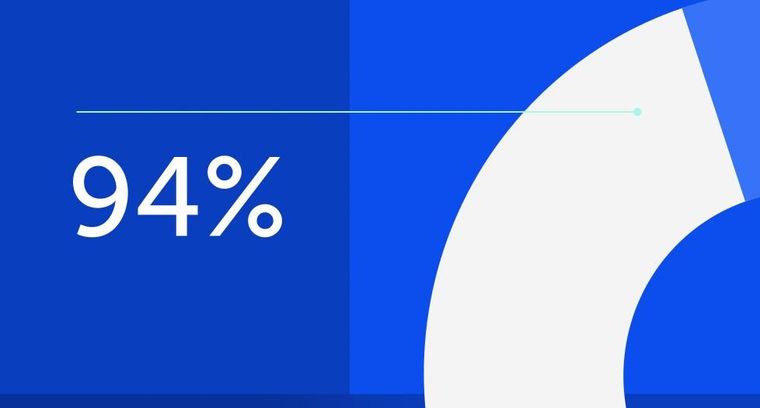
94% of researchers rate our articles as excellent or good
Learn more about the work of our research integrity team to safeguard the quality of each article we publish.
Find out more
REVIEW article
Front. Med., 01 July 2022
Sec. Translational Medicine
Volume 9 - 2022 | https://doi.org/10.3389/fmed.2022.911861
The human history has witnessed the rapid development of technologies such as high-throughput sequencing and mass spectrometry that led to the concept of “omics” and methodological advancement in systematically interrogating a cellular system. Yet, the ever-growing types of molecules and regulatory mechanisms being discovered have been persistently transforming our understandings on the cellular machinery. This renders cell omics seemingly, like the universe, expand with no limit and our goal toward the complete harness of the cellular system merely impossible. Therefore, it is imperative to review what has been done and is being done to predict what can be done toward the translation of omics information to disease control with minimal cell perturbation. With a focus on the “four big omics,” i.e., genomics, transcriptomics, proteomics, metabolomics, we delineate hierarchies of these omics together with their epiomics and interactomics, and review technologies developed for interrogation. We predict, among others, redoxomics as an emerging omics layer that views cell decision toward the physiological or pathological state as a fine-tuned redox balance.
“OMICS,” defined as probing and analyzing large amount of data representing the structure and function of an entire makeup of a given biological system at a particular level, has substantially revolutionized our methodologies in interrogating biological systems. In other words, “top down” approaches, largely attributable to “omics” development, coupled with “bottom up” strategies to offer a holistic tool for efficient biological system investigation. The concept of dissecting complex disorders including cancers has been, accordingly, advanced from static delineation between cell malignant and heathy states in a low-throughput manner to spatio-temporal dynamic deconvolution of complex systems involving multi-layer modifications at genomic, transcriptomic, proteomic, and metabolic levels in a global-unbiased fashion.
Ever since the establishment of the first high-throughput technology, DNA microarray (1), technologies for omics exploration have been developed by leaps and bounds. Following the central dogma, omics technologies have been used to capture the static genomic alterations, temporal transcriptomic perturbations and alternative splicing, as well as spatio-temporal proteomic dynamics and post translational modifications (PTMs) (2). Beyond this, omics technologies have been expanded to analyze various omics at the epi-level (such as epigenome, epitranscriptome, epiproteome that are defined as the collection of all modifications of the referred omics beyond information it covered in a single cell), molecular interactions (i.e., varied levels of interactome), and disease associated hallmarks as metabolome and immunome. Multi-omics integration has become a prevailing trend for constructing a comprehensive causal relationship between molecular signatures and phenotypic manifestations of a particular disease, and single cell sequencing offers additional resolving power that enables investigations at a single cell level. This rapidly-developing and ever-growing field, omics, has empowered us to uncover the intricate molecular mechanism underlying different phenotypic manifestations of disordered traits in an overwhelming and systematic manner at a high accuracy. However, the complexity of the cellular behavior and its decision-making system may persistently drive the establishment of novel omics and associated techniques.
While we are running close to the truth in principle, the ever-growing knowledge on cellular omics persistently transforms our understandings toward cell machinery complexity that challenges our goal toward the fully harness of cell pathological state rewiring. It is, thus, time to comprehensively review what has been done and is being done in omics-relevant studies to forecast what can be done in “omics” as a shortcut toward our goal. Focusing on the four big omics, i.e., genomics, transcriptomics, proteomics and metabolics, their epiomics and pair-wise interactomics, this paper comprehensively reviews high-throughput technologies developed, and forecasts, among others, the emerging role of “redoxomics” on the cell machinery.
Sequencing and mass spectrometry (MS) are basic experimental tools availing in our tour in investigating the omics of a given biological system. While sequencing-based approaches are feasible for studies on genome, transcriptome, their epitomes and interactomes involving DNA/RNA, MS-based techniques can be used to interrogate proteome, metabolome, and interactomes that do not involve DNA/RNA (Figure 1, Table 1).
Figure 1. Conceptual illustration on the hierarchy of different omics covered in this paper. We classify omics technologies into two categories, i.e., technology- and knowledge- based. Technology-based omics are based on technologies developed for understanding the “central dogma,” which can be further divided into three groups, i.e., the “four big omics” (genomics, transcriptomics, proteomics, and metabolomics), epiomics (epigenomics, epitranscriptomics, and epiproteomics), and their interactomics (DNA-RNA interactomics, RNA-RNA interactomics, DNA-protein interactomics, RNA-protein interactomics, protein-protein interactomics, and protein-metabolite interactomics). Omics indicated by the horizontal (above) and vertical (right-hand side) pink boxes of each interactomic term constitute to its two interacting omics. Knowledge-based omics are developed to understand a particular knowledge domain in a systematic way through integrating multiple omics information. Examples of this category include immunomics, microbiomics, and beyond.
Genomic techniques are dedicated to investigate the inter-individual variations at both the germline and somatic levels via sequencing the genome of interest. The development from DNA microarray technology (50), first generation Sanger sequencing (51), second generation massively parallel sequencing, also known as the next generation sequencing (NGS) (52), and the eventual third generation of long reads sequencing (TGS) (53) have enabled the sequencing of the whole genome/exome with sufficient in-depth to characterize the mutational landscape of a given sample.
The DNA microarray technology was firstly established by Schena et al. (1), where thousands of probes were fixed to a surface and samples were labeled with fluorescent dyes for detection after hybridization (54). There are two types of DNA microarrays, i.e., 2-channel and 1-channel arrays, with Agilent (55) and Affymetrix GeneChip (56) being the typical 2- and 1-channel commercial array, respectively. In a 2-channel array, the array slides are fabricated by spotting with cDNA fragments or oligonucleotide probes; after hybridizing both samples, labeled by two types of fluorescent dyes such as Cy®5 and Cy®3, on the array, the gene expression of treated sample relative to the control is quantified by the ratio of the 2-channel intensities of each spot (57). In a 1-channel array, the oligonucleotide probes are synthesized on the slide surface to hybridize the fluorescence-labeled sample cDNAs, where the absolute intensity of hybridization signal is measured (58). As a variation of 1-channel array, Illumina BeadArray synthesizes barcoded probes on the surface of microbeads (59) (https://www.ncbi.nlm.nih.gov/probe/docs/techbeadarray). The DNA microarray technology is relatively mature, with various well-established experimental platforms and analytical tools available (60). Yet, the main drawback of DNA microarray technologies lies in its inability to detect de novo transcripts, since such technologies rely on probes designed according to known nucleotide sequences. Besides, DNA microarray is not a feasible platform when analyzing highly repetitive genomes due to the high occurrence of cross-hybridization events that may lead to inaccurate signal intensity estimation (61).
Sanger sequencing, also known as the first generation of DNA sequencing, was invented in 1977 (62). It is based on the selective incorporation of chain-terminating dideoxynucleotides by DNA polymerase during in vitro DNA replication. With the relatively long read length (i.e., up to ~1,000 bp) and high per-base accuracy (i.e., ~99.999%) (7), Sanger sequencing has been used to achieve a number of monumental accomplishments such as the completion of the Human Genome Project (63), and dominated this filed for almost 30 years (62, 63). Yet, it suffers from high cost and low throughput that calls for novel technologies delivering fast, inexpensive, and accurate solutions (62, 64).
NGS genome sequencing, comprised of primarily four categories, i.e., cyclic-array sequencing (65, 66), microelectrophoretic methods (67), sequencing by hybridization (68), and real-time observation of single molecules (69, 70), has dramatically improved the speed and scalability of genome sequencing. Taking cyclic-array sequencing as an example, the throughput has been substantially improved taking advantages of iterative cycles of enzymatic catalytic processes (4). Several commercial products are of this kind such as 454 Genome Sequencers (Roche Life Science, USA) (66), Illumina Genome Analyzer (Illumina, USA) (71), and SOLiD platform (Applied Biosystems, USA) (72), which have made milestone contributions to the omics field. However, Roche454 Genome Sequencers and the SOLiD platform quitted the market later due to, e.g., poor market acceptance, leaving Illumina the sole company dominating this field. Many mainstream products are from Illumina including, e.g., the MiSeq series such as MiSeq FGx, HiSeq series such as HiSeq X10, NextSeq series such as NextSeq550, and NovaSeq series such as NovaSeq6000. Beijing Genomics Institute (BGI), after the acquisition of Complete Genomics (CG), has entered the sequencing market and become an emerging institution capable of sequencer development, with BGIseq500 and NDBseq-T7 being its representative products. Other NGS platforms such as Ion Torrent (Thermo Fisher) also take market shares. NGS outweighs Sanger sequencing in higher speed and throughput (e.g., >106 reads/array in cyclic array sequencing), easier gene library construction, higher level of parallelism, and less costly in clinical practice [i.e., saving 30–1,249$/patient for cancer diagnosis (73)]. However, NGS suffers from the short read lengths it generated (averaged read length ranges from 32 to 330 bp) that leads to at least 10 folds less accuracy than Sanger sequencing (62, 64). Importantly, these short-read methods cannot well capture structural variants (SVs), repetitive elements, high/low GC content, or sequences with multiple homologous elements in the genome (74, 75). Also, the call for lowering down the overall cost persists as it still costs 1–60$/megabase despite the fact that the cost has already been lowered-down by several orders of magnitude as compared with Sanger sequencing.
TGS, the third revolution in sequencing technology as enabled by Pacific Biosciences (PacBio, 2011) (76) and Oxford Nanopore Technologies (ONT, 2014) (77), is a single molecular and real-time sequencing technique that allows for the long-read sequencing with low alignment and mapping errors during library construction. PacBio adopts the single molecule real-time (SMRT) technique, where ssDNA templates replicate during DNA library preparation automatically. PacBio SMRT has two sequencing modes, i.e., circular consensus sequencing (CCS) and continuous long read (CLR) sequencing, which differ in read length and error rate. While CCS has a higher accuracy at the sacrifice of read length by adopting a circular ssDNA template, CLR outweighs in getting higher coverage of ultra-long insert molecules that can substantially improve the assembly quality. During PacBio SMRT sequencing, the fluorescence signals are activated by a laser during the incorporation of a labeled dNTPs into DNA, and the color and duration of the emitted signals are recorded in real time during cell flow that is equipped with zero mode waveguides (78). In the ONT system, nanopores are inserted in an electrical resistant membrane, where a potential is applied across the membrane to enable a current flow through the nanopore, and signals are measured as characteristic disruptions in the current for each specific single molecule. A hairpin structure is designed to ligate the double DNA strands (dsDNA) during DNA library construction to enable the system read both DNA strands in one continuous read. The dsDNA is attached to the pore by the bound polymerase or helicase enzyme, and the signal of each nucleotide is captured as a characteristic disruption in the electrical current during sequencing while dsDNA moves through the nanopore (79). ONT can detect hundreds of kilobases in one continuous read, and sequence ultra-long reads (ULRs), i.e., with the length over 300 kb or even up to 1 million bp (80). Besides, some ONT sequencers are in the pocket-size that are portable without sophisticated laboratory setup, offering additional flexibility (81).
It is worth mentioning that, despite the rapid development and increasing popularity of NGS and TGS, the DNA microarray technology still gains favor in genome-wide association studies (GWAS) for the sake of economy, and there is a gaining momentum to combine DNA microarray and NGS/TGS in genotyping toward increased resolution of population-specific haplotypes and imputation strength (82).
Genomic sequencing technologies have been applied to characterize many genetic disorders (such as highly identical segmental duplications that account for over 5% of the human genome and are enriched in the short arm of the chromosome 16 (83) and diseases associated with BRCA1/2 mutations (84)), identify intratypic sequence variations [such as that of SARS-CoV-2 variants (85) and bovine papillomaviruses (86)], interrogate the genomic landscape of complex diseases [such as endometrial cancers (87) and thyroid carcinomas (88)], and discover novel alleles of polymorphic gene clusters in the human genome [such as that of the HLA system (89, 90)].
Unlike genome, transcriptome is dynamic and composed of diversified players. It is subjected to alterations imposed by cell development stage, internal and/or external stimuli, and the time point at which the signals are measured. Traditional transcriptome refers to mRNA transcripts, but can also be generalized to include other types of transcripts such as microRNAs (miRNAs), long non-coding RNAs (lncRNAs), and circular RNAs (circRNA). Transcriptomics techniques aim to detect and quantify RNA molecules transcribed from a particular genome at a given time (91).
Prior to the advent of NGS, RNA microarray was used as the conventional experimental technique to detect mRNA alterations within cells of interest at different stages in a high-throughput manner. RNA microarrays can be used to profile differentially expressed genes and identify markers capable of distinguishing cells between the normal and cancer states by concomitantly quantifying the relative mRNA abundance of thousands of genes.
Leveraged by the establishment of NGS technologies, RNA sequencing becomes possible that can be used to identify the presence and abundance of RNA transcripts in an unbiased and high throughput manner. Similar to DNA sequencing, de novo transcripts can be identified using RNA sequencing techniques given its independence on existing probes. Aided with the RNA sequencing technology, a vast amount and diversified types of non-coding RNAs (ncRNAs) have been discovered and found to be pervasively transcribed from the intergenic and intronic genome regions (92). This has substantially revolutionized our concept toward the complexity of mammalian transcriptome and the regulatory mechanisms leading to complex diseases such as cancers (93). RNA sequencing is commonly performed using DNA sequencing instruments given the platform compatibility (94) and technical maturity of commercially available DNA sequencing instruments (95), despite the possibility on direct RNA sequencing (96).
In addition to the whole transcriptome, a myriad of RNA sequencing platforms have been established to achieve ad hoc tasks. These techniques include tag-based methods (using one fragment to represent a transcript) such as digital gene expression (DEG) sequencing and 3' end sequencing, sequencing approaches to probe alternative splicing and gene fusion, targeted RNA sequencing, and single cell RNA sequencing (9). DEG sequencing is a deep sequencing approach derived from serial analysis of gene expression (SAGE) that is more economical than traditional RNA sequencing for a given sequencing depth (97–99). 3' end sequencing has been developed to interrogate alternative cleavage and poly(A) sites, which is comprised of approaches utilizing oligo (dT) for reverse transcription such as PAS sequencing (100), poly A sequencing (101), 3'T-fill (102), methods using RNA-based ligation to capture the 3' end fragments such as 3P sequencing (103) and 3'READS (104), and methods examining both the 3' end and the poly(A) tail length simultaneously such as TAIL sequencing (105) and PAT sequencing (106). Sequencing techniques such as RASL sequencing (107) can be used to analyze splice junction sites through the use of oligo pairs to target specific exon-exon junction sequences. Fusion events are typically revealed by reads containing fusion junctions or differences in expression between the 5' and 3' ends of genes that are fused. Several approaches have been established to specifically achieve this goal. As commercialized by NuGene and ArcherDX (108, 109), fusion genes are identified by amplicon-based sequencing through the use of two sequence-specific primers together with a common primer targeting the adapter sequence. Other approaches for fusion gene detection include RNA sequencing reads enrichment and exon capture (9). Sequencing a selected set of transcripts is sometimes desirable, leading to the development of targeted RNA sequencing. Approaches fell into this category include “target capture” (110–112) and “amplicon sequencing” (109, 113, 114). Driven by the demand of uncovering signals averaged out by examining the behavior of bulk cells at the population level, single cell sequencing has been evolved into a unique area for interrogating all levels of omics, where transcriptome is the first and most well-studied omics that has been interrogated at a single cell level. Sequencing techniques include, e.g., CEL-seq2 (115), STRT-seq (116), and Drop-seq (117).
