- 1División Académica de Ciencias de la Salud, Universidad Juárez Autónoma de Tabasco, Villahermosa, Mexico
- 2Centro de Investigación en Nutrición y Salud, Instituto Nacional de Salud Pública, Cuernavaca, Mexico
- 3Centro de Investigación en Evaluación y Encuestas, Instituto Nacional de Salud Pública, Cuernavaca, Mexico
Background: Chronic kidney disease (CKD) is a major public health problem, with considerable growth in prevalence and mortality in recent years. Screening of CKD at primary care is crucial for the implementation of prevention strategies. The aims of this study are to assess CKD risk prediction scores and to develop a risk prediction score for the Mexican adult population.
Methods: Data from the Mexican National Health and Nutrition Survey 2016 was utilized and 3463 participants ≥ 20 years old were included. Reduced renal function with Glomerular filtration rate and/or the presence of albuminuria was defined as CKD. Multiple logistic regression models were performed for the creation of a training and validation model. Additionally, several models were validated in our Mexican population.
Results: The developed training model included sex, age, body mass index, fast plasma glucose, systolic blood pressure, and triglycerides, as did the validation model. The area under the curve (AUC) was 0.78 (95% CI: 0.72, 0.79) for training model, and 0.76 (95% CI: 0.71, 0.80) in validation model for Mexican adult population. Age, female gender, presence of diabetes and hypertension, elevated systolic and diastolic blood pressure, serum and urinary creatinine, and higher HbA1c were significantly associated with the prevalent chronic kidney disease. Previous CKD risk predictive models were evaluated with a representative sample of the Mexican adult population, their AUC was between 0.61 and 0.78.
Conclusion: The designed CKD risk predictive model satisfactorily predicts using simple and common variables in primary medical care. This model could have multiple benefits; such as, the identification of the population at risk, and prevention of CKD.
Background
Chronic kidney disease (CKD) is a major public health problem associated with major adverse health events (e.g., greater cognitive impairment, higher prevalence of anemia, hypertension, and metabolic bone disease), progression to kidney failure, and death (1, 2).
Worldwide, in 2017, the prevalence of CKD was 9.1% (95% uncertainty interval [UI] 8.5–9.8), which was roughly 700 million cases. There were 7.3 million (95% UI 5.4–9.2) years of healthy life lost due to disability (YLDs), 28.5 million (95% IU 27.6–29.3) years of life lost (YLLs) and 35.8 million (95% IU 33.7–38.0) disability-adjusted life years (DALYs). There were 1.2 million (95% IU 1.2–1.3) deaths as a result of CKD. In addition, 1.4 million (95% UI 1.2–1.6) deaths from cardiovascular disease (CVD) were attributable to impaired kidney function (7.6% of deaths from CVD) (1, 3). For Mexico, in 2017, the prevalence of CKD was 12.2% (14.5 million cases) (1), 210.9 thousand YLDs, 1.5 million YLLs, 1.7 million DALYs, and 51.4 deaths per 100,000 inhabitants (65 thousand deaths); thus, made this disease the second leading cause of death in that year. From 1990 to 2017, CKD mortality rate increased by 102.3% (2).
Excessive growth in prevalence has been related to accelerated demographic and epidemiological changes (4), such as the high prevalence of Type 2 diabetes (T2D) (15.7%) (5), hypertension (49.4%) (6), overweight, and obesity (75.2%) (7), which are the main risk factors for developing non-communicable diseases and also contribute to deaths from CKD, accounting for half of these deaths.
Up to 98% of people with CKD due to T2D in Mexico are in stages one to three where the disease process can be delayed and controlled, while 2% will require complex and expensive treatments such as peritoneal dialysis, hemodialysis and/or kidney transplantation as replacement and restitutive therapies to survive (stages 4 and 5, considered irreversible) (8). In Mexico CKD is having a significant impact on the finances of the institutions and family economy. In 2014, the average annual health expenditure per person for this disease was US$ 8,966 in the Ministry of Health, and US$ 9,091 in the Mexican Institute of Social Security (9).
