- 1Department of Family and Community Medicine, College of Medicine and Medical Sciences, Arabian Gulf University, Manama, Bahrain
- 2Department of Family Medicine, Faculty of Medicine, Helwan University, Helwan, Egypt
- 3Department of Microbiology, College of Medicine and Medical Sciences, Arabian Gulf University, Manama, Bahrain
- 4Department of Medical Education, College of Medicine and Medical Sciences, Arabian Gulf University, Manama, Bahrain
- 5Department of Medical Education, Faculty of Medicine, Suez Canal University, Ismailia, Egypt
Introduction: Admission to medical school is one of the most competitive entry points in higher education. Medical school admissions committees need accurate and precise screening tools to select among well-qualified applicants. This study explores data from a cohort of graduated medical students over 6 years to offer a critical perspective on predictive validity in medical school admissions.
Methods: A retrospective cohort study of 160 students was performed to identify the predictive validity of admission criteria for medical students to predict academic performance over 6 years for a cohort of all students enrolled in the medical program during the academic year 2013–2014.
Results: The main results indicated that there was a statistically significant positive correlation between the admission criteria and Students’ performance in Year 1, Year 4, B.Sc. (Bachelor of Medical Science) exam, and Medical Doctor (MD) exam across the 6 years of the medical program, except for the English Test, which showed that there was no significant correlation with average MD exam scores for students who enrolled directly in Year 1. The results related to students who were admitted to the Foundation Program showed that there was no significant correlation between high school Grade Point Average (HSGPA) and their academic performance in Year 1, Year 4, B.Sc. exam, and MD exam. The overall results related to all study samples indicated that all predictor variables correlate significantly with all outcome variables (academic performance), and the results showed that Science test scores demonstrated 27.7, 15.0, 19.7, and 12.6% of variation in Students’ performance in Year 1, Year 4, B.Sc. exam, and MD exam, respectively.
Conclusion: Science test scores were found to be more predictive of academic performance compared to other predictors. Not all the admission criteria used for student selection are good indicators of their achievement in the medical program. It is recommended that other valid and reliable admission tools, such as the multiple mini-interviews and the questionnaire for a candidate’s suitability to follow a problem-based learning curriculum, should be considered.
Introduction
The number of qualified applicants for medical degree programs sometimes outstrips the number of available seats, making the selection process very competitive. Thus, the selection process must be clear and defendable to balance the supply and demand reasonably and consistently. In addition, it is logical that medical schools strive to select students who may be more inclined to become excellent physicians (1).
The criteria for selecting medical students are debatable, and selection techniques for admission from the substantially bigger applicant pool constitute a significant difficulty (2). Selection techniques are essential to finding individuals with the appropriate aptitude to finish the program and become safe physicians. In fact, such methods must rate students for admittance to the available number of seats. However, establishing the extent to which a specific tool anticipates its predictive validity or outcome measures is most challenging. There are many reasons for this, including deciding the most accurate outcome to validate the tool against; uncertainty on how candidates who achieve lower scores would have fared (3), and how to combine those tools to improve their predictive validity (1, 4).
When it comes to medical school admissions, there is no shortage of information. More students are applying for admission, and admission officers are becoming more stringent in their selection process because of the increasing quantity and quality of potential candidates and the limited number of available seats. A number of studies have questioned the predictive efficacy of different pre-admission indicators on student academic achievement. Consequently, more testing and modification of pre-admission factors are needed to eliminate improper selection criteria that are weakly predictive of the future performance of medical graduates (5, 6).
College performance indicators are often used in predictive validity studies to examine pre-admission characteristics (7). Grade point average (GPA) is a common college performance metric because of its perceived importance in measuring Students’ in-course college achievement. Although the GPA is widely accepted as a credible and accurate indicator of academic performance (8, 9), medical schools have yet to corroborate this. Grade inflation and institutional inequalities in grading are among the serious flaws in this kind of performance metric that may have a negative impact on its validity and reliability (9). Admission examinations for colleges and universities have been the subject of much discussion and debate in the academic literature (10). Pre-university assessments such as high school GPA, aptitude, and achievement tests were studied to see whether they predicted Students’ college GPA, and high school GPA was proven to be a better predictor of college GPA than preparatory examinations (11). On the contrary, Al-Rukban et al. found that the high school grade had less predictive power when it came to Students’ college GPAs (12). Murshid conducted another intriguing investigation to determine the effectiveness of admission criteria in predicting Students’ academic performance (13). He discovered that high school grades and achievement tests were both strong predictors of college GPA. Furthermore, in a recent study conducted by Alamoudi et al. (14) on the relationship between admission criteria and academic performance in basic science courses in health science colleges in a Saudi university, no significant correlation was found between admission exam scores and Students’ academic achievement. Similar studies performed in the US revealed that the Medical College Admission Test (MCAT) was a good predictor of the performance of medical graduates in the first 2 years of college (15, 16). The same trend was seen in the clinical years, where the students who did well in the MCAT performed better in the clinical years and in the residency program (17, 18). Admission procedures and aptitude tests in medical education have been studied extensively for their predictive value. For up to 23% of the variance in medical school performance, a systematic review of more than 150 papers on the relationship between admissions variables and success in medical schools was conducted and discovered that past academic performance was a modest predictor of college success (1).