Sequencing approaches have been established for interrogating other types of RNA species besides mRNA. Small non-coding RNAs such as miRNAs, piRNAs, and endosiRNAs are short in size, i.e., typically below 30 nt. Methods capable of separating them from contaminant DNAs are needed that include electrophoresis separation and blocking the 3′ adapter from ligating with the 5′ adapter by adding the reverse transcription primer (9). CircRNAs are generated by back-splicing (118), which can be sequenced by digesting and removing linear RNAs using exonuclease R, followed by regular RNA sequencing (9).
A growing number of platforms have been established to investigate RNAs at different stages of biogenesis, metabolism, and interactions with molecules such as proteins and other RNAs. These approaches share similar protocols in cDNA library preparation, but differ significantly in RNA-capturing that ranges from RNase protection to immunoprecipitation and to metabolic labeling (9).
Transcriptomic sequencing approaches have been widely used in medical studies, such as constructing the transcriptomic signatures of intestinal failure-associated liver diseases (119), revealing the pathogenesis of COVID-19 (120), identifying diagnostic biomarkers and therapeutic targets of multiple meylomas (121), and deciphering cell heterogeneity during osteogenesis of human adipose-derived mesenchymal stem cells (122).
Epigenomics explains alterations in the regulation of gene activities that function without modifying genetic sequences, which serves as a major regulatory mechanism on gene transcription (123). It involves characterization of higher order chromatin structure (also constitutes to the DNA-DNA interactome) and DNA/RNA modifications such as DNA/RNA methylation (124).
Hi-C is a comprehensive technique developed to capture chromosome conformation, where chromatin is crosslinked with formaldehyde, digested, and re-ligated such that only DNA fragments covalently linked together are ligated. In Hi-C, a biotin-labeled nucleotide is incorporated at the ligation junction to enable selective purification of chimeric DNA ligation products followed by deep sequencing, where the ligation products contain the physical information of their genomic location origin and 3D genome organization (23).
Chemical modulations on certain DNA base as represented by DNA methylation may create dramatic impact on gene expression. Whole genome bisulfite sequencing represents a standard approach for methylated Cytosine base detection, which involves treating genomic DNA using sodium bisulfite followed by sequencing to generate a genome-wide landscape of methylated Cytosine at a single base resolution. MBD-isolated genome sequencing (MiGS) is another tool for whole genome methylation profiling that relies on the precipitation of methylated DNA by the recombinant methyl-CpG binding domain of MBD2 followed by sequencing (24).
Illumina short-read sequencing has been coupled with immunoprecipitation for DNA modification detection which, though being feasible for identifying epigenetic alterations in broad genomic regions, cannot reach the resolution at a single base level nor differentiate reads from different cells. Long-read sequencing technologies such as PacBio and Oxford nanopore sequencing techniques have thus been adapted for epigenome interrogation. PacBio SMRT, which monitors the polymerase in real time during DNA sequencing, detects epigenetic modifications by monitoring the inter-pulse durations in the reading rate of a polymerase as a result of kinetic variation (125). ONT for DNA modification detection relies on the different electric current alterations generated by molecules of different forms (e.g., methylated nucleotides) when they pass through a nanopore (126). Several groups have demonstrated the feasibility of TGS technologies in detecting DNA modifications, and tools such as Multi-MotifMaker (125) and NanoMod (126) have been designed accordingly for large scale de novo DNA modification detection.
Epigenomic sequencing technologies have enabled, for example and among others, non-invasive epigenomic molecular phenotyping of the human brain (127), rapid epigenomic diagnosis of brain cancers (128), and inference of epigenomic cell-state dynamics (129).
Epitranscriptomics seeks to elucidate the role of RNA structure and modifications in regulating gene expression, where RNA modification focuses on modified nucleotides in mRNA (130).
Sequencing-based methodologies for mapping RNA structures can be classified into enzyme-based in vitro methods, chemical-based in vitro methods, and chemical-based in vivo methods (25). Enzyme-based in vitro approaches include parallel analysis of RNA structure (PARS) (131), fragmentation sequencing (FragSeq) (132), parallel analysis of RNA structures with temperature elevation (PARTE) (28), and protein interaction profile sequencing (PIP-seq) (133). These methods are limited to in vitro structural analysis and leverage different ribonucleases (RNases; including RNase V1, RNase S1, RNase P1, and RNase T1) to generate a mixture of RNA fragments that, once been analyzed by sequencing, allows for RNA secondary structure inference. Chemical-based methods utilize small membrane permeable molecules such as nucleobase-specific chemicals, carbodiimide modifying reagents, and ribose-specific probes to interrogate RNA structure, which can be applied both in vitro and in vivo and often capable of achieving single-nucleotide resolution. Chemical-based in vitro methods include structure-seq (134), dimethyl sulfate sequencing (DMS-seq) (135), and high-throughput sequencing for chemical probing of RNA structure (Mod-seq) (136). Chemical-based in vivo methods include chemical inference of RNA structures sequencing (CIRS-seq) (137), selective 20-hydroxyl acylation analyzed by primer extension and mutational profiling (SHAPE-MaP) (138), in vivo click selective 2-hydroxyl acylation and profiling experiment (icSHAPE) (139), and mapping RNA-RNA interactome and RNA structure in vivo (MARIO) (140). Combining data from both chemical- and enzyme- based sequencing approaches is considered ideal to gain a more comprehensive RNA structural information.
While chemical modifications on RNA are evolutionarily conserved traits of structural RNAs such as rRNA and tRNA (141), their presence in lncRNAs and small regulatory RNAs (srRNAs) has attracted renewed attention to define their roles in gene expression regulation and disease initiation/progression. The rapid evolution of RNA sequencing technologies has enabled the development of methodologies to interrogate the topography of RNA modifications in the whole transcriptome.
The 13 chemical modifications identified so far in mRNAs can be divided into two categories, i.e., modifications of nucleotides adjacent to the 5' cap and internal RNA modifications. Cap-adjacent nucleotide modifications typically regulate RNA stability and translation, and can occur in mRNA, primary miRNA transcript, lncRNA, small nucleolar RNA (snoRNA), and small nuclear RNA (snRNA) (142). Internal modifications can reside in 5' and 3' untranslated regions (UTRs), coding regions, and mRNA introns, with m6A (N6-methyladenosine) and adenosine to inosine (A to I) editing being the most abundant (143). Internal modifications participate in a diverse spectrum of gene regulatory programs such as mRNA splicing, 3'-end processing, export, stability, localization and translation (144).
As the abundance of mRNA modifications is in general low, large amounts of mRNAs are needed or very deep sequencing is required using current experimental approaches. This has catalyzed the generation of many sequencing-based approaches for transcriptome-wide mapping of mRNA modifications. The most prevalent approach is RNA immunoprecipitation (RIP) sequencing (RIP-seq), where modified nucleotides are recognized by antibodies followed by whole transcriptome sequencing (RIP-seq) (145). Other approaches such as PA-m6A-seq (146), miCLIP-seq (147), m1A-MAP (148), and m7G-MeRIP-seq (149) have been established to enhance the resolution to a single base level taking advantages of nucleotide mismatches or truncation signatures induced to the modified nucleotides before reverse transcription and sequencing by crosslinking antibodies. LAIC-seq can differentiate m6A methylation levels between mRNA isoforms without prior fragmentation at the expense of losing the positional information (150).
Besides, using chemical reactions specific to a given RNA modification followed by short read sequencing provides an alternative approach for RNA modification detection. For instance, RNA bisulfite sequencing (RNA-BisSeq) is one of these approaches that relies on chemical deamination of cytidine to uridine by sodium bisulfite, leaving m5C intact (151–153). A similar yet different category of approaches is enzyme-based, which includes, e.g., MAZTER-seq (154) and m6A-REF-seq (155). In these approaches, the endoRNases MazF and ChpBK cut unmethylated RNA at ACA and UAC motifs without touching m6A methylated RNA (154, 155).
Epitranscriptomic sequencing technologies have enabled us to profile the landscape of epitranscriptomic RNA modifications (156) toward enhanced understandings of biological systems such as a prototype baculovirus (157).
Given the prevalence of DNA-RNA interactions at the transcription start sites (158, 159), diversified modes of chromatin-RNA interactions (160–162), and the high correlation between RNA-chromatin attachment and histone modification events such as H3K27ac and H3K4me3 (158), a variety of tools have been established to investigate the DNA-RNA interactome. These technologies can be divided into two categories, i.e., mapping genome-wide locations of a specific RNA, and mapping all chromatin-interacting RNAs together with their genomic interacting regions.
Methods fell into the first category include chromatin isolation by RNA purification (ChIRP) (163), capture hybridization analysis of RNA targets (CHART) (164), and RNA antisense purification (RAP) (160). These approaches take advantages of biotinylated complementary oligonucleotides to pull down a specific target RNA together with its binding partners followed by characterization of these binding molecules through sequencing or MS.
Technologies of the second category include mapping RNA–genome interactions (MARGI) (158), chromatin-associated RNA sequencing (ChAR-seq) (165), and mapping global RNA interactions with DNA by deep sequencing (GRID-seq) (159). These methodologies leverage crosslinking reagents to preserve DNA-RNA interactions followed by proximity ligation to convert RNA and its binding DNA into a chimeric sequence complex before sequencing.
Approaches of this category have been used for, among others, identifying de novo targets of chromatin-bound RNAs including nascent transcripts, chromosome-specific dosage compensation ncRNAs, and genome-wide trans-associated RNAs participating in co-transcriptional RNA processing (165, 166).
The diversity, flexibility and complexity of the RNA kingdom regarding the types and molecular functions of RNAs have rendered RNA-RNA interactomics a unique omics layer that had attracted much attention.
Methodologies developed for probing RNA-RNA interaction had once been restricted by the prior knowledge on one of the interacting RNAs such as X-ray crystallography, nuclear magnetic resonance (NMR), and psoralen cross-linking (167). The discovery of chimeric RNAs from transcriptome data has made it possible to detect de novo RNA-RNA interactions despite the low prevalence of chimeric RNAs (168). Identifying RNA-RNA interactions by purifying proteins bringing RNA interactants together is the rational of RNA hybrid and individual-nucleotide resolution UV crosslinking and immunoprecipitation (hiCLIP) (169) and crosslinking, ligation, and sequencing of hybrids (CLASH) (170). Yet, both approaches differ in the utility of antibody-based isolation of target protein in hiCLIP and using ectopic expression of a tagged protein in CLASH.
Methods established for high-throughput RNA interactome analysis leverage proximity ligation to produce chimeric sequences, which include, e.g., psoralen analysis of RNA interactions and structures (PARIS) (171), sequencing of psoralen-crosslinked, ligated, and selected hybrids (SPLASH) (172), ligation of interacting RNA followed by high-throughput sequencing (LIGR-seq) (173), and MARIO (140). These methods share similar protocols that contain in vivo RNA crosslinking, RNA fragmentation, intramolecular ligation and reverse crosslinking. It is noteworthy that the choice of crosslinking reagents may determine the types of RNA interactions to be discovered. For example, psoralen or its derivatives as used in PARIS, SPLASH, and LIGR-seq intercalate in RNA helices and can be used to identify hybridized RNA pairs.
Interrogating the RNA-RNA interactome has enabled us to construct the higher-order transcriptome structure of living cells that guided the discovery of lncRNA structures and functionalities (171), has aided in defining the principles of how RNAs interact with themselves and with other RNAs in gene regulation and ribosome biogenesis (172), and has helped in revealing novel interactions between snoRNAs and mRNAs (173).
Interactions between proteins and DNA play fundamental roles in transducing genetic information into functionalities. Methods for characterizing such interactions include electrophoretic mobility shift assays, DNase footprinting, ChIP, and systematic evolution of ligands by exponential enrichment (SELEX) (174–177) which, however, are only useful if the DNA remains intact.
Protein binding microarrays have also been adopted to investigate transcription factor (TF)-DNA interactions; yet, the binding sites of many TFs are longer than those that can be combined to the array (178). ChIP-hybridized association mapping platforms provide another category of approaches to investigate protein-DNA interactions (179). SELEX, coupled with NGS, represents a high-throughput solution for studying protein-DNA interactome which, however, is not feasible to use if DNA is cleaved during protein binding (180). These aforementioned platforms unanimously require the known identify of the protein of interest, with approaches capable of identifying de novo protein-DNA interactions urgently called for.
ChIP-Chip, also known as the genome-wide location analysis, combines chromatin immunoprecipitation (ChIP) and DNA microarray analysis to identify protein-DNA interactions occurring in living cells (181). It has been considered as the conventional approach used in analyzing histone modifications before the invention of NGS, which shares the same drawbacks as ChIP (e.g., constrained by the availability of an organism-specific microarray). ChIP sequencing, coupling ChIP with NGS, outweighs ChIP-Chip by generating outputs with higher spatial resolution, dynamic range, and genomic coverage and, importantly, capable of exploring any species with a sequenced genome in principle (181, 182). In ChIP sequencing, DNA-bound proteins are immunoprecipitated by specific antibody followed by extraction, purification, and sequencing of the bound DNA. This enabled us to interrogate histone modifications and the interactome that offer deep insights into genomic regulatory events.
The pervasive transcription of the genome creates the diversified reservoir of ncRNAs. Despite our limited knowledge regarding the functions and regulatory mechanisms of ncRNAs, it has been well-accepted that interactions between RNA binding proteins (RBPs) and RNAs play vital roles in cell homeostasis maintenance. The dynamic combination, competition and coordination between RBPs and RNA offer many pointcuts to the mechanisms of action of these RNAs. Many experimental techniques have been established to systematically investigate such interactions. High-throughput sequencing of RNA isolated by cross-linking immunoprecipitation, namely HITS-CLIP or CLIP-seq (39), as well as its variants such as photoactivatable-ribonucleoside-enhanced cross-linking and immunoprecipitation (PAR-CLIP) (183), individual-nucleotide resolution cross-linking and immunoprecipitation (iCLIP) (184), cross-linking and analysis of cDNAs (CRAC) (185) and cross-linking, ligation, and sequencing of hybrids (CLASH) (167) have been consecutively established to decode protein-RNA interactomes.
While these technologies have been successfully applied to decode miRNA-target interactions (186), identify RNA binding sites of splicing factors (187, 188), investigate epigenetic modification-associated RNAs (189), and explore functions of ceRNAs (190), they suffer from several limitations that await additional improvements. These technical issues include, e.g., how to further reduce the background noise [that though is already lower than RIP (191)] and simultaneously enhance RNA output efficiency to study low abundance RBPs, as CLIP achieves a high signal-to-noise ratio at the cost of RNA abundance (40).
Proteomics investigates the functional relevance of all expressed proteins in a cell, tissue or organism [namely proteome (192)] by interrogating the information flow through protein signaling (193). Since most biological functionalities are actioned by proteins, it is important to reliably measure proteome alterations during cellular state transitions such as in the context of carcinogenesis (2).
MS is a primary protein characterization technique that can be used to determine the amino acid sequence of a protein and PTM sites. MS measures charged molecules based on their mass-to-charge ratios, where the mass analyzer characterizes molecules by their mass-to-charge ratios, and the signal intensities of charged peptides reflect the quantity. One significant advance brought by MS to the omics field is the rate at which it identifies proteins in an entirely discovery-driven way. The advent of the high-resolution “LTQ™Orbitrap™ (194)” MS instruments coupled with powerful analytical tools such as MaxQuant enabled the first draft of human proteome (194).
