- 1College of Pharmacy and Institute of Pharmaceutical Science and Technology, Hanyang University, Ansan, Republic of Korea
- 2Department of Oral and Maxillofacial Surgery, School of Medicine, Ewha Womans University, Seoul, Republic of Korea
- 3College of Pharmacy and Graduate School of Pharmaceutical Sciences, Ewha Womans University, Seoul, Republic of Korea
Objective: The purpose of this study was to evaluate the effect of estrogen receptor 1 (ESR1) polymorphisms on the development of medication-related osteonecrosis of the jaws (MRONJ) in women with osteoporosis.
Methods: A total of 125 patients taking bisphosphonates was evaluated the relationship between MRONJ occurrence and single nucleotide polymorphisms (SNPs) of ESR1. Clinical information was collected, including current age, treatment duration, and comorbidity. Univariate and Multivariable regression analyzes were performed to evaluate the independent predictive factors for MRONJ occurrence. Predictive models were constructed using machine learning methods such as Lasso regression, Random forest (RF), and Support vector machine (SVM). The area under the receiver-operating curve (AUROC) was used to evaluate the performance of a binary classifier.
Result: Two SNPs of ESR1 (rs4870056 and rs78177662) were significantly associated with MRONJ development. Patients with variant allele (A) of rs4870056 showed 2.45 times (95% CI, 1.03–5.87) the odds of MRONJ occurrence compared to those with wild-type homozygote (GG) after adjusting covariates. Additionally, carriers with variant allele (T) of rs78177662 had higher odds than those with wild-type homozygote (CC) (adjusted odds ratio (aOR), 2.64, 95% CI, 1.00–6.94). Among demographic variables, age ≥ 72 years (aOR, 3.98, 95% CI, 1.60–9.87) and bisphosphonate exposure ≥48 months (aOR, 3.16, 95% CI, 1.26–7.93) were also significant risk factors for MRONJ occurrence. AUROC values of machine learning methods ranged between 0.756–0.806 in the study.
Conclusion: Our study showed that the MRONJ occurrence was associated with ESR1 polymorphisms in osteoporotic women.
1. Introduction
Bisphosphonates (BPs) are commonly used agents with anti-resorptive actions for skeletal protection in osteoporosis, multiple myeloma, and cancer bone metastasis (1, 2). Despite their clinical usefulness, they have some limitations because of safety concerns. In 2003, Marx first reported 36 cases of jaw bone necrosis that appeared to be related to BPs (3). Since then, similar cases have been reported, which are commonly called bisphosphonate-related osteonecrosis of the jaws (4, 5). In 2014, the American Association of Oral and Maxillofacial Surgeons (AAOMS) changed to the broad term “Medication-Related Osteonecrosis of the Jaws” (MRONJ) (6), as it is not only caused by bisphosphonates, but also by other anti-resorptive and anti-angiogenic drugs (7).
As case reports of MRONJ increased, a large number of researches have been performed to find the mechanisms, and its pathogenesis presumed disturbed bone remodeling, inflammation or infection, altered immunity, and angiogenesis inhibition (8–10). It was reported that 63.7% of MRONJ cases are related to tooth extraction (11). Therefore, the influence of medications including BPs on bone remodeling has been extensively studied (12). Bone remodeling consists of three consecutive phases: resorption, reversal, and formation (13, 14). During the healing period of tooth extraction, especially in the early stages, osteoclastic resorption of the bone is considered the first important feature to repair from bone remodeling (15).
Estrogen is a well-known regulator of bone turnover and acts by binding estrogen receptors (ESR) (16–18). For bone remodeling, it has been reported that estrogen receptor 1 (ESR1, ERα) affects both osteoblasts and osteoclasts, and ESR2 (ERβ) mainly affects osteoblasts (19–21). ESR1 mediates the direct effects of estrogen on osteoclasts. For the effects on osteoblast, estrogen binds ESR1 of osteoblast progenitors and leads to attenuated bone resorption at the endo-cortical surface. Additionally, the ESR1 of osteoblast progenitors stimulates periosteal bone development in response to mechanical strain, independently of estrogens (22, 23).
ESR1 is located on chromosome 6q25. Genetic screening of the ESR1 has found several meaningful polymorphic sites. Among polymorphisms, rs2234693 (PvuII) has been studied most actively, especially for its effects on bone mineral density and fracture risk, although results are conflicting (24, 25). Given the wide role of ESR1 in bone turnover, ESR1 polymorphisms in postmenopausal women could also be considered in the occurrence of MRONJ. However, it has not been studied much possibly because of involving complex processes (25).
Moreover, many of MRONJ studies have been carried out in oncology patients with solid tumors and multiple myeloma (24, 26). In addition, most such studies enrolled healthy controls without taking a BP (27, 28).
Therefore, the aim of the present study is to investigate the association between ESR1 polymorphism and MRONJ occurrence in osteoporosis patients taking BPs. Additionally, to quantify the risk of MRONJ, this study attempts to construct a predictive model to apply several machine learning techniques.
