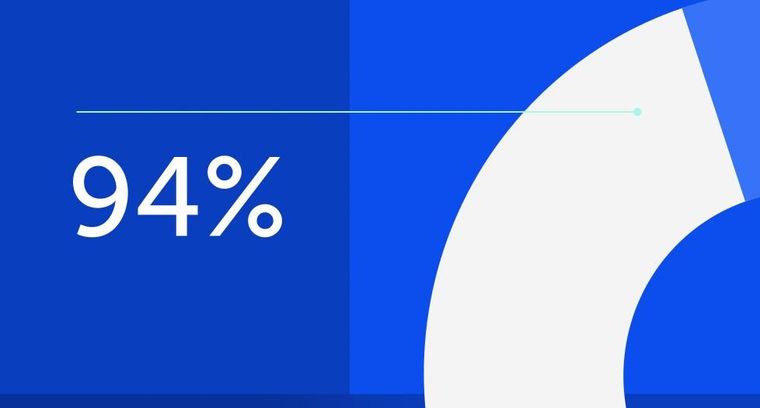
94% of researchers rate our articles as excellent or good
Learn more about the work of our research integrity team to safeguard the quality of each article we publish.
Find out more
ORIGINAL RESEARCH article
Front. Med., 29 November 2023
Sec. Hepatobiliary Diseases
Volume 10 - 2023 | https://doi.org/10.3389/fmed.2023.1274961
Background: Sepsis is a severe and common complication of liver transplantation (LT) with a high risk of mortality. However, effective tools for evaluating its risk factors are lacking. Therefore, this study identified the risk factors of early post-liver transplantation sepsis and established a nomogram.
Methods: We analyzed the risk factors of post-liver transplantation sepsis in 195 patients. Patients with infection and a systemic inflammatory response syndrome (SIRS) score ≥ 2 were diagnosed with sepsis. The predictive indicators were screened with the least absolute shrinkage and selection operator (LASSO) and collinearity analyses to develop a nomogram. The prediction performance of the new nomogram model, Sequential Organ Failure Assessment (SOFA) score, and Modified Early Warning Score (MEWS) was compared through assessment of the area under the curve (AUC), decision curve analysis (DCA), net reclassification index (NRI), and integrated discrimination improvement (IDI).
Results: The nomogram was based on postoperative heart rate, creatinine concentration, PaO2/FiO2 ratio < 400 mmHg, blood glucose concentration, and international normalized ratio. The AUC of the nomogram, the SOFA score, and MEWS were 0.782 (95% confidence interval CI: 0.716–0.847), 0.649 (95% CI: 0.571–0.727), and 0.541 (95% CI: 0.469–0.614), respectively. The DCA curves showed that the net benefit rate of the nomogram was higher than that of the SOFA score and MEWS. The NRI and IDI tests revealed better predictive performance for the nomogram than SOFA score and MEWS.
Conclusion: Heart rate, creatinine concentration, PaO2/FiO2, glucose concentration, and international normalized ratio should be monitored postoperatively for patients at risk of post-liver transplantation sepsis. The nomogram based on the aforementioned risk factors had a better predictive performance than SOFA score and MEWS.
Liver disease leads to approximately 2 million deaths each year globally (1), imposing a financial burden on the healthcare system. Conventional medical treatments can improve the prognosis in some patients. Of these, liver transplantation (LT) is the most effective life-saving treatment for end-stage liver diseases (2); however, the management of postoperative complications remains challenging. Infections and sepsis in particular are the primary causes of postoperative mortality during the first month following LT (3).
Sepsis is a life-threatening organ dysfunction caused by the dysregulated host response to infection (4). Patients who develop infections or sepsis after LT may experience deterioration in their health. This may prolong hospitalization and increase financial burden, which may result in severe outcomes and even death. A retrospective analysis revealed that the incidence of sepsis after LT is as high as 50%–80%, and that sepsis-related deaths account for 50–90% of all postoperative mortality (5–9). According to the Surviving Sepsis Campaign recommendations, early fluid resuscitation and timely initiation of antibiotics are cornerstones for the successful treatment of patients with sepsis (10). Therefore, early and accurate prediction of sepsis can help clinicians to intervene promptly and significantly improve the prognosis of LT. Studies have reported that exhaled nitric oxide, aerobic capacity (11), plasma amino acid profile (12), and muscle wasting (13) are indicators for sepsis after LT. However, these indicators cannot be easily identified in clinical practice. Therefore, simple, precise, and specific predictive models for early detection of post-LT sepsis are needed.
This study aimed to analyze the risk factors for early sepsis and develop a nomogram for early sepsis after LT.
In this study, we retrospectively enrolled 227 consecutive patients who underwent LT at the Ningbo Medical Center, LiHuiLi Hospital (Zhejiang, China), between January 2016 and December 2021. The study was approved by the hospital ethics committee (KY2022PJ026).
Patients were excluded if they met the following criteria: age of <18 years or > 65 years, death during surgery or within 3 days after LT (n = 3), or incomplete clinical data (n = 29). Finally, 195 patients were enrolled and classified into the sepsis and sepsis-free groups (Figure 1). Patients with infection and a systemic inflammatory response syndrome (SIRS) score ≥ 2 were diagnosed with sepsis. Infection is defined as a microbial phenomenon characterized by an inflammatory response to the presence of microorganisms or the invasion of normally sterile host tissue by those organisms. The diagnostic criteria for SIRS are two or more of the following clinical manifestations: heart rate > 90 bpm; body temperature<36°C or > 38°C; white blood cell count <4 × 109/L or > 12 × 109 /L; respiratory rate > 20 cycles/min or arterial partial pressure of carbon dioxide (PaCO2) <32 mmHg (1 mmHg = 0.133 kPa) (14).
Figure 1. Study flow chart. The enrolled patients were divided into sepsis and sepsis-free groups within two weeks after LT.
