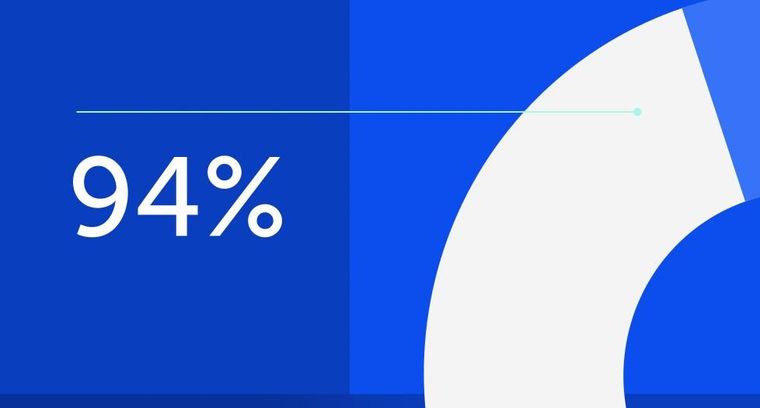
94% of researchers rate our articles as excellent or good
Learn more about the work of our research integrity team to safeguard the quality of each article we publish.
Find out more
ORIGINAL RESEARCH article
Front. Med., 13 July 2023
Sec. Rheumatology
Volume 10 - 2023 | https://doi.org/10.3389/fmed.2023.1192153
Introduction: Rheumatoid arthritis (RA) is a chronic immune disease characterized by synovial inflammation and bone destruction, with a largely unclear etiology. Evidence has indicated that ferroptosis may play an increasingly important role in the onset and development of RA. However, ferroptosis-related genes are still largely unexplored in RA. Therefore, this work focused on identifying and validating the potential ferroptosis-related genes involved in RA through bioinformatics analysis.
Methods: We screened differentially expressed ferroptosis-related genes (DEFGs) between RA patients and healthy individuals based on GSE55235 dataset. Subsequently, correlation analysis, protein-protein interaction (PPI) network analysis, GO, and KEGG enrichment analyses were performed using these DEFGs. Finally, our results were validated by GSE12021 dataset.
Results: We discovered 34 potential DEFGs in RA based on bioinformatics analysis. According to functional enrichment analysis, these genes were mainly enriched in HIF-1 signaling pathway, FoxO signaling pathway, and Ferroptosis pathway. Four genes (GABARPL1, DUSP1, JUN, and MAPK8) were validated to be downregulated by GSE12021 dataset and were diagnostic biomarkers and therapeutic targets for RA via the regulation of ferroptosis.
Discussion: Our results help shed more light on the pathogenesis of RA. Ferroptosis-related genes in RA are valuable diagnostic biomarkers and they will be exploited clinically as therapeutic targets in the future.
Rheumatoid arthritis (RA) is a chronic autoimmune inflammatory disease characterized by inflammation and affects about 1% of the world population (1). As a result, RA can lead to joint deformity and disability, severely reducing the quality of life for RA patients. Although the pathogenesis of RA has not been entirely clarified, genetic and environmental factors have been shown to be involved (2). Several studies also demonstrated that the pathogenesis of RA was associated with certain biological functions such as osteoclast differentiation, inflammatory cell infiltration, angiogenesis, and ferroptosis (3, 4). Among these biological functions, ferroptosis is an interesting topic because of its potential links to cell death, suggesting a possible crucial role in the occurrence of RA.
Ferroptosis is a newly identified cell death form, which is characterized by iron-dependent accumulation and lipid peroxidation (5, 6). Evidence have reported that ferroptosis can trigger undesirable inflammatory responses (7, 8), and its inhibitor has an anti-inflammatory effect on different inflammatory diseases (9, 10). Various evidence has indicated that several pathways or compounds are related to ferroptosis in the pathogenesis of RA (4, 11, 12). Ferroptosis has been shown to be associated with RA, osteoarthritis, gout arthritis, and ankylosing spondylitis (13). However, the exact mechanism between ferroptosis and RA remains largely unclear, and it is necessary and urgent to elucidate the role in RA pathogenesis. The identification of potential ferroptosis-related genes in RA may help to derive potential biomarkers for treatment. Woetzel et al. (14) leveraged the GSE55235 dataset to obtain DEGs between RA patients and healthy individuals, their study sought to distinguish RA patients and healthy individuals by applying rule-based classifiers. However, they neglect the effect of ferroptosis on RA. Therefore, it is essential to analyze the relationship between ferroptosis and synovial inflammation, which may provide potential therapeutic targets for RA.
