- 1Department of Orthopedics, First Affiliated Hospital of Dalian Medical University, Dalian, China
- 2Graduate School of Dalian Medical University, Dalian Medical University, Dalian, China
Purpose: Contralateral hip refracture following initial hip fracture surgery is life-threatening in the elderly with high incidence and mortality. This study investigated the associated independent risk factors and established a nomogram prediction model.
Methods: Totally 734 elderly patients with hip fractures who underwent surgical treatment (January 2016–December 2020) were enrolled. Following analyses on demographic variables, clinical characteristics, and laboratory examination, independent risk factors of contralateral hip fractures in the elderly were identified through the least absolute shrinkage and selection operator (LASSO) regression, and univariate and multivariate logistic regression. Patients were randomly allocated into training (n = 513) and validation sets (n = 221). A training set-based nomogram prediction model was established and assessed for predictability, discriminatory ability, and clinical applicability using the receiver operating characteristic (ROC) curves, calibration curves, and decision curve analysis (DCA) in both sets.
Results: Contralateral hip refractures occurred in 7.08% (52/734) patients within 2 years after surgery. Age, hemoglobin (Hb), heart disease, neurovascular disease, Parkinson’s disease (PD), Alzheimer’s disease (AD), chronic obstructive pulmonary disease (COPD), and chronic kidney disease (CKD) were independent risk factors. The nomogram prediction model had a favorable discriminatory ability, as indicated by the areas under the ROC curves (AUC): 0.906 (95% CI, 0.845–0.967) in the training set and 0.956 (95% CI, 0.927–0.985) in the validation set. The calibration curves demonstrated a good consistency between the actual subsequent contralateral hip fracture incidence and the predicted probability. The DCA of the nomogram demonstrated the model’s excellent clinical efficacy.
Conclusion: The nomogram model enabled accurate individualized prediction for the occurrence of subsequent contralateral hip fracture in the elderly within 2 years after surgical treatment, which might help clinicians with precise references for appropriate perioperative management and rehabilitation education following initial hip surgery for their patients.
1 Introduction
Elderly osteoporotic hip fractures categorized as femoral neck and intertrochanteric fractures in accordance with the location were associated with significant mortality and extensive medico-economic burden (1). Prior osteoporotic fractures were considered a robust predictor of subsequent fractures, with a relative risk of approximately 2.7-fold (2). As previously reported, the subsequent contralateral hip fracture has an incidence of 4.4%–17.9% (3–5), occurring primarily within 2 years following surgical treatment of the previous hip fracture (6, 7).
Subsequent hip fractures involved prolonged hospitalization and rehabilitation, severely diminishing the quality of life, accompanied by some other consequences (disability, cardiovascular diseases, etc.) (8, 9). Furthermore, previous literature revealed that patients with subsequent hip fractures presented an additional 55% higher mortality risk than those without subsequent hip fractures (10).
Targeted prevention interventions contribute positively to reducing the occurrence of subsequent hip fractures, enhancing patients’ life quality and alleviating the socioeconomic burden (9, 11). In recent years several clinical investigations focused on exploring the subsequent contralateral hip fractures-associated risk factors including age, gender, cardiovascular disease, visual impairment, neurovascular disease, COPD, and AD (12–14). However, all the above research neglected the effect of laboratory examination indicators on subsequent contralateral hip fractures. Additionally, it remains a lack of research studies that translate the associated risk factors into practical risk scales or predictive models.
Nomogram, a visual way to present multifactorial predictive models, can simplify models to a single numerical estimate of event probabilities, which shows a wide application in orthopedic clinical practice (15–18). Therefore, our study aimed to establish a nomogram model for the contralateral hip fracture postoperatively that can comprehensively analyze its independent risk factors from demographic statistics, clinical characteristics, and laboratory examination indicators, which contribute to the individualized prediction of refracture risks within 2 years after fracture surgery in the elderly. This study may provide a reference for early intervention for improving outcomes.
2 Materials and methods
2.1 Patient
This retrospective study involved elderly hip fracture patients who underwent surgical intervention in our level-1 trauma center between January 2016 and December 2020. The inclusion criteria were: age ≥ 60 years; low energy damage-induced initial hip fracture (diagnosed as femoral neck fracture and intertrochanteric fracture with radiological and clinical symptoms); unilateral fresh closed fracture (injury-to-surgery time < 3 weeks); surgical treatment includes joint replacement, open reduction and closed reduction internal fixation; the clinical data were completed. The exclusion criteria were: hip fractures due to diseases such as joint tuberculosis and tumors; multiple fractures or multiple traumas; patients with previous hip arthroplasty or internal fixation of the hip; ipsilateral hip refracture (periprosthetic fracture) within 2 years; patients with blood system disorders or other disorders affecting blood tests; incomplete follow-up information owing to patient death (The death may be due to factors such as advanced age and severe complications, which may affect the expected outcome), etc. According to the requirements for developing a clinical prediction model, the sample size should be at least 10 times the number of variables (19, 20). In our study there were 38 variables, thus the sample size should be at least 380, and therefore our sample size is adequate.
This study got approval from the Ethics Committee of the First Affiliated Hospital of Dalian Medical University. The ethical approval number is PJ-KS-KY-2023-279. All the experiments were carried out following the Helsinki Declaration.
2.2 Data collection
Data (demographic variables, clinical characteristics, and laboratory examination) of all patients were collected. Demographic variables included age, gender, place of residence, height, weight, and body mass index (BMI). Clinical characteristics were hospitalization time, American Society of Anesthesiologists Physical Status Classification (ASA class), fracture type, surgical approach, hypertension, heart disease (coronary artery disease, heart failure, arrhythmia, heart dysfunction, chronic pulmonary heart disease), diabetes, neurovascular disease (encephalorrhagia, cerebral thromboembolism, cerebral infarction sequela), PD, AD, eye disease (cataract, glaucoma, diabetic eye disease), COPD, CKD, and chronic liver disease (chronic hepatitis, hepatic cirrhosis, hepatic insufficiency). Laboratory examination involved white blood cell (WBC), Hb, platelet (PLT), neutrophil (NEU), lymphocyte (LYM), neutrophil-to-lymphocyte ratio (NLR), platelet-to-lymphocyte ratio (PLR), systemic immune-inflammation index (SII; platelet count × neutrophil count/lymphocyte count), potassium, sodium, calcium, and phosphorus in serum, calcium-phosphorus product (serum calcium × serum phosphorus × 12.4), fasting blood glucose (FBG), albumin (ALB), alkaline phosphatase (ALP), creatinine (Cr), and blood urea nitrogen (BUN).
The blood samples were collected in the morning after admission, following an overnight fasting period. The data of demographic variables, clinical characteristics and comorbidities were acquired from the patient’s hospital records.
2.3 Development and validation of the prediction model
The (glmnet) package of R-studio 4.2.1 (R Foundation for Statistical Computing, Austria) was used for LASSO regression to screen out statistically significant variables for subsequent contralateral hip fracture. The independent risk factors were identified through univariate and multivariate logistic regression analysis on variables with non-zero coefficients. Patients were allocated (training set: validation set = 7:3) at random. The predictive nomogram model was established on the training set, and its performance was assessed by the validation set. The “rms” R package was utilized for nomogram construction and visualization. The ROC curve was applied to assess model discrimination. The calibration of the nomogram was evaluated by the Hosmer-Lemeshow (HL) goodness-of-fit test on the comparison of actual risk with predicted risk. Additionally, the DCA curve was conducted to assess the model’s clinical practicability.