The goal of this study was to verify if the admission criteria at Arabian Gulf University (AGU) were predictive of Students’ academic achievement during a 6-year program. This is the first study at AGU to look at this topic.
Materials and methods
Study context
The College of Medicine and Medical Sciences, Arabian Gulf University (CMMS-AGU) in Bahrain is a regional institution that provides medical education for students from Gulf Cooperation Council (GCC) countries, namely Bahrain, Saudi Arabia, Kuwait, Oman, United Arab Emirates, and Qatar. Currently, around 1,200 students are enrolled in all study years. The medical program is almost completely delivered in English. Therefore, students who are admitted to the college are required to achieve excellent scores in an English language test prior to acceptance to make sure that they meet the required language skills. Candidates who fail to demonstrate adequate English language proficiency are offered a 1-year foundation program where they receive intensive English language instruction.
The curriculum of the CMMS-AGU follows an integrated, problem-based approach. The medical program is offered in three phases. Phase I of the program extends over the first year of the program and mainly introduces basic science courses (medical physics, biology, biochemistry, and biostatistics) in addition to other general courses, including Islam, medical ethics, English language, computer science, social science, and psychology. Phase II of the program—or the pre-clerkship phase—focuses on basic medical sciences. This phase extends from year 2 to year 4 of the program and is fully integrated and problem-based. Phase III of the program is the clinical phase, where students receive clerkship clinical training in several training sites. Students are awarded the Doctor of Medicine (MD) degree following successfully completing the final MD exam at the end of Phase III.
Study design, participants, and data collection
This is a retrospective cohort study conducted on 160 medical students who were enrolled in the CMMS-AGU in the academic year 2013–2014 and graduated in the academic year 2018–2019 to study the relationship between their performance during the study years and the admission criteria to determine the most predictive admission criteria that predict their performance (Table 1).
The collected data included Students’ demographics, pre-admission variables [high school grade point average (HSGPA), AGU-MCAT (biology, chemistry, physics, and mathematics) scores, and their English language test scores], and college academic performance indicators (Year 1 GPA, Year 4 GPA, average B.Sc. (Bachelor of Medical Science) exam scores, and average MD exam scores). AGU-MCAT (Science Test) and English language test are collectively called the “Entrance Exam.”
The Science Test covers basic topics in biology, chemistry, physics, and mathematics, which consists of a hundred A-type multiple-choice questions. Biology represents 40% of the exam questions, while chemistry, physics, and mathematics each represent 20%. Candidates are allowed a total of 3 h to complete the test.
The English language tests aim to assess the proficiency of students in English (reading, listening, and writing).
An overall total score is reported (100 points) for each test (Science test and English language test).
Multiple filters are used to screen candidates as follows: only students with an overall high-school score of at least 90% and an overall average score of at least pass in English Language Tests and Science Test are allowed to apply for year 1 or foundation year program. Candidates then undergo a semi-structured personal interview to evaluate their personality traits to determine the suitability of each candidate to study medicine and the extent to which they will be suited to the study program at the AGU, in which they are rated only as “pass” or “fail.” Candidates who pass the interview are then listed according to their balanced percentage (high school GPA and scores of Science tests and English language tests) in descending order, and those at the top of the list are admitted to the year 1 program, while others are admitted to the foundation year program depending on the available seats.
Predictor variables
In addition to the high school GPA, predictor variables included the Science test and English language tests.
Performance outcomes
These included the GPA of the final exams of year 1 (Year 1 GPA) and year 4 (Year 4 GPA), in addition to a comprehensive assessment of basic medical sciences after completion of the first 4 years of the program (average B.Sc. scores) and an exit assessment of all the clinical sciences studied during the clerkship phase (average MD scores).