In this paper, we attempted to investigate the pathogenesis of RA from the ferroptosis perspective. To functionally identify and characterize the DEFGs in RA, we screened DEFGs based on the GSE55235 dataset and further validated our results in the GSE12021 dataset. Finally, this work may provide potential biomarkers for RA therapy and benefit the understanding of RA pathogenesis.
The publicly available database FerrDb (15) (http://www.zhounan.org/ferrdb/) contains 259 ferroptosis-related genes. These downloaded data were used for subsequent analyses. Furthermore, we also collected gene expression profiles for 10 RA patients and 10 healthy individuals from the GSE55235 dataset to screen differentially expressed ferroptosis-related genes (DEFGs). The microarray data were quantified using the GPL96 platform from Affymetrix Human Genome U133A Array (14).
First, we used the “limma” package to normalize the gene expression profile. Second, the probes were annotated using “annotation” package. We applied principal component analysis (PCA) to evaluate the repeatability of the GSE55235 dataset. The bioconductor “limma” package was subsequently utilized to screen DEFGs between RA patients and healthy individuals. |logFC| > 1 and adjusted p-value < 0.01 were set as the cutoff criteria of DEFGs. Lastly, we leveraged the “heatmap” and “ggplot2” packages to present the result of DEFGs.
Gene ontology (GO) is the widely used tool for gene function annotations. The GO terms are classified into three types: molecular function (MF), biological process (BP), and cellular component (CC). Pathway enrichment analysis was conducted using KEGG pathway database. The “clusterprofile” package (16) was utilized to perform the functional enrichment analyses of the DEFGs, with the cutoff criteria set at p < 0.01.
The STRING database was used to schematically represent functional relationship network of DEFGs, named PPI network. We considered STRING interactions that were of medium confidence (combined STRING score > 0.4), which were experimentally derived and curated interactions. The PPI networks of DEFGs were visualized using the STRING database. Correlation analysis was performed using Pearson correlation coefficient with the “corrplot” package.
The GSE12021 dataset was downloaded from the Gene Expression Omnibus (GEO) database, which includes 12 RA patients and 9 healthy individuals. The differentially expressed genes were also identified using the “limma” package according to the adjusted p-value < 0.01 and |logFC| > 1. Finally, we obtained the overlapping genes of 259 ferroptosis-associated genes, GSE55235 DEGs, and GSE12021 DEGs.
R software (version 3.6.2) was used for statistical analysis. We applied the “limma” package to screen differentially expressed genes between RA patients and normal samples. The threshold was set at p < 0.01.
The result of PCA showed good biological replicate concordance of each biological sample in the GSE55235 dataset (Figure 1A). We integrated 259 ferroptosis-related genes and DEGs between RA patients and healthy individuals from the GSE55235 database to screen DEFGs. We obtained a total of 34 DEFGs, of which 8 were upregulated and 26 were downregulated genes (Figures 1B,C).
Figure 1. Differential expression analysis between RA patients and health samples. (A) PCA analysis of the GSE55235 dataset. (B) Volcano plot of the 259 ferroptosis-related genes. The significantly upregulated and downregulated genes are represented by red and blue dots, respectively. (C) Heatmap of the 34 DEFGs between the patients with rheumatoid arthritis and healthy individuals.
Furthermore, we generated a violin diagram to illustrate the expression patterns of the 34 DEFGs (Figure 2). There are 8 upregulated genes (Figure 2A) including SLC2A6, ALOX5, AURKA, CYBB, NCF2, CAPG, RRM2, BLOC1S5-TCNDC5, and 26 downregulated genes (Figure 2B) included ANGPTL7, ATF3, BNIP3, CBS, DDIT4, DUSP1, GDF15, NNMT, PTGS2, SLC3A2, TF, VEGFA, CDO1, DUOX2, EGFR, GABARPL1, MAPK8, ZEB1, AKR1C2, CDKN1A, JUN, MT1G, PLIN2, SCD, ZFP36.
Figure 2. The violin diagram of 34 DEFGs between the patients with rheumatoid arthritis and healthy individuals. (A) 8 upregulated genes (B) 26 downregulated genes. **: p-value < 0.01, ***: p-value < 0.001, ****: p-value < 0.0001.