There are several types of commercially available mass spectrometers. These include high-resolution MS methods such as LTQ™Orbitrap™ (194), matrix-assisted laser desorption ionization-time of flight-time of flight (MALDI-TOF-TOF), and Fourier transform ion cyclotron resonance (FT-ICR) that measures the exact masses [i.e., the theoretical mass of specific isotopic composition of a charged molecule (195)], and low resolution MS approaches such as quadrupole and ion-trap that measure the nominal mass [defined as the integer mass of the most abundant stable isotope of a molecular ion (195)].
Various tandem mass spectrometric techniques (MS/MS) approaches have been established to generate unique fragmentation profiles from different proteins that enables de novo protein characterization and isobaric peptides differentiation (196). In MS/MS, peptides isolated from the first mass analyzer are subjected to the second mass analyzer on collision with a neutral gas or interaction with activated electrons, where sequential fragmentation can be performed as needed. Several MS/MS platforms are available including electron-based approaches such as electron capture dissociation (ECD) (197), electron-induced dissociation (EID) (198), electron transfer dissociation (ETD) (199), and collision-induced dissociation (CID) where fragment ions are generated from collision with neutral gases (200). It is often to collect the structural information of the target molecules using different MS/MS methods that are complementary in revealing the true identities of unknown proteins (201).
Liquid chromatography-mass spectrometry (LC-MS) and gas chromatography-mass spectrometry (GC-MS) are MS coupled with chromatography-based separation modules that convey several advantages over MS. One critical benefit of using LC or GC before MS is the alleviation of the matrix effect and ion suppression by separating analytes from interfering endogenous compounds such as salts and ion pairing agents using an appropriate chromatography. Also, peptides can be quantified by measuring the area under the chromatographic peak. LC-MS has been used to characterize various kinds of proteins with broad ranges of physicochemical properties and molecular weights, and the use of GC-MS in protein characterization is rather rare that has been limited to profile relatively more volatile molecules with lower molecular weights such as protein adducts.
Proteome interrogation has diverse applications such as identifying novel ceramide-binding proteins (202), analyzing Ophiocordyceps sinensis at different culture periods (203), and interrogating the proteomic landscape of cardiometabolic diseases (204).
Metabolites, typically defined as low molecular weight biomolecules (<1,500 Da) participating in cell endogenous metabolism, function as energy sources, signaling molecules, and metabolic intermediates with protein modulatory roles (see section on epiproteomics) in complex biological systems. Metabolites have been demonstrated to serve as important biological modulators across multilayer omics toward the maintenance of cellular homeostasis. Metabolome is referred to as a collection of all metabolites in a cell that encompass all biomolecules except for the genome, transcriptome, proteome, and metals.
Methods for metabolome interrogation include Fourier transform-infrared (FT-IR) spectroscopy (205), Raman spectroscopy (206), NMR spectroscopy (18, 207), MS-based approaches such as MS (20), MS/MS (208), liquid chromatography (LC)-MS (209), gas chromatography (GC)-MS (210, 211), as well as others.
FT-IR, Raman and NMR spectroscopies provide non-destructive and rapid solutions for metabolite analysis, where absorption spectra at specific wavelengths determines the structure of unknown metabolites and the area under the curve (AUC) of the absorption spectra quantifies the amount. However, these approaches do not have sufficient sensitivity and selectivity (212–215).
MS is the most feasible tool to probe the metabolome that can detect a wide spectrum of metabolites (216). Isobaric metabolites can be distinguished by various MS/MS (208) and/or being coupled with LC (209) or GC (210, 211).
Metabolomics is comprised of targeted and non-targeted metabolomics. While targeted metabolomics addresses specific biological hypotheses by providing quantitative information for target metabolites involved in specific metabolic pathways, non-targeted metabolomics generates hypotheses and can be used for de novo target identification by charactering as many metabolites as possible in a biological sample (217).
Metabolome profiling has been used in identifying candidate genes and metabolites (218), as well as revealing the metabolic mechanism of the therapeutic efficacy (219).
Besides sequencing-based detection approaches, RNA modification can also be investigated using LC-MS/MS. In these approaches, total RNA or purified mRNA are digested into individual nucleotides followed by LC-MS/MS, and the presence and quantification of all RNA modifications can be determined by comparing the MS peaks from the sample with that of standards (30, 149, 220). Though these methods are quantitative and the results are concordant across studies, they require large amounts of input samples and are not feasible for detecting low abundance nucleotides such as caps, and do not provide information on the location of the modified positions.
Epitranscriptome landscape has been used to decode the atlas of RNA modifications (221), and characterize the topology of human and mouse m5C epitranscriptome (222).
Epiproteome includes PTMs occurring in histone and non-histone proteins such as protein phosphorylome, methylome, acetylome, ubiquitinome, SUMOylation, and newly discovered lactylome, succinome, and etc. While histone constitutes as an important player in the protein-DNA interactome and takes a central role in shaping the epigenome (223), and non-histone proteins participate in signal relay and actualize genetic information into cell functions, epiproteome of various kinds offer a unique view on how epigenetic regulations impact critical events associated with the central dogma and cell behavior through marking proteins with varied epiproteomic barcodes.
Microsequencing is the first approach ever used for epiproteomic studies that adopts Edman degradation to determine protein sequence, which is time-consuming and requires a large amount of highly purified sample (31). Later, antibody-based methods such as western blotting, immunofluorescence analysis and ChIP gained a wide popularity in low-throughput PTM studies. However, antibody-based assays rely on modification-specific antibodies which are not always available, require a priori knowledge of the type and position of the modification of interest, and are not capable of measuring multiple PTMs occurring within the same protein. MS-based proteomics has enabled the characterization of protein PTMs in a high-throughput manner. MS-based methodologies enable unbiased profiling of diverse modifications simultaneously, quantitative analysis of protein modifications, and de novo identification of unknown modification patterns.
MS-based strategies for epiproteome investigation can be classified into three categories, i.e., “bottom-up” where a target protein is proteolytically digested into short peptides (5–20 Aa) prior to MS analysis, “top-down” that defines the proteomes present in a sample through analyzing intact proteins, and “middle-down” that is designed for analyzing histone PTMs as histone N-terminal tails can be cleaved off by specific proteases to generate polypeptides with accessible size for MS detection. The “top-down” approach in a high-throughput fashion can depict a comprehensive and accurate view on the epiproteome of the targeted system, but is challenging as larger molecules are difficult to be separated by LC and analyzed by MS, and the chance of having isobaric proteomes (species sharing the same mass and similar physico-chemical properties such as H3K27me1K36me2 and H3K27me2K36me1) increases with the portion of protein analyzed (224).
Phosphoproteome is perhaps the most pervasive PTM landscape, the analysis of which adopts the “bottom-up” approach. Peptide digests are typically enriched (through the use of, e.g., immunoaffinity chromatography, ion exchange chromatography, immobilized metal ion affinity chromatography, metal oxide affinity chromatography, chemical derivatization), fractioned off- or on-line (by chromatographic separation techniques such as reversed phase high performance liquid chromatography) prior to mass spectrometry analysis (225). A recently commercialized aerodynamic high-field asymmetric waveform ion mobility spectrometry (FAIMS) device was recently incorporated into the phosphoproteomic workflow to aid in the gas-phase fractionation, which resulted in the identification of around 15–20% additional phosphorylation sites and a 26% increase of the reproducibility (226).
Epiproteome interrogation has been largely applied to capture the dynamic phosphoproteome profile of a particular biological system such as prostate cancer (227), human urine (228), influenza A/B (229), and budding yeast (230), or map cellular alterations in response to specific perturbations (231) such as HIV-infected brain (232).
Protein-protein interactions (PPIs) represent the most prominent and well-studied molecular interactions within cells. The earliest high-throughput technology employed for PPI identification is yeast two hybrid (Y2H) screening, which works by separating two functional domains of a single TF that elicits signals once brought into close proximity (233). Y2H screening is laboriousness and cannot identify multi-protein complexes in one run (42). MS-based protein characterization allows rapid identification of multiple interacting proteins without the need of antibodies (234–236), with the outputs easily confirmable using targeted approaches such as IP or immunofluorescence (237). As one example, ~5,700 proteins and over 27000 complex PPIs were identified using LC-MS/MS and merged into a protein-protein interactome, termed BraInMap (238). Lastly, it is worth to mention co-immunoprecipitation (coIP-MS) followed by mass spectrometry, which is a common practice to interrogate proteins interacting with a given protein bait (44).
A high-throughput screening approach has been established to characterize PPIs directly from cell-free protein synthesis reactions. Proteins of interest are immobilized non-covalently on the donor and acceptor beads that produce chemiluminescent signals on interactions. This technique relies on the Amplified Luminescent Proximity Homogeneous Linked Immunosorbent Assay (AlphaLISA) that enables rapid PPI characterization without the need for protein purification and a highly parallel and miniaturized workflow taking advantages of robotic and acoustic liquid handling. This recent technology can characterize competitive binding of proteins for specific epitopes besides direct PPIs, and thus has been applied to screen candidate antibodies capable of competing with the spike receptor-binding domain (RBD) of SARS-CoV-2 for human angiotensin-converting enzyme 2 (ACE2) (239).
Protein-metabolite interactions are essential in maintaining cell homeostasis under the conventional state, and coordinating responses to internal or external stress or perturbations. Protein-metabolite interactions are prevalent in cells which were estimated to be at the scale of millions (240–242).
Early methods exploring protein-metabolite interactions adopt protein tagging or metabolite modification (46, 243) and are limited to the detection of protein-lipid interactions and those involving hydrophobic molecules (244–246). A systematic and unbiased method to identify de novo protein-metabolite interactions was proposed, under the hypothesis that the binding of a metabolite to a protein of interest can block its Proteinase K cleavage sites (247). In this approach, proteins are extracted under non-denaturing conditions with metabolites being cleared off using size-exclusion chromatography; a specific metabolite is added to an aliquot of the protein followed by Proteinase K-mediated proteolysis of both metabolite-containing and metabolite-free proteomes and trypsin-mediated complete digestion; the MS output of metabolite-bound proteome is expected to contain two non-tryptic termini whereas the other one does not (247). Though this approach offers a non-biased solution without the need of any chemical modifications, it does not provide a comprehensive set of protein-metabolite interactions as currently available MS cannot detect all peptides within any enzymatically digested samples. Another critical issue of this approach that challenges its application in eukaryote cells is the loss of compartmentation information by cell lysing (247). PROtein-Metabolite Interactions using Size separation (PROMIS), a simultaneous global interrogation tool of the protein-metabolite interactome, has been developed that is featured by low false positives related to a high concentration of the bait molecule (proteins or metabolites) and low false negatives related to small-molecule modifications (47). An NMR-based approach permitting the direct detection of interactions between any set of water-soluble proteins and metabolites was proposed (48), and applied to investigate protein-metabolite interactions in the central metabolism of Escherichia coli (248). An approach based on high-resolution NMR relaxometry that does not require any invasive procedure or separation step was established to detect weak metabolite-macromolecule interactions in complex media such as biological fluids (49).
Through interrogating the protein-metabolite interactome, novel enzyme-substrate relationships and cases of metabolite-induced protein complex remodeling have been identified (247), and a wide range of proteins participating in lipid pathways have been pharmacologically characterized in mammalian cells, among which a selective ligand for NUCB1 (a compound that perturbs the hydrolytic and oxidative metabolism of endocannabinoids in cells) was identified (244).
The concept of “omics” has been extended from a set of experimental and computational approaches as well as a particular layer of molecular information interrogated using these established tools to a cocktail of knowledge gained by integrating multiple omics data in a particular research domain. This concept shift has led to the generation of omics such as immunomics and microbiomics (Figure 1, Table 1).
The term of “immunomics” was firstly introduced in 2001 (249), and refers to the interrogation of immunology through the integration of information from genomics, proteomics and transcriptomics, with the aim of translating molecular immunology into clinics (250). The immunome can be defined as the set of antigens or epitopes that interface with the host immune system (251). Immunomics has been used to improve disease diagnosis, treatment and prevention (252), with the potential of revolutionizing our rational on vaccine design and antigen discovery (250).
Microbiomics is the science of collecting, characterizing and quantifying molecules responsible for the structure, function, and dynamics of a microbial community by integrating multiple omics information such as genomics, transcriptomics, proteomics and metabolomics, where all microorganisms of a given environment, called microbiome, are analyzed to study the potential role that such microorganisms have in diseases (253, 254). Human microbiome, emerged in 2008 from the human microbiome project (http://commonfund.nih.gov/hmp/index), is comprised of trillions of microbes inhabiting inside the human body and interacting with their host (255). Evidence from rodent models of microbiome studies has suggested strong associations between microbiomes and human diseases (256), and microbiome can provide unique insights into human diseases, with unprecedented ability demonstrated in interrogating and modulating the communities that co-inhabit with human (257, 258).
The suffix “omics” represents the revolutionary technological advancement made in the past three decades that enables us to simultaneously analyze thousands of molecules. Genomics, transcriptomics, proteomics and metabolomics, namely the “four big omics” (240), have led to the creation of their epiomics (epigenomics, epitranscriptomics, and epiproteomics) and interactomics (e.g., DNA-RNA interactomics, RNA-RNA interactomics, DNA-protein interactomics, RNA-protein interactomics, protein-protein interactomics, and protein-metabolite interactomics) which are technology-based, and other omics such as immunomics and microbiomics that are knowledge-based.
Despite the abundancy and diversity of the experimental and computational approaches available for omics interrogation, translating knowledge gained by diving into varied levels of omics into clinical practice is still at its nascent stage. Whether omics studies can significantly impact disease diagnosis and therapeutics depends on the resolvent of issues from the technical aspect and existing in the knowledge extraction process. Technical problems include, e.g., how to substantially reduce the sample amount without sacrificing statistical accuracy when the sample is rare and precious, how to reduce replicates without negatively affecting output stability, and how to increase the accuracy of the output by reducing both false negative (FN) and false positive (FP) rates. The reproducibility of MS-based omics is, in general, lower than sequencing-based omics. For example, the results of some MS-based omics such as proteomics, metabolomics and their epiomics are highly dependent on the type of mass spectrometer, as well as the sample processing protocol and data processing pipeline used. This renders the compromise of the accuracy and reproducibility of some aforementioned omics unavoidable in pursuit of the global landscape. Domain specific languages such as Nextflow (259) have been established to help partially resolve the reproducibility issue by sharing the codes and workflows used for data analysis. Different omics interrogation approaches have different FN and FR rates, depending on many computational indexes such as the calling pipeline parameters and read coverage. Take short-read NGS as an example for sequencing-based omics, the FN rate was reported to vary between ~6 and 18%, and the FR rate was <3% (260). The FN and FR rates for MS-based omics are, in general, higher than that of sequencing-based omics due to inadequate capture of some protein contents in a sample (FN) or misidentification of a chemically modified peptide as a biological variation (FR) (261). Concerns impeding the knowledge gaining process include, e.g., the heterogeneity across studies regarding the study design, sample size and sampling approach, treatment, and follow-up duration, leading to the little generality of the varied outputs consecutively being reported (Figure 2).
Figure 2. Conceptual illustration on the future trend in omics technology development. There are three trends regarding the developmental paths of omics technologies. The first category of tasks is to resolve the technical problems existing in current omics techniques. The second trend is the identification of novel types of omics especially novel epiomics derived from modifications by various intermediate metabolites. With increased understanding on the importance of cell homeostasis at various levels regarding human disease management, there is a trend of cutting into a particular knowledge domain from a systematic angle via omics data integration as demonstrated by immunomics and microbiomics. In this trend, we propose “redoxomics” as an emerging type of knowledge-based omics given the critical roles of redox homeostasis in maintaining cells at the healthy state and the pathogenesis of various diseases including cancers.
