of the data. However, these methods used retrospectively may have underestimated the occurrence of comorbid health conditions. Future studies may be able to collect this information from health care providers prospectively to improve this aspect of the data. Given the limited sample size specific to our tertiary care setting, it is unclear whether it is the obesity or a combination of other less statistically significant comorbidities in association with the obesity that negatively impacted these outcome measures along with the FM. The study assessed the prevalence of comorbidities in our FM patients, but further evaluation needs to be done regarding the generalizability of our results. Further, in our population of FM patients, headaches, osteoarthritis, and hypertension were the most common comorbidities and although cannot be directly linked to gender or age, they are common concerns in middle-aged women (90% of our FM population). Lastly, the limited sample size may impact the percentage of FM patients with concomitant rheumatoid arthritis compared to other studies.
The most important clinical implication of this study is that obesity, which is a modifiable comorbidity, had a significant influence on treatment outcomes. In other words, treatment of obesity in tandem with treatment of FM may yield improved patient outcomes compared to treatment of FM alone for patients with comorbid obesity.
.Our data suggests that specific comorbidities may need to be addressed more aggressively by primary and specialty physicians to improve outcomes in FM. In particular, obesity was found to negatively impact the success of an intense cognitive behavioral treatment program and needs further investigation. This finding has important implications in the treatment of FM as obesity has been linked to many other modifiable comorbid conditions. Healthcare providers who take care of these patients should keep in mind the potential impact of associated comorbidities on outcomes and address and modify them if possible.
The original contributions presented in the study are included in the article/supplementary material, further inquiries can be directed to the corresponding author.
The studies involving humans were approved by Mayo Clinic Institutional Review Board. The studies were conducted in accordance with the local legislation and institutional requirements. The participants provided their written informed consent to participate in this study.
FR: Conceptualization, Writing – original draft, Writing – review & editing, Methodology, Project administration. BM: Conceptualization, Writing – original draft, Writing – review & editing. MA: Conceptualization, Methodology, Writing – review & editing. DH: Conceptualization, Data curation, Formal analysis, Investigation, Methodology, Writing – review & editing. MW: Conceptualization, Data curation, Formal analysis, Investigation, Writing – review & editing. BW: Conceptualization, Methodology, Writing – review & editing. AA: Conceptualization, Methodology, Writing – review & editing. AP: Conceptualization, Methodology, Writing – review & editing. DK: Conceptualization, Methodology, Writing – review & editing. BB: Conceptualization, Investigation, Methodology, Writing – original draft, Writing – review & editing.
The author(s) declare that no financial support was received for the research, authorship, and/or publication of this article.
The authors declare that the research was conducted in the absence of any commercial or financial relationships that could be construed as a potential conflict of interest.
All claims expressed in this article are solely those of the authors and do not necessarily represent those of their affiliated organizations, or those of the publisher, the editors and the reviewers. Any product that may be evaluated in this article, or claim that may be made by its manufacturer, is not guaranteed or endorsed by the publisher.
CES-D Score, Center for Epidemiologic Studies Depression Scale; FIQR Score, Fibromyalgia Impact Questionnaire-Revised; PCS Score, Pain Catastrophizing Scale; IBS, Irritable bowel syndrome; GERD, Gastroesophageal reflux; RA, Rheumatoid arthritis
1. Branco, JC , Bannwarth, B , Failde, I , Abello Carbonell, J , Blotman, F , Spaeth, M, et al. Prevalence of fibromyalgia: a survey in five European countries. Semin Arthritis Rheum. (2010) 39:448–53. doi: 10.1016/j.semarthrit.2008.12.003
2. Heidari, F , Afshari, M , and Moosazadeh, M . Prevalence of fibromyalgia in general population and patients, a systematic review and meta-analysis. Rheumatol Int. (2017) 37:1527–39. doi: 10.1007/s00296-017-3725-2
3. Fitzcharles, M-A , Shir, Y , Ablin, JN , Buskila, D , Amital, H , Henningsen, P, et al. Classification and clinical diagnosis of fibromyalgia syndrome: recommendations of recent evidence based interdisciplinary guidelines. Evid Based Complement Altern Med. (2013) 2013:528952. doi: 10.1155/2013/528952
4. Bilge, U , Sari, YE , Balcioglu, H , Yasar Bilge, NS , Kasifoglu, T , Kayhan, M, et al. Prevalence of comorbid diseases in patients with fibromyalgia: a retrospective cross-sectional study. J Pak Med Assoc. (2018) 68:729–32.