2. Methods
2.1. Patients and data collection
This study was an analysis of prospectively collected saliva samples from January 2014 to December 2018 at EWHA Womans University Mokdong Hospital. The detailed explanation of the study patients have already been provided in our previous paper (29). Briefly, all participants with current or previous BP use were diagnosed with osteoporosis by a physician. MRONJ was diagnosed by oral surgeons in accordance with AAOMS’ guidelines. Case group was identified as those who developed MRONJ, and control group was defined as those who had not developed MRONJ after dentoalveolar surgery. Clinical information was collected during the patients’ outpatient clinic visits. The collected clinical information included patients’ age, comorbidities, and duration of BP use.
The study protocol was approved by ethics committee of EWHA Womans University Mokdong Hospital Institutional Review Board (IRB number: 14–13-01), and written informed consent was obtained from all patients before their participation in the study.
2.2. Genotyping
Genomic DNA was extracted from saliva samples collected using the tube format (OG300) of the Oragene®•DNA Self-Collection Kit (DNAgenotek, Ontario, Canada), according to manufacturer’s instructions. SNPs of ESR1 were selected based on other studies and genetic information obtained from the SNP database of the National Center for Biotechnology Information (dbSNP) (25, 30–32). For the selection of ESR1 SNPs with minor allele frequency (MAF) of ≥20% in Japanese and Han Chinese populations, the following two methods were used: genetic information was obtained from Haploreg v4.1 and the tagger function was implemented in Haploview v4.2 program (33). Linkage disequilibrium blocks were constructed following the D′ method (34). One SNP in 5’UTR (rs78177662), eight intronic SNPs (rs827420, rs4870056, rs6912184, rs722208, rs851967, rs17081716, rs2175898, rs9371226) were selected.
2.3. In silico analysis
Several computational tools were used to predict the possible effects of given variants on splicing. ESEfinder, SpliceAid2, and EX-SKIP were used to evaluate alternations of the splicing factor-binding site pattern caused by the given point mutation (35–37). The default threshold value was used and a score for a sequence above the threshold was considered to be potentially significant. Also, most splicing factors bind short (4–10 nucleotide) and degenerate sequences (38). For SNP sequence information, a total of 15 nucleotides was used for analysis using dbSNP.
2.4. Statistical analysis and machine learning methods
The chi-square and Student’s t-test were used to compare categorical and continuous variables between case and control groups, respectively. Multivariable logistic regression analysis was used to examine independent risk factors for MRONJ. The clinical and genetic variables selected were those with p < 0.2 on univariate analysis. Variables were entered by stepwise selection for p < 0.1 and were removed for p > 0.05. Odds ratios (ORs) and adjusted odds ratios (aORs) were calculated from univariate and multivariable analyzes, respectively. All statistical tests were conducted with a two-tailed alpha of 0.05. Machine learning models were run on each dataset in 5 iterations of tenfold cross-validation on hyperparameter tuning to enhance the effectiveness of verification. Train and verification data were divided into 0.75 and 0.25 of the total. The importance of features of the model was also confirmed. Discrimination of the model was assessed by an analysis of the area under receiver operating curve (AUROC) and its 95% confidence interval (CI) of each model (39).
A risk scoring system was developed from the multivariable and machine learning analyzes. We randomly divided the data by a ratio of 75:25. To obtain the risk score, each coefficient from the logistic regression model was divided by the smallest one and rounded to two decimal places.
Statistical Package for Social Sciences Version 20.0 for Windows (SPSS, Chicago, IL, United States,1) was used for all analyzes. Machine learning algorithms were constructed using R software version 3.6.0 (RFoundation for Statistical Computing, Vienna, Austria,2).
3. Results
Initially, a total of 149 patients were screened and enrolled in this study. Twenty who had additional indications other than osteoporosis, two who had missed clinical information, and two men were excluded. A total of 125 patients were included in the final analysis and 58 (46.4%) developed MRONJ after dental procedures. Table 1 shows the demographic and clinical characteristics of the study population stratified by MRONJ occurrence. The mean age was 72.9 ± 9.4, and the most frequent comorbidity was hypertension. Sixty four patients had a history of hypertension, which showed statistical significance in relation to MRONJ occurrence (p = 0.024). The proportion of patients who had taken BPs for 48 months or longer was higher in the case group than the control group (p = 0.003).
The effects of 9 SNPs of ESR1 on the occurrence of MRONJ were evaluated (Table 2). Minor allele frequencies (MAFs) in our study population ranged from 28.9 to 48.8%. In univariate analysis, rs827420 (G > A) was significantly associated with the occurrence of MRONJ. Carriers with variant A allele of rs827420 had a higher incidence rate of MRONJ than did those with wild homozygote carriers (p = 0.02). The polymorphisms of rs4870056 (G > A), rs78177662 (C > T), rs851967 (C > T) and rs9371226 (G > T) reached marginal significance (Table 2).