As we learn more about known cell components, novel types of biological players are being discovered that further complicate our existing knowledge on how human cells behave under physiological and pathological conditions. Besides, the number and variety of cells comprising the system to be studied regarding human health has been expanded by an order of magnitude by microbiome (262). These technological advances sometimes make our efforts toward complete understanding of human cell system frustrating as the finishing line seems to be at an ultimate distance that can never be reached given the consistently being identified new factors with prominent roles on the cell machinery. For instance, diversified types of protein modifications have been recognized such as acetylation, lactylation, succination, and crotonylation in the past few years, most modifiers of which are intermediates from the ATP production processes such as glycolysis and the TCA cycle. This not only centers the role of metabolism in cell behavior regulation, but also inspires us to think whether other intermediates (such as fumarate) also regulate protein functionalities through creating marks on proteins? Is this one of the primary routes that external intakes (such as food) regulate cellular behavior that bridges the gap between cell metabolism and functionalities? These, by all means, deserve our deep thinking and represent interesting topics to explore (Figure 2).
Another important factor contributing to the huge complexity of cells that challenges translational omics is the multi-layer heterogeneity. Omics technologies at a particular level can only present one picture of a cellular system that dynamically transits among varied states. In addition, cells presented in tissues or biopsies can be very heterogeneous, rendering accurate interpretation of omics information challenging. Further, differential clinical manifestations and treatment responses among patients add an additional layer of complexity regarding the clinical use of omics technologies.
Accordingly, there had been a trend of integrating multiple omics information toward improved understanding of a particular knowledge domain as evidenced by the generation of immunomics and microbiomics. This enables us to portrait a systematic view on a research area that gains additional value if the topic conveys systematic impacts on human cells regarding its decision toward either homeostasis maintenance under the healthy state or running into a chaotic state that drives cells malignant.
Redox imbalance has been indicated as a causal factor of a variety of disorders such as cancer (263–265) and hypertension (266) with translational significance. Besides, reactive oxygen and nitrogen species (RONS) are also important signaling molecules with known roles on normal function regulation such as insulin and growth factor signaling (267). Also along with this line is oxidative cysteine modification that had been considered as a central PTM event associated with many diseases (268–270). The significance of redox homeostasis in disease state control may characterize redox biology at the omics scale, possibly named as “redoxomics,” which offers new avenues for therapeutic intervention (Figure 2). Already there exist some strategies to characterize protein thiol modifications (271), making investigations on redoxomics technically possible. Methods of this kind can be categorized to MS-based modalities that require efficient trapping of the native redox state of the thiol proteome given the labile nature of cysteine residues. In these methods, thiols are protonated by strong acid followed by free thiol blockage using a reactive thiol alkylating reagent such as N-ethyl maleimide (NEM) or iodoacetaminde (IAM); Cysteine residues are then labeled by, e.g., isotopically modified derivatives of thiol alkylating reagents, and characterized by LC-MS, LC-MS/MS or peptide mass fingerprinting (272). In most cases, this is just the first step toward cysteine modification, and orthogonal technology development represents an essential research direction that may lead the trend in omics technique development if redoxomics gains sufficient attention as it deserves.
By leveraging multiplexed fluorescence, DNA, RNA and isotype labeling, and taking advantages of multiomics data interrogation, “spatial omics” has emerged to enable the detection of variations in, e.g., transcriptome and proteome, within their native spatial context (273) and been commonly coupled with single cell technologies (274). Spatially resolved transcriptomics (SRT) is the first and most well-developed technology of this kind that identifies gene expression profiles in tissue biopsies prior to histopathological annotations. Following the trend in multiple omics integration, techniques on spatial multiomics have been established by integrating whole SRT with immunofluorescence protein detection in the same tissue section, allowing the gaining of a holistic view on variations in colocalized protein and gene expression profiles with tissue organization (275). Visium, a software provided by 10 × Genomics, can be used for constructing high-resolution microscopic images with transcriptomic data aligned to the tissue footprint, and extended for interpreting spatial multiomics. The authors anticipate an emerging trend of other “info-omics” beyond “spatial omics” in the future by integrating omics with other dimension of information such as “time” in the form of, e.g., treatment duration, and follow-up time.
Last but not the least is the critical contribution of bioinformatics to omics interrogation. Various toolboxes have been made available for, e.g., data quality control, pre-processing, abnormal molecule identification, interaction prediction, enrichment analysis, pathway analysis, network construction, as well as more advanced or focused analyses such as pseudo-time and trajectory inference in single cell sequencing. Besides, numerous databases and computational tools have been developed to allow for the easy access and analysis of omics data. The Cancer Genome Atlas (TCGA) (276) and Gene Expression Omnibus (GEO) (277), among others, have been frequently used for omics data deposit and retrieval. Genome Sequence Archive (GSA) (278) developed by the Chinese National Genomic Data Center has been launched to compliment this giant-size database portfolio, with GSA-human recently announced as part of GSA to provide a repository for human genetic related omics data (279). Various databases smaller in size and/or with more specialized focuses have been established by different groups such as “LCMD” for lung cancer metabolome (280), “DBSAV” for deleterious single amino acid variation prediction in human proteome (281), “SARS-CoV-2 3D” for coronavirus proteome (282), and “CMVdb” for cytomegalovirus multi-omes (283). A plethora of machine-learning algorithms and computational tools have been developed to interrogate these omics data toward gained knowledge or new discoveries such as WeiBI for PPI taking into account of functional enrichment (284), DTI-MLCD for drug-target interactions utilizing multi-label learning (285), and MDF-SA-DDI for predicting interaction events between two drugs based on a transformer self-attention mechanism (286). We foresee a reciprocal transformation between our dry and wet lab powers in omics investigation, i.e., while technical advances urge the development of novel bioinformatics tools, intelligent computational strategies broaden our horizon on the complexity of the biological network that urges the emergence of novel experimental approaches. This will boost both fields flourish toward our enhanced abilities for cellular omics interrogation.
XD conceptualized the idea, drafted the paper, provided financial support, and final proved the manuscript. XD and LS conducted literature searching and prepared figures and tables. All authors agree with the content and its publication.
This study was funded by the National Natural Science Foundation of China (Grant No. 81972789) and Fundamental Research Funds for the Central Universities (Grant No. JUSRP22011).
The authors declare that the research was conducted in the absence of any commercial or financial relationships that could be construed as a potential conflict of interest.
All claims expressed in this article are solely those of the authors and do not necessarily represent those of their affiliated organizations, or those of the publisher, the editors and the reviewers. Any product that may be evaluated in this article, or claim that may be made by its manufacturer, is not guaranteed or endorsed by the publisher.
1. Schena M, Shalon D, Davis RW, Brown PO. Quantitative monitoring of gene expression patterns with a complementary DNA microarray. Science. (1995) 270:467–70. doi: 10.1126/science.270.5235.467
2. Chakraborty S, Hosen MI, Ahmed M, Shekhar HU. Onco-multi-OMICS approach: a new frontier in cancer research. Biomed Res Int. (2018) 2018:9836256. doi: 10.1155/2018/9836256
3. Shendure JA, Porreca GJ, Church GM, Gardner AF, Hendrickson CL, Kieleczawa J, et al. Overview of DNA sequencing strategies. Curr Protoc Mol Biol. (2011) 7:mb0701s96. doi: 10.1002/0471142727.mb0701s96
4. Mitra RD, Church GM. In situ localized amplification and contact replication of many individual DNA molecules. Nucleic Acids Res. (1999) 27:e34. doi: 10.1093/nar/27.24.e34
5. Suarez-Farinas M, Magnasco MO. Comparing microarray studies. Methods Mol Biol. (2007) 377:139–52. doi: 10.1007/978-1-59745-390-5_8
6. Mantione KJ, Kream RM, Kuzelova H, Ptacek R, Raboch J, Samuel JM, et al. Comparing bioinformatic gene expression profiling methods: microarray and RNA-Seq. Med Sci Monit Basic Res. (2014) 20:138–42. doi: 10.12659/MSMBR.892101
7. Shendure J, Ji H. Next-generation DNA sequencing. Nat Biotechnol. (2008) 26:1135–45. doi: 10.1038/nbt1486
8. van Dijk EL, Jaszczyszyn Y, Naquin D, Thermes C. The third revolution in sequencing technology. Trends Genet. (2018) 34:666–81. doi: 10.1016/j.tig.2018.05.008
9. Hrdlickova R, Toloue M, Tian B. RNA-Seq methods for transcriptome analysis. Wiley Interdiscip Rev RNA. (2017) 8:wrna.1364. doi: 10.1002/wrna.1364
10. Ding J, Adiconis X, Simmons SK, Kowalczyk MS, Hession CC, Marjanovic ND, et al. Systematic comparison of single-cell and single-nucleus RNA-sequencing methods. Nat Biotechnol. (2020) 38:737–46. doi: 10.1038/s41587-020-0465-8
11. Haag AM. Mass analyzers and mass spectrometers. Adv Exp Med Biol. (2016) 919:157–69. doi: 10.1007/978-3-319-41448-5_7
12. Qi Y, Volmer DA. Structural analysis of small to medium-sized molecules by mass spectrometry after electron-ion fragmentation (ExD) reactions. Analyst. (2016) 141:794–806. doi: 10.1039/C5AN02171E
13. Hart-Smith G. A review of electron-capture and electron-transfer dissociation tandem mass spectrometry in polymer chemistry. Anal Chim Acta. (2014) 808:44–55. doi: 10.1016/j.aca.2013.09.033
14. Chen X, Wang Z, Wong YE, Wu R, Zhang F, Chan TD. Electron-ion reaction-based dissociation: a powerful ion activation method for the elucidation of natural product structures. Mass Spectrom Rev. (2018) 37:793–810. doi: 10.1002/mas.21563
15. Jurczak E, Mazurek AH, Szeleszczuk L, Pisklak DM, Zielinska-Pisklak M. Pharmaceutical hydrates analysis-overview of methods and recent advances. Pharmaceutics. (2020) 12:959. doi: 10.3390/pharmaceutics12100959
16. Jurowski K, Kochan K, Walczak J, Baranska M, Piekoszewski W, Buszewski B. Analytical techniques in lipidomics: state of the art. Crit Rev Anal Chem. (2017) 47:418–37. doi: 10.1080/10408347.2017.1310613
17. Eberhardt K, Stiebing C, Matthaus C, Schmitt M, Popp J. Advantages and limitations of Raman spectroscopy for molecular diagnostics: an update. Expert Rev Mol Diagn. (2015) 15:773–87. doi: 10.1586/14737159.2015.1036744
18. Eom JS, Kim ET, Kim HS, Choi YY, Lee SJ, Lee SS, et al. Metabolomics comparison of rumen fluid and milk in dairy cattle using proton nuclear magnetic resonance spectroscopy. Anim Biosci. (2021) 34:213–22. doi: 10.5713/ajas.20.0197
19. Emwas AH, Roy R, McKay RT, Tenori L, Saccenti E, Gowda GAN, et al. NMR spectroscopy for metabolomics research. Metabolites. (2019) 9:123. doi: 10.3390/metabo9070123
20. Want EJ, Cravatt BF, Siuzdak G. The expanding role of mass spectrometry in metabolite profiling and characterization. Chembiochem. (2005) 6:1941–51. doi: 10.1002/cbic.200500151
21. Vogeser M, Parhofer KG. Liquid chromatography tandem-mass spectrometry (LC-MS/MS)–technique and applications in endocrinology. Exp Clin Endocrinol Diabetes. (2007) 115:559–70. doi: 10.1055/s-2007-981458
22. Seger C. Usage and limitations of liquid chromatography-tandem mass spectrometry (LC-MS/MS) in clinical routine laboratories. Wien Med Wochenschr. (2012) 162:499–504. doi: 10.1007/s10354-012-0147-3
23. Belton JM, McCord RP, Gibcus JH, Naumova N, Zhan Y, Dekker J, et al. A comprehensive technique to capture the conformation of genomes. Methods. (2012) 58:268–76. doi: 10.1016/j.ymeth.2012.05.001
24. Serre D, Lee BH, Ting AH. MBD-isolated Genome Sequencing provides a high-throughput and comprehensive survey of DNA methylation in the human genome. Nucleic Acids Res. (2010) 38:391–9. doi: 10.1093/nar/gkp992
25. Nguyen TC, Zaleta-Rivera K, Huang X, Dai X, Zhong S RNA. Action through Interactions. Trends Genet. (2018) 34:867–82. doi: 10.1016/j.tig.2018.08.001
26. Underwood JG, Uzilov AV, Katzman S, Onodera CS, Mainzer JE, Mathews DH, et al. FragSeq: transcriptome-wide RNA structure probing using high-throughput sequencing. Nat Methods. (2010) 7:995–1001. doi: 10.1038/nmeth.1529