5. Wolfe, F , Clauw, DJ , FitzCharles, MA , Goldenberg, DL , Häuser, W , Katz, RL, et al. 2016 revision to the 2010/2011 fibromyalgia diagnostic criteria. Semin Arthritis Rheum. (2016) 46:319–29. doi: 10.1016/j.semarthrit.2016.08.012
6. Borchers, AT , and Gershwin, ME . Fibromyalgia: a critical and comprehensive review. Clin Rev Allergy Immunol. (2015) 49:100–51. doi: 10.1007/s12016-015-8509-4
7. Rehm, SE , Koroschetz, J , Gockel, U , Brosz, M , Freynhagen, R , Tolle, TR, et al. A cross-sectional survey of 3035 patients with fibromyalgia: subgroups of patients with typical comorbidities and sensory symptoms profiles. Rheumatol Oxf Engl. (2010) 49:1146–52. doi: 10.1093/rheumatology/keq066
8. Wolfe, F . Fibromyalgia research criteria. J Rheumatol. (2014) 41:187. doi: 10.3899/jrheum.131224
9. Suso-Ribera, C , García-Palacios, A , Botella, C , and Ribera-Canudas, MV . Pain catastrophizing and its relationship with health outcomes: does pain intensity matter? Pain Res Manag. (2017) 3:9762864. doi: 10.1155/2017/9762864
10. D'Onghia, M , Ciaffi, J , Lisi, L , Mancarella, L , Ricci, S , Stefanelli, N, et al. Fibromyalgia and obesity: a comprehensive systematic review and meta-analysis. Semin Arthritis Rheum. (2021) 51:409–24. doi: 10.1016/j.semarthrit.2021.02.007
11. Jiao, J , Davis III, JM , Cha, SS , Luedtke, CA , Vincent, A , and Oh, TH . Association of rheumatic diseases with symptoms severity, quality of life, and treatment outcome in patients with fibromyalgia. Scand J Rheumatol. (2015) 45:49–56. doi: 10.3109/03009742.2015.1052553
12. Bennett, RM , Friend, R , Jones, KD , Ward, R , Han, BK , and Ross, RL . The revised fibromyalgia impact questionnaire (FIQR); validation and psychometric properties. Arthritis Res Ther. (2009) 11:R120. doi: 10.1186/ar2783
13. Fan, A , Pereira, B , Tourmadre, A , Tatar, Z , Malochet-Guinamand, S , Mathieu, S, et al. Frequency of concomitant fibromyalgia in rheumatic diseases: monocentric study of 691 patients. Semin Arthritis Rheum. (2017) 47:129–32. doi: 10.1016/j.semarthrit.2017.01.005
14. van den Akker, M , Buntinx, F , and Knottnerus, JA . Comorbidity or multimorbidity: What's in a name. A review of literature. Eur J Gen Pract. (1996) 2:65–70. doi: 10.3109/13814789609162146
15. Galvez-Sánchez, CM , Duschek, S , and Reyes Del Paso, GA . Psychological impact of fibromyalgia: current perspectives. Psychol Res Behav Manag. (2019) 12:117–27. doi: 10.2147/PRBM.S178240
16. Su, C-H , Chen, J-H , Lan, J-L , Wang, YC , Tseng, CH , Hsu, CY, et al. Increased risk of coronary heart disease in patients with primary fibromyalgia and those with concomitant comorbidity: a Taiwanese population-based cohort study. PLoS One. (2015) 10:e0137137. doi: 10.1371/journal.pone.0137137
17. Tseng, C-H , Chen, J-H , Wang, Y-C , Lin, MC , and Kao, CH . Increased risk of stroke in patients with fibromyalgia: a population-base cohort study. Medicine (Baltimore). (2016) 95:e2860. doi: 10.1097/MD.0000000000002860
18. Acosta-Manzano, P , Segura-Jiménez, V , Estévez-López, F , Álvarez-Gallardo, IC , Soriano-Maldonado, A , Borges-Cosic, M, et al. Do women with fibromyalgia present higher cardiovascular disease risk profile than healthy women? Clin Exp Rheumatol. (2017) 35:61–7.