To elucidate the relationship of these 34 DEFGs, we performed PPI network analysis. The results demonstrated that 34 DEFGs were closely interconnected (Figure 3). We find several hub genes (VEGFA, JUN, CDKN1A, DUSP1, MAPK8, and EGFR) that have high degree. In addition, to examine if there was a correlation between the expression levels of these DEFGs, we conducted correlation analysis. The results showed that the correlation relationship of most genes is positive, only a few have negative correlation relationship (Figure 4).
To elucidate the biological functions of the 34 DEFGs, GO and KEGG enrichment analyses were performed using the “clusterprofiler” package to identify significantly enriched terms. The most significantly enriched GO terms of BP were cellular response to oxidative stress, response to extracellular stimulus, and reactive oxygen species metabolic process (Figure 5A). In the CC category, they were involved in nicotinamide adenine dinucleotide phosphate (NADPH) oxidase complex, oxidoreductase complex, and nuclear envelope (Figure 5B). In the MF category, they were involved in oxidoreductase activity, superoxide generating NADPH oxidase activity, and oxidoreductase activity acting on nicotinamide adenine dinucleotide phosphate (NADPH; Figure 5C). KEGG enrichment was mainly distributed in HIF-1 signaling pathway, FoxO signaling pathway, and Ferroptosis pathway (Figure 5D).
Figure 5. Functional enrichment analysis of 34 DEFGs. (A) BP, (B) CC, (C) MF, (D) KEGG. BP: biological process; CC: cellular component; MF: molecular function.
To validate the reliability of 34 DEFGs expression levels, we used another dataset (GSE12021) (17) to verify expression levels. Compared with the results of the GSE55235 dataset, the expression levels of GABARPL1, DUSP1, JUN, and MAPK8 were decreased in the RA samples (−1.75-, −4.47-, −3.44-, and −1.28-fold, respectively) compared to normal samples (Figure 6). It indicated that the expression levels of four genes were consistent with the results of GSE55235.
Figure 6. Validation of gene expression in the GSE12021 dataset. The violin diagram showed 4 DEFGs between RA patients and healthy individuals. The Wilcox test was used to compare RA patients with normal samples. ***: p-value < 0.001, ****: p-value < 0.0001.
DGIbd (18) and CMAP database (19) were utilized to find potential candidate agents for GABARPL1, DUSP1, JUN and MAPK8. In the DGIbd or CMap database, Albuterol, Hydroxyurea, or Vasopressin was found to be the targeted medicine of DUSP1, Irisolidone, Holacanthone, Sergeolide or Irbesartan was found to be the targeted medicine of JUN, and Cardamomin, CC-401, Tanzisertib or BI-78D3 was found to be the targeted medicine of MAPK8 (Table 1). Ehrlich et al. (17) found that low-dose hydroxyurea held potential efficacy in the treatment of rheumatoid arthritis (RA). Petersson et al. (30) reported potential involvement of arginine-vasopressin and parathyroid hormone-related protein in the pathophysiological mechanisms of RA. Chang et al. (31) discovered that the angiotensin receptor blockers (ARBs) losartan and irbesartan effectively reduced superoxide levels, downregulated the expression and activity of NAD(P)H oxidases, and ameliorated endothelial dysfunction in antigen-induced arthritis (AIA). Zhou et al. (32) conducted a study in which they found that the addition of irbesartan to chemotherapy demonstrated potential for enhancing therapeutic effectiveness in patients with pancreatic ductal adenocarcinoma (PDAC) who exhibited elevated c-Jun expression levels. Irbesartan demonstrated significant efficacy in overcoming chemotherapy resistance by effectively suppressing the Hippo/YAP1/c-Jun/stemness/iron metabolism axis.
Although great progress has been made in exploring new treatments for RA. To date, elucidating the pathogenesis of RA remains a major challenge, thus it is urgent to explore the molecular mechanisms of these diseases. Moreover, several studies have illustrated that ferroptosis may be involved in various tumor types (33, 34). However, there have been few studies focused specifically on ferroptosis-associated genes in RA.
In our study, there were 34 potential ferroptosis-related genes that were identified between RA patients and healthy individuals by using bioinformatic analyses. Furthermore, GO and KEGG enrichment analyses were conducted to investigate the biological functions of the 34 DEFGs. The oxidative stress, oxidoreductase complex, and NADPH oxidase were significantly enriched by these DEFGs, indicating that these DEFGs were associated with oxidation, which was consistent with previous reports (35).