27. Silverman IM, Berkowitz ND, Gosai SJ, Gregory BD. Genome-wide approaches for RNA structure probing. Adv Exp Med Biol. (2016) 907:29–59. doi: 10.1007/978-3-319-29073-7_2
28. Wan Y, Qu K, Ouyang Z, Kertesz M, Li J, Tibshirani R, et al. Genome-wide measurement of RNA folding energies. Mol Cell. (2012) 48:169–81. doi: 10.1016/j.molcel.2012.08.008
29. Zhao LY, Song J, Liu Y, Song CX, Yi C. Mapping the epigenetic modifications of DNA and RNA. Protein Cell. (2020) 11:792–808. doi: 10.1007/s13238-020-00733-7
30. Yuan BF. Liquid chromatography-mass spectrometry for analysis of RNA adenosine methylation. Methods Mol Biol. (2017) 1562:33–42. doi: 10.1007/978-1-4939-6807-7_3
31. Brandt WF, von Holt C. The determination of the primary structure of histone F3 from chicken erythrocytes by automatic Edman degradation. 2. Sequence analysis of histone F3. Eur J Biochem. (1974) 46:419–29. doi: 10.1111/j.1432-1033.1974.tb03635.x
32. Mishra M, Tiwari S, Gomes AV. Protein purification and analysis: next generation Western blotting techniques. Expert Rev Proteomics. (2017) 14:1037–53. doi: 10.1080/14789450.2017.1388167
33. Hoffman MD, Sniatynski MJ, Kast J. Current approaches for global post-translational modification discovery and mass spectrometric analysis. Anal Chim Acta. (2008) 627:50–61. doi: 10.1016/j.aca.2008.03.032
34. Giepmans BN, Adams SR, Ellisman MH, Tsien RY. The fluorescent toolbox for assessing protein location and function. Science. (2006) 312:217–24. doi: 10.1126/science.1124618
35. Im K, Mareninov S, Diaz MFP, Yong WH. An introduction to performing immunofluorescence staining. Methods Mol Biol. (2019) 1897:299–311. doi: 10.1007/978-1-4939-8935-5_26
36. Park PJ. ChIP-seq: advantages and challenges of a maturing technology. Nat Rev Genet. (2009) 10:669–80. doi: 10.1038/nrg2641
37. Zhang Z, Wu S, Stenoien DL, Pasa-Tolic L. High-throughput proteomics. Annu Rev Anal Chem. (2014) 7:427–54. doi: 10.1146/annurev-anchem-071213-020216
38. Ferraz RAC, Lopes ALG, da Silva JAF, Moreira DFV, Ferreira MJN, de Almeida Coimbra SV. DNA-protein interaction studies: a historical and comparative analysis. Plant Methods. (2021) 17:82. doi: 10.1186/s13007-021-00780-z
39. Murigneux V, Sauliere J, Roest Crollius H, Le Hir H. Transcriptome-wide identification of RNA binding sites by CLIP-seq. Methods. (2013) 63:32–40. doi: 10.1016/j.ymeth.2013.03.022
40. Zhang Y, Xie S, Xu H, Qu L. CLIP viewing the RNA world from an RNA-protein interactome perspective. Sci China Life Sci. (2015) 58:75–88. doi: 10.1007/s11427-014-4764-5
41. Carneiro DG, Clarke T, Davies CC, Bailey D. Identifying novel protein interactions: proteomic methods, optimisation approaches and data analysis pipelines. Methods. (2016) 95:46–54. doi: 10.1016/j.ymeth.2015.08.022
42. Lathouwers T, Wagemans J, Lavigne R. Identification of protein-protein interactions using pool-array-based yeast two-hybrid screening. Methods Mol Biol. (2018) 1794:29–48. doi: 10.1007/978-1-4939-7871-7_3
43. von Mering C, Krause R, Snel B, Cornell M, Oliver SG, Fields S, et al. Comparative assessment of large-scale data sets of protein-protein interactions. Nature. (2002) 417:399–403. doi: 10.1038/nature750
44. LagundŽin D, Krieger KL, Law HC, Woods NT. An optimized co-immunoprecipitation protocol for the analysis of endogenous protein-protein interactions in cell lines using mass spectrometry. STAR Protoc. (2022) 3:101234. doi: 10.1016/j.xpro.2022.101234
45. Zhou M, Li Q, Wang R. Current experimental methods for characterizing protein-protein interactions. ChemMedChem. (2016) 11:738–56. doi: 10.1002/cmdc.201500495
46. Diether M, Sauer U. Towards detecting regulatory protein-metabolite interactions. Curr Opin Microbiol. (2017) 39:16–23. doi: 10.1016/j.mib.2017.07.006
47. Veyel D, Sokolowska EM, Moreno JC, Kierszniowska S, Cichon J, Wojciechowska I, et al. PROMIS, global analysis of PROtein-metabolite interactions using size separation in Arabidopsis thaliana. J Biol Chem. (2018) 293:12440–53. doi: 10.1074/jbc.RA118.003351
48. Nikolaev YV, Kochanowski K, Link H, Sauer U, Allain FH. Systematic identification of protein-metabolite interactions in complex metabolite mixtures by ligand-detected nuclear magnetic resonance spectroscopy. Biochemistry. (2016) 55:2590–600. doi: 10.1021/acs.biochem.5b01291
49. Wang Z, Pisano S, Ghini V, Kaderavek P, Zachrdla M, Pelupessy P, et al. Detection of metabolite-protein interactions in complex biological samples by high-resolution relaxometry: toward interactomics by NMR. J Am Chem Soc. (2021) 2021:13603301. doi: 10.26434/chemrxiv.13603301
50. Thomas R, Pontius JU, Borst LB, Breen M. Development of a genome-wide oligonucleotide microarray platform for detection of DNA copy number aberrations in feline cancers. Vet Sci. (2020) 7:88. doi: 10.3390/vetsci7030088
51. Moniruzzaman M, Hossain MU, Islam MN, Rahman MH, Ahmed I, Rahman TA, et al. Coding-complete genome sequence of SARS-CoV-2 isolate from Bangladesh by Sanger Sequencing. Microbiol Resour Announc. (2020) 9:e00626–e0. doi: 10.1128/MRA.00626-20
52. Chen X, Dong Y, Huang Y, Fan J, Yang M, Zhang J. Whole-genome resequencing using next-generation and Nanopore sequencing for molecular characterization of T-DNA integration in transgenic poplar 741. BMC Genomics. (2021) 22:329. doi: 10.1186/s12864-021-07625-y
53. Ou YJ, Ren QQ, Fang ST, Wu JG, Jiang YX, Chen YR, et al. Complete genome insights into Lactococcus petauri CF11 isolated from a healthy human gut using second- and third-generation sequencing. Front Genet. (2020) 11:119. doi: 10.3389/fgene.2020.00119
54. Kinaret PAS, Serra A, Federico A, Kohonen P, Nymark P, Liampa I, et al. Transcriptomics in toxicogenomics, part i: experimental design, technologies, publicly available data, and regulatory aspects. Nanomaterials. (2020) 10:750. doi: 10.3390/nano10040750
55. Painter HJ, Altenhofen LM, Kafsack BF, Llinas M. Whole-genome analysis of Plasmodium spp. Utilizing a new agilent technologies DNA microarray platform. Methods Mol Biol. (2013) 923:213–9. doi: 10.1007/978-1-62703-026-7_14
56. Graham NS, May ST, Daniel ZC, Emmerson ZF, Brameld JM, Parr T. Use of the Affymetrix Human GeneChip array and genomic DNA hybridisation probe selection to study ovine transcriptomes. Animal. (2011) 5:861–6. doi: 10.1017/S1751731110002533
57. Smyth GK, Altman NS. Separate-channel analysis of two-channel microarrays: recovering inter-spot information. BMC Bioinformatics. (2013) 14:165. doi: 10.1186/1471-2105-14-165
58. Liu H, Bebu I, Li X. Microarray probes and probe sets. Front Biosci. (2010) 2:325–38. doi: 10.2741/e93
59. National Center for Biotechnology Information. Bead Arrays. Available online at: https://www.ncbi.nlm.nih.gov/probe/docs/techbeadarray (accessed 31 March, 2022).
60. Slonim DK, Yanai I. Getting started in gene expression microarray analysis. PLoS Comput Biol. (2009) 5:e1000543. doi: 10.1371/journal.pcbi.1000543
61. Draghici S, Khatri P, Eklund AC, Szallasi Z. Reliability and reproducibility issues in DNA microarray measurements. Trends Genet. (2006) 22:101–9. doi: 10.1016/j.tig.2005.12.005
62. Sanger F, Nicklen S, Coulson AR. DNA sequencing with chain-terminating inhibitors. Proc Natl Acad Sci USA. (1977) 74:5463–7. doi: 10.1073/pnas.74.12.5463
63. Sanger F. Determination of nucleotide sequences in DNA. Biosci Rep. (2004) 24:237–53. doi: 10.1007/s10540-005-2733-8
64. Tran B, Dancey JE, Kamel-Reid S, McPherson JD, Bedard PL, Brown AM, et al. Cancer genomics: technology, discovery, and translation. J Clin Oncol. (2012) 30:647–60. doi: 10.1200/JCO.2011.39.2316
65. Shendure J, Porreca GJ, Reppas NB, Lin X, McCutcheon JP, Rosenbaum AM, et al. Accurate multiplex polony sequencing of an evolved bacterial genome. Science. (2005) 309:1728–32. doi: 10.1126/science.1117389
66. Margulies M, Egholm M, Altman WE, Attiya S, Bader JS, Bemben LA, et al. Genome sequencing in microfabricated high-density picolitre reactors. Nature. (2005) 437:376–80. doi: 10.1038/nature03959
67. Blazej RG, Kumaresan P, Mathies RA. Microfabricated bioprocessor for integrated nanoliter-scale Sanger DNA sequencing. Proc Natl Acad Sci USA. (2006) 103:7240–5. doi: 10.1073/pnas.0602476103
68. Gresham D, Dunham MJ, Botstein D. Comparing whole genomes using DNA microarrays. Nat Rev Genet. (2008) 9:291–302. doi: 10.1038/nrg2335
69. Soni GV, Meller A. Progress toward ultrafast DNA sequencing using solid-state nanopores. Clin Chem. (2007) 53:1996–2001. doi: 10.1373/clinchem.2007.091231
70. Healy K. Nanopore-based single-molecule DNA analysis. Nanomedicine. (2007) 2:459–81. doi: 10.2217/17435889.2.4.459
71. Bentley DR, Balasubramanian S, Swerdlow HP, Smith GP, Milton J, Brown CG, et al. Accurate whole human genome sequencing using reversible terminator chemistry. Nature. (2008) 456:53–9. doi: 10.1038/nature07517
72. Valouev A, Ichikawa J, Tonthat T, Stuart J, Ranade S, Peckham H, et al. A high-resolution, nucleosome position map of C. elegans reveals a lack of universal sequence-dictated positioning. Genome Res. (2008) 18:1051–63. doi: 10.1101/gr.076463.108
73. Pruneri G, De Braud F, Sapino A, Aglietta M, Vecchione A, Giusti R, et al. Next-generation sequencing in clinical practice: is it a cost-saving alternative to a single-gene testing approach? Pharmacoecon Open. (2021) 5:285–98. doi: 10.1007/s41669-020-00249-0
74. Salzberg SL, Yorke JA. Beware of mis-assembled genomes. Bioinformatics. (2005) 21:4320–1. doi: 10.1093/bioinformatics/bti769
75. Treangen TJ, Salzberg SL. Repetitive DNA and next-generation sequencing: computational challenges and solutions. Nat Rev Genet. (2011) 13:36–46. doi: 10.1038/nrg3117
76. Quail MA, Smith M, Coupland P, Otto TD, Harris SR, Connor TR, et al. A tale of three next generation sequencing platforms: comparison of Ion Torrent, Pacific Biosciences and Illumina MiSeq sequencers. BMC Genom. (2012) 13:341. doi: 10.1186/1471-2164-13-341
77. Jain M, Fiddes IT, Miga KH, Olsen HE, Paten B, Akeson M. Improved data analysis for the MinION nanopore sequencer. Nat Methods. (2015) 12:351–6. doi: 10.1038/nmeth.3290
78. Xiao T, Zhou W. The third generation sequencing: the advanced approach to genetic diseases. Transl Pediatr. (2020) 9:163–73. doi: 10.21037/tp.2020.03.06
79. Schatz MC. Nanopore sequencing meets epigenetics. Nat Methods. (2017) 14:347–8. doi: 10.1038/nmeth.4240
80. Midha MK, Wu M, Chiu KP. Long-read sequencing in deciphering human genetics to a greater depth. Hum Genet. (2019) 138:1201–15. doi: 10.1007/s00439-019-02064-y
81. Kafetzopoulou LE, Pullan ST, Lemey P, Suchard MA, Ehichioya DU, Pahlmann M, et al. Metagenomic sequencing at the epicenter of the Nigeria 2018 Lassa fever outbreak. Science. (2019) 363:74–7. doi: 10.1126/science.aau9343
82. Gudbjartsson DF, Helgason H, Gudjonsson SA, Zink F, Oddson A, Gylfason A, et al. Large-scale whole-genome sequencing of the Icelandic population. Nat Genet. (2015) 47:435–44. doi: 10.1038/ng.3247
83. Nicolle R, Siquier-Pernet K, Rio M, Guimier A, Ollivier E, Nitschke P, et al. 16p13.11p11.2 triplication syndrome: a new recognizable genomic disorder characterized by optical genome mapping and whole genome sequencing. Eur J Hum Genet. (2022) 22:1094. doi: 10.1038/s41431-022-01094-x
84. Lee JH, Ryoo NH, Ha JS, Park S, Kim KB, Hee KS, et al. Next generation sequencing is a reliable tool for detecting BRCA1/2 mutations, including large genomic rearrangements. Clin Lab. (2022) 68:210609. doi: 10.7754/Clin.Lab.2021.210609
85. Kuchinski KS, Nguyen J, Lee TD, Hickman R, Jassem AN, Hoang LMN, et al. Mutations in emerging variant of concern lineages disrupt genomic sequencing of SARS-CoV-2 clinical specimens. Int J Infect Dis. (2022) 114:51–4. doi: 10.1016/j.ijid.2021.10.050
86. Gysens L, Vanmechelen B, Haspeslagh M, Maes P, Martens A. New approach for genomic characterisation of equine sarcoid-derived BPV-1/-2 using nanopore-based sequencing. Virol J. (2022) 19:8. doi: 10.1186/s12985-021-01735-5
87. Hong JH, Cho HW, Ouh YT, Lee JK, Chun Y, Gim JA. Genomic landscape of advanced endometrial cancer analyzed by targeted next-generation sequencing and the cancer genome atlas (TCGA) dataset. J Gynecol Oncol. (2022) 33:e29. doi: 10.3802/jgo.2022.33.e29
88. Iqbal MA Li M, Lin J, Zhang G, Chen M, Moazzam NF, et al. Preliminary study on the sequencing of whole genomic methylation and transcriptome-related genes in thyroid carcinoma. Cancers. (2022) 14:1163. doi: 10.3390/cancers14051163
89. Maria L, Daria S, Svetlana D, Igor P. Genomic full-length sequence of the HLA-B*44:348 allele was identified by next generation sequencing. HLA. (2022) 2022:tan.14615. doi: 10.1111/tan.14615
90. Liacini A, Peters L, Pfau K, Mancini S, Geier S. Full genomic sequence of the HLA-DPA1*02:46 allele identified by next generation sequencing. HLA. (2022). doi: 10.1111/tan.14593
91. Wang Z, Gerstein M, Snyder M. RNA-Seq: a revolutionary tool for transcriptomics. Nat Rev Genet. (2009) 10:57–63. doi: 10.1038/nrg2484
92. Djebali S, Davis CA, Merkel A, Dobin A, Lassmann T, Mortazavi A, et al. Landscape of transcription in human cells. Nature. (2012) 489:101–8. doi: 10.1038/nature11233
93. Li M, Sun Q, Wang X. Transcriptional landscape of human cancers. Oncotarget. (2017) 8:34534–51. doi: 10.18632/oncotarget.15837
94. Eid J, Fehr A, Gray J, Luong K, Lyle J, Otto G, et al. Real-time DNA sequencing from single polymerase molecules. Science. (2009) 323:133–8. doi: 10.1126/science.1162986
95. Manzoni C, Kia DA, Vandrovcova J, Hardy J, Wood NW, Lewis PA, et al. Genome, transcriptome and proteome: the rise of omics data and their integration in biomedical sciences. Brief Bioinform. (2018) 19:286–302. doi: 10.1093/bib/bbw114
