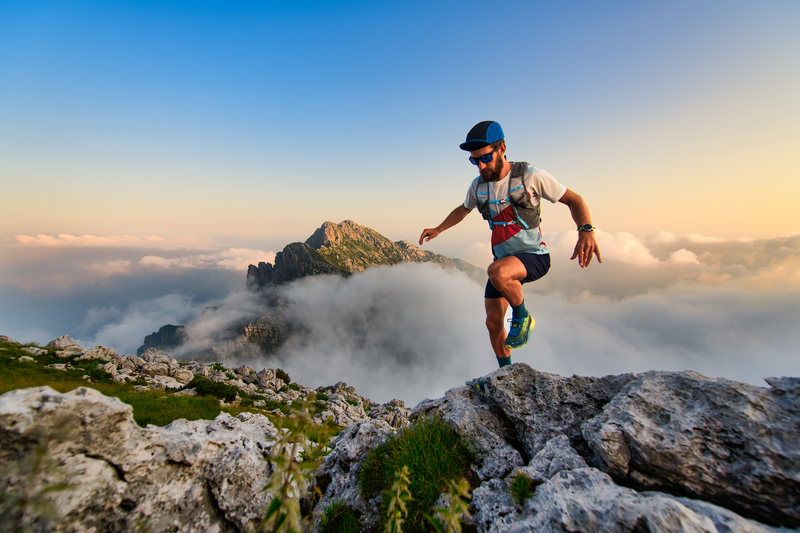
94% of researchers rate our articles as excellent or good
Learn more about the work of our research integrity team to safeguard the quality of each article we publish.
Find out more
EDITORIAL article
Front. Med. , 11 October 2023
Sec. Infectious Diseases: Pathogenesis and Therapy
Volume 10 - 2023 | https://doi.org/10.3389/fmed.2023.1294969
This article is part of the Research Topic Reproducibility and Rigour in Infectious Diseases - Surveillance, Prevention and Treatment View all 7 articles
Editorial on the Research Topic
Reproducibility and rigour in infectious diseases - surveillance, prevention and treatment
The US National Institutes of Health defines scientific rigor as “the strict application of the scientific method to ensure robust and unbiased experimental design, methodology, analysis, interpretation and reporting of results” (1). Scientific rigor can be conveniently divided into six levels (2): (i) insidious (or unethical) rigor, where the researcher fakes data and research findings; (ii) creative rigor, where the researcher reports data that are only consistent with his/her working hypothesis; (iii) careless rigor, where the researcher applies rigor only when asked or where it is easy to apply; (iv) selective rigor, where rigor is applied only to scientific procedures that prior experience dictates to be strictly necessary; (v) careful rigor, where the researcher seeks to avoid misleading and biased results by adhering to standards outlined by funding agencies, journals, or publishers; (vi) enduring rigor, where findings are independently replicated at different levels. Clearly, the application of insidious and creative rigors is an undesirable source of irreproducible findings. In contrast, careful and enduring rigor have a high chance of leading to reproducible findings. In theory, the research community should aim at the research standards and rules dictated by these high levels of rigor.
In Mathematics and related disciplines, research is often thought to operate at the level of careful and enduring rigor due to the logical, coherent, and deductive nature of the mathematical practice. The underlying assumption is that “the use of formal language, axioms and strict rules of inference in mathematics leads to unquestionable mathematical knowledge” (3); note that the infallibility view of mathematical research has been challenged by empirical data (4). In contrast, medical and public health research on infectious diseases is often conducted at a fast pace because of its potential impact on addressing dramatic humanitarian crises, such as the Cholera outbreak in Yemen (5), the Ebola Virus epidemic in West Africa (6), the spread of the Zika Virus in the Americas (7) and, of course, the devastating COVID-19 pandemic (8, 9). The emergency nature of this research exerts additional pressure on a research community that is already living under a “publish-or-perish” mindset due to the limited number of university positions (10). To make the situation worse, the acceptance rate of reviewers willing to review submitted manuscripts is declining over time (11), a trend that has been interpreted as “reviewers' fatigue.” These conditions promote an unintended reduction in the scientific rigor.