Statistical analysis
The data were entered and analyzed using SPSS (Chicago, IL, United States) software version 28. Qualitative variables were presented as frequencies and percentages, and quantitative variables were presented as means and SD. Pearson correlation coefficients were used to test the relationship between the admission criteria (predictor variables) and academic performance (outcome variables). Multiple linear regression analysis was performed for each outcome variable with all variables included. Stepwise multiple linear regression analysis was used to verify the predictive value of admission criteria. Standard coefficients with 95% confidence intervals, SE, and R2-values (coefficient of determination) were tabulated. In addition, standardized coefficients (β-values) were presented to show the relative independent contribution of the predictor variables. The regression model was tested using partial least squares structural equation modeling (PLS-SEM) and using Smart PLS version 3.2.7. A p-value of less than 0.05 was considered statistically significant.
Ethical considerations
This research was approved by the Research and Ethics Committee of CMMS College of Medicine and Medical Sciences, AGU, Bahrain (Approval Number: E040-PI-2/21). The Research and Ethics Committee waived the need to obtain informed consent since this study used secondary data from the participants. All authors confirm that all methods were performed in accordance with the relevant guidelines and regulations.
Results
Demographic data
This cohort of students was enrolled at CMMS-AGU in the academic year 2013–2014. Out of 160 students, 105 (65.6%) were female students, and 55 (34.4%) were male students. Regarding the admission status, the majority of the students (69.4%) were accepted in Year 1 of medical school, while 49 students (30.6%) were accepted in the foundation program (Pre-Year 1). The enrolled students were Bahraini (41.3%), Kuwaiti (35%), Saudi (18.1%), and Omani (5.6%). The majority of the students (78.8%) came from governmental schools, while only 21.2% came from private schools (Table 1).
Descriptive statistics and correlation matrix of study variables
The Students’ high school grades and their entrance exam scores (which are used as admission criteria) along with Students’ performance in Year 1, Year 4, B.Sc. exam, and MD exam are presented as means and SD. Results show that although the mean high school grades were high, the mean grades of the entrance exams were low (less than 60%) with a large variation (19.32) in the English test scores compared to the Science test scores (11.36). Students’ performance at the end of Year 1 (81.94 ± 6.89) and Year 4 (77.66 ± 6.58) was better than in B.Sc. (71.24 ± 7.38) and MD (71.99 ± 6.60) exams, as the latter two are comprehensive exams (Table 2).
The correlation study indicated that all predictor variables correlate significantly with academic performance (Year 1 GPA, Year 4 GPA, average B.Sc. exam scores, and average MD exam scores). This is an indication of the validity of these tests to predict the performance of medical students in medical school. Science test scores showed the strongest correlation with all academic performance indicators, while HSGPA showed the lowest correlation with all academic performance indicators.
The correlation between the English test and high school GPA (HSGPA) was a weak negative, statistically insignificant correlation (-0.017). Also, the results showed that the correlation between Year 4 GPA and average B.Sc. exam scores was the highest (r = 0.941) compared to the correlations of the predictors and outcome variables. The strongest correlation between two predictor variables was between Science test scores and English test scores (r = 0.379), and the shared variance between these two variables was about 14.4% (R2 = 0.1436).
Relationship between predictor variables and academic performance
The correlation between admission exam scores and Students’ performance scores according to the admission type is presented in Table 3. For students who were ineligible to join Year 1 and were enrolled in an extra Pre-Year 1 foundation program, the results showed no statistically significant correlation between HSGPA and their academic performance in Year 1, Year 4, B.Sc. exam, and MD exam. In contrast, a moderate positive statistically significant correlation (ranging from r = 0.298 to r = 0.392) between HSGPA and academic performance in Year 1, Year 4, B.Sc. exam, and MD exam was noted for students who were enrolled directly in Year 1.