For the multivariable analysis, the SNP of rs827420 was excluded because of multi-collinearity with age variable in our population. After adjusting covariates, carriers with variant allele of rs4870056 had approximately 2.45 times (95% CI 1.03–5.87) the odds of MRONJ occurrence compared to those with wild-type homozygotes (GG). Carriers with variant T allele of rs78177662 had 2.64 times higher incidence of MRONJ than did those with other genotypes. The age over 72 years (aOR 3.98, 95% CI 1.60–9.87) and treatment duration over 48 months (aOR 3.16, 95% CI 1.26–7.93) were both significant clinical predictors of MRONJ development (Table 3).
Analysis of SNP rs4870056 with ESEfinder 3.0, which detects exonic splicing enhancer (ESE) for the SR protein, showed that this mutation decreased the score of SF2/ASF binding from −0.308 to −2.372 (threshold: 1.956). The polymorphism of rs78177662, which located on 5’UTR also associated with the change of exonic splicing silencer (ESS)/ESE ratio in EX-SKIP program, showed that this mutation increased the ESS/ESE ratio from 0.00 to 2.00 (Table 4).
The top four important features of machine learning methods were the highest at aged over 72 years, followed by duration over 48 months from Random forest (RF) and Support vector machine (SVM) and rs4870056 from Lasso regression (Table 5). The AUROC values (mean, 95% CI) using ten-fold cross-validated Lasso regression, SVM and RF models were 0.756 (0.573–0.938), 0.761 (0.583–0.939), and 0.806 (0.645–0.967), respectively (Figure 1). The hyperparameters and R code that we used are shown in Supplementary file S1.
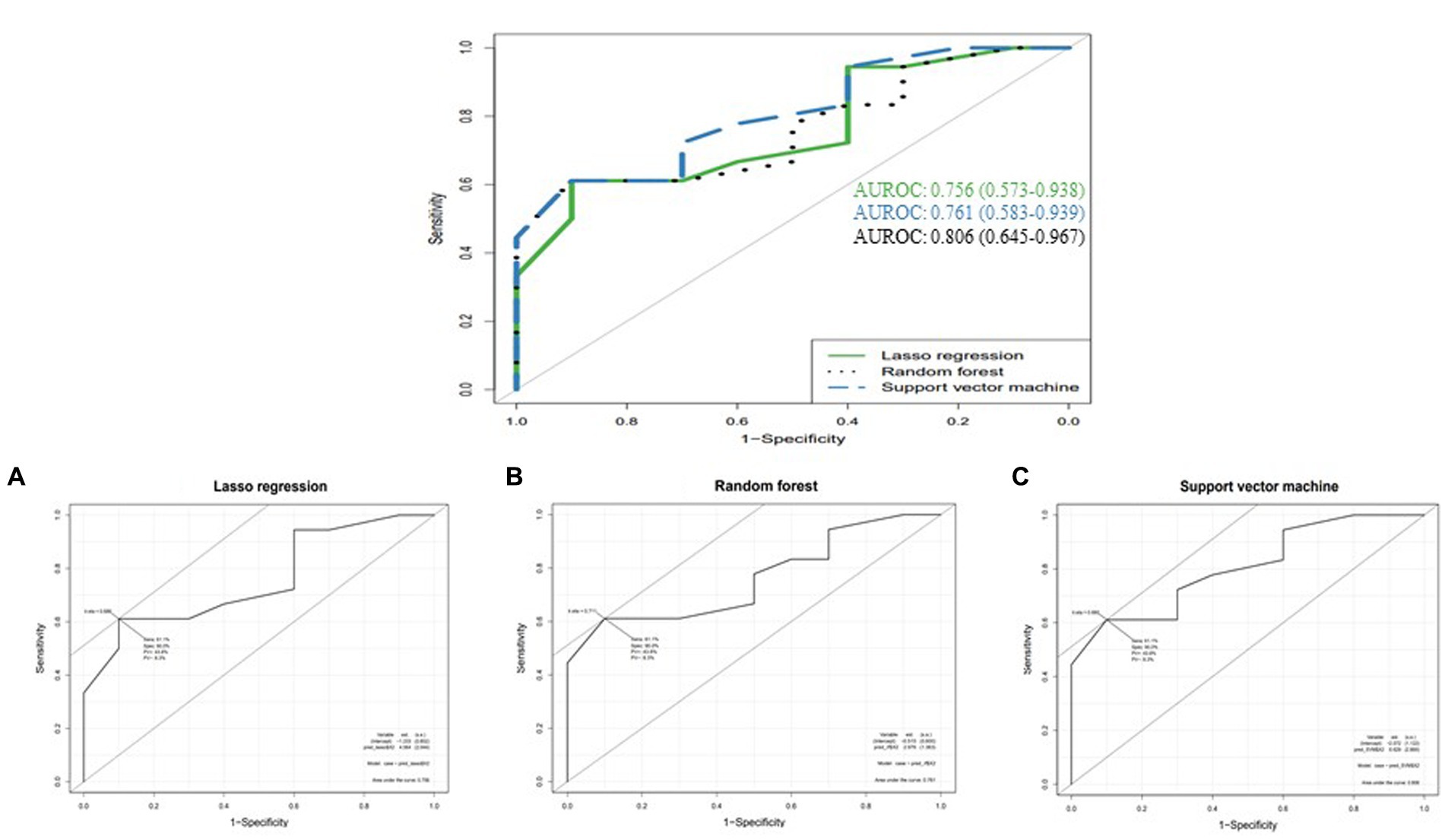
Figure 1. Comparison of AUROCs between machine learning methods (A) Lasso regression, (B) Random forest, and (C) Support vector machine.