Based on KEGG pathway enrichment, we found that these genes were significantly enriched in FoxO signaling pathway, HIF-1 signaling pathway, and Ferroptosis pathway. Lee et al. (36) identified FoxO3 as a biomarker of RA severity, and its haplotype was associated with erosion scores of RA. Kok et al. (37) reported that SIRT-1/FoxO3a signaling played a crucial role in the occurrence and development of RA. Besides, FoxO signaling was also vital in OA. Abnormal expression of FoxO had been reported to be closely associated with osteoarthritis (38). HIF-1α is a very important transcription factor that regulates developmental and cellular responses to hypoxia. A deficiency of HIF-1α has been reported to exacerbate MMP13 expression levels to lead to degrading cartilage tissue (39). Our results represented these RA-related pathways, which might enhance our understanding of RA pathogenesis.
Furthermore, we identified 4 DEFGs (GABARPL1, DUSP1, JUN, and MAPK8) and observed that the expression levels of these genes in the GSE55235 were consistent with the GSE12021 dataset. Several genes had been shown to be closely related to RA. For example, DUSP1 is a phosphatase with dual specificity for tyrosine and threonine, which involves cellular processes by regulating MAPK1/ERK2. Vattakuzhi et al. (40) found that DUSP1 can regulate MAPK signaling and its low expression may be related to osteolytic lesions in arthritis. JUN is the putative transforming gene of avian sarcoma virus 17. It regulated gene expression by interacting with specific target DNA sequences (41). Huber et al. (42) revealed that Jun/Fos proto-oncogene was significantly decreased at the mRNA level in RA. MAPK8, also known as Jun nuclear kinase (JNK), was a member of the MAP kinase family, which was involved in various cellular processes (proliferation, differentiation, transcription regulation, and development) (43). Ding et al. (44) reported that abnormal activation of MAPKs in synovial tissues of RA patients promoted pannus formation. Thus, MAPK is considered a promising potential target in the treatment of RA. GABARAPL1, a constituent of the GABARAP family, demonstrates a remarkable degree of evolutionary conservation. Its coding gene was orignally identified as an early estrogen-induced gene (45). Gao et al. (46) uncovered that GABARAPL1 was a potential positive regulator of ferroptosis by RNAi screening. Furthermore, the expression of GABARAPL1 was notably diminished in various types of cancers (47–49). A comprehensive analysis of breast cancer biopsies in a cohort study revealed a significant association between lower GABARAPL1 expression and an increased risk of recurrence (48). Overexpression of GABARAPL1 exerted inhibitory effects on cell proliferation, colony formation, and invasion in breast cancer cells in-vitro. Xie et al. (50) demonstrated that DUSP1 inhibited ferroptosis by limiting lipid peroxidation while not affecting iron accumulation. Among the various mechanisms investigated in ferroptosis research, lipid peroxidation, which leads to the generation of lipid oxidation products, has garnered substantial attention. Cao et al. (51) discovered that the overexpression of JUN significantly suppressed the impact of T4O on both glioma cell proliferation and ferroptosis. Luo et al. (52) demonstrated that MAPK8 could reverse the impact of LINC01564 ablation on both cell apoptosis and ferroptosis.
However, this study still has some limitations. First, this work utilized a small sample size, which might lead to bias. Second, more in-vivo and in-vitro studies will be required to verify the reliability and significance of the results. Finally, it is necessary to understand the hub genes of RA for diagnosis and treatment.
In conclusion, we derived 34 potential ferroptosis-related genes between RA patients and healthy individuals using bioinformatics method. There were four genes (GABARPL1, DUSP1, JUN, and MAPK8) were verified to be differentially expressed and may serve as important diagnostic markers and new potential therapeutic targets for RA through the regulation of ferroptosis. Our study may be beneficial to enhance the understanding of RA pathogenesis and potential for clinical use in the future.
The original contributions presented in the study are included in the article/supplementary material, further inquiries can be directed to the corresponding authors.
XL designed the study. XL and AH supervised the study and contributed equally to this work and should be regarded as co-first authors. XL, AH, YL, YH, and XZ analyze the data. XL and YL did the digital visualization and wrote and revised the manuscript. All authors contributed to the article and approved the submitted version.
This work was supported by the National Natural Science Foundation of China (62202115), China Postdoctoral Science Foundation (2022M722746), and Zhejiang Province Natural Science Foundation (Q23H010006).