During the perioperative period, β-lactam antibiotics were used to prevent postoperative infection in most patients.
Recipients were administered baliximab 30 min before LT and on day 4 postoperatively. Methylprednisolone (500 mg) was administered once at the beginning of the hepatic-free period. The postoperative immunosuppressive regimen comprised tacrolimus in combination with mertilmicosporin.
The artificial liver was used to treat patients with end-stage liver disease before LT. The main modes included plasma replacement or a combination of plasma replacement and continuous renal replacement therapy (CRRT). In the sepsis group, plasma replacement alone was employed for eight cases and plasma replacement combined with CRRT for ten. In contrast, in the sepsis-free group, three cases utilized plasma replacement alone, whereas two utilized plasma replacement in conjunction with CRRT.
The general clinical data included age, sex, primary disease, surgical method, invasive operations (including surgery, interventional therapy, artificial liver, kidney replacement therapy, and various forms of paracentesis), and artificial liver treatment within 1 month before LT. In our study, the primary diseases that necessitated LT included hepatocellular carcinoma (including hepatocellular carcinoma complicated with liver cirrhosis), liver cirrhosis, and acute liver failure, among others.
The preoperative laboratory indicators were as follows: heart rate (HR), mean arterial pressure (MAP), white blood cell (WBC) count, Neutrophil to Lymphocyte ratio (NLR), hemoglobin concentration (Hb), platelet count (PLT), albumin concentration (ALB), total bilirubin (TBil), creatinine concentration (Cr), prothrombin time (PT), international normalized ratio (INR), serum sodium concentration (Na+), and model for end-stage liver disease (MELD). The intraoperative indicators were as follows: anhepatic phase, blood loss, and plasma transfusion volume. The postoperative laboratory indicators were the first values of the following measures on postoperative day (POD) 3: HR, MAP, Systolic blood pressure (SBP), shock index (SI = HR-to-SBP ratio), use of hyperensort (including norepinephrine, dopamine, and mesalamine), WBC, NLR, Hb, PLT, ALB, TBil, Cr, PT, INR, arterial partial oxygen pressure (PaO2), fraction of inspired O2 (FiO2), PaO2-to-FiO2 ratio (PaO2/FiO2), lactic acid concentration (Lac), C-reactive protein concentration (CRP), Blood FK506 concentration, blood glucose concentration (Glu), abnormal liver blood supply (abnormalities of hepatic arteriovenous blood flow and hepatic ischemia), and hydropericardium. Laboratory results were collected on the day before transplantation and POD 3. Notably, CRP peaked within 3 days after LT, and blood concentration of FK506 was collected from patients 7 days after LT. We also investigated the characteristics of pathogenic bacteria distribution in patients with sepsis after LT.
We employed least absolute shrinkage and selection operator (LASSO) regression to screen the variables and generalized linear modeling to obtain regression coefficients, odds ratios (OR), and 95% confidence intervals (CI) for the modeled variables.
The area under the curve (AUC) was used to evaluate the discriminative ability of the nomogram. Hosmer–Lemeshow (H-L) test was used to assess goodness-of-fit. Calibration curves were plotted based on the predicted and true probabilities. We assessed the nomogram performance and compared it to SOFA scores and MEWS using metrics such as the area under the curve (AUC), decision curve analysis (DCA), net reclassification index (NRI) and integrated discrimination index (IDI). NRI was calculated using the nricens package. The cut points were selected as 0.3 and 0.7, and 1,000 resampling iterations were performed using the bootstrap method (niter = 1,000). The updown parameter was configured to assess categories, distinguishing between low, medium and high risk categories.
Statistical analyses were performed with R 4.0.3 (https://www.r-project.org/; The R Foundation). Normally distributed variables, non-normally distributed variables, and categorical variables were expressed as mean and standard deviation, median (first quartile, third quartile), and frequency (percentage), respectively. The independent t-test was used to compare the normally distributed variables. Mann–Whitney U-test was applied to the non-normally distributed variables, and the chi-squared test was used to compare the categorical variables. Univariate analysis was used to screen for the risk factors of sepsis, and the Youden index was used to determine the optimal cutoff values for the independent risk factors. p < 0.05 was considered statistically significant.
We included 195 patients (157 males and 38 females) in this study. The average age was 52.29 ± 9.45 years, and the indications for LT were liver cancer (n = 91, 46.67%), liver cirrhosis (n = 96, 49.23%), liver failure (n = 5, 2.56%), and other diseases (n = 3, 1.54%). The pathogenic factors included viral hepatitis B (n = 147, 75.38%), alcoholic liver injury (n = 12, 6.15%), autoimmune liver disease (n = 15, 7.69%), and others (n = 21, 10.77%). The main surgical methods used were classical orthotopic LT (n = 144, 73.85%), piggyback liver allograft transplantation (n = 26, 13.34%), split LT (n = 17, 8.72%), and living donor LT (n = 8, 4.10%). A total of 116 recipients of LT developed sepsis within 1 month, with 115 cases occurring within the first 2 weeks after transplantation. The median time to sepsis onset was 5 days.