Patients with 0, 1–2, 2–3, 3–4, and 4–5 points on the training set showed approximately 0, 20, 41, 63, and 92% risk of MRONJ, respectively. The respective value of the validation set was 0, 25, 36, 71, and 100%. The logistic regression curve by mapping the scores to risk scores is presented in Figure 2.
4. Discussion
This study showed that ESR1 polymorphisms affected MRONJ development in postmenopausal women. Variant A allele of rs4870056 and variant T allele of rs78177662 increased the risk of MRONJ by 2.45 fold and 2.64 fold, respectively. Patients aged 72 and older, and treatment duration over 48 months had an increased risk of MRONJ by 3.98 fold and 3.16, respectively. Machine learning analyzes indicated good performance (higher than 0.7) of the constructed model.
Previous pharmacogenomic studies have shown the relationships of some genes with the occurrence of MRONJ using population-based or healthy controls without taking BPs (27, 28, 40). In contrast, our group recently reported that VEGFA polymorphisms, involved in the modulation of angiogenesis, could affect the development of MRONJ, comparing osteoporotic controls taking BPs (29). Furthermore, it may essential in our study population to evaluate the impact of genetic variants of ESR1, which encodes estrogen receptor that binds estrogen playing a crucial role in bone turnover.
BPs suppress mandibular bone remodeling in experimental animals (41). It has been proposed that reduced jaw bone turnover may impede the healing process even for a mild injury such as a dental extraction (42). Estrogen deprivation in postmenopausal women results in accelerated bone resorption and remodeling (43–45). It leads to reduce bone turnover. Another potential mechanism is that ESR1 affects the activation of the insulin-like growth factor 1 (IGF-1) signaling pathway, mainly involving anti-inflammatory reactions and re-epithelialization (46, 47). IGF-1 can promote wound recovery in estrogen-deficient animal models. However, it showed that IGF-1 does not promote healing and increases inflammatory responses in ESR1-null mice (48). In patients taking BPs, it suggested that ESR1 polymorphism leads to decreased bone turnover and poor wound healing and may contribute to the development of MRONJ (25).
The SNP of rs4870056, located in ESR1 intron, this SNP has been studied in several other clinical fields. It reported that rs4870056 was associated with cardiovascular disease risk during postmenopausal hormone therapy (49, 50). Checking the linkage disequilibrium, rs4870056 appeared to have a strong relationship with rs2234693 (Pvull), which is currently one of the most widely studied SNPs among ESR1 SNPs. From studies using rs2234693, it was explained that the SNP might contribute to determining of bone mineral density and fracture in postmenopausal women (32, 51, 52). In addition, in silico analysis showed that splicing factors tended to decrease due to changes in motifs in the mutant sequence. The polymorphism of rs78177662 is located at 5’UTR, but the current study has not revealed its function. It could only be inferred from the in silico analysis.
Recently, several studies reported the influence of gene polymorphism on the development of disease and drug-related adverse reactions applying machine learning (53–55). Some machine learning methods such as RF, SVM, and decision trees classification were used, and the performance of each model was compared with AUROC values (53). In this study, the purpose of generating models is to provide a warning for and screen a group with an increased risk for MRONJ occurrence. Applying machine learning methods can improve the performance in predicting (55, 56). To evaluate AUROC values, all our machine learning models employed performed well. Lasso regularization shrinks regression coefficients toward zero, effectively selecting significant predictors and improving the interpretability of the model (57). As a result of regulating variable weights with lasso, 0.756 of AUROC value was obtained. Using the bagging technique, RF can generate multiple versions of predictors, and use them to obtain aggregated predictors (58). An AUROC value was 0.761 from the test set. As another classification model is the maximization of separating margin, and the AUROC value of SVM was 0.806 after setting the optimal cost and gamma using the tune function (59).
Risk assessment systems, including biomarkers and risk factors, are helpful for rapid clinical decision-making. Risk scoring systems for adverse drug reactions (ADR), such as the GerontoNet ADR risk score, have been developed (60). Therefore, this study developed the risk scoring system for MRONJ occurrence in addition to previous work. The constructed risk scoring system may be helpful to screen the high-risk group for MRONJ occurrence, and further studies are needed to improve its generalizability.
This study has several limitations due to the study design and sample size. The mechanism that polymorphisms of ESR1 affect osteonecrosis could not be investigated. However, we attempted to understand the roles of SNP through in silico analysis. The study’s strength is that it collected data from MRONJ patients with osteoporosis and created predictive models and a risk-scoring system through machine learning methods. This study showed that ESR1 polymorphism in female patients with estrogen deficiency was associated with medication-related osteonecrosis.