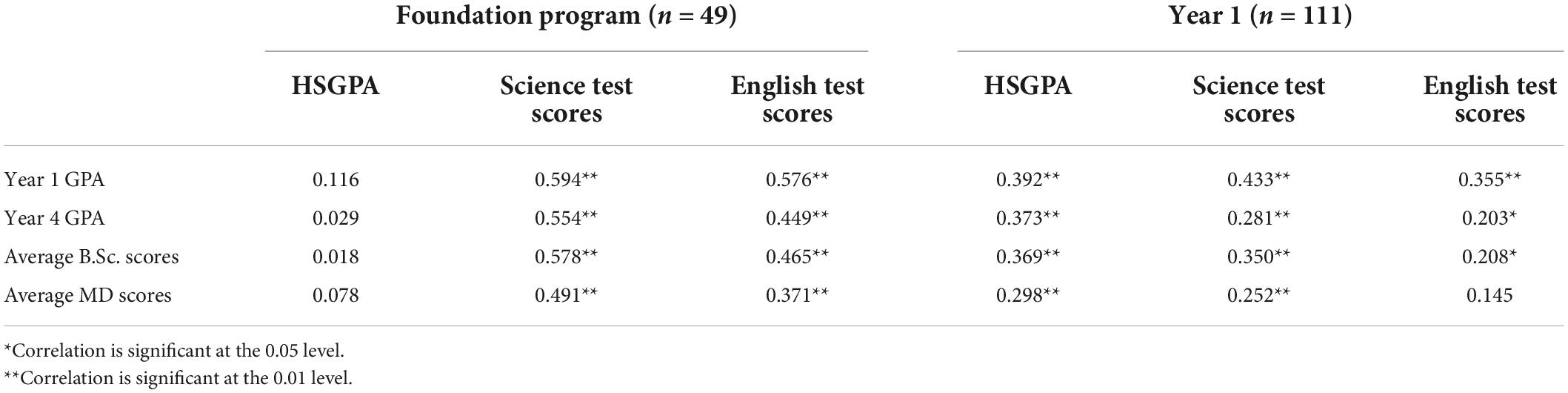
Table 3. Pearson correlation coefficients for predictor variables and academic performance (outcome indicators) according to admission types (foundation program and year 1 admission).
Positive, statistically significant correlations were found between English test scores and Science test scores on one hand, and Students’ performance in Year 1, Year 4, B.Sc. exam, and MD exam, on the other hand, for the students who went through the foundation program. This was found to be true also for those students who were directly enrolled in Year 1, except for a statistically insignificant positive correlation between English test scores and average MD exam scores. The correlation was consistently stronger for students who were enrolled in the foundation program than those for students who were directly enrolled in Year 1. Moreover, Science test scores have higher correlation coefficients than English test scores for both admission types.
Regression analysis
A multiple regression model was fitted to study the linear relationship between Students’ performance in Year 1, Year 4, B.Sc. exam, and MD exam on the one hand and their admission criteria scores (HSGPA, English test scores, and Science test scores) on the other hand.
Regression analysis indicated that there is a statistically significant linear relationship between Students’ performance and admission scores. The amounts of variation in Students’ performance in Year 1, Year 4, B.Sc. exam, and MD exam explained by the predictors (adjusted R2) was 39.5, 20.3, 24.5, and 15.9%, respectively.
The values of the coefficients β fell from Year 1 to MD but were consistently higher for Year 1 GPA than Year 4 GPA, average B.Sc. exam scores, and average MD exam scores for each admission criteria. The values of the coefficients β for Science test scores were consistently higher than those for HSGPA and English test scores; this indicates that the Science test is one of the most important admission criteria in explaining variation in academic performance compared to the other predictors (Table 4).
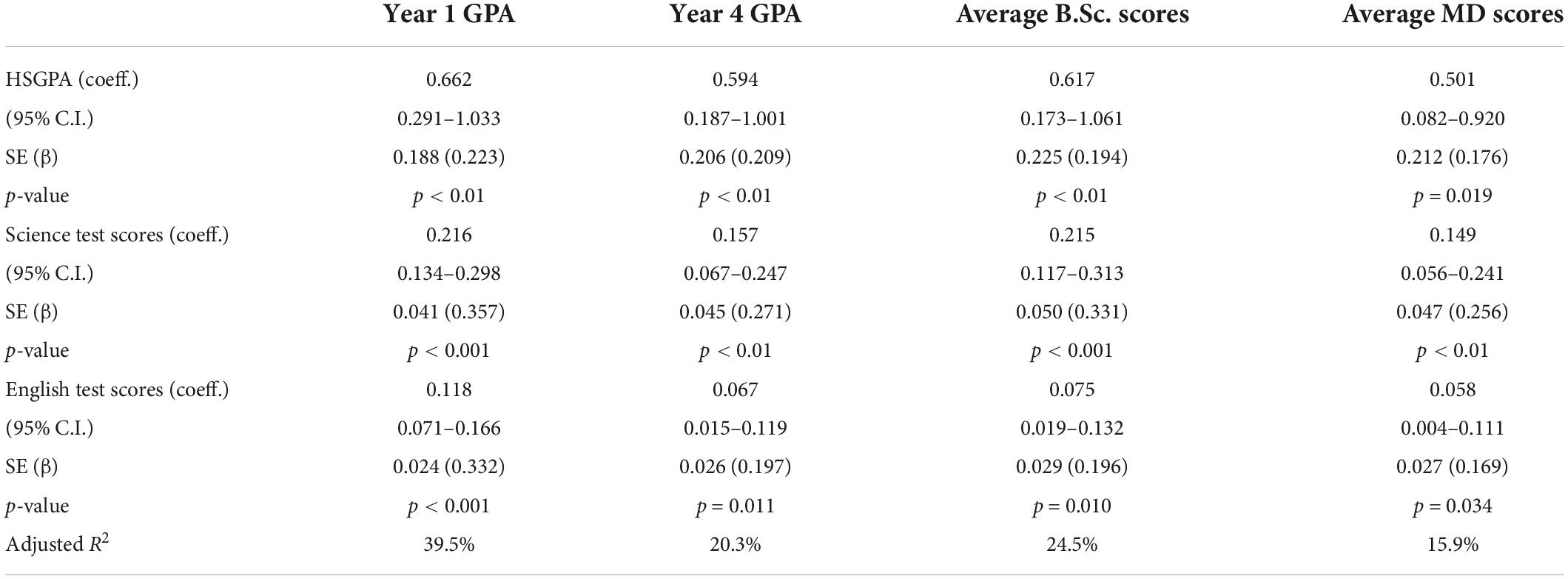
Table 4. Regression modeling of the relationship between admission criteria and academic performance.
Stepwise regression analysis
Stepwise multiple regression analysis was employed to identify and select the predictor variable that explains more variation in the academic performance indicator of medical students. Science test scores, English test scores, and HSGPA were entered into the regression model but in different steps (Table 5).
