- 1Department of Psychosomatic Medicine and Psychotherapy, University Hospital of Ulm, Ulm, Germany
- 2Department of Anaesthesiology and Intensive Care Medicine, University Hospital of Ulm, Ulm, Germany
Background: In the clinical context, the assessment of pain in patients with inadequate communication skills is standardly performed externally by trained medical staff. Automated pain recognition (APR) could make a significant contribution here. Hereby, pain responses are captured using mainly video cams and biosignal sensors. Primary, the automated monitoring of pain during the onset of analgesic sedation has the highest relevance in intensive care medicine. In this context, facial electromyography (EMG) represents an alternative to recording facial expressions via video in terms of data security. In the present study, specific physiological signals were analyzed to determine, whether a distinction can be made between pre-and post-analgesic administration in a postoperative setting. Explicitly, the significance of the facial EMG regarding the operationalization of the effect of analgesia was tested.
Methods: N = 38 patients scheduled for surgical intervention where prospectively recruited. After the procedure the patients were transferred to intermediate care. Biosignals were recorded and all doses of analgesic sedations were carefully documented until they were transferred back to the general ward.
Results: Almost every biosignal feature is able to distinguish significantly between ‘before’ and ‘after’ pain medication. We found the highest effect sizes (r = 0.56) for the facial EMG.
Conclusion: The results of the present study, findings from research based on the BioVid and X-ITE pain datasets, staff and patient acceptance indicate that it would now be appropriate to develop an APR prototype.
1. Introduction
In the clinical context, the assessment of pain in patients with inadequate communication skills is still standardly performed externally by trained medical staff. This is usually done with external observation scales and is limited to and valid only at the time of assessment. Fine-grained continuous documentation is not possible given the lack of personnel time. Essentially, observational scales rely on a classification of pain components (1) that are used to assign pain-associated stress. Such indirect pain indicators are also referred to as surrogate markers. Mainly, surrogate markers such as facial expressions, vocalization, posture, and respiration are numerically assessed to determine a total pain score. Especially, the behavioral pain scale (BPS) is used in intensive care units when patients are unresponsive (2). All external pain assessment observation scales are only meaningful to a limited extent. In addition, a subjective coating by the observer is unconsciously included in the assessment. Automated pain recognition (APR) could make a significant contribution here. It is a visionary means to exploit valid and robust pain response patterns that can be measured multimodally (= multiple signals) for a dynamic, high temporal resolution, objective automated pain recognition system. The idea and development of an APR was primarily driven by the awareness that patients with impaired communication skills are at high risk of over-or underuse of pain-relieving analgesics. In recent years, machine learning algorithms related to APR have continuously emerged and evolved with the intention of further exploring pain configuration. The validation of these algorithms was always performed on healthy volunteers and pain patients. In this respect, APR actually represents an external objective observation method in which artificial intelligence is combined with hardware and software components with the goal of robustly and validly detecting pain. Werner et al. (3) and Frisch et al. (4) provide an excellent overview of the development status of the APR methodology for this purpose. The current focus of APR is on the detection of pain and its distinction between the classes “no pain” vs. “pain threshold” vs. “pain tolerance.” In this context, pain responses are explicitly recorded using video (for facial expressions (5, 6) and gestures (7–9)), microphones (for paralinguistics (10, 11)), and biosignal sensors which capture physiological activity, such as electrodermal activity [EDA], skin temperature, muscle activity [= electromyography, EMG], cardiac activity [= electrocardiogram, ECG] (12–16). The first commercial prototypes used only a single sensor for a rudimentary pain detection (17). In contrast, Medasense1 designed a device that records EDA, heart rate variability, and temperature synchronously for use during surgical procedures. There are preliminary empirical findings on postoperative use. Several studies showed that pain recognition rates were significantly higher (18) when different modalities were synchronously merged (19) than rather using a single signal/modality.
Explicitly, checking pain intensity during the onset of analgesic sedation is of utmost relevance in intensive care medicine to avoid over-or underdosing. Therefore, an automated monitoring of pain could be of highest relevance. The basics for such a method have not been sufficiently investigated so far.