As shown in Supplementary Table S1, there were no significant differences between the sepsis and sepsis-free groups (p > 0.05) in gender, age, preoperative infection, prevalence of diabetes mellitus, invasive operations, preoperative indicators [WBC (z = −0.676, p = 0.499), NLR (z = −0.132, p = 0.895), Hb (t = 1.158, p = 0.254), PLT (z = 0.588, p = 0.556), ALB (t = −0.392, p = 0.699), TBil (z = −1.351, p = 0.176), Na+ (t = 1.198, p = 0.232), Cr (z = 0.464, p = 0.642), PT (t = −0.733, p = 0.464), INR (z = −0.788, p = 0.431), HR (t = 1.099, p = 0.273) and MAP (t = 0.749, p = 0.455)], intraoperative indicators [anhepatic phase (z = −1.534, p = 0.125), blood loss (z = −1.726, p = 0.084), and plasma transfusion volume (z = −1.245, p = 0.213)], postoperative indicators [SBP (t = 0.120, p = 0.903), use of hyperensort (χ2 = 2.953, p = 0.086), MAP (t = −0.079, p = 0.067), WBC (t = −1.941, p = 0.067), Hb (t = 0.996, p = 0.322), PLT (t = 1.169, p = 0.231), CRP (z = −1.498, p = 0.134), NLR (z = −0.168, p = 0.536), Blood FK506 concentration (t = 1.299, p = 0.195), abnormal liver blood supply (χ2 = 0.039, p = 0.844), hydropericardium (χ2 = 2.898, p = 0.089), and length of intensive care unit (ICU) stay (t = −1.902, p = 0.105)]. However, in the sepsis group, the proportion of patients who received artificial liver treatment before LT (χ2 = 4.00, p = 0.045), the proportion of patients with a MELD score > 20 before LT (χ2 = 3.92, p = 0.048), and the proportion of patients with PaO2/FiO2 < 400 mmHg (χ2 = 14.23, p < 0.001) after LT were higher than those in the sepsis-free group. These differences between groups were statistically significant. Meanwhile, HR (t = −3.56, p = 0.001), SI (t = −2.79, p = 0.007), TBil (z = −2.71, p = 0.007), Cr (z = −2.99, p = 0.003), INR (z = −4.26, p < 0.001), Lac (z = −3.74, p < 0.001), and Glu (z = −2.35, p = 0.012) were significantly higher for the sepsis group on POD 3 than that for the sepsis-free group (p < 0.05). Conversely, the postoperative ALBs for the sepsis group were significantly lower than those for the sepsis-free group (t = −0.39, p = 0.046).
AUC was used to evaluate the efficiency of the indicators in discriminating between sepsis and sepsis-free groups. The Youden index, which is defined as the maximum vertical distance between the receiver operating characteristic (ROC) curve and the diagonal line, was used to determine the optimal cutoff value. As shown in Table 1, the optimal cutoff values for the risk factors of sepsis were ALB <33.7 g/L, HR ≥ 90 bpm, HR/SBP ≥ 0.64, TBil ≥79.25 μmol/L, Cr ≥ 89 μmol/L, INR ≥ 1.52, Lac ≥2.75 mmol/L, Glu ≥ 16.50 mmol/L, and PaO2/FiO2 < 400 mmHg on the third day after LT (p < 0.05).
Table 1. Statistical differences in indicators between the sepsis-free and sepsis groups after liver transplantation.
During the LT perioperative period, β-lactam antibiotics (n = 75, 93.80%), enzyme inhibitors (n = 2, 2.50%), β-lactam antibiotics combined with antifungals (n = 2, 2.50%), and β-lactam antibiotics combined with glycopeptide antibiotics (n = 1, 1.30%) were used as prophylactic treatment for the sepsis-free group. Furthermore, β-lactam antibiotics (n = 103, 89.60%), enzyme inhibitors (n = 1, 0.90%), β-lactam antibiotics in combination with antifungals (n = 10, 8.70%), and β-lactam antibiotics in combination with glycopeptide antibiotics (n = 1, 0.90%) were used as prophylactic treatment for the sepsis group. These differences between two groups were not statistically significant (χ2 = 3.20, p = 0.271).
In the sepsis group, 19 (16.52%) of the patients had mixed infections with two or more bacteria, 13 (11.30%) had infections with multiple flora at a single site, and 33 (28.70%) had infections at multiple sites. The most common being gram-negative strains (n = 26, 22.60%) dominated by Acinetobacter baumannii (n = 8, 30.77%, including five multi/pan-drug resistant strains); gram-positive strains (n = 15, 13.04%), dominated by Enterococcus faecium (n = 10, 66.67%), and fungi (n = 17, 14.78%), dominated by Candida albicans (n = 4, 23.53%). The most common site of infection in patients with sepsis was the lungs (n = 70, 60.87%).
We used LASSO logistic regression analysis to screen for the predictors of sepsis. We identified five significant predictors (Table 2), including postoperative HR (95% CI: 1.006–1.050, p = 0.012), postoperative Cr (95% CI: 1.000–1.023, p = 0.067), postoperative INR (95% CI: 2.396–25.568, p = 0.001), postoperative PaO2/FiO2 < 400 mmHg (95% CI: 1.527–5.681, p = 0.001), and postoperative Glu (95% CI: 1.000–1.147, p = 0.089), which were used to establish the prediction nomogram for sepsis within 2 weeks after LT (Figure 2).
Figure 2. Nomogram to predict the risk of sepsis in patients within two weeks following LT. The nomogram assigned a specific score on the point scale axis for each variable, and these individual scores were summed to calculate the total score. This total score can be projected to estimate the risk of early sepsis after LT. HR, heart rate; Cr, creatinine; INR, international normalized ratio; PaO2/FiO2, PaO2-to-FiO2 ratio; Glu, blood glucose.
ROC analysis was used to evaluate the discriminatory power of the nomogram, and the AUC of the nomogram was 0.782 (95% CI, 0.716–0.847) (Figure 3A). The H-L test showed a good fit of the actual data (χ2 = 9.793, p < 0.05). Furthermore, the calibration plots demonstrated a high level of concordance between the predicted and actual probabilities (Figure 4).
Figure 3. Comparison of the performances of the nomogram, SOFA score and MEWS in predicting sepsis. (A) ROC curves of the nomogram, SOFA score and MEWS in predicting risk of sepsis within two weeks after LT and (B) DCA for evaluation of the validity ability of the nomogram, SOFA score and MEWS.