The paper is published in a preprint (41) according to the following link: https://www.researchsquare.com/article/rs-2380843/v1.
The authors declare that the research was conducted in the absence of any commercial or financial relationships that could be construed as a potential conflict of interest.
All claims expressed in this article are solely those of the authors and do not necessarily represent those of their affiliated organizations, or those of the publisher, the editors and the reviewers. Any product that may be evaluated in this article, or claim that may be made by its manufacturer, is not guaranteed or endorsed by the publisher.
1. Kuchler-Bopp, S, Mariotte, A, Strub, M, Po, C, De Cauwer, A, Schulz, G, et al. Temporomandibular joint damage in K/BxN arthritic mice. Int J Oral Sci. (2020) 12:5. doi: 10.1038/s41368-019-0072-z
2. Ansari, MA, Nadeem, A, Bakheet, SA, Attia, SM, Shahid, M, Alyousef, FS, et al. Chemokine receptor 5 antagonism causes reduction in joint inflammation in a collagen-induced arthritis mouse model. Molecules. (2021) 26:1839. doi: 10.3390/molecules26071839
3. Wei, K, Nguyen, HN, and Brenner, MB. Fibroblast pathology in inflammatory diseases. J Clin Invest. (2021) 13,20:e149538. doi: 10.1172/jci149538
4. Zhao, T, Yang, Q, Xi, Y, Xie, Z, Shen, J, Li, Z, et al. Ferroptosis in rheumatoid arthritis: a potential therapeutic strategy. Front Immunol. (2022) 13:779585. doi: 10.3389/fimmu.2022.779585
5. Dixon, SJ, Lemberg, KM, Lamprecht, MR, Skouta, R, Zaitsev, EM, Gleason, CE, et al. Ferroptosis: an iron-dependent form of nonapoptotic cell death. Cells. (2012) 149:1060–72. doi: 10.1016/j.cell.2012.03.042
6. Li, J, Cao, F, Yin, HL, Huang, ZJ, Lin, ZT, Mao, N, et al. Ferroptosis: past, present and future. Cell Death Dis. (2020) 11:88. doi: 10.1038/s41419-020-2298-2
7. Bebber, CM, Müller, F, Prieto Clemente, L, Weber, J, and von Karstedt, S. Ferroptosis in cancer cell biology. Cancers. (2020) 12:1. doi: 10.3390/cancers12010164
8. Tsurusaki, S, Tsuchiya, Y, Koumura, T, Nakasone, M, Sakamoto, T, Matsuoka, M, et al. Hepatic ferroptosis plays an important role as the trigger for initiating inflammation in nonalcoholic steatohepatitis. Cell Death Dis. (2019) 10:449. doi: 10.1038/s41419-019-1678-y
9. Linkermann, A, Skouta, R, Himmerkus, N, Mulay, SR, Dewitz, C, De Zen, F, et al. Synchronized renal tubular cell death involves ferroptosis. Proc Natl Acad Sci U S A. (2014) 111:16836–41. doi: 10.1073/pnas.1415518111
10. Chen, B, Chen, Z, Liu, M, Gao, X, Cheng, Y, Wei, Y, et al. Inhibition of neuronal ferroptosis in the acute phase of intracerebral hemorrhage shows long-term cerebroprotective effects. Brain Res Bull. (2019) 153:122–32. doi: 10.1016/j.brainresbull.2019.08.013
11. Qiu, Y, Cao, Y, Cao, W, Jia, Y, and Lu, N. The application of Ferroptosis in diseases. Pharmacol Res. (2020) 159:104919. doi: 10.1016/j.phrs.2020.104919
12. Ursini, F, and Maiorino, M. Lipid peroxidation and ferroptosis: the role of GSH and GPx4. Free Radic Biol Med. (2020) 152:175–85. doi: 10.1016/j.freeradbiomed.2020.02.027
13. Chang, S, Tang, M, Zhang, B, Xiang, D, and Li, F. Ferroptosis in inflammatory arthritis: a promising future. Front Immunol. (2022) 13:955069. doi: 10.3389/fimmu.2022.955069
14. Woetzel, D, Huber, R, Kupfer, P, Pohlers, D, Pfaff, M, Driesch, D, et al. Identification of rheumatoid arthritis and osteoarthritis patients by transcriptome-based rule set generation. Arthritis Res Ther. (2014) 16:R84. doi: 10.1186/ar4526
15. Zhou, N, and Bao, J. FerrDb: a manually curated resource for regulators and markers of ferroptosis and ferroptosis-disease associations. Database. (2020) 2020:baaa021. doi: 10.1093/database/baaa021
16. Yu, G, Wang, LG, Han, Y, and He, QY. clusterProfiler: an R package for comparing biological themes among gene clusters. Omics. (2012) 16:284–7. doi: 10.1089/omi.2011.0118