19. Lichtenstein, A , Tiosano, S , and Amital, H . The complexities of fibromyalgia and its comorbities. Curr Opin Rheumatol. (2018) 30:94–100. doi: 10.1097/BOR.0000000000000464
20. Vincent, A , Whipple, MO , McAllister, SJ , Aleman, KM , and St Sauver, JL . A cross-sectional assessment of the prevalence of multiple chronic conditions and medication use in a sample of community-dwelling adults with fibromyalgia in Olmstead County Minnesota. BMJ Open. (2015) 5:e006681. doi: 10.1136/bmjopen-2014-006681
21. Walitt, B , Nahin, RL , Katz, RS , Begman, MJ , and Wofe, F . The prevalence and characteristics of fibromyalgia in the 2012 National Health Interview Survey. PLoS One. (2015) 10:e0138024. doi: 10.1371/journal.pone.0138024
22. Hudson, JI , Godenberg, DL , Pope, HG , Keck, PE , and Schlesinger, L . Comorbidity of fibromyalgia with medical and psychiatric disorders. Am J Med. (1992) 92:363–7. doi: 10.1016/0002-9343(92)90265-D
23. Fitzcharles, MA , Perrot, S , and Hauser, W . Comorbid fibromyalgia: a qualitative review of prevalence and importance. Eur J Pain. (2018) 22:1565–76. doi: 10.1002/ejp.1252
24. Arnold, LM , Hudson, JI , Keck, PE , Auchenbach, MB , Javaras, KN , and Hess, EV . Comorbidity of fibromyalgia and psychiatric disorders. J Clin Psychiatry. (2006) 67:1219–25. doi: 10.4088/JCP.v67n0807
25. van den Akker, M , Buntinx, F , Metsemakers, JFM , Roos, S , and Knottnerus, JA . Multimorbidity in general practice: prevalence, incidence, and determinants of co-occuring chronic and recurrent diseases. J Clin Epidemiol. (1998) 51:367–75. doi: 10.1016/S0895-4356(97)00306-5
26. Barnett, K , Mercer, SW , Norbury, M , Watt, G , Wyke, S , and Guthrie, B . Epidemiology of multimorbidity and implications for health care, and medical education: a cross-sectional study. Lancet. (2012) 380:37–43. doi: 10.1016/50140-6736(12)60240-2
27. Bilge, U . Prevalence of comorbid diseases in patients with fibromyalgia: a retrospective cross-sectional study. J Pak Med Assoc. (2018) 68:729–32.
28. Weir, PT , Harlan, GA , Nkoy, FL , Jones, SS , Hegmann, KT , Gren, LH, et al. The incidence of fibromyalgia and its associated comorbidities: a population-based retrospective cohort study based on international classification of diseases, 9th revision codes. J Clin Rheumatol. (2006) 12:124–8. doi: 10.1097/01.rhu.0000221817.46231.18
29. Haviland, MG , Banta, JE , and Przekop, P . Fibromyalgia: prevalence, course, and co-morbidities in hospitalized patient in the United States, 1999–2007. Clin Exp Rheumatol. (2011) 29:S79–87.
30. Sleurs, D , Tebeka, S , Scognamiglio, C , Dubertret, C , and le Strat, Y . Comorbidities of self-reported fibromyalgia in United States adults: a cross-sectional study from the National Epidemiological Survey on alcohol and related conditions (NESARC-III). Eur J Pain. (2020) 24:1471–83. doi: 10.1002/ejp.1585
31. Salidbury, C , Johnson, L , Purdy, S , Valderas, JM , and Montgomery, AA . Epidemiology and impact of multimorbidity in primary care: a retrospective cohort study. Br J Gen Practice. (2011) 61:e12–21. doi: 10.3399/bjgp11X548929
32. Radloff, LS . The CES-D scale: a self-report depression scale for research in the general population. Appl Psychol Meas. (1977) 1:385–401. doi: 10.1177/014662167700100306
33. Devins, GM , Orme, CM , Costello, CG , Binik, YM , Frizzell, B , Stam, HJ, et al. Measuring depressive symptoms in illness populations: psychometric properties of the Center for Epidemiologic Studies Depression (CES-D) scale. Psychol Health. (1988) 2:139–56. doi: 10.1080/08870448808400349
34. Mascarenhas, RO , Bastos Souza, M , Oliveira, MX , Lacerda, AC , Mendonça, VA , Henschke, N, et al. Association of Therapies with Reduced Pain and Improved Quality of life in patients with fibromyalgia. A systemic review and meta-analysis. JAMA Intern Med. (2021) 181:104–12. doi: 10.1001/jamainternmed.2020.5651
35. Sullivan, MJ , Bishop, SR , and Pivik, J . The pain catastrophizing scale: development and validation. Psychol Assess. (1995) 7:524–32. doi: 10.1037/1040-3590.7.4.524
36. Osman, A , Barrios, FX , Kopper, BA , Hauptmann, W , Jones, J , and O'Neill, E . Factor structure, reliability, and validity of the pain catastrophizing scale. J Behav Med. (1997) 20:589–605. doi: 10.1023/A:1025570508954
37. Bruce, BK , Allman, ME , Rivera, FA , Wang, B , Berianu, F , Butendieck, RR, et al. Intensive multicomponent fibromyalgia treatment. A translational study to evaluate effectiveness in routine care delivery. J Clin Rheumatol. (2020) 27:e496–500. doi: 10.1097/RHU.0000000000001555
38. Bilgici, A , Akdeniz, O , Guz, H , and Ulusoy, H . The role of depression and social adjustment in fibromyalgia syndrome. Turk J Phys Med Rehab. (2005) 5:98–102.