Year 1 grade point average
Science Test scores were entered first as predictors into model 1, which accounted for significant variation and explained 27.7% of the variation in Year 1 GPA. Thus, Science test scores were more important in explaining the variation in Year 1 GPA than English Test scores and HSGPA [R2 = 0.277, F(1, 158) = 60.652, p < 0.001]. When English test scores were added as a second predictor in model 2, the two variables (Science test and English test) accounted for significant variation in Year 1 GPA [R2 = 0.359, F(1, 158) = 43.911, p < 0.001], and the prediction of Year 1 GPA has improved by 8.2% (change in R2 = 0.082). When HSGPA was added as a third predictor in model 3, the prediction of Year 1 GPA has improved by 4.6% [change in R2 = 0.046, F(1, 158) = 35.545, p < 0.001].
Year 4 grade point average
Science test scores were entered first as a predictor into model 1, which accounted for significant variation and explained 15.0% of the variation in Year 4 GPA. Thus, Science test scores were more important in explaining the variation in Year 4 GPA than English Test scores and HSGPA [R2 = 0.150, F(1, 158) = 27.835, p < 0.001]. When HSGPA was added as a second predictor in model 2, the two variables (Science test and HSGPA) accounted for significant variation in Year 4 GPA [R2 = 0.185, F(1, 158) = 17.773, p < 0.001], and the prediction of Year 4 GPA has improved by 3.5% (change in R2 = 0.035). When English test scores were added as a third predictor in model 3, the prediction of Year 4 GPA has improved by 3.0% [change in R2 = 0.030, F(1, 158) = 14.463, p < 0.001].
Average bachelor of medical science exam scores
Science test scores were entered first as a predictor into model 1, which accounted for significant variation and explained 19.7% of the variation in the average B.Sc. exam scores. Thus, Science test scores were more important in explaining the variation in the average B.Sc. exam scores than English test scores and HSGPA [R2 = 0.197, F(1, 158) = 38.740, p < 0.001]. When HSGPA was added as a second predictor in model 2, the two variables (Science test and HSGPA) accounted for significant variation in the average B.Sc. exam scores [R2 = 0.226, F(1, 158) = 22.976, p < 0.001], and the prediction of the average B.Sc. exam scores has improved by 2.9% (change in R2 = 0.029). When English test scores were added as a third predictor in model 3, the prediction of average B.Sc. exam scores has improved by 3.1% [change in R2 = 0.031, F(1, 158) = 18.190, p < 0.001].