2.4 Statistical analysis
SPSS 25.0 (IBM, Armonk, NY, United States) and R-studio software were applied for data analysis. For continuous variables, the normality was measured using the Shapiro–Wilk test. Normally distributed data were shown as mean ± standard deviation (SD), accompanied by independent Student’s t-test analysis, while those with non-normal distribution were described as the median and interquartile range M (IQR), accompanied by Mann–Whitney U test analysis. Categorical data were described as numbers and percentages (%), followed by the chi-square test or Fisher’s exact test analysis. A two-tailed p value of less than 0.05 indicated a significant difference.
3 Results
3.1 Patient baseline data
Totally 734 patients were enrolled. Among these patients, 52 patients suffered a contralateral hip refracture within 2 years postoperatively. The baseline demographic variables, clinical characteristics, and laboratory examination were summarized in Table 1. Patients were assigned to training and validation sets randomly. As shown in Table 2, no significant difference in all variables between the two sets was found except in PLR (p = 0.047), indicating that the training and validation sets were randomly assigned.
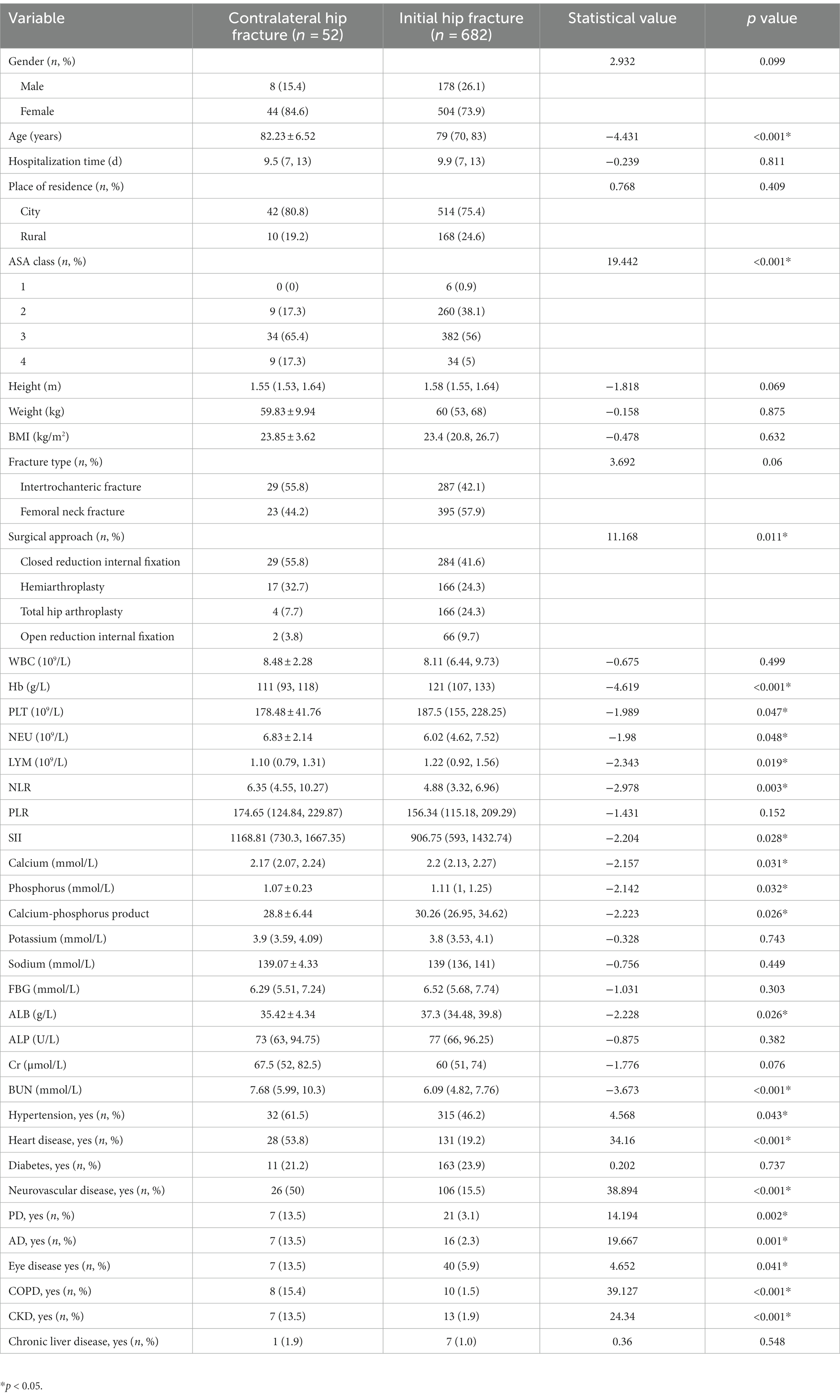
Table 1. Baseline characteristics of patients with or without subsequent contralateral hip fracture after initial hip fracture surgery.
3.2 Screening for predictive factors
The Lasso regression analysis revealed that when lambda. Min (0.009215216) was selected as the best lambda value, and eight variables with nonzero coefficients were screened out to be the critical variables associated with the subsequent contralateral hip fracture, including age, Hb, heart disease, neurovascular disease, PD, AD, COPD, and CKD, as shown in Figure 1. Subsequently, the optimal model variables were further screened using logistic regression analysis, which demonstrated that age (OR, 1.09, 95% CI, 1.032–1.156, p = 0.003), Hb (OR, 0.981, 95% CI, 0.963–0.999, p = 0.049), heart disease (OR, 4.462, 95% CI, 2.211–9.174, p < 0.001), neurovascular disease (OR, 6.049, 95% CI, 2.947–12.625, p < 0.001), PD (OR, 11.618, 95% CI, 3.583–36.299, p < 0.001), AD (OR, 12.14, 95% CI, 3.545–39.225, p < 0.001), COPD (OR, 18.591, 95% CI, 5.492–63.181, p < 0.001), CKD (OR, 8.29, 95% CI, 2.339–28.117, p = 0.001) were statistically significant independent risk factors. The details can be seen in Table 3.