5. Conclusion
Our study showed that MRONJ development was associated with ESR1 gene polymorphism in osteoporotic women. Predictive modeling created through machine learning techniques showed good performances. As a result, from the perspective of bone remodeling, it suggests the possibility of predictive diagnosis.
Data availability statement
The original contributions presented in the study are publicly available. This data can be found here: https://www.ncbi.nlm.nih.gov/SNP/snp_viewBatch.cgi?sbid=1063487.
Ethics statement
The studies involving human participants were reviewed and approved by EWHA Womans University Mokdong Hospital. The patients/participants provided their written informed consent to participate in this study.
Author contributions
HG and J-EC: conceptualization. J-WK and S-JK: data curation. S-YC, S-HO, and SC: formal analysis. J-WK and J-EC: funding acquisition. JY, HG, and J-EC: methodology. J-WK, HG, and J-EC: supervision. S-YC, HG, and J-EC: writing–original draft. S-YC, J-WK, S-HO, SC, JY, S-JK, HG, and J-EC: writing–review and editing. All authors contributed to the article and approved the submitted version.
Funding
This work was supported by a grant of the Korea Health Technology R&D Project through the Korea Health Industry Development Institute (KHIDI) funded by the Ministry of Health & Welfare, Republic of Korea (HI22C1377), and the National Research Foundation of Korea (NRF) grants funded by the Ministry of Education (2020R1A2C4001842 and 2022R1F1A1075439).
Conflict of interest
The authors declare that the research was conducted in the absence of any commercial or financial relationships that could be construed as a potential conflict of interest.
Publisher’s note
All claims expressed in this article are solely those of the authors and do not necessarily represent those of their affiliated organizations, or those of the publisher, the editors and the reviewers. Any product that may be evaluated in this article, or claim that may be made by its manufacturer, is not guaranteed or endorsed by the publisher.
Supplementary material
The Supplementary material for this article can be found online at: https://www.frontiersin.org/articles/10.3389/fmed.2023.1140620/full#supplementary-material
Footnotes
References
1. Drake, MT, Clarke, BL, and Khosla, S. Bisphosphonates: mechanism of action and role in clinical practice. Mayo Clin Proc. (2008) 83:1032–45. doi: 10.4065/83.9.1032
2. Rogers, MJ. New insights into the molecular mechanisms of action of bisphosphonates. Curr Pharm Des. (2003) 9:2643–58. doi: 10.2174/1381612033453640
3. Marx, RE. Pamidronate (Aredia) and zoledronate (Zometa) induced avascular necrosis of the jaws: a growing epidemic. J Oral Maxillofac Surg. (2003) 61:1115–7. doi: 10.1016/S0278-2381(03)00720-1
4. Bedogni, A, Fusco, V, Agrillo, A, and Campisi, G. Learning from experience. Proposal of a refined definition and staging system for bisphosphonate-related osteonecrosis of the jaw (BRONJ). Oral Dis. (2012) 18:621–3. doi: 10.1111/j.1601-0825.2012.01903.x
5. Sarasquete, ME, García-Sanz, R, Marín, L, Alcoceba, M, Chillón, MC, Balanzategui, A, et al. Bisphosphonate-related osteonecrosis of the jaw is associated with polymorphisms of the cytochrome P450 CYP2C8 in multiple myeloma: a genome-wide single nucleotide polymorphism analysis. Blood. (2008) 112:2709–12. doi: 10.1182/blood-2008-04-147884
6. Ruggiero, SL, Dodson, TB, Fantasia, J, Goodday, R, Aghaloo, T, and Mehrotra, B. American association of oral and maxillofacial surgeons position paper on medication-related osteonecrosis of the jaw -- 2014 update. J Oral Maxillofac Surg. (2014) 72:1938–56. doi: 10.1016/j.joms.2014.04.031
7. King, R, Tanna, N, and Patel, V. Medication-related osteonecrosis of the jaw unrelated to bisphosphonates and denosumab-a review. Oral Surg Oral Med Oral Pathol Oral Radiol. (2019) 127:289–99. doi: 10.1016/j.oooo.2018.11.012