Average medical doctor exam scores
Science Test scores were entered first as a predictor into model 1, which accounted for significant variation and explained 12.6% of the variation in the average MD exam scores. Thus, Science test scores were more important in explaining the variation in the average MD exam scores than English test and HSGPA [R2 = 0.126, F(1, 158) = 22.764, p < 0.001]. When HSGPA was added as a second predictor in model 2, the two variables (Science test and HSGPA) accounted for significant variation in average MD exam scores [R2 = 0.151, F(1, 158) = 13.912, p < 0.001], and the prediction of the average MD exam scores has improved by 2.5% (change in R2 = 0.025). When English test scores were added as a third predictor in model 3, the prediction of the average MD exam scores has improved by 2.2% [change in R2 = 0.022, F(1, 158) = 11.007, p < 0.001].
Combined admission criteria
From the stepwise multiple regression analysis (Table 5), the three admission criteria (HSGPA, English test scores, and Science test scores) together explained 40.5, 21.5, 25.7, and 17.3% of the variation in student performance in Year 1 GPA, Year 4 GPA, B.Sc. exam, and MD exam, respectively (Figure 1).
Discussion
This study was conducted to verify the predictive validity of the admission criteria used in the CMMS-AGU. It explored the admission data (as predictor variables) and the performance of students in Year 1 final exam (represented by Year 1 GPA), Year 4 final exam (represented by Year 4 GPA), B.Sc. exam (a comprehensive assessment of basic medical sciences after completion of the first 4 years of the curriculum), and MD exam (an exit assessment of all the clinical sciences studied during the clerkship phase). Participating students in this study were both men and women who came from four Gulf countries.
This study found that there is a statistically significant correlation between most of the predictor variables and the performance indicators. The highest correlation was found to be with the scores of the Science test (part of the admission exam). Robi (19) found similar results, although they reported that HSGPA was superior to the entrance exam as a predictor of Students’ performance in medical school. The findings are partly consistent with a study by Mercer and Puddey (20), who found a highly statistically significant correlation between high school scores and the weighted average in the first 3 years of the medical program, and Alkushi and Althewini (21), who found that high school grades were the best predictor of college performance for women, while scholastic achievement admission test scores were a better predictor of college performance for men. This is also consistent with the findings of two studies (22, 23), which reported that high GPA in science courses in high school and admission tests (MCAT) were the best predictors of later success in medical school. In the same context, Simpson et al. (24) found that the university admission index (based mainly on high school grades) had a high value in predicting overall and knowledge-based outcomes of medical school. Moreover, recent studies found that medical school admission test scores were one of the strongest predictors of academic performance later on during school years (25, 26).
Students in this study were divided according to admission type based on their performance in admission exams (English and Science tests). Students who were ineligible for enrollment in Year 1 were directed to join a foundation program to prepare them to join Year 1 of the medical school. In contrast to the students who were enrolled directly in Year 1, the HSGPA of the students who were enrolled in the foundation program showed no correlation with their performance in medical school. This might mean that admission should not depend on the HSGPA of those students and also explains the necessity of the foundation program in the rectification of the linguistic and intellectual readiness of those students to join medical school.
Regarding the performance of foundation year students, the results indicated that there is no statistically significant relationship between the HSGPA and the academic performance of all levels of study (Year 1, Year 4, B.Sc., and MD). In contrast, the results indicated that there is a positive and statistically significant correlation between the foundation year student scores in each of the Science and English language admission tests and their academic performance for all levels of study. The results indicated that the values of the correlation coefficients came to a medium degree, and this can be justified by the fact that the foundation year students study intensive courses in mathematics and the English language, and this contributed to increasing their performance in the different academic years.
Comparing the predicting value of Science test scores and English test scores revealed that the first was more predictive of Students’ performance in medical school than the latter. Moreover, the Science test was found to be more important in explaining the variation in Students’ academic performance in medical school than the English test. This was found to be true for all academic performance indicators, which are Year 1 GPA, Year 4 GPA, average M.Sc. test scores, and average MD test scores. This can be explained by the fact that the content of such Science test is very similar to the basic medical sciences studied during the first 2 or 3 years of medical school. These findings are consistent with the study of Dunleavy et al. (27), who reported that the biological and physical sciences were the strongest predictors of academic performance in medical school through graduation. They are also consistent with a study by Wilkinson et al. (28), who reported a significant correlation between six admission measures (grouped together as a biomedical science domain consisting of a human biology course, biochemistry course, cellular biology course, chemistry course, physics course, and epidemiology and public health course) and performance in medical school.
The study results indicate that both the Science test and HSGPA could predict Students’ performance in Year 1. However, it should be noted that the Science test is more predictive compared to other predictors. Also, both the Science test and English test could predict Students’ performance at the different stages (Year 4, B.Sc., and MD).