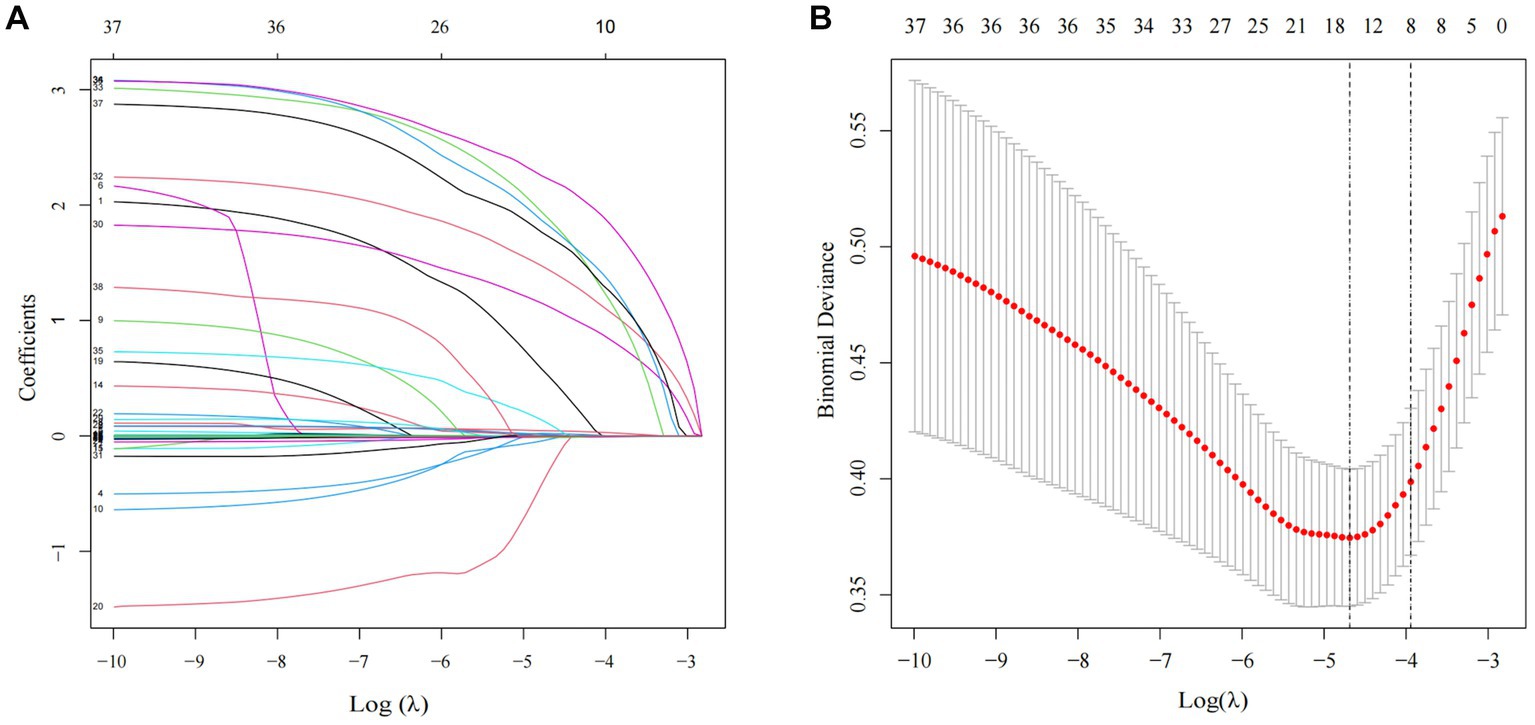
Figure 1. Clinical characteristics of LASSO regression analysis. (A) Predictor coefficient graphic. Each colored solid line in the figure represents a variable with decreasing variable coefficients as Log (λ) increases and part of the variable coefficients become zero. (B) Lasso regression model cross-validation graph. The dotted vertical line on the left and right of the graph represents the value of log (λ. min) and (λ.1se).
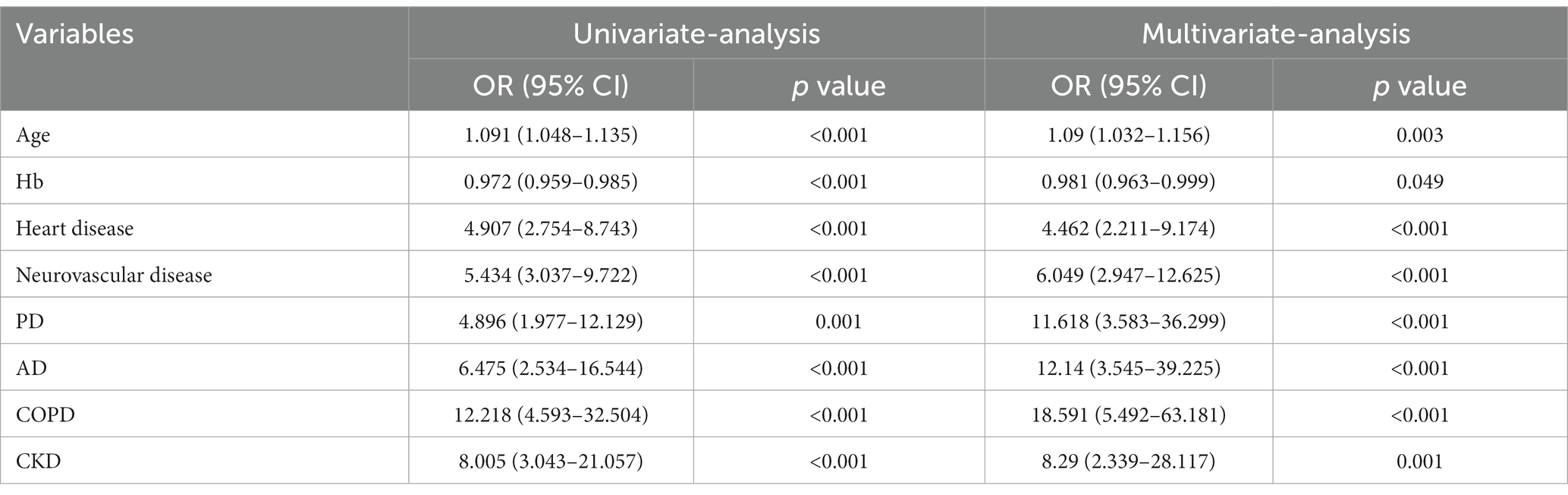
Table 3. Univariate and multivariate logistic regression analyses of variables relating to subsequent contralateral hip fracture.
3.3 Nomogram construction and evaluation
A nomogram of the training set was constructed based on the eight optimal predictors screened by Lasso regression combined with logistic regression (Figure 2). The scores corresponding to the different categories of independent risk factors for each patient were calculated by summing the patient’s total score values, and the corresponding total score scales represented the probability of the patients suffering subsequent contralateral hip fractures. The nomogram model could be applied for incidence calculation. For assessing the nomogram’s discriminatory ability, we plotted the ROC curve for the training and validation sets and calculated the AUC. The model’s cut-off value was identified: 0.064 in the training set (specificity 0.842 and sensitivity 0.903); 0.081 in the validation set (specificity 0.91 and sensitivity 0.81). The AUC was 0.906 (95% CI, 0.845–0.967) and 0.956 (95% CI, 0.927–0.985) in the training and validation sets, separately (Figure 3), indicating that the prediction model has a great discriminatory performance. The Hosmer-Lemeshow test demonstrated no significant difference in the training and validation sets (p = 0.149 and 0.972, respectively). This demonstrated that the predictions of the model are similar to actual subsequent contralateral hip fracture incidence, illustrating the robust calibration ability of the nomogram (Figure 4). According to DCA in the training set, the nomogram brought positive net benefit with a threshold probability of 1–75%. The validation set showed a higher net benefit with a threshold probability of 1%–56% (Figure 5). The results demonstrated excellent clinical efficacy of the model and promising agreement consistency between the training and validation sets.