39. Bernardy, K , Fuber, N , Kollner, V , and Hauser, W . Efficacy of cognitive-Behavioral therapies in fibromyalgia syndrome — a systematic review and meta-analysis of randomized controlled trials. J Rheumatol. (2010) 37:1991–2005. doi: 10.3899/jrheum.100104
40. Bazzichi, L , Rossi, A , Zirafa, C , Monzani, F , Tognini, S , Dardano, A, et al. Thyroid autoimmunity may represent a predisposition for the development of fibromyalgia. Rheumatol Int. (2012) 32:335–41. doi: 10.1007/s00296-010-1620-1
41. Nishioka, K , Uchida, T , Usui, C , Tanaka, R , Matsushima, T , Matsumoto, Y, et al. Hattori N high prevalence of anti-TSH receptor antibody in fibromyalgia syndrome. Int J Rheum Dis. (2017) 20:685–90. doi: 10.1111/1756-185X.12964
42. Ranganathan, P , Pramesh, CS , and Aggarwal, R . Common pitfalls in statistical analysis: logistic regression. Perspect Clin Res. (2017) 8:148–51. doi: 10.4103/picr
43. Munipalli, B , Allman, M , Chauhan, M , Niazi, SK , Rivera, F , Abril, A, et al. Depression: a modifiable risk factor for poor outcomes in fibromyalgia. J Prim Care Community Health. (2022) 13:215013192211207. doi: 10.1177/21501319221120738
44. Aguglia, A , Salvi, V , and Maina, G . Fibromyalgia syndrome and depressive symptoms: comorbidity and clinical correlates. J Affect Disord. (2011) 128:262–6. doi: 10.1016/j.jad.2010.07.004
45. Løge-Hagen, JS , Sæle, A , Juhl, C , Bech, P , Stenager, E , and Mellentin, AI . Prevalence of depressive disorder among patients with fibromyalgia: systematic review and meta-analysis. J Affect Disord. (2019) 15:1098–105. doi: 10.1016/j.jad.2018.12.001
46. McCrae, CS , Curtis, AF , Craggs, J , Deroche, C , Sahota, P , Siva, C, et al. Protocol for the impact of CBT for insomnia on pain symptoms and central sensitization in fibromyalgia: a randomized controlled trial. BMJ Open. (2020) 10:e033760. doi: 10.1136/bmjopen-2019-033760
47. Bernardy, K , Klose, P , Busch, AJ , Choy, EH , and Häuser, W . Cognitive-behavioral therapies for Fibromyalgia. The Cochrane database of systematic reviews. (2013). CD009796. doi: 10.1002/14651858.CD009796.pub2
48. McCrae, CS , Williams, J , Roditi, D , Anderson, R , Mundt, JM , Miller, MB, et al. Cognitive behavioral treatments for insomnia and pain in adults with comorbid chronic insomnia and fibromyalgia: clinical outcomes from the SPIN randomized controlled trial. Sleep J. (2019) 42:zsy234. doi: 10.1093/sleep/zsy234
Keywords: fibromyalgia FM, comorbidities, outcome domains, multimorbidities, treatment
Citation: Rivera FA, Munipalli B, Allman ME, Hodge DO, Wieczorek MA, Wang B, Abril A, Perlman A, Knight D and Bruce B (2024) A retrospective analysis of the prevalence and impact of associated comorbidities on fibromyalgia outcomes in a tertiary care center. Front. Med. 10:1301944. doi: 10.3389/fmed.2023.1301944
Received: 25 September 2023; Accepted: 08 December 2023;
Published: 15 January 2024.
Edited by:
Maria Jesus Casuso-Holgado, Sevilla University, SpainReviewed by:
Hatice Bodur, Ankara Yıldırım Beyazıt University, TürkiyeCopyright © 2024 Rivera, Munipalli, Allman, Hodge, Wieczorek, Wang, Abril, Perlman, Knight and Bruce. This is an open-access article distributed under the terms of the Creative Commons Attribution License (CC BY). The use, distribution or reproduction in other forums is permitted, provided the original author(s) and the copyright owner(s) are credited and that the original publication in this journal is cited, in accordance with accepted academic practice. No use, distribution or reproduction is permitted which does not comply with these terms.
*Correspondence: Fernando A. Rivera, cml2ZXJhLmZlcm5hbmRvQG1heW8uZWR1
Disclaimer: All claims expressed in this article are solely those of the authors and do not necessarily represent those of their affiliated organizations, or those of the publisher, the editors and the reviewers. Any product that may be evaluated in this article or claim that may be made by its manufacturer is not guaranteed or endorsed by the publisher.
Research integrity at Frontiers
Learn more about the work of our research integrity team to safeguard the quality of each article we publish.