Therefore, the first level of medical care is crucial for the implementation of primary prevention strategies aimed at the early and timely control of cardiovascular risk factors, strategies for a stricter control of glycemia and blood pressure, promotion of healthy eating habits, health education, rationalization of the use of potentially nephrotoxic drugs and preventive treatment of hyperfiltration (10). One of the strategies for the timely CKD diagnosis is CKD risk predictive scores, these should be simple but precise and the variables included should be easily accessible in routine clinical settings (11).
The use of risk scores holds promise for large-scale CKD risk stratification and would allow the identification of all segments of the population that would benefit from CKD detection. To date, several studies have shown CKD risk predictive scores, and the predictive capacity of the scores ranges between 0.63 and 0.91 area under the receiver operating characteristic curve (AUROC). However, none have been evaluated in the healthy Mexican population.
Thus, the aims are: (1) to assess previous designed CKD risk prediction scores in Mexican adult population, (2) to develop a training and validation risk prediction score based on the Mexican National Health and Nutrition Survey 2016 (ENSANUT-MC 2016, by its acronym in Spanish).
Materials and methods
Design and study population
Data from ENSANUT-MC 2016 was used, a cross-sectional, multistage, stratified, and clustered probabilistic sample of the Mexican population, with national, regional, and urban-rural representation. Detailed survey’s design, sample size calculation and methodology were previously described (12, 13).
For the current study, individuals with at least 8 h of fasting at blood sample collection time were included. In addition, complete serum, urinary biomarker data, and complete survey.
Sociodemographic variables
This variables and risk factors included were based on self-reported information in the applied survey, such as age, sex, education level (Illiterate, elementary school, high school, and bachelor’s degree), socioeconomic level, place of residence (rural or urban), indigenism and region (center, Mexico City, south and north). Trained and standardized personnel applied the interviews.
Clinical variables
The trained personnel performed two blood pressure measurements with 30 s difference between each one, with 5 min of rest before the first measurement with the patient seated, an automatic device (Omron HEM-907 XL) was used and the mean of the two measurements was chosen (14). The mean of the two measurements was chosen. Hypertension was considered according to the new criteria of the ISH 2020: systolic blood pressure (SBP) was 140 mmHg or higher and/or diastolic blood pressure (DBP) was 90 mmHg or higher, or when hypertension was self-reported (15). History of kidney stone was measured by self-reporting kidney stone.
Biochemical variables
Prediabetes was considered when fasting serum glucose was between 100 mg/dl – 125 mg/dl or HbA1c between 5.6 and 6.4%, T2D when fasting serum glucose was 126 mg/dl or greater, or HbA1c was 6.5% or greater, or T2D was self-reported, or use of glucose lowering drugs, and low control of T2D when HbA1c was higher than 7% (16).
The homeostasis model assessment-estimated insulin resistance (HOMA-IR) was used to calculate insulin resistance, it was calculated multiplying fasting plasma insulin (FPI) by fasting plasma glucose (FPG), then dividing by the constant 22.5 [HOMA-IR = (FPI × FPG)/22.5]. The cut-off point (17) to define insulin resistance was higher than 3.80.
Urine albumin was measured with the immunoturbidimetric assay. Urine creatinine was measured using the isotope dilution mass spectrometry (IDMS) standardized methodology, and serum creatinine was measured with the Jaffe method (13). Urinary albumin-creatinine ratio (UACR) was computed and reported in milligrams per gram. Albuminuria was considered when the UACR was 30 mg/g or higher.
Definition of chronic kidney disease
Reduced renal function [estimated glomerular filtration rate (eGFR) less than 60 mL/min/1.73 m2] and/or the presence of albuminuria (UACR) was 30 mg/g or higher were used to define CKD (18).
Glomerular filtration rate (GFR) was estimated using Chronic Kidney Disease Epidemiology Collaboration (CKD-EPI) equation (19).