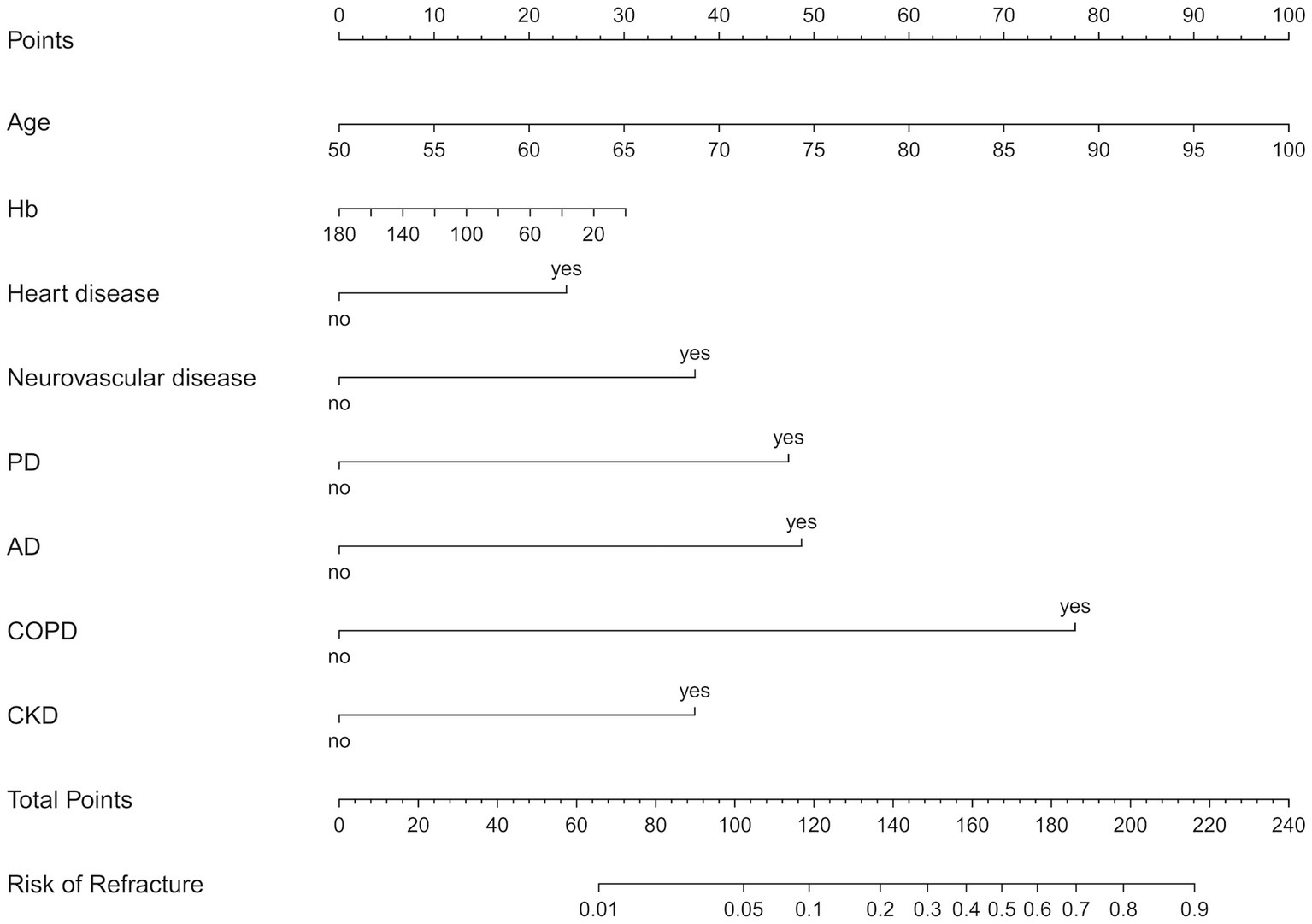
Figure 2. The nomogram predicts the risk of s subsequent contralateral hip fracture in elderly patients within 2 years after hip fracture surgery, based on age, Hb, heart disease, neurovascular disease, PD, AD, COPD, and CKD. Hb, hemoglobin; PD, Parkinson’s disease; AD, Alzheimer’s disease; COPD, chronic obstructive pulmonary disease; CKD, chronic kidney disease.
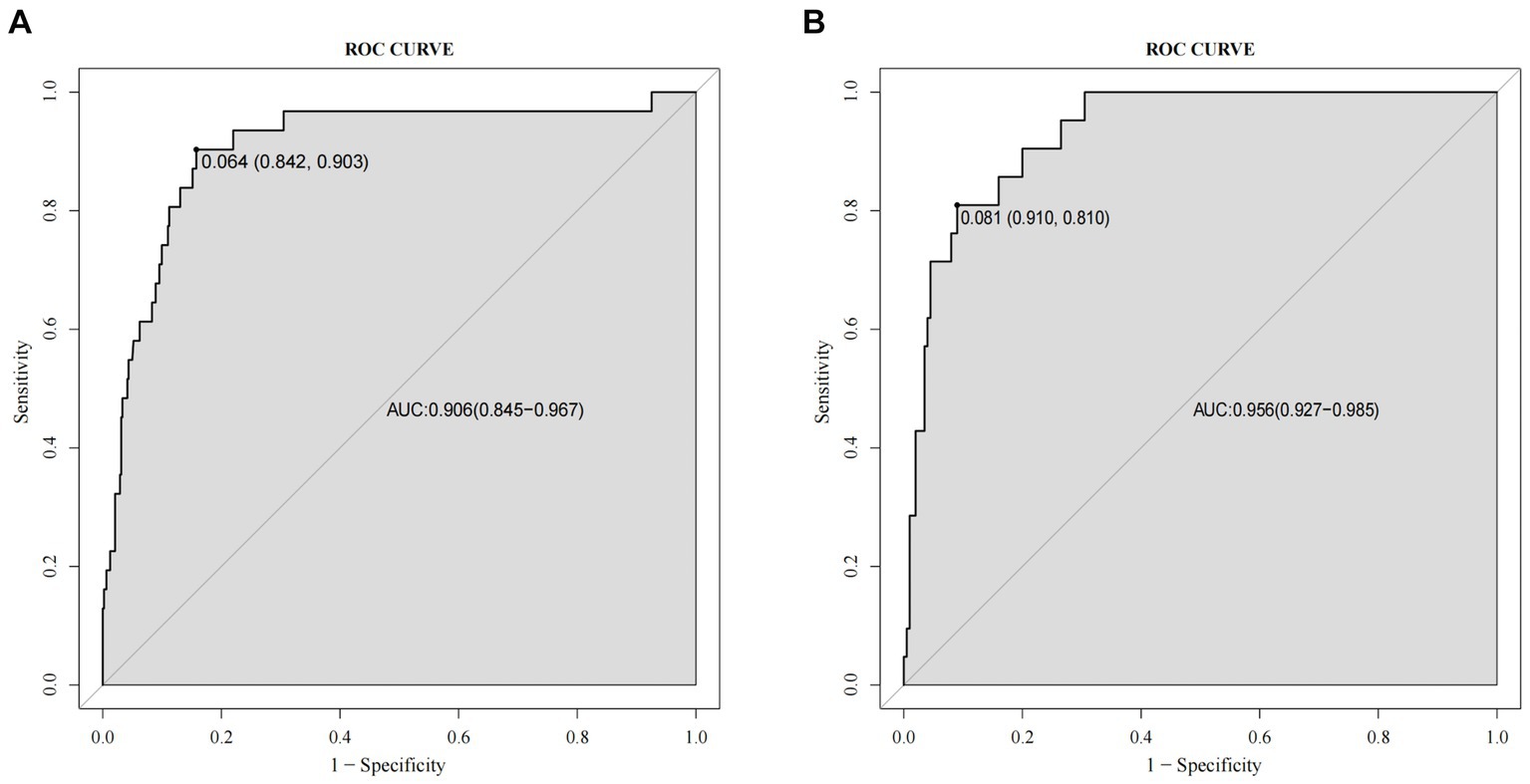
Figure 3. Comparison of the receiver operating characteristic curve (ROC) and the area under the curve (AUC) of the nomogram in the training set (A) and the validation set (B).