In this scenario, we called for participation in a Research Topic dedicated to collect opinions and perspectives on scientific rigor and reproducibility in medical and public health research studies on infectious diseases. The call for participation was held open from 18/11/2021 to 30/11/2022, a time window that was still under the strong influence of the COVID-19 pandemic. Unsurprisingly, almost all the published papers referred to comments on this disease. Below we provided a brief overview of the 6 published papers.
Retrospective analysis of the COVID-19 literature revealed three waves of Research Topics during the pandemic (12). Initially, research efforts focused on the basic epidemiologic and clinical characterization of the disease. This effort then shifted to questions about COVID-19 herd immunity, serologic testing, and asymptomatic characterization. In the latter stages of the pandemic, research shifted to vaccines and their therapeutic efficacy and comparability. It also aimed at predicting new infection waves and the generation of new variants.
Given this historical perspective of the COVID-19 literature, Schwab et al. focused on the rigor of estimating the mortality rate during the first and second waves of COVID-19 in Switzerland. This study found SARS-CoV-2 in lung tissues from autopsies of deceased individuals who were not tested for the virus. The main conclusion of the study is that the COVID-19 mortality rate is likely to be underestimated. Saunders et al. then discussed the claims of an eventual causal relationship between SARS-CoV-2 infection and hearing symptoms. This study identified several problems in the published studies, such as the reliance on self-reported data, the presence of recall bias and potential nocebo effects, and the use of non-COVID-19 pseudo-control groups. Hence, claims of a possible causal effect of SARS-CoV-2 infection on auditory symptoms should be revised.
Three publications commented on issues related to SARS-CoV-2 vaccines. Günther et al. identified an inconsistency between the reported mortality rate from three published vaccine trials and the same rate predicted from the German general population. The authors also provided statistical evidence that the trials were overly optimistic about the safety/efficacy of the vaccines by focusing on total number of people vaccinated rather than the entire cohort. These authors called for improved reporting of mortality in pivotal clinical trials and for the data to be made available upon publication. Also in a clinical trial setting, Wei et al. investigated the theoretical properties of confidence interval estimation for vaccine efficacy in the presence of imperfect COVID-19 diagnostic testing. These authors also provided guidelines that are important for obtaining reliable results in terms of the respective interval estimation. In the third study, Bourdon and Pantazatos commented on the statistical errors in the calculation of the risk of myocarditis in unvaccinated and vaccinated individuals from a large-scale study. Given these statistical errors, the authors recommended that the findings of the original study should not be used to support any public health policy.
Finally, Orlando et al. identified a misstatement in the reporting of lost-to-follow-up data in a clinical trial evaluating chelation therapy. In their comment, they shared the attempt to submit an erratum and/or expression of concern and its refusal by the journal that published the original trial. Although their comment does not relate to scientific rigor in infectious disease research, it is worth noting as it might be applicable to all areas of science.
The most positive aspect of this Research Topic is that all the comments did not suggest any insidious or creative rigors that could indicate ethical issues or scientific misconduct. A less positive note is the recurrent problems in terms of study design, data quality and population representativeness, and the application of sound statistical methods (13–16). We speculate that these problems result from the complexity of today's science combined with insufficient statistical skills within research teams (Figure 1). The lack of statistical skills is evident in the current research culture of consulting biostatisticians, data scientists, bioinformaticians, and mathematical modelers as a last resort (for example, when the peer-review process is already ongoing). This culture can be traced back to 1938, when Fisher famously wrote (17): “To consult the statistician after an experiment finished is often merely to ask him to conduct a post-mortem examination. He can perhaps say what the experiment died of.” Unfortunately, this culture is aligned with a careless rigor where a given research team randomly applies rigor only when necessary or if asked to by reviewers. It can be argued that funding limitations lead applicants to prioritize budgets for laboratory or field activities rather than data analysis. From this perspective, adherence to the highest standards of scientific rigor correlates with higher research costs and training, and thus the costs of careful and sustained rigors might only be affordable by successful institutions and research teams working in developed countries (18). This situation can be illustrated by the molecular surveillance of drug resistance in several infectious diseases. Rigorous surveillance might encompass the execution of massive sequencing efforts in a large number of biological samples, as demonstrated by studies on malaria and tuberculosis (19, 20). Researchers from developing countries might find difficult to conduct these surveillance studies with high scientific rigor on their own due to limited capacity in bioinformatics, genomics, statistics, and mathematical modeling. On the one hand, such a situation might set a strong foundation for collaborative work between researchers from developing and developed countries. On the other hand, the pursuit of a high standard of rigor might intentionally create a leadership bias toward researchers from developed countries who have the capacity and experience to conduct cutting-edge research. In this scenario, researchers from developing countries might be seen as sole data providers and secondary research players. Irrespective of this complex discussion, researchers on infectious diseases should actively seek out collaborators with more quantitative inclinations and make them real members (and not just consultants) of research teams. On the other hand, biostatisticians and related professionals might actively seek to improve their leadership skills to strengthen their collaborative and networking capacities (21). If both research communities go hand in hand, science should gain in terms of scientific rigor and reproducibility.