Nevertheless, the recording of facial expressions, gestures, and paralinguistics is critical for ethical reasons and data security. In this context, EMGs of the facial muscles zygomaticus major and corrugator supercilii represent an alternative to recording facial expressions via video. They can serve as a substitute by appropriate interpretation of their activities. In general, very few studies have investigated the applications of facial EMGs (20, 21).
The following research questions are the focus of this brief report:
In the present study, the activities of specific physiological signals (facial EMG, ECG, skin conductance level (SCL) and Temperature (TEMP)) were analyzed to determine, whether a substantial distinction can be made between pre-and post-analgesic administration in a postoperative setting. Explicitly, the significance of the facial EMG in operationalization the effect of analgesia was investigated.
2. Methods
2.1. Patients
Patients scheduled for a surgical intervention within the next 3 days with the necessity of post-monitoring in an intermediate care (ICM) unit were prospectively enrolled in the study after completion of a written, informed consent. They had to be at least 18 years old, oriented and capable of providing information. Exclusion criteria were pregnancy, neurological diseases or having a pacemaker or defibrillator. All procedures were carried out in the ICM (35 beds) at the University Medical Center of Ulm, Germany. From September 29th, 2020 to April 13th, 2021, N = 38 (men = 28, women = 10) patients were included in the study. The ages ranged from 36 to 81 years, mean age was 62.3 years. Unfortunately, due to COVID-19 restrictions, the target sample size of 50 could not been reached.
2.2. Procedures and morphine equivalents
After the surgical procedure, the patients were transferred to intermediate care. There, after attaching electrodes to them to acquire and monitor biosignals relevant for the study, recording was started immediately and continued continuously throughout the patient’s stay in the ICM (see Figure 1). All patients remained under constant observation by 2 medical doctoral candidates until they were able to be transferred back to the general ward. During this time, all pain medications were meticulously documented and manually marked in the recordings. The main analgesics administered were tramadol, tilidine, piritramide, oxycodone, and remifentanil. To make the different drugs comparable, a conversion to their morphine equivalents was performed based on (22, 23) as follows: 1 mg morphine ≅ 0.1 mg tramadol/tilidine ≅ 0.7 mg piritramide ≅ 2 mg oxycodone ≅ 100 mg remifentanil/fentanyl ≅ 1,000 mg sufentanil. The average time the patients spent in intermediate care was about 22.8 h, the average number of analgesic administrations was 10.4 with an average dose of 5.45 mg (converted to morphine equivalents).
2.3. Recorded biosignals
Physiological signals were recorded at a sample rate of 1,024 Hz, using the NeXus-10-amplifier and its associated software “BioTrace+ NX10” from Mind Media.2 All biosensors were applied according to the instructions in Gruss et al. (24). Bipolar pairs of Ag/AgCl electrodes were placed over the right corrugator supercilii and right zygomaticus major muscles to measure the Electromyography of the face. To record the ECG, three Ag/AgCl electrodes were attached to the patients’ upper body. Two more electrodes were attached on the bottom edge of the left hand to capture the SCL. At last, to obtain the peripheral body temperature, a temperature (TEMP) sensor was fixed to the tip of the left little finger with a medical tape. The software offered the possibility of manually setting markers during recording. Each time a pain medication was administered (see 2.2), a corresponding marker was set to mark the exact time in the biosignal recordings.
2.4. Biosignal processing and feature extraction
The processing of all physiological signals and subsequent feature extraction was done utilizing the “MATLAB 2018b” software from MathWorks.3 Firstly, all biosignals were downsampled to 512 Hz to speed up the processing procedures. In a next step, each type of biosignal was individually filtered as follows:
TEMP & ECG: We applied a moving average to both of the signals to smoothen their data. For the temperature we chose a 513 data-points and for the ECG a 67 data-points sliding window. In addition, all ECG signals were detrended.
SCL: The signal was filtered with a 20 Hz-low-pass filter and also smoothened with a 1,025 data-points sliding window.