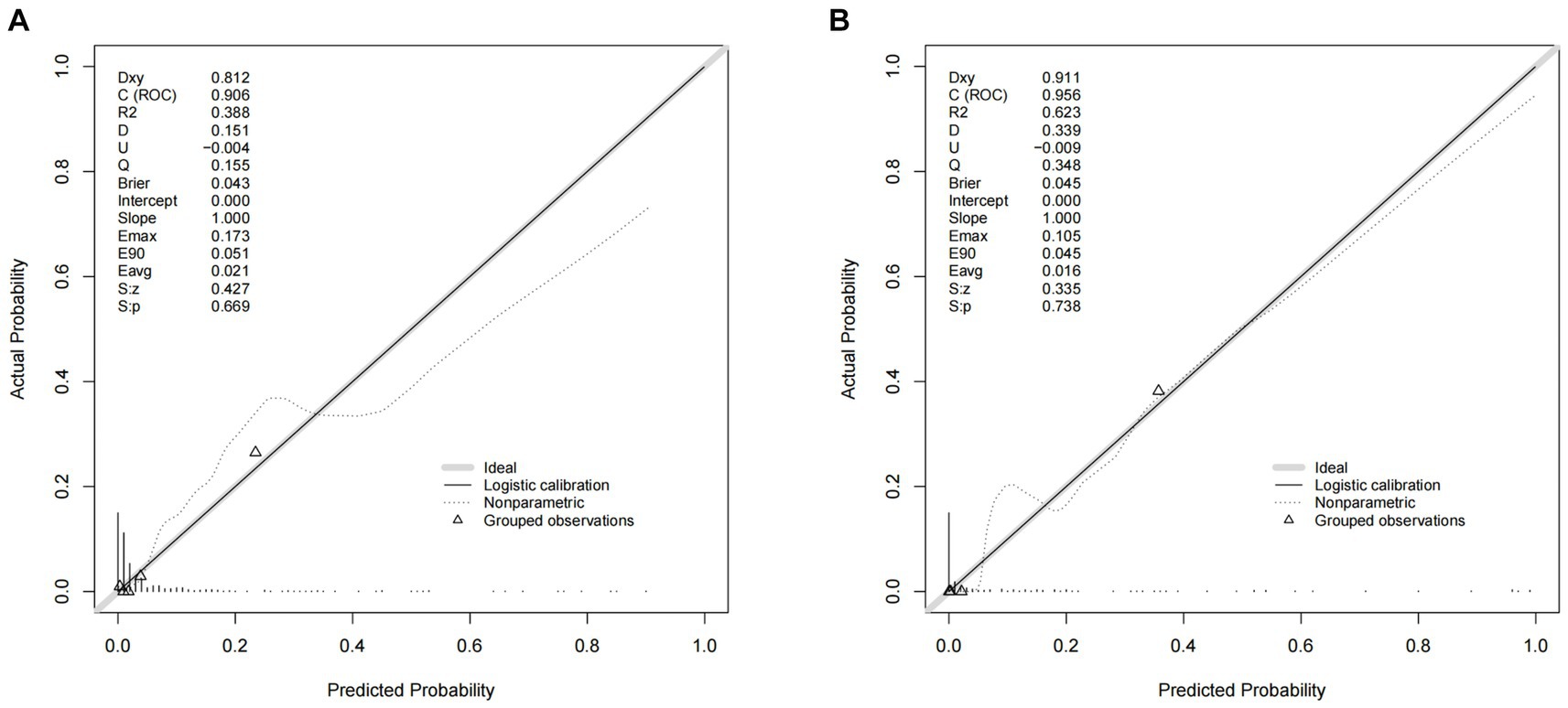
Figure 4. Comparison of the calibration curves of the nomogram in the training set (A) and the validation set (B).
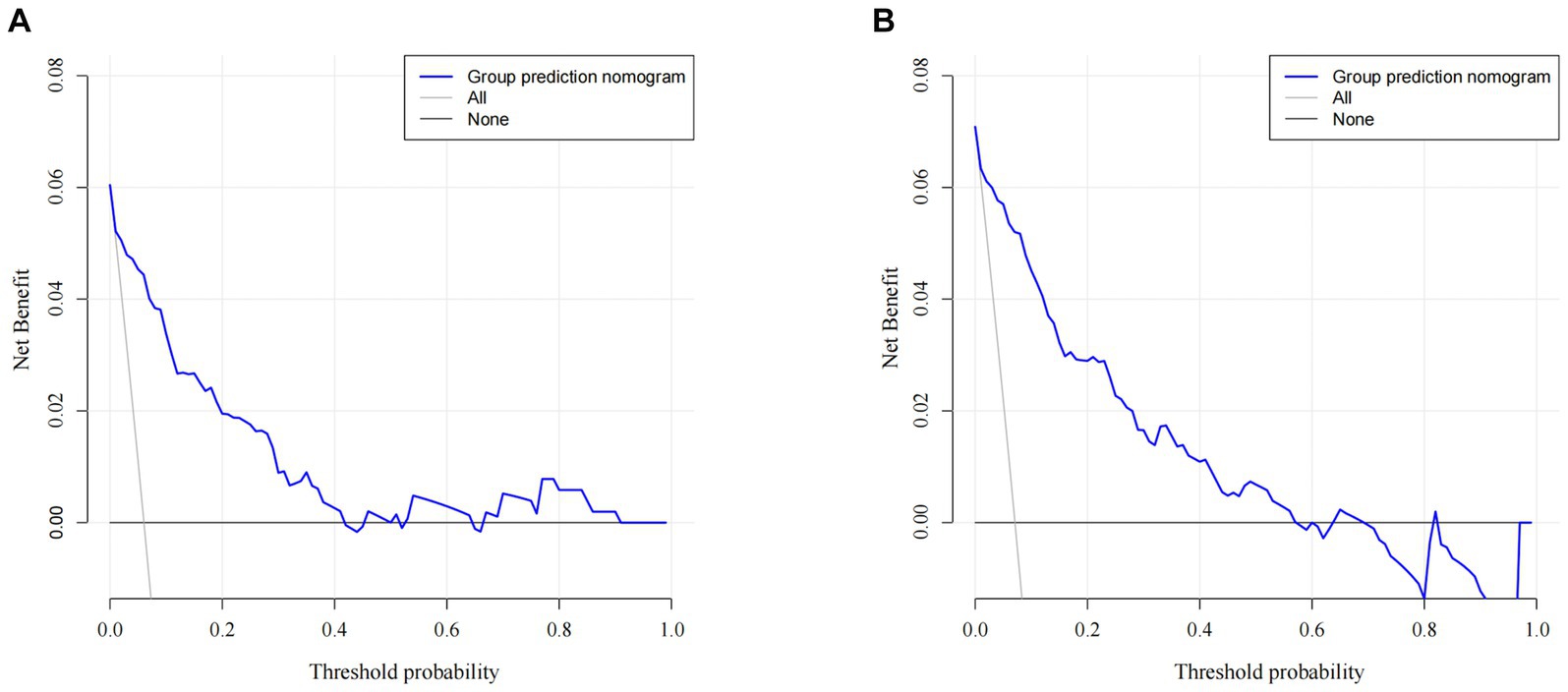
Figure 5. Comparison of the decision curve analyses (DCA) of the nomogram in the training set (A) and the validation set (B).