Conclusion
The Science test results were found to be more predictive of academic performance compared to other predictors. Not all the admission criteria used for student selection are good predictors of Students’ academic performance in the medical program. The current admission criteria provide some insight into the predicted future academic performance of students. However, we believe that there is a pressing need to develop more sensitive admission criteria that can better predict student performance in both the pre-clinical and clinical years. Care should be taken to develop comprehensive admissions criteria, covering both cognitive and non-cognitive factors, to identify the best applicants to become good, future-ready doctors. Moreover, the inclusion of other valid and reliable admission tools, such as the multiple mini-interviews and the questionnaire for candidates’ suitability to follow a problem-based learning curriculum, is recommended. It would also be useful to conduct further studies to determine the predictive validity of new admission criteria. As a further research point, we recommend comparing the academic achievement of students with no foundation year with their peers who have foundation year to explore the value of such foundation year in predicting student performance in medical programs.
Data availability statement
The raw data supporting the conclusions of this article will be made available by the authors, without undue reservation.
Author contributions
AA and MS conceived and designed the study. AI and MS performed the research process and collected the data. AA performed the statistical analyses and was the project manager. AA, AJ, and MS wrote the original draft of the manuscript. AA, AJ, and HA prepared the figure and tables. HA, AJ, AA, and MS edited and revised the manuscript. All authors read and approved the final version of the manuscript.
Conflict of interest
The authors declare that the research was conducted in the absence of any commercial or financial relationships that could be construed as a potential conflict of interest.
Publisher’s note
All claims expressed in this article are solely those of the authors and do not necessarily represent those of their affiliated organizations, or those of the publisher, the editors and the reviewers. Any product that may be evaluated in this article, or claim that may be made by its manufacturer, is not guaranteed or endorsed by the publisher.
Abbreviations
AGU, Arabian Gulf University; CMMS, College of Medicine and Medical Sciences; GPA, Grade Point Average; HSGPA, High School Grade Point Average; MD, Medical Doctor; B.Sc., Bachelor of Medical Science; MCAT, Medical College Admission Test; MCQs, multiple-choice questions.
References
1. Ferguson E, James D, Madeley L. Factors associated with success in medical school: systematic review of the literature. BMJ. (2002). 324:952–7. doi: 10.1136/bmj.324.7343.952
2. Powis D. Selecting medical students: an unresolved challenge. Med Teach. (2015) 37:252–60. doi: 10.3109/0142159X.2014.993600
3. Griffin B, Harding DW, Wilson IG, Yeomans ND. Does practice make perfect? The effect of coaching and retesting on selection tests used for admission to an Australian medical school. Med J Austr. (2008) 189:270–3.
4. Siu E, Reiter HI. Overview: what’s worked and what hasn’t as a guide towards predictive admissions tool development. Adv Health Sci Educ. (2009) 14:759–75. doi: 10.1007/s10459-009-9160-8
5. Poole P, Shulruf B, Rudland J, Wilkinson T. Comparison of UMAT scores and GPA in prediction of performance in medical school: a national study. Med Educ. (2012) 46:163–71. doi: 10.1111/j.1365-2923.2011.04078.x
6. Yates J, James D. The UK clinical aptitude test and clinical course performance at Nottingham: a prospective cohort study. BMC Med Educ. (2013) 13:32. doi: 10.1186/1472-6920-13-32
7. Zhou Y-X, Zhao Z-T, Li L, Wan C-S, Peng C-H, Yang J, et al. Predictors of first-year GPA of medical students: a longitudinal study of 1285 matriculates in China. BMC Med Educ. (2014) 14:87. doi: 10.1186/1472-6920-14-87
8. Bacon DR, Bean B. GPA in research studies: an invaluable but neglected opportunity. J Mark Educ. (2006) 28:35–42.
9. Richardson M, Abraham C, Bond R. Psychological correlates of university students’ academic performance: a systematic review and meta-analysis. Psychol Bull. (2012) 138:353.
10. Sulphey MM, Al-Kahtani NS, Syed AM. Relationship between admission grades and academic achievement. Entrepreneurship Sustain Issues. (2018) 5:648–58.
11. Hassan SM, Al-Razgan MS. Pre-university exams effect on students GPA: a case study in IT department. Proc Comput Sci. (2016) 82:127–31.
12. Al-Rukban MO, Munshi FM, Abdulghani HM, Al-Hoqail I. The ability of the pre-admission criteria to predict performance in a Saudi medical school. Saudi Med J. (2010) 31:560–4.