96. Ozsolak F, Platt AR, Jones DR, Reifenberger JG, Sass LE, McInerney P, et al. Direct RNA sequencing. Nature. (2009) 461:814–8. doi: 10.1038/nature08390
97. Asmann YW, Klee EW, Thompson EA, Perez EA, Middha S, Oberg AL, et al. 3' tag digital gene expression profiling of human brain and universal reference RNA using Illumina Genome Analyzer. BMC Genom. (2009) 10:531. doi: 10.1186/1471-2164-10-531
98. Morrissy AS, Morin RD, Delaney A, Zeng T, McDonald H, Jones S, et al. Next-generation tag sequencing for cancer gene expression profiling. Genome Res. (2009) 19:1825–35. doi: 10.1101/gr.094482.109
99. Chen EQ, Bai L, Gong DY, Tang H. Employment of digital gene expression profiling to identify potential pathogenic and therapeutic targets of fulminant hepatic failure. J Transl Med. (2015) 13:22. doi: 10.1186/s12967-015-0380-9
100. Shepard PJ, Choi EA, Lu J, Flanagan LA, Hertel KJ, Shi Y. Complex and dynamic landscape of RNA polyadenylation revealed by PAS-Seq. RNA. (2011) 17:761–72. doi: 10.1261/rna.2581711
101. Derti A, Garrett-Engele P, Macisaac KD, Stevens RC, Sriram S, Chen R, et al. A quantitative atlas of polyadenylation in five mammals. Genome Res. (2012) 22:1173–83. doi: 10.1101/gr.132563.111
102. Wilkening S, Pelechano V, Jarvelin AI, Tekkedil MM, Anders S, Benes V, et al. An efficient method for genome-wide polyadenylation site mapping and RNA quantification. Nucleic Acids Res. (2013) 41:e65. doi: 10.1093/nar/gkt364
103. Jan CH, Friedman RC, Ruby JG, Bartel DP. Formation, regulation and evolution of Caenorhabditis elegans 3'UTRs. Nature. (2011) 469:97–101. doi: 10.1038/nature09616
104. Hoque M, Ji Z, Zheng D, Luo W, Li W, You B, et al. Analysis of alternative cleavage and polyadenylation by 3' region extraction and deep sequencing. Nat Methods. (2013) 10:133–9. doi: 10.1038/nmeth.2288
105. Chang H, Lim J, Ha M, Kim VN. TAIL-seq: genome-wide determination of poly(A) tail length and 3' end modifications. Mol Cell. (2014) 53:1044–52. doi: 10.1016/j.molcel.2014.02.007
106. Swaminathan A, Harrison PF, Preiss T, Beilharz TH. PAT-Seq: a method for simultaneous quantitation of gene expression, poly(A)-site selection and poly(A)-length distribution in yeast transcriptomes. Methods Mol Biol. (2019) 2049:141–64. doi: 10.1007/978-1-4939-9736-7_9
107. Simon JM, Paranjape SR, Wolter JM, Salazar G, Zylka MJ. High-throughput screening and classification of chemicals and their effects on neuronal gene expression using RASL-seq. Sci Rep. (2019) 9:4529. doi: 10.1038/s41598-019-39016-5
108. Scolnick JA, Dimon M, Wang IC, Huelga SC, Amorese DA. An efficient method for identifying gene fusions by targeted RNA sequencing from fresh frozen and FFPE samples. PLoS ONE. (2015) 10:e0128916. doi: 10.1371/journal.pone.0128916
109. Otazu IB, Zalcberg I, Tabak DG, Dobbin J, Seuanez HN. Detection of BCR-ABL transcripts by multiplex and nested PCR in different haematological disorders. Leuk Lymphoma. (2000) 37:205–11. doi: 10.3109/10428190009057647
110. Levin JZ, Berger MF, Adiconis X, Rogov P, Melnikov A, Fennell T, et al. Targeted next-generation sequencing of a cancer transcriptome enhances detection of sequence variants and novel fusion transcripts. Genome Biol. (2009) 10:R115. doi: 10.1186/gb-2009-10-10-r115
111. Links MG, Dumonceaux TJ, McCarthy EL, Hemmingsen SM, Topp E, Town JR. CaptureSeq: hybridization-based enrichment of cpn60 gene fragments reveals the community structures of synthetic and natural microbial ecosystems. Microorganisms. (2021) 9:816. doi: 10.3390/microorganisms9040816
112. Portal MM, Pavet V, Erb C, Gronemeyer H. TARDIS a targeted RNA directional sequencing method for rare RNA discovery. Nat Protoc. (2015) 10:1915–38. doi: 10.1038/nprot.2015.120
113. Mamedov IZ, Britanova OV, Zvyagin IV, Turchaninova MA, Bolotin DA, Putintseva EV, et al. Preparing unbiased T-cell receptor and antibody cDNA libraries for the deep next generation sequencing profiling. Front Immunol. (2013) 4:456. doi: 10.3389/fimmu.2013.00456
114. Fan HC, Fu GK, Fodor SP. Expression profiling. Combinatorial labeling of single cells for gene expression cytometry. Science. (2015) 347:1258367. doi: 10.1126/science.1258367
115. Hashimshony T, Senderovich N, Avital G, Klochendler A, de Leeuw Y, Anavy L, et al. CEL-Seq2: sensitive highly-multiplexed single-cell RNA-Seq. Genome Biol. (2016) 17:77. doi: 10.1186/s13059-016-0938-8
116. Natarajan KN. Single-cell tagged reverse transcription (STRT-Seq). Methods Mol Biol. (2019) 1979:133–53. doi: 10.1007/978-1-4939-9240-9_9
117. Klein AM, Macosko E. InDrops and Drop-seq technologies for single-cell sequencing. Lab Chip. (2017) 17:2540–1. doi: 10.1039/C7LC90070H
118. Yang L. Splicing noncoding RNAs from the inside out. Wiley Interdiscip Rev RNA. (2015) 6:651–60. doi: 10.1002/wrna.1307
119. Jiang L, Wang N, Cheng S, Liu Y, Chen S, Wang Y, et al. RNA-sequencing identifies novel transcriptomic signatures in intestinal failure-associated liver disease. J Pediatr Surg. (2021) 2021:S0022-346800846-0. doi: 10.1016/j.jpedsurg.2021.12.015
120. Liu Y, Wu Y, Liu B, Zhang Y, San D, Chen Y, et al. Biomarkers and immune repertoire metrics identified by peripheral blood transcriptomic sequencing reveal the pathogenesis of COVID-19. Front Immunol. (2021) 12:677025. doi: 10.3389/fimmu.2021.677025
121. Alaterre E, Vikova V, Kassambara A, Bruyer A, Robert N, Requirand G, et al. RNA-sequencing-based transcriptomic score with prognostic and theranostic values in multiple myeloma. J Pers Med. (2021) 11:988. doi: 10.3390/jpm11100988
122. Qu R, He K, Fan T, Yang Y, Mai L, Lian Z, et al. Single-cell transcriptomic sequencing analyses of cell heterogeneity during osteogenesis of human adipose-derived mesenchymal stem cells. Stem Cells. (2021) 39:1478–88. doi: 10.1002/stem.3442
123. Piunti A, Shilatifard A. Epigenetic balance of gene expression by Polycomb and COMPASS families. Science. (2016) 352:aad9780. doi: 10.1126/science.aad9780
124. Wang KC, Chang HY. Epigenomics: technologies and applications. Circ Res. (2018) 122:1191–9. doi: 10.1161/CIRCRESAHA.118.310998
125. Li T, Zhang X, Luo F, Wu FX, Wang J. MultiMotifMaker: a multi-thread tool for identifying DNA methylation motifs from pacbio reads. IEEE/ACM Trans Comput Biol Bioinform. (2020) 17:220–5. doi: 10.1109/TCBB.2018.2861399
126. Liu Q, Georgieva DC, Egli D, Wang K. NanoMod: a computational tool to detect DNA modifications using Nanopore long-read sequencing data. BMC Genom. (2019) 20:78. doi: 10.1186/s12864-018-5372-8
127. Pan L, McClain L, Shaw P, Donnellan N, Chu T, Finegold D, et al. Non-invasive epigenomic molecular phenotyping of the human brain via liquid biopsy of cerebrospinal fluid and next generation sequencing. Eur J Neurosci. (2020) 52:4536–45. doi: 10.1111/ejn.14997
128. Euskirchen P, Bielle F, Labreche K, Kloosterman WP, Rosenberg S, Daniau M, et al. Same-day genomic and epigenomic diagnosis of brain tumors using real-time nanopore sequencing. Acta Neuropathol. (2017) 134:691–703. doi: 10.1007/s00401-017-1743-5
129. Farlik M, Sheffield NC, Nuzzo A, Datlinger P, Schonegger A, Klughammer J, et al. Single-cell DNA methylome sequencing and bioinformatic inference of epigenomic cell-state dynamics. Cell Rep. (2015) 10:1386–97. doi: 10.1016/j.celrep.2015.02.001
130. Anreiter I, Mir Q, Simpson JT, Janga SC, Soller M. New twists in detecting mRNA modification dynamics. Trends Biotechnol. (2021) 39:72–89. doi: 10.1016/j.tibtech.2020.06.002
131. Kertesz M, Wan Y, Mazor E, Rinn JL, Nutter RC, Chang HY, et al. Genome-wide measurement of RNA secondary structure in yeast. Nature. (2010) 467:103–7. doi: 10.1038/nature09322
132. Uzilov AV, Underwood JG. High-throughput nuclease probing of RNA structures using FragSeq. Methods Mol Biol. (2016) 1490:105–34. doi: 10.1007/978-1-4939-6433-8_8
133. Kramer MC, Gregory BD. Using protein interaction profile sequencing (PIP-seq) to identify RNA secondary structure and RNA-protein interaction sites of long noncoding RNAs in plants. Methods Mol Biol. (2019) 1933:343–61. doi: 10.1007/978-1-4939-9045-0_21
134. Ritchey LE, Su Z, Assmann SM, Bevilacqua PC. In vivo genome-wide RNA structure probing with structure-seq. Methods Mol Biol. (2019) 1933:305–41. doi: 10.1007/978-1-4939-9045-0_20
135. Umeyama T, Ito T. DMS-seq for in vivo genome-wide mapping of protein-DNA interactions and nucleosome centers. Curr Protoc Mol Biol. (2018) 123:e60. doi: 10.1002/cpmb.60
136. Lin Y, May GE, Joel McManus C. Mod-seq: a high-throughput method for probing RNA secondary structure. Methods Enzymol. (2015) 558:125–52. doi: 10.1016/bs.mie.2015.01.012
137. Incarnato D, Neri F, Anselmi F, Oliviero S. Genome-wide profiling of mouse RNA secondary structures reveals key features of the mammalian transcriptome. Genome Biol. (2014) 15:491. doi: 10.1186/s13059-014-0491-2
138. Guo SK, Nan F, Liu CX, Yang L, Chen LL. Mapping circular RNA structures in living cells by SHAPE-MaP. Methods. (2021) 196:47–55. doi: 10.1016/j.ymeth.2021.01.011
139. Chen L, Chang HY, Artandi SE. Analysis of RNA conformation in endogenously assembled RNPs by icSHAPE. STAR Protoc. (2021) 2:100477. doi: 10.1016/j.xpro.2021.100477
140. Nguyen TC, Cao X, Yu P, Xiao S, Lu J, Biase FH, et al. Mapping RNA-RNA interactome and RNA structure in vivo by MARIO. Nat Commun. (2016) 7:12023. doi: 10.1038/ncomms12023
141. Soller M, Fray R. RNA modifications in gene expression control. Biochim Biophys Acta Gene Regul Mech. (2019) 1862:219–21. doi: 10.1016/j.bbagrm.2019.02.010
142. Galloway A, Cowling VH. mRNA cap regulation in mammalian cell function and fate. Biochim Biophys Acta Gene Regul Mech. (2019) 1862:270–9. doi: 10.1016/j.bbagrm.2018.09.011
143. Li J, Lu Y, Akbani R, Ju Z, Roebuck PL, Liu W, et al. TCPA: a resource for cancer functional proteomics data. Nat Methods. (2013) 10:1046–7. doi: 10.1038/nmeth.2650
144. Chakravarthi BV, Nepal S, Varambally S. Genomic and epigenomic alterations in cancer. Am J Pathol. (2016) 186:1724–35. doi: 10.1016/j.ajpath.2016.02.023
145. Bersani C, Huss M, Giacomello S, Xu LD, Bianchi J, Eriksson S, et al. Genome-wide identification of Wig-1 mRNA targets by RIP-Seq analysis. Oncotarget. (2016) 7:1895–911. doi: 10.18632/oncotarget.6557
146. Cancer Genome Atlas Research N. The molecular taxonomy of primary prostate cancer. Cell. (2015) 163:1011–25. doi: 10.1016/j.cell.2015.10.025
147. Linder B, Grozhik AV, Olarerin-George AO, Meydan C, Mason CE, Jaffrey SR. Single-nucleotide-resolution mapping of m6A and m6Am throughout the transcriptome. Nat Methods. (2015) 12:767–72. doi: 10.1038/nmeth.3453
148. Li X, Xiong X, Zhang M, Wang K, Chen Y, Zhou J, et al. Base-resolution mapping reveals distinct m(1)a methylome in nuclear- and mitochondrial-encoded transcripts. Mol Cell. (2017) 68:993–1005 e9. doi: 10.1016/j.molcel.2017.10.019
149. Zhang LS, Liu C, Ma H, Dai Q, Sun HL, Luo G, et al. Transcriptome-wide mapping of internal N(7)-methylguanosine methylome in mammalian mRNA. Mol Cell. (2019) 74:1304–16 e8. doi: 10.1016/j.molcel.2019.03.036
150. Molinie B, Wang J, Lim KS, Hillebrand R, Lu ZX, Van Wittenberghe N, et al. m(6)A-LAIC-seq reveals the census and complexity of the m(6)A epitranscriptome. Nat Methods. (2016) 13:692–8. doi: 10.1038/nmeth.3898
151. Amort T, Rieder D, Wille A, Khokhlova-Cubberley D, Riml C, Trixl L, et al. Distinct 5-methylcytosine profiles in poly(A) RNA from mouse embryonic stem cells and brain. Genome Biol. (2017) 18:1. doi: 10.1186/s13059-016-1139-1
152. Squires JE, Patel HR, Nousch M, Sibbritt T, Humphreys DT, Parker BJ, et al. Widespread occurrence of 5-methylcytosine in human coding and non-coding RNA. Nucleic Acids Res. (2012) 40:5023–33. doi: 10.1093/nar/gks144
153. Yang X, Yang Y, Sun BF, Chen YS, Xu JW, Lai WY, et al. 5-methylcytosine promotes mRNA export - NSUN2 as the methyltransferase and ALYREF as an m(5)C reader. Cell Res. (2017) 27:606–25. doi: 10.1038/cr.2017.55
154. Garcia-Campos MA, Edelheit S, Toth U, Safra M, Shachar R, Viukov S, et al. Deciphering the “m(6)A Code” via antibody-independent quantitative profiling. Cell. (2019) 178:731–47 e16. doi: 10.1016/j.cell.2019.06.013
155. Zhang Z, Chen LQ, Zhao YL, Yang CG, Roundtree IA, Zhang Z, et al. Single-base mapping of m(6)A by an antibody-independent method. Sci Adv. (2019) 5:eaax0250. doi: 10.1126/sciadv.aax0250
156. Pichot F, Marchand V, Helm M, Motorin Y. Non-redundant tRNA reference sequences for deep sequencing analysis of tRNA abundance and epitranscriptomic RNA modifications. Genes. (2021) 12:81. doi: 10.3390/genes12010081
157. Torma G, Tombacz D, Moldovan N, Fulop A, Prazsak I, Csabai Z, et al. Dual isoform sequencing reveals complex transcriptomic and epitranscriptomic landscapes of a prototype baculovirus. Sci Rep. (2022) 12:1291. doi: 10.1038/s41598-022-05457-8
158. Sridhar B, Rivas-Astroza M, Nguyen TC, Chen W, Yan Z, Cao X, et al. Systematic mapping of RNA-chromatin interactions in vivo. Curr Biol. (2017) 27:610–2. doi: 10.1016/j.cub.2017.01.068
159. Li X, Zhou B, Chen L, Gou LT, Li H, Fu XD. GRID-seq reveals the global RNA-chromatin interactome. Nat Biotechnol. (2017) 35:940–50. doi: 10.1038/nbt.3968
160. Engreitz JM, Pandya-Jones A, McDonel P, Shishkin A, Sirokman K, Surka C, et al. The Xist lncRNA exploits three-dimensional genome architecture to spread across the X chromosome. Science. (2013) 341:1237973. doi: 10.1126/science.1237973
161. Colak D, Zaninovic N, Cohen MS, Rosenwaks Z, Yang WY, Gerhardt J, et al. Promoter-bound trinucleotide repeat mRNA drives epigenetic silencing in fragile X syndrome. Science. (2014) 343:1002–5. doi: 10.1126/science.1245831