17. Huber, R, Hummert, C, Gausmann, U, Pohlers, D, Koczan, D, Guthke, R, and Kinne, RW. Identification of intra-group, inter-individual, and gene-specific variances in mRNA expression profiles in the rheumatoid arthritis synovial membrane. JArthritis Res The. (2008) 10:R98. doi: 10.1186/ar2485
18. Wagner, AH, Coffman, AC, Ainscough, BJ, Spies, NC, Skidmore, ZL, Campbell, KM, et al. DGIdb 2.0: mining clinically relevant drug-gene interactions. Nucleic Acids Res. (2016) 44:D1036–44. doi: 10.1093/nar/gkv1165
19. Lamb, J, Crawford, ED, Peck, D, Modell, JW, Blat, IC, Wrobel, MJ, et al. The connectivity map: using gene-expression signatures to connect small molecules, genes, and disease. Science (New York, NY). (2006) 313:1929–35. doi: 10.1126/science.1132939
20. Jin, Y, Hu, D, Peterson, EL, Eng, C, Levin, AM, Wells, K, et al. Dual-specificity phosphatase 1 as a pharmacogenetic modifier of inhaled steroid response among asthmatic patients. J Allergy Clin Immunol. (2010) 126:618–25.e1-2. doi: 10.1016/j.jaci.2010.06.007
21. Abrams, MT, Robertson, NM, Litwack, G, and Wickstrom, E. Evaluation of glucocorticoid sensitivity in 697 pre-B acute lymphoblastic leukemia cells after overexpression or silencing of MAP kinase phosphatase-1. J Cancer Res Clin Oncol. (2005) 131:347–54. doi: 10.1007/s00432-004-0659-3
22. Xu, Q, Fawcett, TW, Gorospe, M, Guyton, KZ, Liu, Y, and Holbrook, NJ. Induction of mitogen-activated protein kinase phosphatase-1 during acute hypertension. Hypertension. (1997) 30:106–11. doi: 10.1161/01.hyp.30.1.106
23. Kang, KA, Zhang, R, Piao, MJ, Ko, DO, Wang, ZH, Kim, BJ, et al. Protective effect of irisolidone, a metabolite of kakkalide, against hydrogen peroxide induced cell damage via antioxidant effect. Bioorg Med Chem. (2008) 16:1133–41. doi: 10.1016/j.bmc.2007.10.085
24. Beutler, JA, Kang, MI, Robert, F, Clement, JA, Pelletier, J, Colburn, NH, et al. Quassinoid inhibition of AP-1 function does not correlate with cytotoxicity or protein synthesis inhibition. J Nat Prod. (2009) 72:503–6. doi: 10.1021/np800732n
25. Jones, MR, Schrader, KA, Shen, Y, Pleasance, E, Ch'ng, C, Dar, N, et al. Response to angiotensin blockade with irbesartan in a patient with metastatic colorectal cancer. Ann Oncol. (2016) 27:801–6. doi: 10.1093/annonc/mdw060
26. Kim, YJ, Kang, KS, Choi, KC, and Ko, H. Cardamonin induces autophagy and an antiproliferative effect through JNK activation in human colorectal carcinoma HCT116 cells. Bioorg Med Chem Lett. (2015) 25:2559–64. doi: 10.1016/j.bmcl.2015.04.054
27. Ma, FY, Flanc, RS, Tesch, GH, Han, Y, Atkins, RC, Bennett, BL, et al. A pathogenic role for c-Jun amino-terminal kinase signaling in renal fibrosis and tubular cell apoptosis. J Am Soc Nephrol. (2007) 18:472–84. doi: 10.1681/asn.2006060604
28. Atsriku, C, Hoffmann, M, Moghaddam, M, Kumar, G, and Surapaneni, S. In vitro metabolism of a novel JNK inhibitor tanzisertib: interspecies differences in oxido-reduction and characterization of enzymes involved in metabolism. Xenobiotica. (2015) 45:465–80. doi: 10.3109/00498254.2014.991367
29. Stebbins, JL, de, SK, Machleidt, T, Becattini, B, Vazquez, J, Kuntzen, C, et al. Identification of a new JNK inhibitor targeting the JNK-JIP interaction site. Proc Natl Acad Sci U S A. (2008) 105:16809–13. doi: 10.1073/pnas.0805677105
30. Petersson, M, Bucht, E, Granberg, B, and Stark, A. Effects of arginine-vasopressin and parathyroid hormone-related protein (1-34) on cell proliferation and production of YKL-40 in cultured chondrocytes from patients with rheumatoid arthritis and osteoarthritis. Osteoarthr Cartil. (2006) 14:652–9. doi: 10.1016/j.joca.2006.01.003
31. Chang, Y, and Wei, W. Angiotensin II in inflammation, immunity and rheumatoid arthritis. Clin Exp Immunol. (2015) 179:137–45. doi: 10.1111/cei.12467.