Decision curve analysis (DCA) was used to assess the validity and clinical usefulness of the nomogram. The decision curve of the nomogram was situated above the two extreme curves (Figure 3B), indicating an overall net benefit within a broad threshold probability range of 30%–80%.
The AUCs of the SOFA scores and MEWS were 0.649 (95% CI, 0.571–0.727) and 0.541 (95% CI, 0. 469–0.614), respectively, indicating that the nomogram demonstrated superior predictive performance for early sepsis (Figure 3A). The DCA curves showed that the net benefit rate of the nomogram was higher than that of the SOFA score and MEWS with the threshold in the range of 0.30–0.95 (Figure 3B). Further, the nomogram had a markedly higher discriminative power than the SOFA score and MEWS, with NRI values of 1.973 (95% CI: 1.668–1.823, p < 0.001) and 1.973 (95% CI: 1.941–2.000, p < 0.001), and IDI values of 0.171 (95% CI: 0.118–0.225, p < 0.001) and 0.222 (95% CI: 0.160–0.284, p < 0.001), respectively. Taken together, the nomogram developed in this study may be effective for predicting early sepsis in clinical practice.
As LT is one of the most effective treatments for end-stage liver diseases, 34,694 LTs were performed globally in 2021, according to the International Registry on Organ Donation and Transplantation. However, the complications of LT, especially sepsis, are associated with a high risk of mortality (15, 16). A single-center retrospective study showed that the incidence of early sepsis after LT was up to 67%, and the median time to infection was 9 days (6, 17). The present study enrolled 195 patients who underwent LT, and found the median time to sepsis to be 5 days and the prevalence of early sepsis after LT to be 59%, which is much higher than the incidence of sepsis in other diseases in the intensive care unit (1, 18). The high incidence of sepsis may be related to intraoperative ischemia–reperfusion injury (19, 20), length of ICU stay (21), invasive procedures (22, 23), and high postoperative doses of hormones and immunosuppressants (24), among others. Early source control in sepsis (<6 h) is associated with a reduced risk-adjusted odds of 90-day mortality (25–27). Therefore, clinical data on POD 3 were collected in the present study due to the shorter incubation period (28). Bacterial infections are prevalent at first 30 days after LT (29, 30), which account for up to 70% of all infections in liver transplant recipients (31). In this study, Enterococcus faecalis was the most common pathogen isolated in the sepsis group after LT (17.24%). Enterococcus faecium bloodstream infections (BSI) is an independent risk factor for death compared to other bacterial BSI. And the mean 30-day mortality rate for Enterococcus faecium BSI is high to 25% (32). However, differentiating sepsis from non-infectious conditions is difficult, especially in patients who may develop systemic inflammatory response syndrome after LT. Therefore, establishing an effective predictive model for sepsis after LT is critical.
A previous study reported that the SOFA score and CRP can be combined to evaluate the risk of sepsis after LT (33). However, this is controversial because of the use of sedative and analgesic drugs after LT, which could affect the Glasgow Coma Scale score included in the SOFA score. Meanwhile, the most commonly used markers of inflammation such as C-reactive protein and procalcitonin, are unable to represent the development of sepsis because of factors affecting preoperative recipient status and the intraoperative and postoperative courses, particularly during the early stages after LT (34, 35). The MEWS is a commonly used scoring system for sepsis severity (36). Nonetheless, its applicability in predicting sepsis following LT may be limited due to its inclusion of a consciousness score. As mentioned above, there was no significant difference in the CRP between the sepsis and sepsis-free groups in our study, which may be attributed to the severe trauma caused by LT, high doses of antibiotics, and immunosuppressants that prevent inflammatory indicators from reflecting the infection status of the patient.
Nomograms have emerged as valuable tools in various fields, enabling clinicians to predict the likelihood of clinical events based on individual variables. In this study, we identified postoperative HR, Cr, PaO2/FiO2 < 400 mmHg, Glu, and INR as independent risk factors for sepsis after LT. The AUC for the nomogram was 0.782, demonstrating excellent performance in distinguishing between patients who will or will not develop sepsis within 2 weeks after LT.
Tachycardia is one of the most important indicators in the diagnostic criteria of sepsis (14) and is widely acknowledged as a robust indicator of sepsis-related morbidity and mortality (37–39). By analyzing the ROC curves, we determined that an HR threshold of 90 bpm was ideal for predicting the risk of sepsis, which is consistent with previous reports of research on multiorgan dysfunction syndrome (40, 41). Tachycardia is an early and reliable sign of hypotension that helps in sustaining sufficient cardiac output and circulating volume (10). However, in our study, the increase in heart rate was not accompanied by hypotension. Hypotension is a condition that may be associated with inflammation, which leads to vasodilatation, a decrease in preload, and an overall decrease in cardiac output (42). This results in the activation of sympathetic nerves, leading to compensatory tachycardia and vasoconstriction.
A previous study showed the PaO2/FiO2 ratio is crucial for assessing the risk of mortality and severity of sepsis (43, 44). Our study also showed that PaO2/FiO2 ratios below 400 mmHg were significant risk indicators for sepsis, and 88.7% of patients in the sepsis group had PaO2/FiO2 ratios below 400 mmHg. Previously, preoperative and intraoperative inflammatory response syndrome, perioperative massive blood transfusion, and ischemia–reperfusion injury have been identified as risk factors for postoperative hypoxemia (45). Rational interventions and timely treatment of hypoxemia are essential to reduce the morbidity of sepsis.
Based on the laboratory test results, Glu has emerged as a potential risk factor for sepsis (46, 47). One study reported that the peak Glu can be used as an adjunctive tool for mortality risk stratification in critically ill patients with sepsis (48, 49). Patients with end-stage liver disease have impaired glucose metabolism (50). In most cases, Glu rapidly decreases within 2 days after LT (51). Our study found that the risk of sepsis was significantly increased when Glu was ≥16.5 mmol/L on POD 3. Controlling hyperglycemia can be a challenging task, however employing a number of measures preoperatively can help control a patient’s intraoperative and postoperative glucose levels and reduce postoperative infections (52).