For this equation: Scr: serum creatinine (mg/dL), κ: 0.7 for females and 0.9 for males, α: –0.329 for females and –0.411 for males, min indicates the minimum of Scr/κ or 1, and max indicates the maximum of Scr/κ or 1.
Anthropometric variables
Trained personnel measured height, weight, and waist circumference for all participants. The body mass index (BMI) was calculated from the data of height (m2) and weight (kg), and the criteria of the World Health Organization (WHO) was used to classify underweight (<18.5), normal weight (18.5–24.9), overweight (25–29.9) and obesity (higher than 30.0). We define abdominal obesity as; 88 cm or higher waist circumference for men and 102 cm or higher for women (20).
Chronic kidney disease risk predictive scores
After a review of the literature, scores that had AUROC greater than 0.70 were selected, and included variables to be applied in the first level of medical care, shown in Table 3.
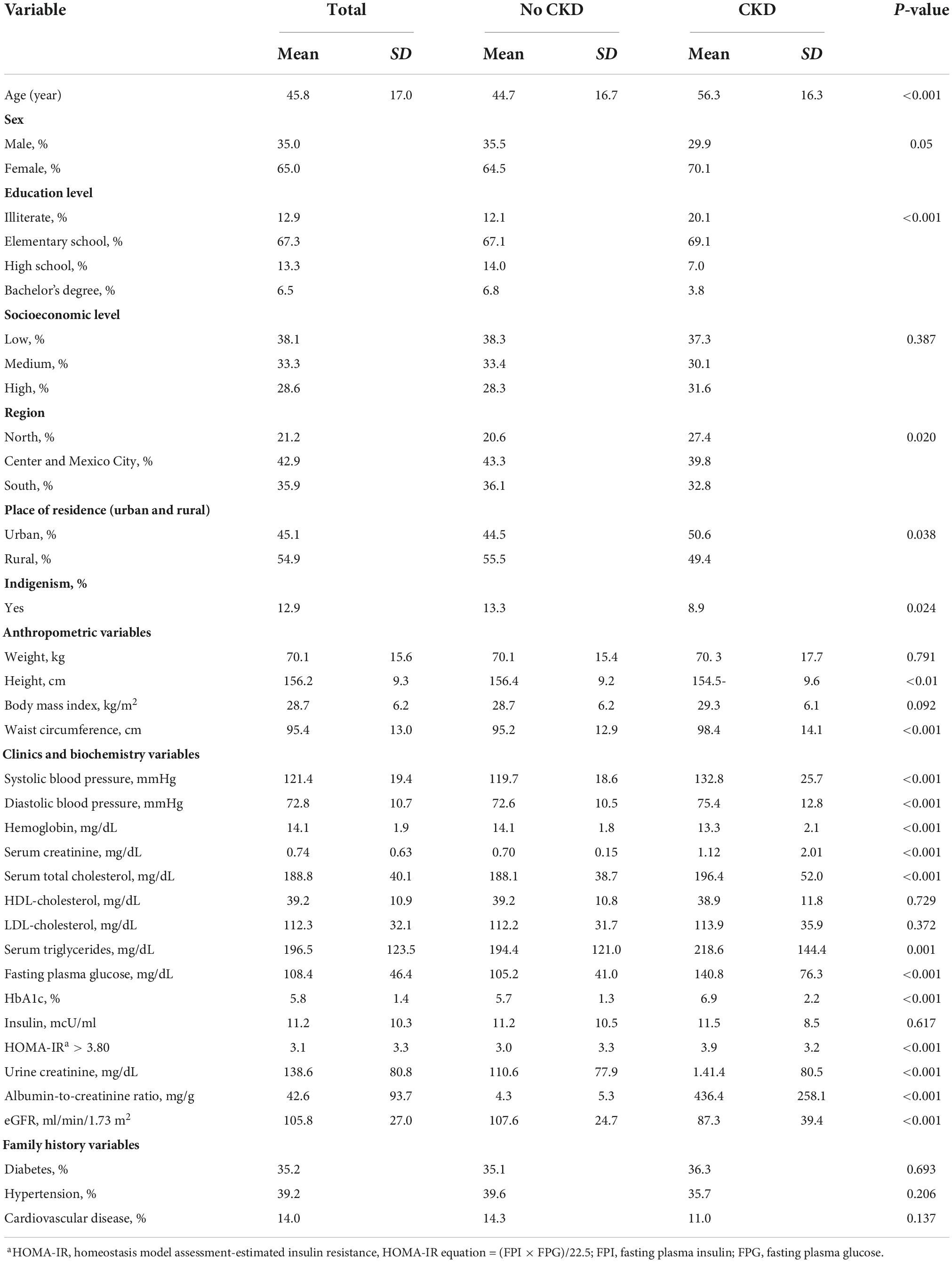
Table 1. Main characteristics of Mexican National Health and Nutrition Survey 2016, according to the presence of chronic kidney disease.