EMG: The 2 EMG channels were processed with a 3rd-order Butterworth bandpass filter with cut-off frequencies of 20 and 250 Hz. Next, a Hilbert-transformation was performed and absolute values of the resulted signal were obtained. Finally, the data were low-pass filtered with a cut-off frequency of 4 Hz (25).
For statistical calculations, we extracted features for all biosignals from relevant data windows. The windows had a length of 60 s and were cut out of the signals directly ‘before’ and 5 min ‘after’ pain medication if possible. Those analgesic administrations had been manually marked in the biosignal recordings (see 2.3). All windows were visually inspected for artifacts and outliers. If necessary and doable, corrupted segments were corrected, otherwise the data window was discarded. Finally, features were derived that differed in number and type depending on the biosignal. With future machine learning in mind, we chose relevant features for biosignals suggested by Gruss et al. (16) and Werner et al. (3). To calculate the only ECG feature, we additionally used the Pan-Tompkins QRS-algorithm to detect R-peaks in the signal (26). They were grouped according to their corresponding windows (‘before’ and ‘after’ pain medication). All specific features are shown in Table 1.
2.5. Statistical Analysis
For all statistical tests we used the software “SPSS Statistics 28”.4 We performed non-parametric comparisons between the groups ‘before’ and ‘after’ for all biosignals and their associated features. With regard to the repetition of measurements, a Wilcoxon signed-rank test for dependent samples was chosen. A p-value of <0.05 indicated statistical significance. In addition, for each p-value, we calculatedthe associated effect size r using the formula r =, Z = teststatistic, N = number of samples. Effect size measures help to assess the practical relevance of the results of statistical tests. Cohen’s classification (27) was used to assess the size of the effect: 0.1 ≤ r < 0.3 = small, 0.3 ≤ r < 0.5 = medium, and r ≥ 0.5 = large effect. Additionally, we were interested in whether the activities of both facial muscles were related to each other. In this sense, we calculated the differences of the related ‘before’ and ‘after’ values for all features for both muscles if both EMG signals were usable within the same window. The differences represented the change in activity and were used for non-parametric correlation analyses of the corrugator and the associated zygomaticus activities. Spearman’s ρ (= rho) and associated p-values were used to assess the association: 0.1 ≤ |ρ| < 0.3 = small, 0.3 ≤ |ρ| < 0.5 = moderate, and |ρ| ≥ 0.5 = strong correlation (28).
3. Results
After discarding unusable data windows, at least more than 330 samples per biosignal were left to examine statistically significant differences (NEMG_corrugator = 349, NEMG_zygomaticus = 344, NSCL = 331, NTEMP = 350, NECG = 349). A sample always consisted of a ‘before’ event and its related ‘after’ event. For the correlation analyses, there were a total of 310 samples, since both facial EMGs within the same sample always had to be qualitatively usable. Table 2 shows the results of the Wilcoxon tests for the comparisons of ‘before’ and ‘after’ groups for each biosignal feature.