Figure 1. The relationship between scientific rigor (divided into six levels), statistical (and quantitative) skills, and the chance of scientific reproducibility.
FW: Writing—original draft, Writing—review and editing. NS: Writing—original draft, Writing—review and editing. FW and NS designed Figure 1 using images downloaded from Stock Adobe (https://stock.adobe.com/at/) under an educational institution license provided by FH Joanneum University of Applied Sciences, Graz, Austria.
NS acknowledges partial funding from FCT—Fundação para a Ciência e Tecnologia, Portugal (Grant ref. UIDB/00006/2020).
We thank the Frontiers in Medicine and Frontiers in Public Health for giving us the opportunity to handle and organize this Research Topic.
The authors declare that the research was conducted in the absence of any commercial or financial relationships that could be construed as a potential conflict of interest.
The author(s) declared that they were an editorial board member of Frontiers, at the time of submission. This had no impact on the peer review process and the final decision.
All claims expressed in this article are solely those of the authors and do not necessarily represent those of their affiliated organizations, or those of the publisher, the editors and the reviewers. Any product that may be evaluated in this article, or claim that may be made by its manufacturer, is not guaranteed or endorsed by the publisher.
1. Enhancing Reproducibility through Rigor and Transparency. Available online at: https://grants.nih.gov/policy/reproducibility/index.htm (accessed September 12, 2023).
2. Hofseth LJ. Getting rigorous with scientific rigor. Carcinogenesis. (2018) 39:21–5. doi: 10.1093/carcin/bgx085
3. Frans J, Kosolosky L. Mathematical proofs in practice: revisiting the reliability of published mathematical proofs. Theoria. (2014) 29:345–60. doi: 10.1387/theoria.10758
4. Löwe B. Measuring the agreement of mathematical peer reviewers. Axiomathes. (2022) 32:1205–19. doi: 10.1007/s10516-022-09647-x
5. Federspiel F, Ali M. The cholera outbreak in Yemen: lessons learned and way forward. BMC Public Health. (2018) 18:1–8. doi: 10.1186/s12889-018-6227-6
6. Delamou A, Delvaux T, El Ayadi AM, Beavogui AH, Okumura J, Van Damme W, et al. Public health impact of the 2014–2015 Ebola outbreak in West Africa: seizing opportunities for the future. BMJ Glob Heal. (2017) 2:e000202. doi: 10.1136/bmjgh-2016-000202
7. Sharma V, Sharma M, Dhull D, Sharma Y, Kaushik S, Kaushik S. Zika virus: an emerging challenge to public health worldwide. Can J Microbiol. (2020) 66:87–98. doi: 10.1139/cjm-2019-0331
8. Nunn CL. COVID-19 and evolution, medicine, and public health. Evol Med Public Heal. (2023) 11:42–4. doi: 10.1093/emph/eoad002
9. DeSalvo K, Hughes B, Bassett M, Benjamin G, Fraser M, Galea S, Gracia JN, Howard J. Public health COVID-19 impact assessment: lessons learned and compelling needs. NAM Perspect. (2021) 2021:4. doi: 10.31478/202104c
10. Neill US. Publish or perish, but at what cost? J Clin Invest. (2008) 118:2368–2368. doi: 10.1172/JCI36371
11. Vesper I. Peer reviewers unmasked: largest global survey reveals trends. Nature. (2018). doi: 10.1038/d41586-018-06602-y [Epub ahead of print].