4 Discussion
This study for the first time established a predictive model for subsequent contralateral hip fractures after initial surgery. In the previous studies, the majority of risk factors were initially screened out by univariate analysis, followed by identifying independent risk factors by multivariate logistic regression analysis. Considering the interactions among these independent risk factors, biases such as multicollinearity and model overfitting were inevitable. Our study utilized the LASSO regression analysis with cross-validation to screen out the potentially associated risk factors, reducing the influence of multiple covariances on the ultimate prediction model and enhancing the model fitting performance. In our study, we found that age, Hb, heart disease, neurovascular disease, PD, AD, COPD, and CKD acted as independent risk factors. Consistently, a previous high-quality prospective cohort study has also provided related evidence (21).
As has been evidenced, old age can independently affect subsequent contralateral hip fractures (12, 14, 22). Our study shows that the odds ratio increases by 1.09 for each additional year after 60 years old. Analyzing the underlying reasons, generally elderly patients may be more likely to suffer from severe osteoporosis and various medical comorbidities. A prospective study revealed that patients with anemia have an enhanced risk of hip fracture (23). As has been demonstrated by Sim et al., preoperative anemia (Hb < 10.0 g / dL) is related to worse physical function and life quality postoperatively, which increased the risk of falls (24). Anaemia was commonly considered to be associated with osteoporosis and sarcopenia in the elderly (25), whereas muscle mass and function loss enhance fragility fracture risk through increasing fall risk (26).
In our study, heart disease can also independently affect contralateral hip refracture, which was in agreement with previous findings (27, 28). A large analysis conducted among 113,600 individuals revealed that atrial fibrillation history enhances subsequent hip fracture risk (29). In a previous systematic review, the odds ratio for heart failure is 1.3 (95% CI, 1.00–1.78) for contralateral hip refracture in elderly patients (30). However, we referred to coronary heart disease, arrhythmias, heart failure, and myocardial infarction collectively as heart disease in our study, which may lead to selection bias. COPD was associated with osteoporosis and hip fractures (12, 31). Analyzing the reasons, patients with COPD received long-term glucocorticoid therapy, which contributed to osteoporosis, and COPD patients had lower physical activity and were consequently deficient in muscle strength and endurance, predisposing to fragility fractures. Patients with glomerular filtration rates (GFR) of 30 to 44 and 15 to 29 mL/min / 1.73m2 have a higher risk of hip fracture than those with GFR > 60 mL/min/1.73m2. Consistent results were also evidenced in our study (32). Vitamin D metabolism is abnormal in CKD patients, as manifested by decreased calcidiol levels due to short daylight exposure and low vitamin D intake, and reduced calcitriol synthesis due to decreased renal function, increasing osteoporosis risk in CKD patients (33).
It has been revealed that the association between neurovascular disease can affect subsequent hip fractures and may be related to increased fall risk due to compromised balance and abnormal proprioception, which was similar to our findings (12, 34, 35). Most hip fractures occur following a fall and that impaired balance and postural instability in patients with PD contribute to the increased risk of falls (36). Nam JS et al. revealed that the risk of hip fracture was about approximately 2-fold higher in people with PD than in the control group, which bolstered our research results (37). AD was a degenerative change in the central nervous system, characterized by progressive loss of memory and cognition. The majority of Alzheimer’s patients had balance impairments and gait deficits, increasing fall risk (38). In addition, some studies demonstrated an association between cognitive decline and hip fracture risk (39).
Unfortunately, there remained a lack of tools to assess the risk of contralateral hip refracture after surgery. The Fracture Risk Assessment Tool (FRAX), a predicting tool based on individual patient models, integrates clinical risk factors and bone mineral density (BMD) at the femoral neck (40). However, the FRAX was primarily designed for predicting an initial fracture risk and the application of its capability in predicting subsequent contralateral hip fractures remains to be further validated (41, 42). QFractureScores was developed to assess an individual’s risk of an osteoporotic fracture within 10 years, based on a prospective cohort study in England, with 25 components including comorbidities (21). However, QFractureScores was also designed for initial fracture assessment and contained excessive variables that might not facilitate clinical application (43).
We first developed a nomogram prediction model for subsequent contralateral fractures in elderly patients within 2 years after hip surgery that included demographic variables, clinical characteristics, and laboratory examination, ultimately identifying the eight optimal predictors. Our nomogram prediction model exhibited good discriminability. Additionally, the nomogram was well-calibrated both in the training and validation sets, indicating good consistency between the actual subsequent contralateral hip fracture incidence and the predicted probability. The DCA confirmed the nomogram model’s excellent clinical utility.
However, there are some limitations. Firstly, all the analyzed data in this retrospective study were limited by the medical records, which can cause methodological bias. Secondly, this study was single-centered without external validation. All patients were included from the same senior trauma center, which meant more severe comorbidities and advanced disease than in other minor medical institutions. Third, the patient size included in our study was relatively small, thus, a large-sample and multi-center clinical study to optimize the clinical prediction model of subsequent contralateral hip fractures after hip fracture surgery will be necessary.
5 Conclusion
Taken together, we developed and validated a nomogram prediction model incorporating age, Hb, heart disease, neurovascular disease, PD, AD, COPD, and CKD to predict the occurrence of subsequent contralateral hip fracture within 2 years after operation in the elderly. We expect that this straightforward and visual predictive model will provide clinicians with precise references for appropriate perioperative management and rehabilitation education following initial hip surgery for their patients.
Data availability statement
The raw data supporting the conclusions of this article will be made available by the authors, without undue reservation.
Ethics statement
The studies involving humans were approved by the Ethics Committee of the First Affiliated Hospital of Dalian Medical University. The studies were conducted in accordance with the local legislation and institutional requirements. The participants provided their written informed consent to participate in this study. Written informed consent was obtained from the individual(s) for the publication of any potentially identifiable images or data included in this article.