INR is also an effective sepsis screening indicator and prognostic factor (53, 54). During the initial stages of infection, coagulation acts as a natural defense mechanism in an attempt to limit the pathogen and prevent its spread to systemic circulation (48, 55). Studies have shown that coagulation function is abnormal in severe infections, and the main reason for its formation is the imbalance between the formation and clearance of fibrin in the blood vessels (56). Patients with INR exceeding 1.52 on POD 3 had considerably heightened susceptibility to sepsis—70.4% of the patients in this study had INR exceeding 1.52. In addition, renal dysfunction is a prevalent complication of LT. A recent study by Mehta et al. showed that 40% of critically ill patients developed sepsis after acute kidney injury (57). In this study, patients with sepsis had a high Cr, with a value of 93.2 μmol/L on POD 3. In contrast, the patients in the sepsis-free group had a lower Cr (73.4 μmol/L) than the sepsis group (93.2 μmol/L). Furthermore, a significant association was established between Cr exceeding 89 μmol/L and the likelihood of sepsis.
To further validate the predictive performance of the sepsis nomogram developed in this study, we compared its performance to that of the SOFA score and MEWS. The nomogram demonstrated a higher prediction accuracy and net benefit than the SOFA score and MEWS. These suggest that our nomogram is practical and viable for predicting postoperative sepsis in patients undergoing LT. The high prognostic value of the nomogram is likely attributable to its ability to combine the prognostic values of renal, respiratory, and liver insufficiency. Furthermore, this study highlighted the potential benefits of personalized medicine and the value of predictive models in improving patient outcomes. Sepsis risk assessment is no longer abstract, and clinical prevention and treatment should focus on patients with these characteristics.
This study had a few limitations. First, the data were retrospectively collected from the medical records of the patients, which may not have contained all the necessary information for analysis. Second, the generalization of the study findings may be limited due to the small sample size, and external validation is required to validate our findings.
In conclusion, this study identified the risk factors for sepsis within 2 weeks after LT and developed a novel nomogram for predicting sepsis. This nomogram can facilitate early identification of sepsis and enable timely clinical intervention, thereby improving the prognosis of patients undergoing LT.
The original contributions presented in the study are included in the article/Supplementary material, further inquiries can be directed to the corresponding authors.
The studies involving humans were approved by Ningbo Medical Centre Lihuili Hospital. The studies were conducted in accordance with the local legislation and institutional requirements. The ethics committee/institutional review board waived the requirement of written informed consent for participation from the participants or the participants’ legal guardians/next of kin because this is a retrospective study that primarily utilizes available clinical data to retrospectively summarize and analyze.
WC: Conceptualization, Formal analysis, Supervision, Writing – original draft. SW: Methodology, Resources, Writing – original draft. LG: Data curation, Resources, Writing – original draft. YG: Investigation, Software, Writing – original draft. LW: Formal analysis, Software, Writing – original draft. HJ: Investigation, Writing – original draft. YZ: Writing – original draft, Data curation. ChL: Visualization, Writing – original draft. CDL: Writing – review & editing. LX: Conceptualization, Writing – review & editing.
The author(s) declare financial support was received for the research, authorship, and/or publication of this article. This work was supported by Foundation of Zhejiang Provincial Key Laboratory for Accurate Diagnosis and Treatment of Chronic Liver Disease (grant number 2020E10014-002), major participant: LX; Ningbo Medical and Health Brand Discipline (grant number PPXK2018-03), project leader: SW; Natural Science Foundation of Zhejiang Province, China (grant number LY21H030004), project leader: LX; Natural Science Foundation of Ningbo City, (grant number 2022J253), project leader: LX; and Key Technology R&D Project of Ningbo City (grant number 2023Z208), project leader: LX.
We would like to express our gratitude to all those who helped us during the writing of this manuscript.
The authors declare that the research was conducted in the absence of any commercial or financial relationships that could be construed as a potential conflict of interest.
All claims expressed in this article are solely those of the authors and do not necessarily represent those of their affiliated organizations, or those of the publisher, the editors and the reviewers. Any product that may be evaluated in this article, or claim that may be made by its manufacturer, is not guaranteed or endorsed by the publisher.