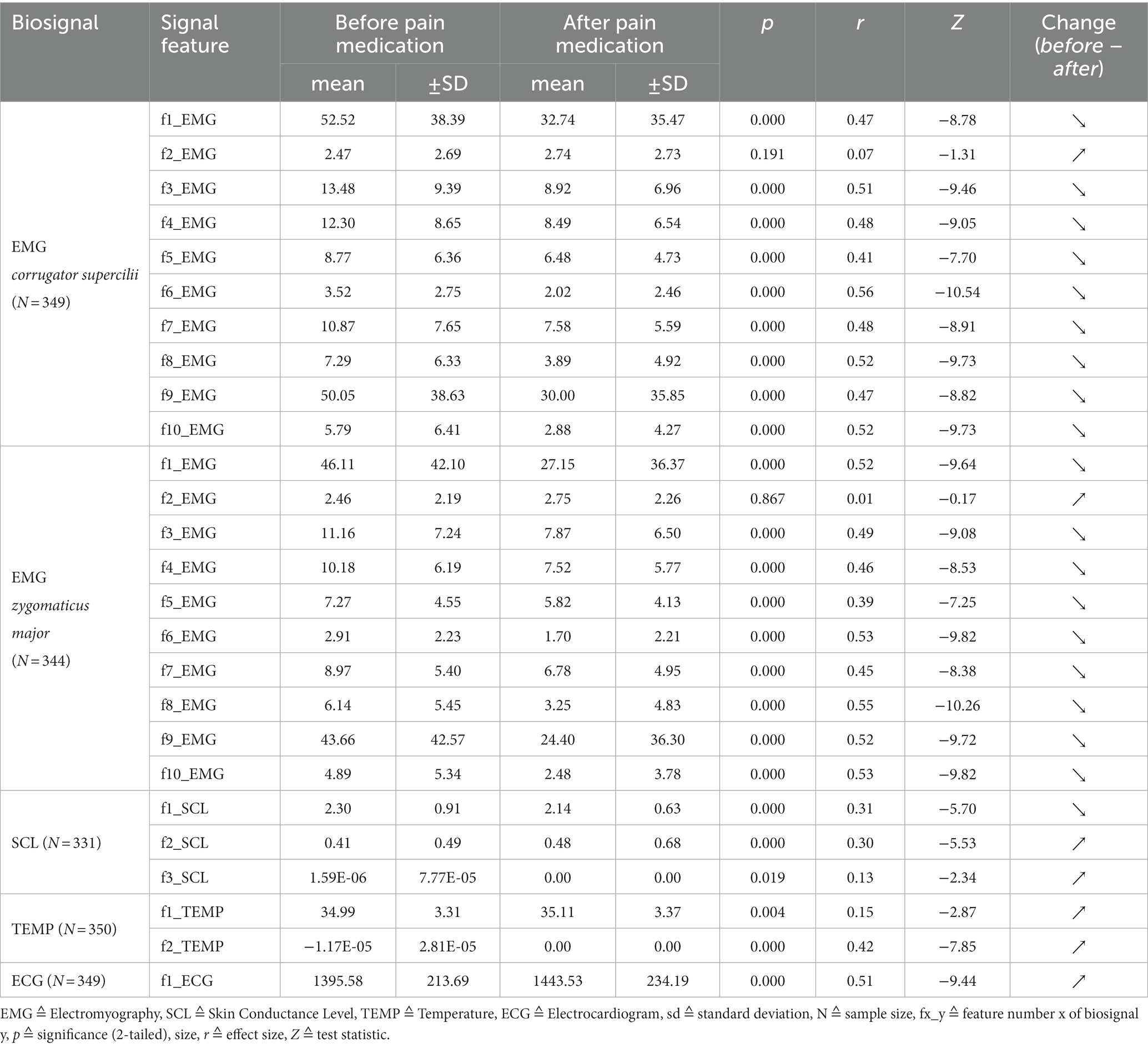
Table 2. Biosignal activity represented through features directly ‘before’ and 5 min ‘after’ pain medication: results of Wilcoxon signed-rank tests and associated effect sizes.
Almost every feature is able to distinguish significantly between the groups. The p-values hereby range from 0.019 to 0.000 with medium (r ≥ 0.3) to large (r ≥ 0.5) effect sizes. The largest effect size within this study was found for feature “f6_EMG” of EMG_corrugator_supercilii with r = 0.56 (p = 0.000). Features “f3_SCL” (p = 0.019, r = 0.13) and “f1_TEMP” (p = 0.004, r = 0.15) show high significant differences but have small effect sizes. No differences between the groups were found for features “f2_EMG” of EMG_corrugator_supercilii (p = 0.191) and “f2_EMG” of EMG_zygomaticus_major (p = 0.867). Except for the two features mentioned above, all biosignal activity changed to the expected directions. Muscle and SCL activity turned down, peripheral body temperature slightly increased and heart beat slowed down. All findings strongly indicate a reduced sympathicus activity after pain medication.