Author contributions
JL: Data curation, Formal analysis, Investigation, Methodology, Software, Writing – original draft, Writing – review & editing. JZ: Writing – original draft, Writing – review & editing. ZL: Formal analysis, Supervision, Writing – review & editing. XT: Investigation, Supervision, Writing – review & editing.
Funding
The author(s) declare that no financial support was received for the research, authorship, and/or publication of this article.
Acknowledgments
We would like to thank Xiaohu Chang for his assistance in the data collection and statistics process.
Conflict of interest
The authors declare that the research was conducted in the absence of any commercial or financial relationships that could be construed as a potential conflict of interest.
Publisher’s note
All claims expressed in this article are solely those of the authors and do not necessarily represent those of their affiliated organizations, or those of the publisher, the editors and the reviewers. Any product that may be evaluated in this article, or claim that may be made by its manufacturer, is not guaranteed or endorsed by the publisher.
References
1. Guzon-Illescas, O, Perez Fernandez, E, Crespí Villarias, N, Quirós Donate, FJ, Peña, M, Alonso-Blas, C, et al. Mortality after osteoporotic hip fracture: incidence, trends, and associated factors. J Orthop Surg Res. (2019) 14:203. doi: 10.1186/s13018-019-1226-6
2. Banefelt, J, Åkesson, KE, Spångéus, A, Ljunggren, O, Karlsson, L, Ström, O, et al. Risk of imminent fracture following a previous fracture in a Swedish database study. Osteoporos Int. (2019) 30:601–9. doi: 10.1007/s00198-019-04852-8
3. Omsland, TK, Emaus, N, Tell, GS, Ahmed, LA, Center, JR, Nguyen, ND, et al. Ten-year risk of second hip fracture. A NOREPOS study. Bone. (2013) 52:493–7. doi: 10.1016/j.bone.2012.09.009
4. Trevisan, C, Bedogni, M, Pavan, S, Shehu, E, Piazzani, F, Manzato, E, et al. The impact of second hip fracture on rehospitalization and mortality in older adults. Arch Gerontol Geriatr. (2020) 90:104175. doi: 10.1016/j.archger.2020.104175
5. Shim, YB, Park, JA, Nam, JH, Hong, SH, Kim, JW, Jeong, J, et al. Incidence and risk factors of subsequent osteoporotic fracture: a nationwide cohort study in South Korea. Arch Osteoporos. (2020) 15:180. doi: 10.1007/s11657-020-00852-y
6. Bonafede, M, Shi, N, Barron, R, Li, X, Crittenden, DB, and Chandler, D. Predicting imminent risk for fracture in patients aged 50 or older with osteoporosis using US claims data. Arch Osteoporos. (2016) 11:26. doi: 10.1007/s11657-016-0280-5
7. Roux, C, and Briot, K. Imminent fracture risk. Osteoporos Int. (2017) 28:1765–9. doi: 10.1007/s00198-017-3976-5
8. Chen, CW, Huang, TL, Su, LT, Kuo, YC, Wu, SC, Li, CY, et al. Incidence of subsequent hip fractures is significantly increased within the first month after distal radius fracture in patients older than 60 years. J Trauma Acute Care Surg. (2013) 74:317–21. doi: 10.1097/ta.0b013e31824bb325
9. Veronese, N, and Maggi, S. Epidemiology and social costs of hip fracture. Injury. (2018) 49:1458–60. doi: 10.1016/j.injury.2018.04.015
10. Sobolev, B, Sheehan, KJ, Kuramoto, L, and Guy, P. Excess mortality associated with second hip fracture. Osteoporos Int. (2015) 26:1903–10. doi: 10.1007/s00198-015-3104-3
11. Leal, J, Gray, AM, Prieto-Alhambra, D, Arden, NK, Cooper, C, Javaid, MK, et al. Impact of hip fracture on hospital care costs: a population-based study. Osteoporos Int. (2016) 27:549–58. doi: 10.1007/s00198-015-3277-9
12. Ganhão, S, Guerra, MG, Lucas, R, Terroso, G, Aguiar, F, Costa, L, et al. Predictors of mortality and Refracture in patients older than 65 years with a proximal femur fracture. J Clin Rheumatol. (2022) 28:e49–55. doi: 10.1097/rhu.0000000000001581
13. Yu, S, Li, C, Zhong, Y, Zang, J, Zhou, Z, Wang, S, et al. Risk factors for contralateral hip refractures in patients aged over 80 years with intertrochanteric femoral fractures. Front Surg. (2022) 9:924585. doi: 10.3389/fsurg.2022.924585
14. Sriruanthong, K, Philawuth, N, Saloa, S, Daraphongsataporn, N, and Sucharitpongpan, W. Risk factors of refracture after a fragility fracture in elderly. Arch Osteoporos. (2022) 17:98. doi: 10.1007/s11657-022-01143-4
15. Iasonos, A, Schrag, D, Raj, GV, and Panageas, KS. How to build and interpret a nomogram for cancer prognosis. J Clin Oncol. (2008) 26:1364–70. doi: 10.1200/jco.2007.12.9791
16. Lin, M, Wen, X, Huang, Z, Huang, W, Zhang, H, Huang, X, et al. A nomogram for predicting residual low back pain after percutaneous kyphoplasty in osteoporotic vertebral compression fractures. Osteoporos Int. (2023) 34:749–62. doi: 10.1007/s00198-023-06681-2
17. Reitman, CA. CORR insights®: can a nomogram predict survival after treatment for an ankylosing spondylitis cervical fracture in a patient with neurologic impairment? A national, multicenter study. Clin Orthop Relat Res. (2023) 481:1412–4. doi: 10.1097/corr.0000000000002570
18. Smolle, MA, Petermeier, V, Ornig, M, Leitner, L, Eibinger, N, Puchwein, P, et al. A nomogram predicting risk for acute compartment syndrome following tibial plateau fractures. Single centre retrospective study. Injury. (2022) 53:669–75. doi: 10.1016/j.injury.2021.10.027
19. Riley, RD, Ensor, J, Snell, KIE, Harrell, FE Jr, Martin, GP, Reitsma, JB, et al. Calculating the sample size required for developing a clinical prediction model. BMJ. (2020) 368:m441. doi: 10.1136/bmj.m441
20. Cheng, X, Lei, X, Wu, H, Luo, H, Fu, X, Gao, Y, et al. Development and validation of a predictive nomogram for preoperative deep vein thrombosis (DVT) in isolated calcaneal fracture. Sci Rep. (2022) 12:5923. doi: 10.1038/s41598-022-10002-8
21. Hippisley-Cox, J, and Coupland, C. Predicting risk of osteoporotic fracture in men and women in England and Wales: prospective derivation and validation of QFractureScores. BMJ. (2009) 339:b4229. doi: 10.1136/bmj.b4229
22. Zidrou, C, Vasiliadis, AV, Rizou, S, and Beletsiotis, A. Second hip fracture in older adults: incidence and risk factors. Eur J Orthop Surg Traumatol. (2022) 33:1599–606. doi: 10.1007/s00590-022-03309-9