32. Zhou, T, Xie, Y, Hou, X, Bai, W, Li, X, Liu, Z, et al. Irbesartan overcomes gemcitabine resistance in pancreatic cancer by suppressing stemness and iron metabolism via inhibition of the hippo/YAP1/c-Jun axis. J Exp Clin Cancer Res. (2023) 42:111. doi: 10.1186/s13046-023-02671-8
33. Wang, SJ, Li, D, Ou, Y, Jiang, L, Chen, Y, Zhao, Y, et al. Acetylation is crucial for p53-mediated Ferroptosis and tumor suppression. Cell Rep. (2016) 17:366–73. doi: 10.1016/j.celrep.2016.09.022
34. Zhang, Y, Shi, J, Liu, X, Feng, L, Gong, Z, Koppula, P, et al. BAP1 links metabolic regulation of ferroptosis to tumour suppression. Nat Cell Biol. (2018) 20:1181–92. doi: 10.1038/s41556-018-0178-0
35. Kim, J, Kim, HY, Song, SY, Go, SH, Sohn, HS, Baik, S, et al. Synergistic oxygen generation and reactive oxygen species scavenging by manganese ferrite/ceria co-decorated nanoparticles for rheumatoid arthritis treatment. ACS Nano. (2019) 13:3206–17. doi: 10.1021/acsnano.8b08785
36. Schaid, DJ, Chen, W, and Larson, NB. From genome-wide associations to candidate causal variants by statistical fine-mapping. Nat Rev Genet. (2018) 19:491–504. doi: 10.1038/s41576-018-0016-z
37. Kok, SH, Lin, LD, Hou, KL, Hong, CY, Chang, CC, Hsiao, M, et al. Simvastatin inhibits cysteine-rich protein 61 expression in rheumatoid arthritis synovial fibroblasts through the regulation of sirtuin-1/FoxO3a signaling. Arthritis Rheum. (2013) 65:639–49. doi: 10.1002/art.37807
38. Lee, KI, Choi, S, Matsuzaki, T, Alvarez-Garcia, O, Olmer, M, Grogan, SP, et al. FOXO1 and FOXO3 transcription factors have unique functions in meniscus development and homeostasis during aging and osteoarthritis. Proc Natl Acad Sci U S A. (2020) 117:3135–43. doi: 10.1073/pnas.1918673117
39. Bouaziz, W, Sigaux, J, Modrowski, D, Devignes, CS, Funck-Brentano, T, Richette, P, et al. Interaction of HIF1α and β-catenin inhibits matrix metalloproteinase 13 expression and prevents cartilage damage in mice. Proc Natl Acad Sci U S A. (2016) 113:5453–8. doi: 10.1073/pnas.1514854113
40. Vattakuzhi, Y, Abraham, SM, Freidin, A, Clark, AR, and Horwood, NJ. Dual-specificity phosphatase 1-null mice exhibit spontaneous osteolytic disease and enhanced inflammatory osteolysis in experimental arthritis. Arthritis Rheum. (2012) 64:2201–10. doi: 10.1002/art.34403
41. Baxter, D, Perry, SR, Hill, TA, Kok, WM, Zaccai, NR, Brady, RL, et al. Downsizing proto-oncogene cFos to short Helix-constrained peptides that bind Jun. ACS Chem Biol. (2017) 12:2051–61. doi: 10.1021/acschembio.7b00303
42. Huber, R, Stuhlmüller, B, Kunisch, E, and Kinne, RW. Discrepancy between Jun/Fos proto-oncogene mRNA and protein expression in the rheumatoid arthritis synovial membrane. Arthritis Res Ther. (2020) 3:181–94. doi: 10.3390/j3020015
43. Johnson, GL, and Lapadat, R. Mitogen-activated protein kinase pathways mediated by ERK, JNK, and p38 protein kinases. Science (New York, NY). (2002) 298:1911–2. doi: 10.1126/science.1072682
44. Ding, C. Drug evaluation: VX-702, a MAP kinase inhibitor for rheumatoid arthritis and acute coronary syndrome. Curr Opin Investig Drugs. (2006) 7:1020–1025.