13. Murshid KR. The predictive value of individual admission criteria on academic performance in a Saudi medical college. J Taibah Univ Med Sci. (2013) 8:18–23.
14. Alamoudi AA, Fallatah HI, Eldakhakhny BM, Kamel FO, AlShawwa LA, Elsamanoudy AZ. Relationship between admission criteria and academic performance in basic science courses in health science colleges in KAU. BMC Med Educ. (2021) 21:94. doi: 10.1186/s12909-021-02502-4
15. Baker H, Cope M, Fisk R, Gorby J, Foster R. Relationship of preadmission variables and first-and second-year course performance to performance on the national board of osteopathic medical examiners’ COMLEX-USA level 1 examination. J Osteopath Med. (2000) 100:153–61.
16. Wiley A, Koenig JA. The validity of the medical college admission test for predicting performance in the first two years of medical school. Acad Med. (1996) 71:S83–5.
17. Carline JD, Cullen TJ, Scott CS, Shannon NF, Schaad D. Predicting performance during clinical years from the new medical college admission test. J Med Educ. (1983) 58:18–25.
18. Jones RF, Thomae-Forgues M. Validity of the MCAT in predicting performance in the first two years of medical school. J Med Educ. (1984) 59:455–64.
19. Robi YS. Determinants of freshman CGPA: high school result, entrance exam result and EGSECE GPA. Int J Psychol Behav Sci. (2015) 5:121–7.
20. Mercer A, Puddey IB. Admission selection criteria as predictors of outcomes in an undergraduate medical course: a prospective study. Med Teach. (2011) 33:997–1004. doi: 10.3109/0142159X.2011.577123
21. Alkushi A, Althewini A. The predictive validity of admission criteria for college assignment in Saudi universities: King Saud bin Abdulaziz university for health sciences experience. Int Educ Stud. (2020) 13:141–9.
22. Schofield W, Garrard J. Longitudinal study of medical students selected for admission to medical school by actuarial and committee methods. Br J Med Educ. (1975) 9:86–90. doi: 10.1111/j.1365-2923.1975.tb01900.x
23. Touron J. High school ranks and admission tests as predictors of first year medical students’ performance. High Educ. (1987) 16:257–66.
24. Simpson PL, Scicluna HA, Jones PD, Cole A, O’Sullivan AJ, Harris PG, et al. Predictive validity of a new integrated selection process for medical school admission. BMC Med Educ. (2014) 14:86. doi: 10.1186/1472-6920-14-86
25. Edwards D, Friedman T, Pearce J. Same admissions tools, different outcomes: a critical perspective on predictive validity in three undergraduate medical schools. BMC Med Educ. (2013) 13:173. doi: 10.1186/1472-6920-13-173
26. Puddey IB, Mercer A. Predicting academic outcomes in an Australian graduate entry medical programme. BMC Med Educ. (2014) 14:31. doi: 10.1186/1472-6920-14-31
27. Dunleavy DM, Kroopnick MH, Dowd KW, Searcy CA, Zhao X. The predictive validity of the MCAT exam in relation to academic performance through medical school: a national cohort study of 2001–2004 matriculants. Acad Med. (2013) 88:666–71. doi: 10.1097/ACM.0b013e3182864299
Keywords: predictive validity, admission criteria, academic performance, foundation year, medical program
Citation: Almarabheh A, Shehata MH, Ismaeel A, Atwa H and Jaradat A (2022) Predictive validity of admission criteria in predicting academic performance of medical students: A retrospective cohort study. Front. Med. 9:971926. doi: 10.3389/fmed.2022.971926
Received: 14 July 2022; Accepted: 18 August 2022;
Published: 08 September 2022.
Edited by:
Yu-Che Chang, Linkou Chang Gung Memorial Hospital, TaiwanReviewed by:
Ayman Zaky Elsamanoudy, Mansoura University, EgyptYvonne Görlich, Private University of Applied Sciences, Germany
Copyright © 2022 Almarabheh, Shehata, Ismaeel, Atwa and Jaradat. This is an open-access article distributed under the terms of the Creative Commons Attribution License (CC BY). The use, distribution or reproduction in other forums is permitted, provided the original author(s) and the copyright owner(s) are credited and that the original publication in this journal is cited, in accordance with accepted academic practice. No use, distribution or reproduction is permitted which does not comply with these terms.
*Correspondence: Amer Almarabheh, amerjka@agu.edu.bh