Regarding a related activity of the two facial muscles, Table 3 shows that almost all correlation coefficients reveal highly significant moderate to strong correlations, ranging from ρ = 0.383** to 0.634**. Only “diff_f2_C/Z” shows a weak correlation (ρ = 0.158**). All in all, the activities of the two facial muscles do not seem to be independent of each other.
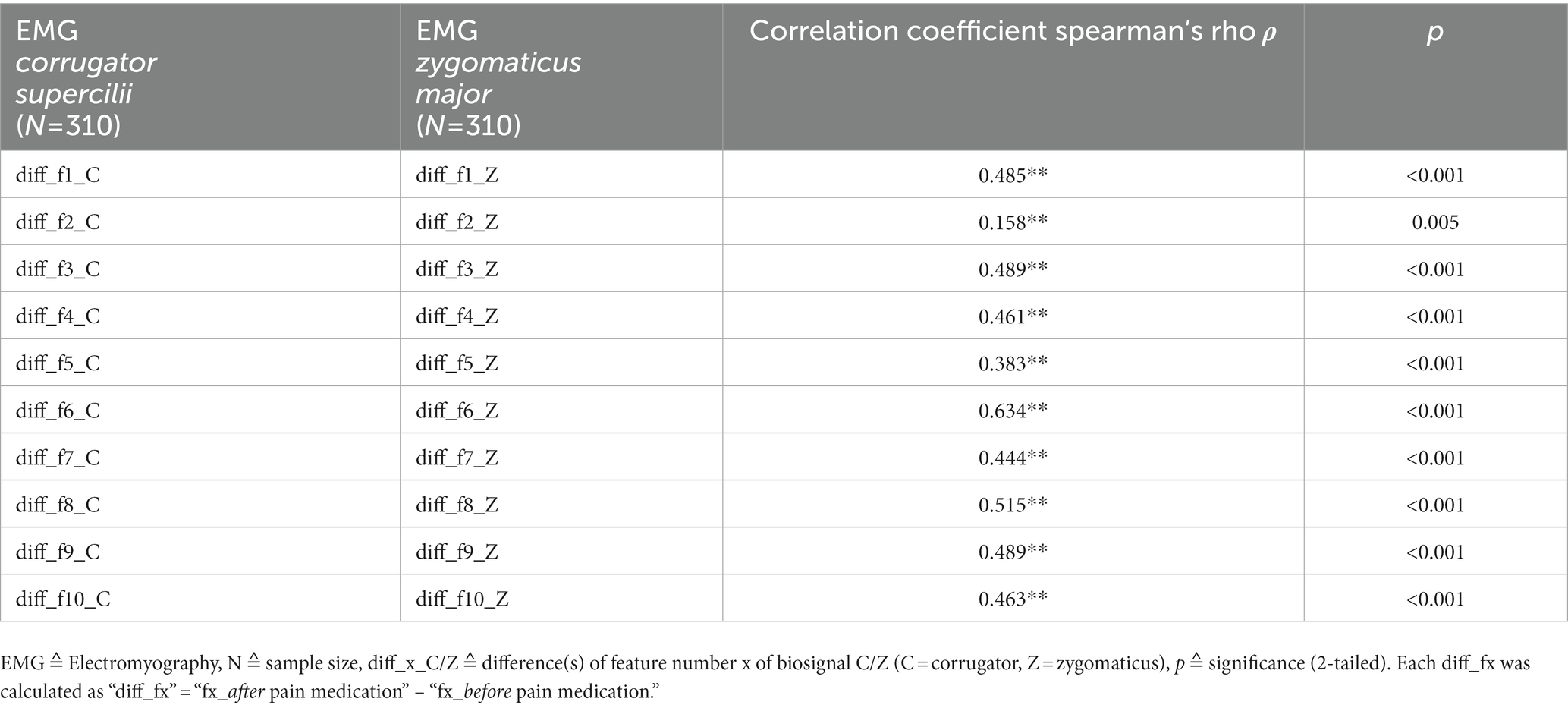
Table 3. Results of the non-parametric correlation analyses between EMG_corrugator_supercilii and EMG_zygomaticus_major.