8. Aghaloo, T, Hazboun, R, and Tetradis, S. Pathophysiology of osteonecrosis of the jaws. Oral Maxillofac Surg Clin North Am. (2015) 27:489–96. doi: 10.1016/j.coms.2015.06.001
9. Chang, J, Hakam, AE, and McCauley, LK. Current understanding of the pathophysiology of osteonecrosis of the jaw. Curr Osteoporos Rep. (2018) 16:584–95. doi: 10.1007/s11914-018-0474-4
10. Kishimoto, H, Noguchi, K, and Takaoka, K. Novel insight into the management of bisphosphonate-related osteonecrosis of the jaw (BRONJ). Jpn Dent Sci Rev. (2019) 55:95–102. doi: 10.1016/j.jdsr.2018.09.002
11. Vescovi, P, Campisi, G, Fusco, V, Mergoni, G, Manfredi, M, Merigo, E, et al. Surgery-triggered and non surgery-triggered bisphosphonate-related osteonecrosis of the jaws (BRONJ): a retrospective analysis of 567 cases in an Italian multicenter study. Oral Oncol. (2011) 47:191–4. doi: 10.1016/j.oraloncology.2010.11.007
12. Jensen, PR, Andersen, TL, Chavassieux, P, Roux, J-P, and Delaisse, J-M. Bisphosphonates impair the onset of bone formation at remodeling sites. Bone. (2021) 145:115850. doi: 10.1016/j.bone.2021.115850
13. Hadjidakis, DJ, and Androulakis, II. Bone remodeling. Ann N Y Acad Sci. (2006) 1092:385–96. doi: 10.1196/annals.1365.035
14. Kenkre, JS, and Bassett, J. The bone remodelling cycle. Ann Clin Biochem. (2018) 55:308–27. doi: 10.1177/0004563218759371
15. Iizuka, T, Miller, SC, and Marks, SC Jr. Alveolar bone remodeling after tooth extraction in normal and osteopetrotic (ia) rats. J Oral Pathol Med. (1992) 21:150–5. doi: 10.1111/j.1600-0714.1992.tb00092.x
16. Deroo, BJ, and Korach, KS. Estrogen receptors and human disease. J Clin Invest. (2006) 116:561–70. doi: 10.1172/jci27987
17. Heldring, N, Pike, A, Andersson, S, Matthews, J, Cheng, G, Hartman, J, et al. Estrogen receptors: how do they signal and what are their targets. Physiol Rev. (2007) 87:905–31. doi: 10.1152/physrev.00026.2006
18. Tang, ZR, Zhang, R, Lian, ZX, Deng, SL, and Yu, K. Estrogen-receptor expression and function in female reproductive disease. Cells. (2019) 8:1123. doi: 10.3390/cells8101123
19. Almeida, M, Iyer, S, Martin-Millan, M, Bartell, SM, Han, L, Ambrogini, E, et al. Estrogen receptor-α signaling in osteoblast progenitors stimulates cortical bone accrual. J Clin Invest. (2013) 123:394–404. doi: 10.1172/jci65910
20. Khalid, AB, and Krum, SA. Estrogen receptors alpha and beta in bone. Bone. (2016) 87:130–5. doi: 10.1016/j.bone.2016.03.016
21. Martin-Millan, M, Almeida, M, Ambrogini, E, Han, L, Zhao, H, Weinstein, RS, et al. The estrogen receptor-alpha in osteoclasts mediates the protective effects of estrogens on cancellous but not cortical bone. Mol Endocrinol. (2010) 24:323–34. doi: 10.1210/me.2009-0354
22. Harris, HA, Albert, LM, Leathurby, Y, Malamas, MS, Mewshaw, RE, Miller, CP, et al. Evaluation of an estrogen receptor-beta agonist in animal models of human disease. Endocrinology. (2003) 144:4241–9. doi: 10.1210/en.2003-0550
23. Windahl, SH, Hollberg, K, Vidal, O, Gustafsson, JA, Ohlsson, C, and Andersson, G. Female estrogen receptor beta−/− mice are partially protected against age-related trabecular bone loss. J Bone Miner Res. (2001) 16:1388–98. doi: 10.1359/jbmr.2001.16.8.1388
24. Cardemil, C, Thomsen, P, and Larsson Wexell, C. Jaw bone samples from bisphosphonate-treated patients: a pilot cohort study. Clin Implant Dent Relat Res. (2015) 17:e679–91. doi: 10.1111/cid.12307
25. Peržeľová, V, Sabol, F, Vasilenko, T, Novotný, M, Kováč, I, Slezák, M, et al. Pharmacological activation of estrogen receptors-α and -β differentially modulates keratinocyte differentiation with functional impact on wound healing. Int J Mol Med. (2016) 37:21–8. doi: 10.3892/ijmm.2015.2351
26. Fung, PL, Nicoletti, P, Shen, Y, Porter, S, and Fedele, S. Pharmacogenetics of bisphosphonate-associated osteonecrosis of the jaw. Oral Maxillofac Surg Clin North Am. (2015) 27:537–46. doi: 10.1016/j.coms.2015.06.005