The Supplementary material for this article can be found online at: https://www.frontiersin.org/articles/10.3389/fmed.2023.1274961/full#supplementary-material
1. Asrani, SK, Devarbhavi, H, Eaton, J, and Kamath, PS. Burden of liver diseases in the world. J Hepatol. (2019) 70:151–71. doi: 10.1016/j.jhep.2018.09.014
2. Arslan, H. Infections in liver transplant recipients. Exp Clin Transplant. (2014) 12:24–7. doi: 10.6002/ect.25liver.l22
3. Laici, C, Gamberini, L, Bardi, T, Siniscalchi, A, Reggiani, MLB, and Faenza, S. Early infections in the intensive care unit after liver transplantation-etiology and risk factors: A single-center experience. Transpl Infect Dis. (2018) 20:e12834. doi: 10.1111/tid.12834
4. Singer, M, Deutschman, CS, Seymour, CW, Shankar-Hari, M, Annane, D, Bauer, M, et al. The Third International Consensus Definitions for Sepsis and Septic Shock (Sepsis-3). JAMA. (2016) 315:801–10. doi: 10.1001/jama.2016.0287
5. Donnelly, JP, Locke, JE, MacLennan, PA, McGwin, G, Mannon, RB, Safford, MM, et al. Inpatient Mortality Among Solid Organ Transplant Recipients Hospitalized for Sepsis and Severe Sepsis. Clin Infect Dis. (2016) 63:186–94. doi: 10.1093/cid/ciw295
6. Wang, Y, Gu, Y, Huang, F, Liu, D, Zhang, Z, Zhou, N, et al. Risk Factors for Sepsis Based on Sepsis-3 Criteria after Orthotopic Liver Transplantation. Mediat Inflamm. (2018) 2018:8703172–8. doi: 10.1155/2018/8703172
7. Lau, WY, and Lai, EC. Liver surgery and transplantation in China: Progress and Challenges. Front Med China. (2007) 1:1–5. doi: 10.1007/s11684-007-0001-y
8. Morioka, D, Egawa, H, Kasahara, M, Ito, T, Haga, H, Takada, Y, et al. Outcomes of adult-to-adult living donor liver transplantation: a single institution's experience with 335 consecutive cases. Ann Surg. (2007) 245:315–25. doi: 10.1097/01.sla.0000236600.24667.a4
9. Rhodes, A, Evans, LE, Alhazzani, W, Levy, MM, Antonelli, M, Ferrer, R, et al. Surviving Sepsis Campaign: International Guidelines for Management of Sepsis and Septic Shock: 2016. Intensive Care Med. (2017) 43:304–77. doi: 10.1007/s00134-017-4683-6
10. Neviere, R, Trinh-Duc, P, Hulo, S, Edme, JL, Dehon, A, Boleslawski, E, et al. Predictive value of exhaled nitric oxide and aerobic capacity for sepsis complications after liver transplantation. Transpl Int. (2016) 29:1307–16. doi: 10.1111/tri.12861
11. Toshima, T, Shirabe, K, Kurihara, T, Itoh, S, Harimoto, N, Ikegami, T, et al. Profile of plasma amino acids values as a predictor of sepsis in patients following living donor liver transplantation: Special reference to sarcopenia and postoperative early nutrition. Hepatol Res. (2015) 45:1170–7. doi: 10.1111/hepr.12484
12. Montano-Loza, AJ. Muscle wasting: a nutritional criterion to prioritize patients for liver transplantation. Curr Opin Clin Nutr Metab Care. (2014) 17:219–25. doi: 10.1097/MCO.0000000000000046
13. Levy, MM, Fink, MP, Marshall, JC, Abraham, E, Angus, D, Cook, D, et al. 2001 SCCM/ESICM/ACCP/ATS/SIS International Sepsis Definitions Conference. Crit Care Med. (2003) 31:1250–6. doi: 10.1097/01.CCM.0000050454.01978.3B
14. Bone, RC, Balk, RA, Cerra, FB, Dellinger, RP, Fein, AM, Knaus, WA, et al. Definitions for sepsis and organ failure and guidelines for the use of innovative therapies in sepsis. The ACCP/SCCM Consensus Conference Committee. American College of Chest Physicians/Society of Critical Care Medicine. Chest. (1992) 101:1644–55. doi: 10.1378/chest.101.6.1644
15. Alcamo, AM, Alessi, LJ, Vehovic, SN, Bansal, N, Bond, GJ, Carcillo, JA, et al. Severe Sepsis in Pediatric Liver Transplant Patients: The Emergence of Multidrug-Resistant Organisms. Pediatr Crit Care Med. (2019) 20:e326–32. doi: 10.1097/PCC.0000000000001983
16. Iida, T, Kaido, T, Yagi, S, Yoshizawa, A, Hata, K, Mizumoto, M, et al. Posttransplant bacteremia in adult living donor liver transplant recipients. Liver Transpl. (2010) 16:1379–85. doi: 10.1002/lt.22165
17. Yokose, T, Takeuchi, M, Obara, H, Shinoda, M, Kawakubo, H, Kitago, M, et al. Diagnostic Utility of Presepsin in Infections After Liver Transplantation: A Preliminary Study. Ann Transplant. (2021) 26:e933774. doi: 10.12659/AOT.933774
18. Nunnally, ME, Ferrer, R, Martin, GS, Martin-Loeches, I, Machado, FR, De Backer, D, et al. Surviving Sepsis Campaign Reasearch Committee. The Surviving Sepsis Campaign: research priorities for the administration, epidemiology, scoring and identification of sepsis. Intensive Care Med Exp. (2021) 9:34. doi: 10.1186/s40635-021-00400-z
19. Shafiekhani, M, Mirjalili, M, and Vazin, A. Prevalence, Risk Factors And Treatment Of The Most Common Gram-Negative Bacterial Infections In Liver Transplant Recipients: A review. Infect Drug Resist. (2019) 12:3485–95. doi: 10.2147/IDR.S226217
20. Aktas, A, Kayaalp, C, Gunes, O, Gokler, C, Uylas, U, Cicek, E, et al. Surgical site infection and risk factors following right lobe living donor liver transplantation in adults: A single-center prospective cohort study. Transpl Infect Dis. (2019) 21:13176. doi: 10.1111/tid.13176
21. Li, C, Wen, TF, Mi, K, Wang, C, Yan, LN, and Li, B. Analysis of infections in the first 3-month after living donor liver transplantation. World J Gastroenterol. (2012) 18:1975–80. doi: 10.3748/wjg.v18.i16.1975
22. Jafarpour, Z, Pouladfar, G, Malek Hosseini, SA, Firoozifar, M, and Jafari, P. Bacterial infections in the early period after liver transplantation in adults: A prospective single-center cohort study. Microbiol Immunol. (2020) 64:407–15. doi: 10.1111/1348-0421.12785