4. Discussion
The fact that analgesia can be directly observed and assessed in a postoperative setting, e.g., with the BPS, is part of the S3-guideline “Analgesia, Sedation and Delirium management in Intensive Care.” That pain intensity has an influence on the autonomic nervous system has been shown in numerous studies (see introduction). However, it has not been operationalized to what extent the activities of biosignals are influenced after an analgesic administration. In this brief report the operationalization of an analgesic effect was proven statistically significant for the recorded biosignals EMG, SCL, ECG, and TEMP. This is an indication that physiological signals are suitable to check for an analgesic effect and should be transferred into an AI-based prototype. This would be a key benefit for clinical staff.
Walter et al. (29) showed that the majority of clinical staff in the ICU would prefer a biosignal-based pain detection. In relation to this, facial EMG would have the benefit of replacing computer vision, in terms of cost effectiveness and data security. For clinical staff, the use of computer vision systems would be more complex, more demanding, and ultimately more error-prone as a result.
Despite initial skepticism of the authors regarding the (patient) acceptability of the facial EMG sensors, the continuous measurement of facial EMG was shown to be unproblematic.
Limitations:
1. The sample is relatively small due to the complexity and duration of measurements and Covid-19 pandemic restrictions.
2. The extent to which a particular analgesic dosage affected the activities of the various biosignals was not investigated.
3. Why feature “f2_EMG” (= minimum of EMG signal) does not show a significant difference, cannot be verified at the moment.
Outlook:
1. Further studies should statistically examine the influence of a certain dosage of analgesics on the sympathetic/parasympathetic response.
2. Findings from research based on the BioVid (30) & X-ITE (24) datasets, the results of the present study, staff acceptance (29), and patient acceptance indicate that it would now be appropriate to develop and test an APR prototype.
Data availability statement
The datasets presented in this article are not readily available because of data security. Requests to access the datasets should be directed to c2FzY2hhLmdydXNzQHVuaS11bG0uZGU=.
Ethics statement
The studies involving human participants were reviewed and approved by Ethics committee of the Ulm University (No. 441/19). The patients/participants provided their written informed consent to participate in this study.
Author contributions
SG: study design, literature search, data analysis, and writing. BS: critical revision and writing. MS and LH: data collection. EB: study design, critical revision, and data interpretation. SW: study design, literature search, and writing. All authors contributed to the article and approved the submitted version.
Conflict of interest
The authors declare that the research was conducted in the absence of any commercial or financial relationships that could be construed as a potential conflict of interest.
Publisher’s note
All claims expressed in this article are solely those of the authors and do not necessarily represent those of their affiliated organizations, or those of the publisher, the editors and the reviewers. Any product that may be evaluated in this article, or claim that may be made by its manufacturer, is not guaranteed or endorsed by the publisher.
Abbreviations
Ag/Cl, silver chloride; APR, Automated Pain Recognition; COVID-19, Coronavirus Disease 2019; EMG, Electromyography; ECG, Electrocardiogram; Hz, hertz; ICM, Intermediate Care; SCL, Skin Conductance Level; TEMP, Temperature.
Footnotes
References
1. Gallacchi, G, and Pilger, B. Schmerzkomponenten. 2., neu be. Schmerzkompendium. Stuttgart: Georg Thieme Verlag (2005).
2. Payen, JF, Bru, O, Bosson, JL, Lagrasta, A, Novel, E, Deschaux, I, et al. Assessing pain in critically ill sedated patients by using a behavioral pain scale. Crit Care Med. (2001) [cited 2015 May 6]) 29:2258–63. doi: 10.1097/00003246-200112000-00004
3. Werner, P, Lopez-Martinez, D, Walter, S, Al-Hamadi, A, Gruss, S, and Picard, R. Automatic recognition methods supporting pain assessmentL: a survey. IEEE Trans Affect Comput. (2019) 13:530–52.