27. Kim, JH, Ko, YJ, Kim, JY, Oh, Y, Hwang, J, Han, S, et al. Genetic investigation of bisphosphonate-related osteonecrosis of jaw (BRONJ) via whole exome sequencing and bioinformatics. PLoS One. (2015) 10:e0118084. doi: 10.1371/journal.pone.0118084
28. Nicoletti, P, Cartsos, VM, Palaska, PK, Shen, Y, Floratos, A, and Zavras, AI. Genomewide pharmacogenetics of bisphosphonate-induced osteonecrosis of the jaw: the role of RBMS3. Oncologist. (2012) 17:279–87. doi: 10.1634/theoncologist.2011-0202
29. Kim, JW, Y, J, Oh, SH, Kim, SH, Kim, SJ, Chung, JE, et al. Machine learning approaches for predicting bisphosphonate-related osteonecrosis in women with osteoporosis using VEGFA gene polymorphisms. J Pers Med. (2021) 11:541. doi: 10.3390/jpm11060541
30. Billings, L, Hsu, K, Ackerman, RJ, Dupuis, J, Voight, BF, Rasmussen-Torvik, LJ, et al. Impact of common variation in bone-related genes on type 2 diabetes and related traits. Diabetes. (2012) 61:2176–86. doi: 10.2337/db11-1515
31. Karsono, R, Haryono, SJ, Karsono, B, Harahap, WA, Pratiwi, Y, and Aryandono, T. ESR1 PvuII polymorphism: from risk factor to prognostic and predictive factor of the success of primary systemic therapy in advanced breast cancer. BMC Cancer. (2021) 21:1348–55. doi: 10.1186/s12885-021-09083-x
32. Kurt, O, Yilmaz-Aydogan, H, Uyar, M, Isbir, T, Seyhan, MF, and Can, A. Evaluation of ERα and VDR gene polymorphisms in relation to bone mineral density in Turkish postmenopausal women. Mol Biol Rep. (2012) 39:6723–30. doi: 10.1007/s11033-012-1496-0
33. Barrett, JC, Fry, B, Maller, J, and Daly, MJ. Haploview: analysis and visualization of LD and haplotype maps. Bioinformatics. (2005) 21:263–5. doi: 10.1093/bioinformatics/bth457
34. Gabriel, SB, Schaffner, SF, Nguyen, H, Moore, JM, Roy, J, Blumenstiel, B, et al. The structure of haplotype blocks in the human genome. Science. (2002) 296:2225–9. doi: 10.1126/science.1069424
35. Cartegni, L, Wang, J, Zhu, Z, Zhang, MQ, and Krainer, AR. ESEfinder: a web resource to identify exonic splicing enhancers. Nucleic Acids Res. (2003) 31:3568–71. doi: 10.1093/nar/gkg616
36. Piva, F, Giulietti, M, Burini, AB, and Principato, G. SpliceAid 2: a database of human splicing factors expression data and RNA target motifs. Hum Mutat. (2012) 33:81–5. doi: 10.1002/humu.21609
37. Raponi, M, Kralovicova, J, Copson, E, Divina, P, Eccles, D, Johnson, P, et al. Prediction of single-nucleotide substitutions that result in exon skipping: identification of a splicing silencer in BRCA1 exon 6. Hum Mutat. (2011) 32:436–44. doi: 10.1002/humu.21458
38. Akerman, M, David-Eden, H, Pinter, RY, and Mandel-Gutfreund, Y. A computational approach for genome-wide mapping of splicing factor binding sites. Genome Biol. (2009) 10:R30. doi: 10.1186/gb-2009-10-3-r30
39. Uddin, S, Khan, A, Hossain, ME, and Moni, MA. Comparing different supervised machine learning algorithms for disease prediction. BMC Med Inform Decis Mak. (2019) 19:281. doi: 10.1186/s12911-019-1004-8
40. Lee, KH, Kim, SH, Kim, CH, Min, BJ, Kim, GJ, Lim, Y, et al. Identifying genetic variants underlying medication-induced osteonecrosis of the jaw in cancer and osteoporosis: a case control study. J Transl Med. (2019) 17, 17:381, 1–11. doi: 10.1186/s12967-019-2129-3
41. Allen, MR, and Burr, DB. Mandible matrix necrosis in beagle dogs after 3 years of daily oral bisphosphonate treatment. J Oral Maxillofac Surg. (2008) 66:987–94. doi: 10.1016/j.joms.2008.01.038
42. Su, J, Feng, M, Han, W, and Zhao, H. The effects of bisphosphonate on the remodeling of different irregular bones in mice. J Oral Pathol Med. (2015) 44:638–48. doi: 10.1111/jop.12281
43. Khosla, S, and Pacifici, R. “Estrogen deficiency, postmenopausal osteoporosis, and age-related bone loss,” in Osteoporosis. ed. R. Marcus (Amsterdam, Netherlands: Elsevier) (2013). 1113–36.