For the creation of the novel prediction score the TRIPOD guidelines were followed (Verification Checklist Supplementary material).
Statistical analysis
Participant’s characteristics are described in percentages if categorical, or in mean and standard deviation if numerical and are compared between subjects with and without CKD using a t-test if numerical or using χ2; test if categorical.
Multivariable logistic regression models were fitted to assess how each risk factor contributes to the probability of developing CKD. The data was split into training and validation on an 80/20 ratio. The training dataset was used to fit the predictors on the outcome and the latter to provide an unbiased evaluation of the final models fitted on the validation dataset.
In the training dataset, we fitted different logistic regression models including CKD as the outcome of interest and combinations of age, sex, fasting plasma glucose, SBP, triglycerides, and BMI as predictors. These variables were chosen because we considered them as the most clinically relevant features in order to predict CKD. We selected the model to build our proposed score using the Akaike Information Criterion (AIC).
For training and internal validation of the risk prediction equations among the testing dataset, the AUROC was used, which measures how well the model differentiates those individuals at higher risk of having an event from those at lower risk, a property known as discrimination. Hosmer–Lemeshow χ2 tests were also calculated to compare the predicted number of events with the number of events seen. All analyses were done with Stata for windows version 13.0.
Results
Main characteristics of the study population
A total of 3,463 subjects from ENSANUT 2016 MC were included in the final analysis to derive the CKD risk prediction scores (Figure 1). The main baseline characteristics of participants, sociodemographic, clinical, and biochemical, according to the CKD presence or absence, are shown in Table 1. The mean age was 45.8 years, 65.0% were females, 67.3% had elementary school, and 54.9% lived in a rural area. According to anthropometric and clinical measures, those with CKD had a higher mean of BMI compared to those without CKD (29.3 vs. 28.7 kg/m2), similar results were observed in the mean of waist circumference (98.4 vs. 95.2 cm), SBP (132.8 vs. 119.7 mmHg), DBP (75.4 vs. 72.6 mmHg), serum creatinine (1.12 vs. 0.70 mg/dL), total serum cholesterol (196.4 vs. 188.1 mg/dL), serum triglycerides (218.6 vs. 194.4 mg/dL), and FPG (140.8 vs. 105.2 mg/dL).
Most of the participants without CKD were overweight (39.7%), while in those with CKD, obesity (39.1%) and abdominal obesity (86.4%) predominated. 14.6% of the participants without CKD lived with T2D, 24.1% lived with hypertension and 23.2% had insulin resistance, while in those with CKD the prevalence was 44.0, 56.6, and 38.1%, respectively (Table 2).