4. Frisch, S, Werner, P, Al-Hamadi, A, Traue, HC, Gruss, S, and Walter, S. From external assessment of pain to automated multimodal measurement of pain intensity: narrative review of state of research and clinical perspectives. Schmerz. (2020) 34:376–87. doi: 10.1007/s00482-020-00473-x
5. Werner, P, Al-Hamadi, A, Limbrecht-Ecklundt, K, Walter, S, Gruss, S, and Traue, HC. Automatic pain assessment with facial activity descriptors. IEEE Trans Affect Comput. (2017) 8:286–99. doi: 10.1109/TAFFC.2016.2537327
6. Sikka, K, Dhall, A, and Bartlett, MS. Classification and weakly supervised pain localization using multiple segment representation. Image Vis Comput. (2014) 32:659–70. doi: 10.1016/j.imavis.2014.02.008
7. Olugbade, T, Bianchi-Berthouze, N, Marquardt, N, and Williams, A. “Pain level recognition using kinematics and muscle activity for physical rehabilitation in chronic pain,” in 2015 International Conference on Affective Computing and Intelligent Interaction (ACII). IEEE. (2015). 243–249.
8. Newbold, JW, Bianchi-berthouze, N, Tajadura-jiménez, A, and Williams, CCA. “Musically Informed Sonification for Chronic Pain Rehabilitation: Facilitating Progress & Avoiding Musically Informed Sonification for Chronic Pain Rehabilitation: Facilitating Progress & Avoiding Over-Doing,” in Proceedings of the 2016 CHI Conference on Human Factors in Computing Systems. San Jose California USA: (2016). 5698–5703.
9. Singh, A, Bianchi-berthouze, N, and Williams, AC. “Supporting Everyday Function in Chronic Pain Using Wearable Technology,” in Proceedings of the 2017 CHI Conference on human factors in computing systems. Denver, Colorado USA; (2017). p. 3903–3915.
10. Thiam, P, Kessler, V, Amirian, M, Bellmann, P, Zhang, Y, Velana, M, et al. Multi-modal pain intensity recognition based on the SenseEmotion database. IEEE Trans Affect Comput. (2019) 12:743–60. doi: 10.1109/TAFFC.2019.2892090
11. Werner, P, Al-hamadi, A, Werner, P, De, AA, Gruss, S, and Walter, S. Twofold-multimodal pain recognition with the X-ITE pain database. 2019 8th Int Conf Affect Comput Intell Interact Work Demos. (2019);290–296.
12. Dowling, J. Autonomic indices and reactive pain reports on the McGill pain questionnaire. Pain. (1982) 14:387–92. doi: 10.1016/0304-3959(82)90146-4
13. Chapman, CR, Nakamura, Y, Donaldson, GW, Jacobson, RC, Bradshaw, DH, Flores, L, et al. Sensory and affective dimensions of phasic pain are indistinguishable in the self-report and psychophysiology of normal laboratory subjects. J Pain. (2001) 2:279–94. doi: 10.1054/jpai.2001.25529
14. Loggia, ML, Juneau, M, and Bushnell, MC. Autonomic responses to heat pain: heart rate, skin conductance, and their relation to verbal ratings and stimulus intensity. Pain. (2011) 152:592–8. doi: 10.1016/j.pain.2010.11.032
15. Treister, R, Kliger, M, Zuckerman, G, Aryeh, IG, and Eisenberg, E. Differentiating between heat pain intensities: the combined effect of multiple autonomic parameters. Pain. (2012) 153:1807–14. doi: 10.1016/j.pain.2012.04.008
16. Gruss, S, Treister, R, Werner, P, Traue, HC, Crawcour, S, Andrade, A, et al. Pain intensity recognition rates via biopotential feature patterns with support vector machines. PLoS One. (2015) 10:e0140330. doi: 10.1371/journal.pone.0140330
17. Ledowski, T, Ang, B, Schmarbeck, T, and Rhodes, J. Monitoring of sympathetic tone to assess postoperative pain: skin conductance vs surgical stress index. Anaesthesia. (2009) 64:727–31. doi: 10.1111/j.1365-2044.2008.05834.x
18. Walter, S, Gruss, S, Traue, H, Werner, P, Al-Hamadi, A, Kachele, M, et al. Data fusion for automated pain recognition. In: Proceedings of the 2015 9th International Conference on Pervasive Computing Technologies for Healthcare, PervasiveHealth (2015).