23. Dohna Schwake, C, Guiddir, T, Cuzon, G, Benissa, MR, Dubois, C, Miatello, J, et al. Bacterial infections in children after liver transplantation: A single-center surveillance study of 345 consecutive transplantations. Transpl Infect Dis. (2020) 22:e13208. doi: 10.1111/tid.13208
24. Selimoğlu, MA, Kaya, S, Güngör, Ş, Varol, Fİ, Gözükara-Bağ, HG, and Yılmaz, S. Infection risk after paediatric liver transplantation. Turk J Pediatr. (2020) 62:46–52. doi: 10.24953/turkjped.2020.01.007
25. Ferrer, R, Martin-Loeches, I, Phillips, G, Osborn, TM, Townsend, S, Dellinger, RP, et al. Empiric antibiotic treatment reduces mortality in severe sepsis and septic shock from the first hour: results from a guideline-based performance improvement program. Crit Care Med. (2014) 42:1749–55. doi: 10.1097/CCM.0000000000000330
26. Kumar, A, Roberts, D, Wood, KE, Light, B, Parrillo, JE, Sharma, S, et al. Duration of hypotension before initiation of effective antimicrobial therapy is the critical determinant of survival in human septic shock. Crit Care Med. (2006) 34:1589–96. doi: 10.1097/01.CCM.0000217961.75225.E9
27. Reitz, KM, Kennedy, J, Li, SR, Handzel, R, Tonetti, DA, Neal, MD, et al. Association Between Time to Source Control in Sepsis and 90-Day Mortality. JAMA Surg. (2022) 157:817–26. doi: 10.1001/jamasurg.2022.2761
28. Miller, HC, Liu, VX, and Prescott, HC. Characteristics and Outcomes of Clinic Visits Immediately Preceding Sepsis Hospitalization. Am J Crit Care. (2021) 30:135–9. doi: 10.4037/ajcc2021456
29. Chelala, L, Kovacs, CS, Taege, AJ, and Hanouneh, IA. Common infectious complications of liver transplant. Cleve Clin J Med. (2015) 82:773–84. doi: 10.3949/ccjm.82a.14118
30. Righi, E. Management of bacterial and fungal infections in end stage liver disease and liver transplantation: Current options and future directions. World J Gastroenterol. (2018) 24:4311–29. doi: 10.3748/wjg.v24.i38.4311
31. Fishman, JA. Infection in solid-organ transplant recipients. N Engl J Med. (2007) 357:2601–14. doi: 10.1056/NEJMra064928
32. Rothe, K, Bachfischer, T, Karapetyan, S, Hapfelmeier, A, Wurst, M, Gleich, S, et al. Are enterococcal bloodstream infections an independent risk factor for a poorer 5-year survival or just a marker for severity of illness?-The Munich multicentric enterococci cohort. Microbiol Spectr. (2023) 4:0258523. doi: 10.1128/spectrum.02585-23
33. Wang, XW, Niu, XG, Li, JX, Zhang, SS, and Jiao, XF. SOFA Score Can Effectively Predict the Incidence of Sepsis and 30-Day Mortality in Liver Transplant Patients: A Retrospective Study. Adv Ther. (2019) 36:645–51. doi: 10.1007/s12325-019-0889-z
34. Grammatikopoulos, T, Dhawan, A, Bansal, S, Wade, J, Sherwood, R, Dew, T, et al. Baseline evaluation of serum markers of inflammation and their utility in clinical practice in paediatric liver transplant recipients. Clin Res Hepatol Gastroenterol. (2012) 36:365–70. doi: 10.1016/j.clinre.2012.01.010
35. Figiel, W, Grąt, M, Niewiński, G, Patkowski, W, and Zieniewicz, K. Applicability of common inflammatory markers in diagnosing infections in early period after liver transplantation in intensive care setting. Sci Rep. (2020) 10:3918. doi: 10.1038/s41598-020-60936-0
36. Evans, L, Rhodes, A, Alhazzani, W, Antonelli, M, Coopersmith, CM, French, C, et al. Surviving Sepsis Campaign: International Guidelines for Management of Sepsis and Septic Shock 2021. Crit Care Med. (2021) 49:e1063–143. doi: 10.1097/CCM.0000000000005337
37. Hasegawa, D, Sato, R, Prasitlumkum, N, Nishida, K, Takahashi, K, Yatabe, T, et al. Effect of Ultrashort-Acting β-Blockers on Mortality in Patients With Sepsis With Persistent Tachycardia Despite Initial Resuscitation: A Systematic Review and Meta-analysis of Randomized Controlled Trials. Chest. (2021) 159:2289–300. doi: 10.1016/j.chest.2021.01.009
38. Sullivan, BA, and Fairchild, KD. Vital signs as physiomarkers of neonatal sepsis. Pediatr Res. (2022) 91:273–82. doi: 10.1038/s41390-021-01709-x
39. Xiao, W, Liu, W, Zhang, J, Liu, Y, Hua, T, and Yang, M. The association of diastolic arterial pressure and heart rate with mortality in septic shock: a retrospective cohort study. Eur J Med Res. (2022) 27:285. doi: 10.1186/s40001-022-00930-6
40. Morelli, A, Ertmer, C, Westphal, M, Rehberg, S, Kampmeier, T, Ligges, S, et al. Effect of heart rate control with esmolol on hemodynamic and clinical outcomes in patients with septic shock: a randomized clinical trial. JAMA. (2013) 310:1683–91. doi: 10.1001/jama.2013.278477
41. Hoke, RS, Müller-Werdan, U, Lautenschläger, C, Werdan, K, and Ebelt, H. Heart rate as an independent risk factor in patients with multiple organ dysfunction: a prospective, observational study. Clin Res Cardiol. (2012) 101:139–47. doi: 10.1007/s00392-011-0375-3
42. Morelli, A, D’Egidio, A, and Passariello, M. Tachycardia in septic shock: pathophysiological implications and pharmacological treatment. Ann Update Intens Care Emerg Med. (2015) 2015:115–28. doi: 10.1007/978-3-319-13761-2_9
43. Ren, E, Xiao, H, Li, J, Yu, H, Liu, B, Wang, G, et al. Clinical characteristics and predictors of mortality differ between pulmonary and abdominal sepsis. Shock. (2023) 60:42–50. doi: 10.1097/SHK.0000000000002151
44. Hu, L, Zhu, Y, Chen, M, Li, X, Lu, X, Liang, Y, et al. Development and validation of a disease severity scoring model for pediatric sepsis. Iran J Public Health. (2016) 45:875–84.