162. Miao Y, Ajami NE, Huang TS, Lin FM, Lou CH, Wang YT, et al. Enhancer-associated long non-coding RNA LEENE regulates endothelial nitric oxide synthase and endothelial function. Nat Commun. (2018) 9:292. doi: 10.1038/s41467-017-02113-y
163. Chu C, Qu K, Zhong FL, Artandi SE, Chang HY. Genomic maps of long noncoding RNA occupancy reveal principles of RNA-chromatin interactions. Mol Cell. (2011) 44:667–78. doi: 10.1016/j.molcel.2011.08.027
164. Simon MD, Wang CI, Kharchenko PV, West JA, Chapman BA, Alekseyenko AA, et al. The genomic binding sites of a noncoding RNA. Proc Natl Acad Sci USA. (2011) 108:20497–502. doi: 10.1073/pnas.1113536108
165. Bell JC, Jukam D, Teran NA, Risca VI, Smith OK, Johnson WL, et al. Chromatin-associated RNA sequencing (ChAR-seq) maps genome-wide RNA-to-DNA contacts. Elife. (2018) 7:e27024. doi: 10.7554/eLife.27024.037
166. Schlüter A, Rodríguez-Palmero A, Verdura E, Vélez-Santamaría V, Ruiz M, Fourcade S, et al. Diagnosis of genetic white matter disorders by singleton whole-exome and genome sequencing using interactome-driven prioritization. Neurology. (2022) 98:e912–e23. doi: 10.1212/WNL.0000000000013278
167. Kudla G, Granneman S, Hahn D, Beggs JD, Tollervey D. Cross-linking, ligation, and sequencing of hybrids reveals RNA-RNA interactions in yeast. Proc Natl Acad Sci USA. (2011) 108:10010–5. doi: 10.1073/pnas.1017386108
168. Bohnsack MT, Tollervey D, Granneman S. Identification of RNA helicase target sites by UV cross-linking and analysis of cDNA. Methods Enzymol. (2012) 511:275–88. doi: 10.1016/B978-0-12-396546-2.00013-9
169. Sugimoto Y, Vigilante A, Darbo E, Zirra A, Militti C, D'Ambrogio A, et al. hiCLIP reveals the in vivo atlas of mRNA secondary structures recognized by Staufen 1. Nature. (2015) 519:491–4. doi: 10.1038/nature14280
170. Helwak A, Kudla G, Dudnakova T, Tollervey D. Mapping the human miRNA interactome by CLASH reveals frequent noncanonical binding. Cell. (2013) 153:654–65. doi: 10.1016/j.cell.2013.03.043
171. Lu Z, Zhang QC, Lee B, Flynn RA, Smith MA, Robinson JT, et al. RNA duplex map in living cells reveals higher-order transcriptome structure. Cell. (2016) 165:1267–79. doi: 10.1016/j.cell.2016.04.028
172. Aw JG, Shen Y, Wilm A, Sun M, Lim XN, Boon KL, et al. In vivo mapping of eukaryotic rna interactomes reveals principles of higher-order organization and regulation. Mol Cell. (2016) 62:603–17. doi: 10.1016/j.molcel.2016.04.028
173. Sharma E, Sterne-Weiler T, O'Hanlon D, Blencowe BJ. Global mapping of human RNA-RNA interactions. Mol Cell. (2016) 62:618–26. doi: 10.1016/j.molcel.2016.04.030
174. Olabi M, Stein M, Watzig H. Affinity capillary electrophoresis for studying interactions in life sciences. Methods. (2018) 146:76–92. doi: 10.1016/j.ymeth.2018.05.006
175. Hawe JS, Theis FJ, Heinig M. Inferring interaction networks from multi-omics data. Front Genet. (2019) 10:535. doi: 10.3389/fgene.2019.00535
176. Funk CC, Casella AM, Jung S, Richards MA, Rodriguez A, Shannon P, et al. Atlas of transcription factor binding sites from ENCODE DNase hypersensitivity data across 27 tissue types. Cell Rep. (2020) 32:108029. doi: 10.1016/j.celrep.2020.108029
177. Kruger A, Zimbres FM, Kronenberger T, Wrenger C. Molecular modeling applied to nucleic acid-based molecule development. Biomolecules. (2018) 8:83. doi: 10.3390/biom8030083
178. Bulyk ML, Gentalen E, Lockhart DJ, Church GM. Quantifying DNA-protein interactions by double-stranded DNA arrays. Nat Biotechnol. (1999) 17:573–7. doi: 10.1038/9878
179. Jung C, Hawkins JA, Jones SK Jr, Xiao Y, Rybarski JR, Dillard KE, et al. Massively parallel biophysical analysis of CRISPR-Cas complexes on next generation sequencing chips. Cell. (2017) 170:35–47 e13. doi: 10.1016/j.cell.2017.05.044
180. Ogawa N, Biggin MD. High-throughput SELEX determination of DNA sequences bound by transcription factors in vitro. Methods Mol Biol. (2012) 786:51–63. doi: 10.1007/978-1-61779-292-2_3
181. Lee TI, Johnstone SE, Young RA. Chromatin immunoprecipitation and microarray-based analysis of protein location. Nat Protoc. (2006) 1:729–48. doi: 10.1038/nprot.2006.98
182. Gao H, Zhao C. Analysis of protein-DNA interaction by chromatin immunoprecipitation and DNA tiling microarray (ChIP-on-chip). Methods Mol Biol. (2018) 1689:43–51. doi: 10.1007/978-1-4939-7380-4_4
183. Hafner M, Landthaler M, Burger L, Khorshid M, Hausser J, Berninger P, et al. PAR-CliP–a method to identify transcriptome-wide the binding sites of RNA binding proteins. J Vis Exp. (2010) 41:2034. doi: 10.3791/2034
184. Huppertz I, Attig J, D'Ambrogio A, Easton LE, Sibley CR, Sugimoto Y, et al. iCLIP: protein-RNA interactions at nucleotide resolution. Methods. (2014) 65:274–87. doi: 10.1016/j.ymeth.2013.10.011
185. Granneman S, Kudla G, Petfalski E, Tollervey D. Identification of protein binding sites on U3 snoRNA and pre-rRNA by UV cross-linking and high-throughput analysis of cDNAs. Proc Natl Acad Sci USA. (2009) 106:9613–8. doi: 10.1073/pnas.0901997106
186. Zhao J, Luo R, Xu X, Zou Y, Zhang Q, Pan W. High-throughput sequencing of RNAs isolated by cross-linking immunoprecipitation (HITS-CLIP) reveals Argonaute-associated microRNAs and targets in Schistosoma japonicum. Parasit Vectors. (2015) 8:589. doi: 10.1186/s13071-015-1203-9
187. Guil S, Caceres JF. The multifunctional RNA-binding protein hnRNP A1 is required for processing of miR-18a. Nat Struct Mol Biol. (2007) 14:591–6. doi: 10.1038/nsmb1250
188. Xue Y, Ouyang K, Huang J, Zhou Y, Ouyang H, Li H, et al. Direct conversion of fibroblasts to neurons by reprogramming PTB-regulated microRNA circuits. Cell. (2013) 152:82–96. doi: 10.1016/j.cell.2012.11.045
189. Saldana-Meyer R, Gonzalez-Buendia E, Guerrero G, Narendra V, Bonasio R, Recillas-Targa F, et al. CTCF regulates the human p53 gene through direct interaction with its natural antisense transcript, Wrap53. Genes Dev. (2014) 28:723–34. doi: 10.1101/gad.236869.113
190. Memczak S, Jens M, Elefsinioti A, Torti F, Krueger J, Rybak A, et al. Circular RNAs are a large class of animal RNAs with regulatory potency. Nature. (2013) 495:333–8. doi: 10.1038/nature11928
191. Darnell RB. HITS-CLIP panoramic views of protein-RNA regulation in living cells. Wiley Interdiscip Rev RNA. (2010) 1:266–86. doi: 10.1002/wrna.31
192. Richard P, Horgan LCK. ‘Omic' technologies: genomics, transcriptomics, proteomics and metabolomics. Obstetr Gynaecol. (2011) 13:189–95. doi: 10.1576/toag.13.3.189.27672
193. Petricoin EF, Zoon KC, Kohn EC, Barrett JC, Liotta LA. Clinical proteomics: translating benchside promise into bedside reality. Nat Rev Drug Discov. (2002) 1:683–95. doi: 10.1038/nrd891
194. Wilhelm M, Schlegl J, Hahne H, Gholami AM, Lieberenz M, Savitski MM, et al. Mass-spectrometry-based draft of the human proteome. Nature. (2014) 509:582–7. doi: 10.1038/nature13319
195. Price P. Standard definitions of terms relating to mass spectrometry: a report from the committee on measurements and standards of the American society for mass spectrometry. J Am Soc Mass Spectrom. (1991) 2:336–48. doi: 10.1016/1044-0305(91)80025-3
196. Coon JJ, Syka JE, Shabanowitz J, Hunt DF. Tandem mass spectrometry for peptide and protein sequence analysis. Biotechniques. (2005) 38:519. doi: 10.2144/05384TE01
197. Zubarev RA, Horn DM, Fridriksson EK, Kelleher NL, Kruger NA, Lewis MA, et al. Electron capture dissociation for structural characterization of multiply charged protein cations. Anal Chem. (2000) 72:563–73. doi: 10.1021/ac990811p
198. Lioe H, O'Hair RA. Comparison of collision-induced dissociation and electron-induced dissociation of singly protonated aromatic amino acids, cystine and related simple peptides using a hybrid linear ion trap-FT-ICR mass spectrometer. Anal Bioanal Chem. (2007) 389:1429–37. doi: 10.1007/s00216-007-1535-1
199. Syka JE, Coon JJ, Schroeder MJ, Shabanowitz J, Hunt DF. Peptide and protein sequence analysis by electron transfer dissociation mass spectrometry. Proc Natl Acad Sci USA. (2004) 101:9528–33. doi: 10.1073/pnas.0402700101
200. Guan S, Marshall AG, Wahl MC. MS/MS with high detection efficiency and mass resolving power for product ions in Fourier transform ion cyclotron resonance mass spectrometry. Anal Chem. (1994) 66:1363–7. doi: 10.1021/ac00080a024
201. Hernandez P, Muller M, Appel RD. Automated protein identification by tandem mass spectrometry: issues and strategies. Mass Spectrom Rev. (2006) 25:235–54. doi: 10.1002/mas.20068
202. Bidlingmaier S, Ha K, Lee NK, Su Y, Liu B. Proteome-wide identification of novel ceramide-binding proteins by yeast surface cDNA display and deep sequencing. Mol Cell Proteomics. (2016) 15:1232–45. doi: 10.1074/mcp.M115.055954
203. Zhang B, Li B, Men XH, Xu ZW, Wu H, Qin XT, et al. Proteome sequencing and analysis of Ophiocordyceps sinensis at different culture periods. BMC Genom. (2020) 21:886. doi: 10.1186/s12864-020-07298-z
204. Gilly A, Park YC, Png G, Barysenka A, Fischer I, Bjornland T, et al. Whole-genome sequencing analysis of the cardiometabolic proteome. Nat Commun. (2020) 11:6336. doi: 10.1038/s41467-020-20079-2
205. Neto V, Esteves-Ferreira S, Inacio I, Alves M, Dantas R, Almeida I, et al. Metabolic profile characterization of different thyroid nodules using FTIR spectroscopy: a review. Metabolites. (2022) 12:53. doi: 10.3390/metabo12010053
206. Lin D, Wang Y, Wang T, Zhu Y, Lin X, Lin Y, et al. Metabolite profiling of human blood by surface-enhanced Raman spectroscopy for surgery assessment and tumor screening in breast cancer. Anal Bioanal Chem. (2020) 412:1611–8. doi: 10.1007/s00216-020-02391-4
207. Scott HD, Buchan M, Chadwick C, Field CJ, Letourneau N, Montina T, et al. Metabolic dysfunction in pregnancy: Fingerprinting the maternal metabolome using proton nuclear magnetic resonance spectroscopy. Endocrinol Diabetes Metab. (2021) 4:e00201. doi: 10.1002/edm2.201
208. Ceglarek U, Leichtle A, Brugel M, Kortz L, Brauer R, Bresler K, et al. Challenges and developments in tandem mass spectrometry based clinical metabolomics. Mol Cell Endocrinol. (2009) 301:266–71. doi: 10.1016/j.mce.2008.10.013
209. Carriot N, Paix B, Greff S, Viguier B, Briand JF, Culioli G. Integration of LC/MS-based molecular networking and classical phytochemical approach allows in-depth annotation of the metabolome of non-model organisms - The case study of the brown seaweed Taonia atomaria. Talanta. (2021) 225:121925. doi: 10.1016/j.talanta.2020.121925
210. Taya N, Katakami N, Omori K, Arakawa S, Hosoe S, Watanabe H, et al. Evaluation of change in metabolome caused by comprehensive diabetes treatment: a prospective observational study of diabetes inpatients with gas chromatography/mass spectrometry-based non-target metabolomic analysis. J Diabetes Investig. (2021) 12:2232–41. doi: 10.1111/jdi.13600
211. Hayashi Y, Komatsu T, Iwashita K, Fukusaki E. (1)H-NMR metabolomics-based classification of Japanese sake and comparative metabolome analysis by gas chromatography-mass spectrometry. J Biosci Bioeng. (2021) 131:557–64. doi: 10.1016/j.jbiosc.2020.12.008
212. Bogdanov B, Smith RD. Proteomics by FTICR mass spectrometry: top down and bottom up. Mass Spectrom Rev. (2005) 24:168–200. doi: 10.1002/mas.20015
213. Samadi A, Theodoridou K, Yu P. Detect the sensitivity and response of protein molecular structure of whole canola seed (yellow and brown) to different heat processing methods and relation to protein utilization and availability using ATR-FT/IR molecular spectroscopy with chemometrics. Spectrochim Acta A Mol Biomol Spectrosc. (2013) 105:304–13. doi: 10.1016/j.saa.2012.11.096
214. Pelletier MJ. Sensitivity-enhanced transmission Raman spectroscopy. Appl Spectrosc. (2013) 67:829–40. doi: 10.1366/13-07115
215. Emwas AH. The strengths and weaknesses of NMR spectroscopy and mass spectrometry with particular focus on metabolomics research. Methods Mol Biol. (2015) 1277:161–93. doi: 10.1007/978-1-4939-2377-9_13
216. Johnson CH, Ivanisevic J, Siuzdak G. Metabolomics: beyond biomarkers and towards mechanisms. Nat Rev Mol Cell Biol. (2016) 17:451–9. doi: 10.1038/nrm.2016.25
217. Kim SJ, Song HE, Lee HY, Yoo HJ. Mass spectrometry-based metabolomics in translational research. Adv Exp Med Biol. (2021) 1310:509–31. doi: 10.1007/978-981-33-6064-8_19
218. Meng X, Xu J, Zhang M, Du R, Zhao W, Zeng Q, et al. Third-generation sequencing and metabolome analysis reveal candidate genes and metabolites with altered levels in albino jackfruit seedlings. BMC Genom. (2021) 22:543. doi: 10.1186/s12864-021-07873-y
219. Geng QS, Liu RJ, Shen ZB, Wei Q, Zheng YY, Jia LQ, et al. Transcriptome sequencing and metabolome analysis reveal the mechanism of Shuanghua Baihe Tablet in the treatment of oral mucositis. Chin J Nat Med. (2021) 19:930–43. doi: 10.1016/S1875-5364(22)60150-X
220. Wang J, Alvin Chew BL, Lai Y, Dong H, Xu L, Balamkundu S, et al. Quantifying the RNA cap epitranscriptome reveals novel caps in cellular and viral RNA. Nucleic Acids Res. (2019) 47:e130. doi: 10.1093/nar/gkz751
221. Zhang XQ, Yang JH. Decoding the atlas of RNA modifications from epitranscriptome sequencing data. Methods Mol Biol. (2019) 1870:107–24. doi: 10.1007/978-1-4939-8808-2_8
222. Wei Z, Panneerdoss S, Timilsina S, Zhu J, Mohammad TA, Lu ZL, et al. Topological characterization of human and mouse m(5)C epitranscriptome revealed by bisulfite sequencing. Int J Genomics. (2018) 2018:1351964. doi: 10.1155/2018/1351964
223. Borg M, Jiang D, Berger F. Histone variants take center stage in shaping the epigenome. Curr Opin Plant Biol. (2021) 61:101991. doi: 10.1016/j.pbi.2020.101991