19. Walter, S, Al-Hamadi, A, Gruss, S, Frisch, S, Traue, HC, and Werner, P. Multimodal recognition of pain intensity and pain modality with machine learning. Schmerz. (2020) 34:400–9. doi: 10.1007/s00482-020-00468-8
20. Limbrecht-Ecklundt, K, Werner, P, Traue, HC, Al-Hamadi, A, and Walter, S. Mimische Aktivität differenzierter Schmerzintensitäten: Korrelation der Merkmale von Facial Action Coding System und Elektromyographie. Schmerz. (2016) 30:248–56. doi: 10.1007/s00482-016-0105-x
21. Hann, A, Walter, BM, Mehlhase, N, and Meining, A. Virtual reality in GI endoscopy: intuitive zoom for improving diagnostics and training. Gut. (2019) 68:957–9. doi: 10.1136/gutjnl-2018-317058
22. Artner, J, Hofbauer, H, and Steffen, P. Medikamente in der Schmerztherapie: Analgetika, Koanalgetika und Adjuvanzien von A-Z. 2nd ed. Berlin, Heidelberg: Springer. (2018).
23. Karow, T, and Lang-Roth, R. Pharmakologie und Toxikologie. 27th ed. Pulheim: Thomas Karow (2018).
24. Gruss, S, Geiger, M, Werner, P, Wilhelm, O, Al-Hamadi, A, and Walter, S. Multimodal signals for analyzing pain responses to thermal and electrical stimuli. JoVe. (2019):e59057. doi: 10.3791/59057
25. Andrade, AO, Kyberd, P, and Nasuto, SJ. The application of the Hilbert spectrum to the analysis of electromyographic signals. Inf Sci. (2008) 178:2176–93. doi: 10.1016/j.ins.2007.12.013
26. Hamilton, PS, and Tompkins, WJ. “Quantitative investigation of QRS detection rules using MIT/BIH arrhythmia database,” in IEEE Transactions on Biomedical Engineering. (1987):1157–1165.
27. Statistical, CJ, and Analysis, P. Statistical Power Analysis. Curr Dir Psychol Sci. (1992) 1:98–101. doi: 10.1111/1467-8721.ep10768783
28. Cohen, J. Statistical Power Analysis for the Behavioral Sciences. 2nd ed. Hillsdale, N.J: L. Erlbaum Associates (1988).
29. Walter, S, Gruss, S, Frisch, S, Liter, J, Jerg-Bretzke, L, Zujalovic, B, et al. “What about automated pain recognition for routine clinical use?” a survey of physicians and nursing staff on expectations, requirements and acceptance. Front Med. (2020) 7:566278. doi: 10.3389/fmed.2020.566278
Keywords: biosignals, morphine equivalents, pain medication, surrogate markers, features, automatic pain recognition
Citation: Gruss S, Schmid M, Walter S, Schick B, Holler L and Barth E (2023) The impact of analgesic on EMG and other biosignals in a postoperative setting. Front. Med. 10:1038154. doi: 10.3389/fmed.2023.1038154
Edited by:
Pedro Miguel Rodrigues, Escola Superior de Biotecnologia – Universidade Católica Portuguesa, PortugalReviewed by:
Jun-Wen Tan, Guangdong Medical University, ChinaLuis Pinto Coelho, Instituto Superior de Engenharia do Porto (ISEP), Portugal
Copyright © 2023 Gruss, Schmid, Walter, Schick, Holler and Barth. This is an open-access article distributed under the terms of the Creative Commons Attribution License (CC BY). The use, distribution or reproduction in other forums is permitted, provided the original author(s) and the copyright owner(s) are credited and that the original publication in this journal is cited, in accordance with accepted academic practice. No use, distribution or reproduction is permitted which does not comply with these terms.
*Correspondence: Sascha Gruss, c2FzY2hhLmdydXNzQHVuaS11bG0uZGU=