Assessment of chronic kidney disease risk predictive models
Chronic kidney disease risk predictive models (21–27) were selected to be assessed with a representative sample of the Mexican adult population, characteristics are shown in Table 3 and the AUROC of the models in Figure 2. The external validation of the different predictive models performed in the Mexican adult population, the AUROC of Kwon et al. model (21) was 0.75; while, in the O’Seaghdha et al. clinical models (22) were 0.74, 0.76, and 0.77 for models 1, 2, and 3, respectively. Additionally, the external validation using Al-Shamsi et al. models (23) showed an AUROC of 0.78 for the first model, and 0.76 the second model. Finally, Lee et al. (24) for model 3 included sex, BMI, level of education, FPG, serum albumin, eGFR and proteinuria, with an AUROC of 0.77 and for model 4 the same variables were included plus Framingham risk score, with an AUROC of 0.77.
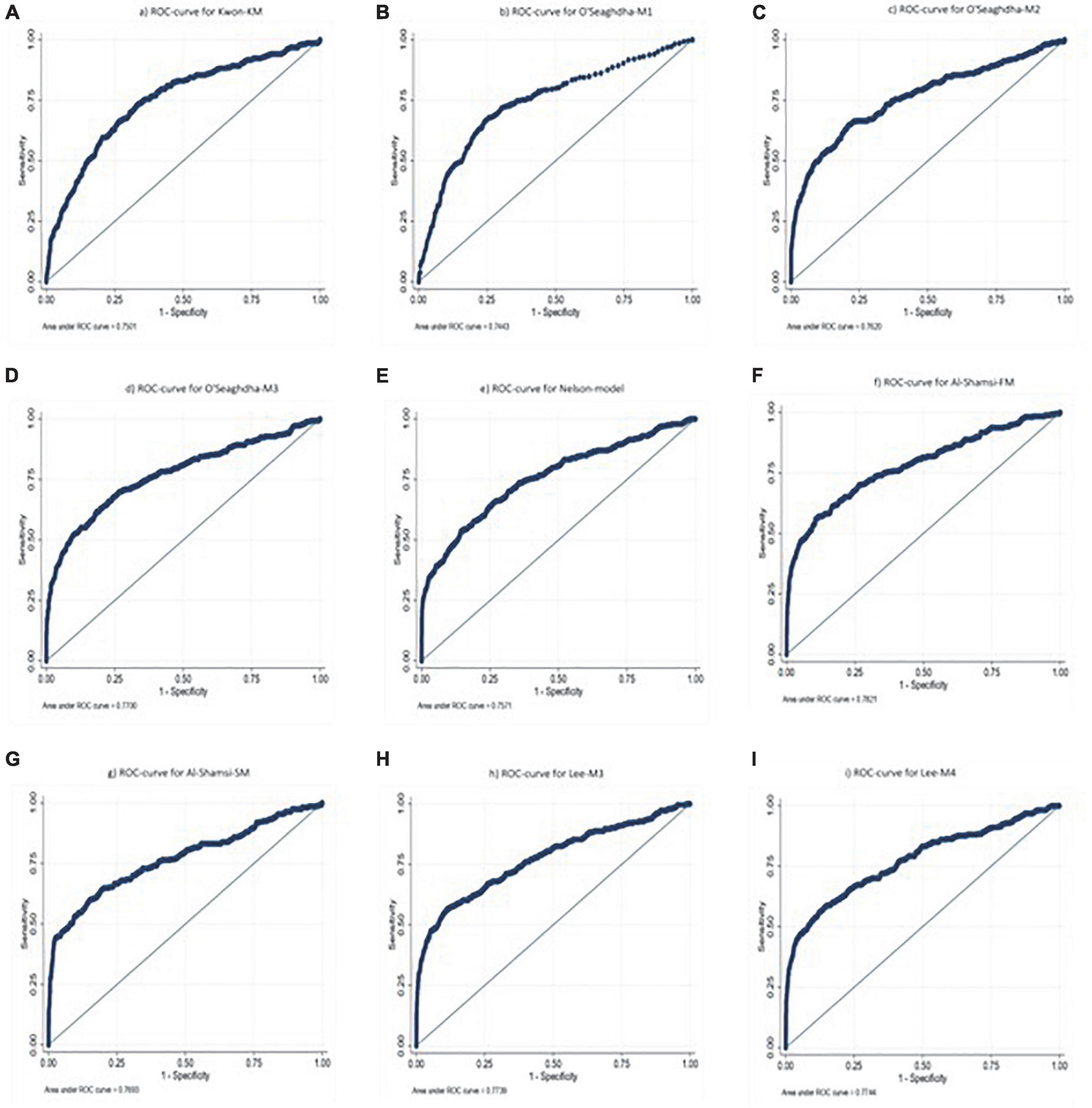
Figure 2. Area under the curve of risk predictive models of chronic kidney disease previously described in the Mexican population, using data of the National Health and Nutrition Survey 2016: (A) Kwon Korean model, (B) O’Seaghdha model 1, (C) O’Seaghdha model 2, (D) O’Seaghdha model 3, (E) Nelson model, (F) Al-Shamsi full model, (G) Al-Shamsi stepwise model, (H) Lee model 3, and (I) Lee model 4.