45. Song, XC, Nie, S, Xiao, JL, Shen, X, Hong, L, Chen, SY, et al. Risk factors and long-term prognosis for postoperative hypoxemia in patients with acute type A aortic dissection: a retrospective observational study. Medicine (Baltimore). (2022) 101:e32337. doi: 10.1097/MD.0000000000032337
46. Lu, Z, Tao, G, Sun, X, Zhang, Y, Jiang, M, Liu, Y, et al. Association of Blood Glucose Level and Glycemic Variability With Mortality in Sepsis Patients During ICU Hospitalization. Front Public Health. (2022) 10:857368. doi: 10.3389/fpubh.2022.857368
47. Wei, Q, Zhao, J, Wang, H, Liu, C, Hu, C, Zhao, C, et al. Correlation Analysis of Blood Glucose Level with Inflammatory Response and Immune Indicators in Patients with Sepsis. Dis Markers. (2022) 2022:8779061–6. doi: 10.1155/2022/8779061
48. Tsao, CM, Ho, ST, and Wu, CC. Coagulation abnormalities in sepsis. Acta Anaesthesiol Taiwanica. (2015) 53:16–22. doi: 10.1016/j.aat.2014.11.002
49. Hung, KY, Tsai, YH, Lin, CY, Chang, YC, Wang, YH, Lin, MC, et al. Application of Peak Glucose Range and Diabetes Status in Mortality Risk Stratification in Critically Ill Patients with Sepsis. Diagnostics (Basel). (2021) 11:1798. doi: 10.3390/diagnostics11101798
50. Park, CS. Predictive roles of intraoperative blood glucose for post-transplant outcomes in liver transplantation. World J Gastroenterol. (2015) 21:6835–41. doi: 10.3748/wjg.v21.i22.6835
51. Park, C, Hsu, C, Neelakanta, G, Nourmand, H, Braunfeld, M, Wray, C, et al. Severe intraoperative hyperglycemia is independently associated with surgical site infection after liver transplantation. Transplantation. (2009) 87:1031–6. doi: 10.1097/TP.0b013e31819cc3e6
52. Leininger, S. Blood Glucose Management for Reducing Cardiac Surgery Infections. Crit Care Nurs Q. (2018) 41:399–406. doi: 10.1097/CNQ.0000000000000227
53. Zhao, L, Zhao, L, Wang, YY, Yang, F, Chen, Z, Yu, Q, et al. Platelets as a prognostic marker for sepsis: A cohort study from the MIMIC-III database. Medicine (Baltimore). (2020) 99:e23151. doi: 10.1097/MD.0000000000023151
54. Liu, B, Du, H, Zhang, J, Jiang, J, Zhang, X, He, F, et al. Developing a new sepsis screening tool based on lymphocyte count, international normalized ratio and procalcitonin (LIP score). Sci Rep. (2022) 12:20002. doi: 10.1038/s41598-022-16744-9
55. Iba, T, and Levy, JH. Sepsis-induced Coagulopathy and Disseminated Intravascular Coagulation. Anesthesiology. (2020) 132:1238–45. doi: 10.1097/ALN.0000000000003122
56. Lordan, R, Tsoupras, A, and Zabetakis, I. Platelet activation and prothrombotic mediators at the nexus of inflammation and atherosclerosis: Potential role of antiplatelet agents. Blood Rev. (2021) 45:100694. doi: 10.1016/j.blre.2020.100694
57. Mehta, RL, Bouchard, J, Soroko, SB, Ikizler, TA, Paganini, EP, Chertow, GM, et al. Program to Improve Care in Acute Renal Disease (PICARD) Study Group. Sepsis as a cause and consequence of acute kidney injury: Program to Improve Care in Acute Renal Disease. Intensive Care Med. (2011) 37:241–8. doi: 10.1007/s00134-010-2089-9
Keywords: sepsis, liver, transplantation, risk factors, predictive model
Citation: Chen W, Wu S, Gong L, Guo Y, Wei L, Jin H, Zhou Y, Li C, Lu C and Xu L (2023) Exploring the risk factors of early sepsis after liver transplantation: development of a novel predictive model. Front. Med. 10:1274961. doi: 10.3389/fmed.2023.1274961
Received: 21 August 2023; Accepted: 10 November 2023;
Published: 29 November 2023.
Edited by:
Han Wu, Eastern Hepatobiliary Surgery Hospital, ChinaReviewed by:
Stephan Schmid, University Hospital Regensburg, GermanyCopyright © 2023 Chen, Wu, Gong, Guo, Wei, Jin, Zhou, Li, Lu and Xu. This is an open-access article distributed under the terms of the Creative Commons Attribution License (CC BY). The use, distribution or reproduction in other forums is permitted, provided the original author(s) and the copyright owner(s) are credited and that the original publication in this journal is cited, in accordance with accepted academic practice. No use, distribution or reproduction is permitted which does not comply with these terms.
*Correspondence: Lanman Xu, MTM1ODc2NDYzMTVAMTYzLmNvbQ==; Caide Lu, bHVjYWlkZUBuYnUuZWR1LmNu
Disclaimer: All claims expressed in this article are solely those of the authors and do not necessarily represent those of their affiliated organizations, or those of the publisher, the editors and the reviewers. Any product that may be evaluated in this article or claim that may be made by its manufacturer is not guaranteed or endorsed by the publisher.
Research integrity at Frontiers
Learn more about the work of our research integrity team to safeguard the quality of each article we publish.