23. Valderrábano, RJ, Buzkova, P, Chang, PY, Zakai, NA, Fink, HA, Robbins, JA, et al. Associations of hemoglobin and change in hemoglobin with risk of incident hip fracture in older men and women: the cardiovascular health study. Osteoporos Int. (2021) 32:1669–77. doi: 10.1007/s00198-021-05873-y
24. Sim, YE, Sim, SD, Seng, C, Howe, TS, Koh, SB, and Abdullah, HR. Preoperative anemia, functional outcomes, and quality of life after hip fracture surgery. J Am Geriatr Soc. (2018) 66:1524–31. doi: 10.1111/jgs.15428
25. Bani Hassan, E, Vogrin, S, Hernandez Viña, I, Boersma, D, Suriyaarachchi, P, and Duque, G. Hemoglobin levels are low in Sarcopenic and Osteosarcopenic older persons. Calcif Tissue Int. (2020) 107:135–42. doi: 10.1007/s00223-020-00706-2
26. Curtis, E, Litwic, A, Cooper, C, and Dennison, E. Determinants of muscle and bone aging. J Cell Physiol. (2015) 230:2618–25. doi: 10.1002/jcp.25001
27. Van Haecke, A, Viste, A, Desmarchelier, R, Roy, P, Mercier, M, and Fessy, MH. Incidence and risk factors for bilateral proximal femoral fractures. Orthop Traumatol Surg Res. (2022) 108:102887. doi: 10.1016/j.otsr.2021.102887
28. Fujita, T, Takegami, Y, Ando, K, Sakai, Y, Nakashima, H, Takatsu, S, et al. Risk factors for second hip fracture in elderly patients: an age, sex, and fracture type matched case-control study. Eur J Orthop Surg Traumatol. (2022) 32:437–42. doi: 10.1007/s00590-021-02996-0
29. Wong, CX, Gan, SW, Lee, SW, Gallagher, C, Kinnear, NJ, Lau, DH, et al. Atrial fibrillation and risk of hip fracture: a population-based analysis of 113,600 individuals. Int J Cardiol. (2017) 243:229–32. doi: 10.1016/j.ijcard.2017.05.012
30. Liu, S, Zhu, Y, Chen, W, Sun, T, Cheng, J, and Zhang, Y. Risk factors for the second contralateral hip fracture in elderly patients: a systematic review and meta-analysis. Clin Rehabil. (2015) 29:285–94. doi: 10.1177/0269215514542358
31. Akyea, RK, McKeever, TM, Gibson, J, Scullion, JE, and Bolton, CE. Predicting fracture risk in patients with chronic obstructive pulmonary disease: a UK-based population-based cohort study. BMJ Open. (2019) 9:e024951. doi: 10.1136/bmjopen-2018-024951
32. Kim, SH, Yi, SW, Yi, JJ, Kim, YM, and Won, YJ. Chronic kidney disease increases the risk of hip fracture: a prospective cohort study in Korean adults. J Bone Min Res. (2020) 35:1313–21. doi: 10.1002/jbmr.3997
33. Moe, SM, Drüeke, T, Lameire, N, and Eknoyan, G. Chronic kidney disease-mineral-bone disorder: a new paradigm. Adv Chronic Kidney Dis. (2007) 14:3–12. doi: 10.1053/j.ackd.2006.10.005
34. Hjelholt, TJ, Johnsen, SP, Brynningsen, PK, Andersen, G, and Pedersen, AB. Impact of stroke history on the risk of recurrent hip fracture or major osteoporotic fractures among patients with incident hip fracture: a Nationwide Cohort Study. J Bone Min Res. (2023) 38:278–87. doi: 10.1002/jbmr.4760
35. Harvey, L, Toson, B, Mitchell, R, Brodaty, H, Draper, B, and Close, J. Incidence, timing and impact of comorbidity on second hip fracture: a population-based study. ANZ J Surg. (2018) 88:577–81. doi: 10.1111/ans.14507
36. Torsney, KM, Noyce, AJ, Doherty, KM, Bestwick, JP, Dobson, R, and Lees, AJ. Bone health in Parkinson's disease: a systematic review and meta-analysis. J Neurol Neurosurg Psychiatry. (2014) 85:1159–66. doi: 10.1136/jnnp-2013-307307
37. Nam, JS, Kim, YW, Shin, J, Chang, JS, and Yoon, SY. Hip fracture in patients with Parkinson's disease and related mortality: a population-based study in Korea. Gerontology. (2021) 67:544–53. doi: 10.1159/000513730
38. Gras, LZ, Kanaan, SF, McDowd, JM, Colgrove, YM, Burns, J, and Pohl, PS. Balance and gait of adults with very mild Alzheimer disease. J Geriatr Phys Ther. (2015) 38:1–7. doi: 10.1519/jpt.0000000000000020
39. Tolppanen, AM, Taipale, H, Tanskanen, A, Tiihonen, J, and Hartikainen, S. Comparison of predictors of hip fracture and mortality after hip fracture in community-dwellers with and without Alzheimer's disease – exposure-matched cohort study. BMC Geriatr. (2016) 16:204. doi: 10.1186/s12877-016-0383-2
40. Beaudoin, C, Moore, L, Gagné, M, Bessette, L, Ste-Marie, LG, Brown, JP, et al. Performance of predictive tools to identify individuals at risk of non-traumatic fracture: a systematic review, meta-analysis, and meta-regression. Osteoporos Int. (2019) 30:721–40. doi: 10.1007/s00198-019-04919-6
41. Kanis, JA, Johnell, O, Oden, A, Johansson, H, and McCloskey, E. FRAX and the assessment of fracture probability in men and women from the UK. Osteoporos Int. (2008) 19:385–97. doi: 10.1007/s00198-007-0543-5
42. Lott, A, Pflug, EM, Parola, R, Egol, KA, and Konda, SR. Predicting the subsequent contralateral hip fracture: is FRAX the answer? J Orthop Trauma. (2022) 36:599–603. doi: 10.1097/bot.0000000000002441
Keywords: contralateral hip fracture, prediction, nomogram, elderly patients, hip fracture surgery
Citation: Liang J, Zhang J, Lou Z and Tang X (2023) Development and validation of a predictive nomogram for subsequent contralateral hip fracture in elderly patients within 2 years after hip fracture surgery. Front. Med. 10:1263930. doi: 10.3389/fmed.2023.1263930
Edited by:
Xinbao Wu, Beijing Jishuitan Hospital, ChinaReviewed by:
Pengbin Yin, People's Liberation Army General Hospital, ChinaBo Hu, Zhejiang Chinese Medical University, China
Copyright © 2023 Liang, Zhang, Lou and Tang. This is an open-access article distributed under the terms of the Creative Commons Attribution License (CC BY). The use, distribution or reproduction in other forums is permitted, provided the original author(s) and the copyright owner(s) are credited and that the original publication in this journal is cited, in accordance with accepted academic practice. No use, distribution or reproduction is permitted which does not comply with these terms.
*Correspondence: Xin Tang, c3VyZ2VvbnRhbmd4aW5AMTYzLmNvbQ==
†These authors have contributed equally to this work and share first authorship