224. Onder O, Sidoli S, Carroll M, Garcia BA. Progress in epigenetic histone modification analysis by mass spectrometry for clinical investigations. Expert Rev Proteom. (2015) 12:499–517. doi: 10.1586/14789450.2015.1084231
225. Palumbo AM, Smith SA, Kalcic CL, Dantus M, Stemmer PM, Reid GE. Tandem mass spectrometry strategies for phosphoproteome analysis. Mass Spectrom Rev. (2011) 30:600–25. doi: 10.1002/mas.20310
226. Muehlbauer LK, Hebert AS, Westphall MS, Shishkova E, Coon JJ. Global phosphoproteome analysis using high-field asymmetric waveform ion mobility spectrometry on a hybrid orbitrap mass spectrometer. Anal Chem. (2020) 92:15959–67. doi: 10.1021/acs.analchem.0c03415
227. Cheng LC Li Z, Graeber TG, Graham NA, Drake JM. Phosphopeptide enrichment coupled with label-free quantitative mass spectrometry to investigate the phosphoproteome in prostate cancer. J Vis Exp. (2018) 138:57996. doi: 10.3791/57996
228. Zhao X, Zhang W, Liu T, Dong H, Huang J, Sun C, et al. A fast sample processing strategy for large-scale profiling of human urine phosphoproteome by mass spectrometry. Talanta. (2018) 185:166–73. doi: 10.1016/j.talanta.2018.03.047
229. Hutchinson EC, Denham EM, Thomas B, Trudgian DC, Hester SS, Ridlova G, et al. Mapping the phosphoproteome of influenza A and B viruses by mass spectrometry. PLoS Pathog. (2012) 8:e1002993. doi: 10.1371/journal.ppat.1002993
230. Jones AW, Flynn HR, Uhlmann F, Snijders AP, Touati SA. Assessing budding yeast phosphoproteome dynamics in a time-resolved manner using TMT10plex mass tag labeling. STAR Protoc. (2020) 1:100022. doi: 10.1016/j.xpro.2020.100022
231. Kaur S, Baldi B, Vuong J, O'Donoghue SI. Visualization and analysis of epiproteome dynamics. J Mol Biol. (2019) 431:1519–39. doi: 10.1016/j.jmb.2019.01.044
232. Uzasci L, Auh S, Cotter RJ, Nath A. Mass spectrometric phosphoproteome analysis of HIV-infected brain reveals novel phosphorylation sites and differential phosphorylation patterns. Proteomics Clin Appl. (2016) 10:126–35. doi: 10.1002/prca.201400134
233. Fields S, Song O. A novel genetic system to detect protein-protein interactions. Nature. (1989) 340:245–6. doi: 10.1038/340245a0
234. Garcia A, Senis YA, Antrobus R, Hughes CE, Dwek RA, Watson SP, et al. A global proteomics approach identifies novel phosphorylated signaling proteins in GPVI-activated platelets: involvement of G6f, a novel platelet Grb2-binding membrane adapter. Proteomics. (2006) 6:5332–43. doi: 10.1002/pmic.200600299
235. Yugandhar K, Gupta S, Yu H. Inferring protein-protein interaction networks from mass spectrometry-based proteomic approaches: a mini-review. Comput Struct Biotechnol J. (2019) 17:805–11. doi: 10.1016/j.csbj.2019.05.007
236. Chasapis CT. Building bridges between structural and network-based systems biology. Mol Biotechnol. (2019) 61:221–9. doi: 10.1007/s12033-018-0146-8
237. Bader GD, Hogue CW. Analyzing yeast protein-protein interaction data obtained from different sources. Nat Biotechnol. (2002) 20:991–7. doi: 10.1038/nbt1002-991
238. Pourhaghighi R, Ash PEA, Phanse S, Goebels F, Hu LZM, Chen S, et al. BraInMap elucidates the macromolecular connectivity landscape of mammalian brain. Cell Syst. (2020) 10:333–50 e14. doi: 10.1016/j.cels.2020.08.006
239. Hunt AC, Vögeli B, Kightlinger WK, Yoesep DJ, Krüger A, Jewett MC, et al. High-throughput, automated, cell-free expression and screening platform for antibody discovery. bioRxiv. (2021) 31:392–403. doi: 10.1101/2021.11.04.467378
240. Veenstra TD. Omics in systems biology: current progress and future outlook. Proteomics. (2021) 21:e2000235. doi: 10.1002/pmic.202000235
241. Milo R. What is the total number of protein molecules per cell volume? A call to rethink some published values. Bioessays. (2013) 35:1050–5. doi: 10.1002/bies.201300066
242. Bennett BD, Kimball EH, Gao M, Osterhout R, Van Dien SJ, Rabinowitz JD. Absolute metabolite concentrations and implied enzyme active site occupancy in Escherichia coli. Nat Chem Biol. (2009) 5:593–9. doi: 10.1038/nchembio.186
243. Milanesi R, Coccetti P, Tripodi F. The regulatory role of key metabolites in the control of cell signaling. Biomolecules. (2020) 10:862. doi: 10.3390/biom10060862
244. Niphakis MJ, Lum KM, Cognetta AB 3rd, Correia BE, Ichu TA, Olucha J, et al. A global map of lipid-binding proteins and their ligandability in cells. Cell. (2015) 161:1668–80. doi: 10.1016/j.cell.2015.05.045
245. Gallego O, Betts MJ, Gvozdenovic-Jeremic J, Maeda K, Matetzki C, Aguilar-Gurrieri C, et al. A systematic screen for protein-lipid interactions in Saccharomyces cerevisiae. Mol Syst Biol. (2010) 6:430. doi: 10.1038/msb.2010.87
246. Li X, Gianoulis TA, Yip KY, Gerstein M, Snyder M. Extensive in vivo metabolite-protein interactions revealed by large-scale systematic analyses. Cell. (2010) 143:639–50. doi: 10.1016/j.cell.2010.09.048
247. Piazza I, Kochanowski K, Cappelletti V, Fuhrer T, Noor E, Sauer U, et al. A map of protein-metabolite interactions reveals principles of chemical communication. Cell. (2018) 172:358–72 e23. doi: 10.1016/j.cell.2017.12.006
248. Diether M, Nikolaev Y, Allain FH, Sauer U. Systematic mapping of protein-metabolite interactions in central metabolism of Escherichia coli. Mol Syst Biol. (2019) 15:e9008. doi: 10.15252/msb.20199008
249. Klysik J. Concept of immunomics: a new frontier in the battle for gene function? Acta Biotheor. (2001) 49:191–202. doi: 10.1023/A:1011901410166
250. De Sousa KP, Doolan DL. Immunomics: a 21st century approach to vaccine development for complex pathogens. Parasitology. (2016) 143:236–44. doi: 10.1017/S0031182015001079
251. Sette A, Fleri W, Peters B, Sathiamurthy M, Bui HH, Wilson S, et al. roadmap for the immunomics of category A-C pathogens. Immunity. (2005) 22:155–61. doi: 10.1016/j.immuni.2005.01.009
252. Basharat Z, Yasmin A, Masood N. Cancer immunomics in the age of information: role in diagnostics and beyond. Curr Pharm Des. (2018) 24:3818–28. doi: 10.2174/1381612824666181106091903
253. Marchesi JR, Ravel J. The vocabulary of microbiome research: a proposal. Microbiome. (2015) 3:31. doi: 10.1186/s40168-015-0094-5
254. Kumar PS. Microbiomics: were we all wrong before? Periodontology. (2021) 85:8–11. doi: 10.1111/prd.12373
255. Hawkins AK, O'Doherty KC. “Who owns your poop?”: insights regarding the intersection of human microbiome research and the ELSI aspects of biobanking and related studies. BMC Med Genom. (2011) 4:72. doi: 10.1186/1755-8794-4-72
256. Chen T, Yu WH, Izard J, Baranova OV, Lakshmanan A, Dewhirst FE. The Human Oral Microbiome Database: a web accessible resource for investigating oral microbe taxonomic and genomic information. Database. (2010) 2010:baq013. doi: 10.1093/database/baq013
257. Grice EA, Segre JA. The human microbiome: our second genome. Annu Rev Genomics Hum Genet. (2012) 13:151–70. doi: 10.1146/annurev-genom-090711-163814
258. Franzosa EA, Huang K, Meadow JF, Gevers D, Lemon KP, Bohannan BJ, et al. Identifying personal microbiomes using metagenomic codes. Proc Natl Acad Sci USA. (2015) 112:E2930–8. doi: 10.1073/pnas.1423854112
259. Di Tommaso P, Chatzou M, Floden EW, Barja PP, Palumbo E, Notredame C. Nextflow enables reproducible computational workflows. Nat Biotechnol. (2017) 35:316–9. doi: 10.1038/nbt.3820
260. Bobo D, Lipatov M, Rodriguez-Flores JL, Auton A, Henn BM. False negatives are a significant feature of next generation sequencing callsets. bioRxiv. (2016) 2016:066043. doi: 10.1101/066043
261. Sheynkman GM, Shortreed MR, Cesnik AJ, Smith LM. Proteogenomics: integrating next-generation sequencing and mass spectrometry to characterize human proteomic variation. Annu Rev Anal Chem. (2016) 9:521–45. doi: 10.1146/annurev-anchem-071015-041722
262. Berg RD. The indigenous gastrointestinal microflora. Trends Microbiol. (1996) 4:430–5. doi: 10.1016/0966-842X(96)10057-3
263. Dai X, Wang D, Zhang J. Programmed cell death, redox imbalance, and cancer therapeutics. Apoptosis. (2021) 26:385–414. doi: 10.1007/s10495-021-01682-0
264. Petronek MS, Stolwijk JM, Murray SD, Steinbach EJ, Zakharia Y, Buettner GR, et al. Utilization of redox modulating small molecules that selectively act as pro-oxidants in cancer cells to open a therapeutic window for improving cancer therapy. Redox Biol. (2021) 42:101864. doi: 10.1016/j.redox.2021.101864
265. Kim B, Lee KJ. Activation of Nm23-H1 to suppress breast cancer metastasis via redox regulation. Exp Mol Med. (2021) 53:346–57. doi: 10.1038/s12276-021-00575-1
266. Rudyk O, Aaronson PI. Redox regulation, oxidative stress, and inflammation in group 3 pulmonary hypertension. Adv Exp Med Biol. (2021) 1303:209–41. doi: 10.1007/978-3-030-63046-1_13
267. Dorrestein PC, Carroll KS. 'Omics' of natural products and redox biology. Curr Opin Chem Biol. (2011) 15:3–4. doi: 10.1016/j.cbpa.2011.01.009
268. Leonard SE, Carroll KS. Chemical 'omics' approaches for understanding protein cysteine oxidation in biology. Curr Opin Chem Biol. (2011) 15:88–102. doi: 10.1016/j.cbpa.2010.11.012
269. Jacob C, Jamier V, Ba LA. Redox active secondary metabolites. Curr Opin Chem Biol. (2011) 15:149–55. doi: 10.1016/j.cbpa.2010.10.015
270. Jones DP, Go YM. Mapping the cysteine proteome: analysis of redox-sensing thiols. Curr Opin Chem Biol. (2011) 15:103–12. doi: 10.1016/j.cbpa.2010.12.014
271. Chouchani ET, James AM, Fearnley IM, Lilley KS, Murphy MP. Proteomic approaches to the characterization of protein thiol modification. Curr Opin Chem Biol. (2011) 15:120–8. doi: 10.1016/j.cbpa.2010.11.003
272. Hansen RE, Winther JR. An introduction to methods for analyzing thiols and disulfides: Reactions, reagents, and practical considerations. Anal Biochem. (2009) 394:147–58. doi: 10.1016/j.ab.2009.07.051
273. Lewis SM, Asselin-Labat ML, Nguyen Q, Berthelet J, Tan X, Wimmer VC, et al. Spatial omics and multiplexed imaging to explore cancer biology. Nat Methods. (2021) 18:997–1012. doi: 10.1038/s41592-021-01203-6
274. Weber C. Single-cell spatial transcriptomics. Nat Cell Biol. (2021) 23:1108. doi: 10.1038/s41556-021-00778-8
275. Tang L. Multiomics sequencing goes spatial. Nat Methods. (2021) 18:31. doi: 10.1038/s41592-020-01043-w
276. Tomczak K, Czerwinska P, Wiznerowicz M. The Cancer Genome Atlas (TCGA): an immeasurable source of knowledge. Contemp Oncol. (2015) 19:A68–77. doi: 10.5114/wo.2014.47136
277. Barrett T, Wilhite SE, Ledoux P, Evangelista C, Kim IF, Tomashevsky M, et al. NCBI GEO: archive for functional genomics data sets–update. Nucleic Acids Res. (2013) 41:D991-5. doi: 10.1093/nar/gks1193
278. Wang Y, Song F, Zhu J, Zhang S, Yang Y, Chen T, et al. GSA: genome sequence archive. Genom Proteom Bioinformat. (2017) 15:14–8. doi: 10.1016/j.gpb.2017.01.001
279. Zhang SS, Chen X, Chen TT, Zhu JW, Tang BX, Wang AK, et al. GSA-human: genome sequence archive for human. Yi Chuan. (2021) 43:988–93.
280. Wu WS, Wu HY, Wang PH, Chen TY, Chen KR, Chang CW, et al. LCMD: lung cancer metabolome database. Comput Struct Biotechnol J. (2022) 20:65–78. doi: 10.1016/j.csbj.2021.12.002
281. Pei J, Grishin NV. The DBSAV database: predicting deleteriousness of single amino acid variations in the human proteome. J Mol Biol. (2021) 433:166915. doi: 10.1016/j.jmb.2021.166915
282. Alsulami AF, Thomas SE, Jamasb AR, Beaudoin CA, Moghul I, Bannerman B, et al. SARS-CoV-2 3D database: understanding the coronavirus proteome and evaluating possible drug targets. Brief Bioinform. (2021) 22:769–80. doi: 10.1093/bib/bbaa404
283. Kaushik AC, Mehmood A, Upadhyay AK, Paul S, Srivastava S, Mali P, et al. CytoMegaloVirus infection database: a public omics database for systematic and comparable information of CMV. Interdiscip Sci. (2020) 12:169–77. doi: 10.1007/s12539-019-00350-x
284. Kaushik AC, Mehmood A, Dai X, Wei DQ. WeiBI (web-based platform): enriching integrated interaction network with increased coverage and functional proteins from genome-wide experimental OMICS data. Sci Rep. (2020) 10:5618. doi: 10.1038/s41598-020-62508-8
285. Chu Y, Shan X, Chen T, Jiang M, Wang Y, Wang Q, et al. DTI-MLCD: predicting drug-target interactions using multi-label learning with community detection method. Brief Bioinform. (2021) 22:bbaa205. doi: 10.1093/bib/bbaa205
Keywords: omics, next generation sequencing, third generation sequencing, mass spectrometry, redoxomics
Citation: Dai X and Shen L (2022) Advances and Trends in Omics Technology Development. Front. Med. 9:911861. doi: 10.3389/fmed.2022.911861
Received: 03 April 2022; Accepted: 09 May 2022;
Published: 01 July 2022.
Edited by:
Roberto Gramignoli, Karolinska Institutet (KI), SwedenReviewed by:
Hector Quezada, Hospital Infantil de México Federico Gómez, MexicoCopyright © 2022 Dai and Shen. This is an open-access article distributed under the terms of the Creative Commons Attribution License (CC BY). The use, distribution or reproduction in other forums is permitted, provided the original author(s) and the copyright owner(s) are credited and that the original publication in this journal is cited, in accordance with accepted academic practice. No use, distribution or reproduction is permitted which does not comply with these terms.
*Correspondence: Xiaofeng Dai, eGlhb2ZlbmcuZGFpQGppYW5nbmFuLmVkdS5jbg==
Disclaimer: All claims expressed in this article are solely those of the authors and do not necessarily represent those of their affiliated organizations, or those of the publisher, the editors and the reviewers. Any product that may be evaluated in this article or claim that may be made by its manufacturer is not guaranteed or endorsed by the publisher.
Research integrity at Frontiers
Learn more about the work of our research integrity team to safeguard the quality of each article we publish.