44. Ongphiphadhanakul, B, Chanprasertyothin, S, Payattikul, P, Saetung, S, Piaseu, N, Chailurkit, L, et al. Association of a G2014A transition in exon 8 of the estrogen receptor-alpha gene with postmenopausal osteoporosis. Osteoporos Int. (2001) 12:1015–9. doi: 10.1007/s001980170010
45. Riggs, BL. The mechanisms of estrogen regulation of bone resorption. J Clin Invest. (2000) 106:1203–4. doi: 10.1172/jci11468
46. Eertmans, F, Dhooge, W, De Wever, O, Bracke, M, Comhaire, F, and Kaufman, JM. Estrogen receptor alpha (ERalpha) and insulin-like growth factor I receptor (IGF-IR) cross-talk in the gonadotropic alphaT3-1 cell line. J Cell Physiol. (2007) 212:583–90. doi: 10.1002/jcp.21053
47. Kahlert, S, Nuedling, S, van Eickels, M, Vetter, H, Meyer, R, and Grohe, C. Estrogen receptor alpha rapidly activates the IGF-1 receptor pathway. J Biol Chem. (2000) 275:18447–53. doi: 10.1074/jbc.M910345199
48. Emmerson, E, Campbell, L, Davies, FC, Ross, NL, Ashcroft, GS, Krust, A, et al. Insulin-like growth factor-1 promotes wound healing in estrogen-deprived mice: new insights into cutaneous IGF-1R/ERα cross talk. J Invest Dermatol. (2012) 132:2838–48. doi: 10.1038/jid.2012.228
49. Lamon-Fava, S, Asztalos, BF, Howard, TD, Reboussin, DM, Horvath, KV, Schaefer, EJ, et al. Association of polymorphisms in genes involved in lipoprotein metabolism with plasma concentrations of remnant lipoproteins and HDL subpopulations before and after hormone therapy in postmenopausal women. Clin Endocrinol. (2010) 72:169–75. doi: 10.1111/j.1365-2265.2009.03644.x
50. Rossouw, J, Bray, P, Liu, J, Kooperberg, C, Hsia, J, Lewis, C, et al. Estrogen receptor polymorphisms and the vascular effects of hormone therapy. Arterioscler Thromb Vasc Biol. (2011) 31:464–9. doi: 10.1161/atvbaha.110.215087
51. Kitamura, I, Ando, F, Koda, M, Okura, T, and Shimokata, H. Effects of the interaction between lean tissue mass and estrogen receptor alpha gene polymorphism on bone mineral density in middle-aged and elderly Japanese. Bone. (2007) 40:1623–9. doi: 10.1016/j.bone.2007.02.016
52. Wang, C, Zhang, Z, Zhang, H, He, JW, Gu, JM, Hu, WW, et al. Susceptibility genes for osteoporotic fracture in postmenopausal Chinese women. J Bone Miner Res. (2012) 27:2582–91. doi: 10.1002/jbmr.1711
53. Choe, EK, Rhee, H, Lee, S, Shin, E, Oh, SW, Lee, JE, et al. Metabolic syndrome prediction using machine learning models with genetic and clinical information from a nonobese healthy population. Genom Inform. (2018) 16:e31–8. doi: 10.5808/GI.2018
54. Kautzky, A, Baldinger-Melich, P, Kranz, GS, Vanicek, T, Souery, D, Montgomery, S, et al. A new prediction model for evaluating treatment-resistant depression. J Clin Psychiatry. (2017) 78:215–22. doi: 10.4088/JCP.15m10381
55. Yang, Y, Hou, X, Ge, W, Wang, X, Xu, Y, Chen, W, et al. Machine-learning models utilizing CYP3A4*1G show improved prediction of hypoglycemic medication in type 2 diabetes. Per Med. (2022) 2022:59. doi: 10.2217/pme-2022-0059
56. Pittarabanjird, T, Cress, C, Nguyen, A, Taylor, A, Bekiranov, S, and McNamara, C. A machine learning model utilizing a novel SNP shows enhanced prediction of coronary artery disease severity. Genes (Basel). (2020) 11:1446–60. doi: 10.3390/genes11121446
57. Tibshirani, R. Regression shrinkage and selection via the lasso. J R Stat Soc B. (1996) 58:267–88. doi: 10.1111/j.2517-6161.1996.tb02080.x
59. Zhang, F, Petersen, M, Johnson, L, Hall, J, and O'Bryant, SE. Accelerating Hyperparameter tuning in machine learning for Alzheimer's disease with high performance computing. Front Artif Intell. (2021) 4:798962. doi: 10.3389/frai.2021.798962
Keywords: genetic polymorphism, ESR1, medication-related osteonecrosis of the jaw, MRONJ, machine learning, risk score
Citation: Choi S-Y, Kim J-W, Oh S-H, Cheon S, Yee J, Kim S-J, Gwak HS and Chung J-E (2023) Prediction of medication-related osteonecrosis of the jaws using machine learning methods from estrogen receptor 1 polymorphisms and clinical information. Front. Med. 10:1140620. doi: 10.3389/fmed.2023.1140620
Edited by:
Yue He, Shanghai Jiao Tong University, ChinaReviewed by:
Federica Di Spirito, University of Salerno, ItalyRiham Fliefel, Ludwig Maximilian University of Munich, Germany
Copyright © 2023 Choi, Kim, Oh, Cheon, Yee, Kim, Gwak and Chung. This is an open-access article distributed under the terms of the Creative Commons Attribution License (CC BY). The use, distribution or reproduction in other forums is permitted, provided the original author(s) and the copyright owner(s) are credited and that the original publication in this journal is cited, in accordance with accepted academic practice. No use, distribution or reproduction is permitted which does not comply with these terms.
*Correspondence: Jee-Eun Chung, jechung@hanyang.ac.kr
†These authors have contributed equally to this work