45. le Grand, JN, Chakrama, FZ, Seguin-Py, S, Fraichard, A, Delage-Mourroux, R, Jouvenot, M, et al. GABARAPL1 (GEC1): original or copycat? Autophagy. (2011) 7:1098–107. doi: 10.4161/auto.7.10.15904
46. Gao, M, Monian, P, Pan, Q, Zhang, W, Xiang, J, and Jiang, X. Ferroptosis is an autophagic cell death process. Cell Res. (2016) 26:1021–32. doi: 10.1038/cr.2016.95
47. Fan, M, Chen, J, Gao, J, Xue, W, Wang, Y, Li, W, et al. Triggering a switch from basal- to luminal-like breast cancer subtype by the small-molecule diptoindonesin G via induction of GABARAPL1. Cell Death Dis. (2020) 11:635. doi: 10.1038/s41419-020-02878-z
48. Berthier, A, Seguin, S, Sasco, AJ, Bobin, JY, De Laroche, G, Datchary, J, et al. High expression of gabarapl1 is associated with a better outcome for patients with lymph node-positive breast cancer. Br J Cancer. (2010) 102:1024–31. doi: 10.1038/sj.bjc.6605568
49. Boyer-Guittaut, M, Poillet, L, Liang, Q, Bôle-Richard, E, Ouyang, X, Benavides, GA, et al. The role of GABARAPL1/GEC1 in autophagic flux and mitochondrial quality control in MDA-MB-436 breast cancer cells. Autophagy. (2014) 10:986–1003. doi: 10.4161/auto.28390
50. Xie, Y, Kuang, F, Liu, J, Tang, D, and Kang, R. DUSP1 blocks autophagy-dependent ferroptosis in pancreatic cancer. J Pancreatol. (2020) 3:154–60. doi: 10.1097/jp9.0000000000000054
51. Cao, W, Li, Y, Zeng, Z, and Lei, S. Terpinen-4-ol induces Ferroptosis of glioma cells via downregulating JUN proto-oncogene. Molecules. (2023) 28:4643. doi: 10.3390/molecules28124643
Keywords: rheumatoid arthritis, ferroptosis, bioinformatics analysis, therapeutic targets, pathway analysis
Citation: Li X, He A, Liu Y, Huang Y and Zhang X (2023) Bioinformatics identification of ferroptosis-related genes and therapeutic drugs in rheumatoid arthritis. Front. Med. 10:1192153. doi: 10.3389/fmed.2023.1192153
Received: 24 March 2023; Accepted: 19 June 2023;
Published: 13 July 2023.
Edited by:
Weikuan Gu, University of Tennessee Health Science Center (UTHSC), United StatesReviewed by:
Yanhong Cao, Harbin Medical University, ChinaCopyright © 2023 Li, He, Liu, Huang and Zhang. This is an open-access article distributed under the terms of the Creative Commons Attribution License (CC BY). The use, distribution or reproduction in other forums is permitted, provided the original author(s) and the copyright owner(s) are credited and that the original publication in this journal is cited, in accordance with accepted academic practice. No use, distribution or reproduction is permitted which does not comply with these terms.
*Correspondence: Xianbin Li, bGl4Yjg4QGd6aHUuZWR1LmNu; Xueli Zhang, emhhbmd4dWVsaTE5ODhAc2luYS5jbg==
†These authors have contributed equally to this work and share first authorship
Disclaimer: All claims expressed in this article are solely those of the authors and do not necessarily represent those of their affiliated organizations, or those of the publisher, the editors and the reviewers. Any product that may be evaluated in this article or claim that may be made by its manufacturer is not guaranteed or endorsed by the publisher.
Research integrity at Frontiers
Learn more about the work of our research integrity team to safeguard the quality of each article we publish.