12. Serio C Di, Malgaroli A, Ferrari P, Kenett RS. The reproducibility of COVID-19 data analysis: paradoxes, pitfalls, and future challenges. PNAS Nexus. (2022) 1:1–8. doi: 10.1093/pnasnexus/pgac125
13. Hirschauer N, Grüner S, Mußhoff O, Becker C, Jantsch A. Inference using non-random samples? Stop right there! Significance. (2021) 18:20–4. doi: 10.1111/1740-9713.01568
14. García-Berthou E, Alcaraz C. Incongruence between test statistics and P values in medical papers. BMC Med Res Methodol. (2004) 4:1–5. doi: 10.1186/1471-2288-4-13
15. Grabowska AD, Lacerda EM, Nacul L, Sepúlveda N. Review of the quality control checks performed by current genome-wide and targeted-genome association studies on myalgic encephalomyelitis/chronic fatigue syndrome. Front Pediatr. (2020) 8:293. doi: 10.3389/fped.2020.00293
16. Rudolph JE, Zhong Y, Duggal P, Mehta SH, Lau B. Defining representativeness of study samples in medical and population health research. BMJ Med. (2023) 2:e000399. doi: 10.1136/bmjmed-2022-000399
17. Fisher RA. Presidential address to the first indian statistical congress. Sankhya. (1938) 4:14–7.
18. Wyatt MD, Pittman DL. Potential unintended consequences of getting rigorous with scientific rigor. Carcinogenesis. (2018) 39:26–7. doi: 10.1093/carcin/bgx101
19. Beshir KB, Muwanguzi J, Nader J, Mansukhani R, Traore A, Gamougam K, et al. Prevalence of Plasmodium falciparum haplotypes associated with resistance to sulfadoxine–pyrimethamine and amodiaquine before and after upscaling of seasonal malaria chemoprevention in seven African countries: a genomic surveillance study. Lancet Infect Dis. (2023) 23:361–70. doi: 10.1016/S1473-3099(22)00593-X
20. Zignol M, Cabibbe AM, Dean AS, Glaziou P, Alikhanova N, Ama C, et al. Genetic sequencing for surveillance of drug resistance in tuberculosis in highly endemic countries: a multi-country population-based surveillance study. Lancet Infect Dis. (2018) 18:675–83. doi: 10.1016/S1473-3099(18)30073-2
Keywords: scientific method, deficient statistical methods, COVID-19, long-COVID, degree of scientific rigor
Citation: Westermeier F and Sepúlveda N (2023) Editorial: Reproducibility and rigour in infectious diseases - surveillance, prevention and treatment. Front. Med. 10:1294969. doi: 10.3389/fmed.2023.1294969
Received: 15 September 2023; Accepted: 02 October 2023;
Published: 11 October 2023.
Edited and reviewed by: Shisan Bao, The University of Sydney, Australia
Copyright © 2023 Westermeier and Sepúlveda. This is an open-access article distributed under the terms of the Creative Commons Attribution License (CC BY). The use, distribution or reproduction in other forums is permitted, provided the original author(s) and the copyright owner(s) are credited and that the original publication in this journal is cited, in accordance with accepted academic practice. No use, distribution or reproduction is permitted which does not comply with these terms.
*Correspondence: Nuno Sepúlveda, Ti5TZXB1bHZlZGFAbWluaS5wdy5lZHUucGw=
Disclaimer: All claims expressed in this article are solely those of the authors and do not necessarily represent those of their affiliated organizations, or those of the publisher, the editors and the reviewers. Any product that may be evaluated in this article or claim that may be made by its manufacturer is not guaranteed or endorsed by the publisher.
Research integrity at Frontiers
Learn more about the work of our research integrity team to safeguard the quality of each article we publish.