Development of a chronic kidney disease risk predictive model
A model to predict the CKD presence in Mexican adult population was computed. In this sense, the training (1) and validation model (2) included age, sex, BMI, FPG, SBP, and triglycerides. The AUROC for the validation model was 0.76 (95% CI: 0.71, 0.80) and for the training model was 0.78 (95% CI: 0.72, 0.79) (Table 4).
Chronic kidney disease risk prediction algorithms
Prediction equations for training and validation models for CKD are presented below:
Training model:
Validation model:
*β values taken from ORs reported on Table 4
Discussion
A predictive risk model was designed for the Mexican adult population using data from the ENSANUT 2016 and it was observed that sex, age, BMI, FPG, SBP, and triglycerides variables predict the CKD risk with the 0.78 AUROC value.
Kwon et al. (21), Al-Shamsi et al. (23), and Lee et al. (24), as the study showed that CKD patients were older, prevalence was higher in T2D and hypertension, mean was higher in serum creatinine and HbA1c, compared to patients without CKD. Two studies (21, 24) reported that the mean total cholesterol, triglycerides and FPG were higher in those with CKD, as was found in this study’s population. The CKD population in Kwon et al. (21) and this study’s, present a higher mean waist circumference and a lower mean hemoglobin compared to those without CKD. For their part, Lee et al. (24) showed that elevated SBP and DBP was significant in CKD patients, as in this study, but not in Al-Shamsi et al. (23). Only this study evaluated HOMA-IR, the mean was 3.0 in patients without CKD and 3.9 in those with CKD, 38.1% of the patients with CKD had insulin resistance.
External validation of all CKD risk predictive models (21–27) was carried out with a representative sample of the Mexican National Health and Nutrition Survey, all of them were population-based, except Al-Shamsi et al. which was clinic-based. The CKD risk predictive models had a fair to good AUROC in their population; however, when replicating these models in our population, the predictive capacity was diminished.
These authors agree with Echouffo-Tcheugui et al. (28) that strategies for early identification and treatment of people with CKD are needed worldwide, and although the model equations incorporate several risk factors that are independently associated with the occurrence of CKD, these should be easily evaluable in routine clinical settings. In this sense, this study’s model for the Mexican adult population was developed with variables which are easily available at the first medical care level. The validation model included sex, age, BMI, FPG, SBP, and triglycerides.
In agreement with the previous studies (21–27), older age, and presence of T2D and hypertension are the main risk factors for developing CKD in stages 3–5. This study’s model uses variables which has been previously used in other predictive models, i.e., age, sex, T2D, and hypertension.
To our knowledge, the present study is the first to assess CKD risk predictive models in an apparently healthy Mexican adult population. The AUROC for this study’s training model was 0.78 and for the validation model was 0.76, compared to Kwon et al. model (21), the AUROC was 0.87 in its primary population and 0.75 in the external validation. O’Seaghdha et al. (22) developed three models, AUROC was between 0.78 and 0.81 in its population, 0.76 in its external validation, and in our validation, it was between 0.74 and 0.77. The AUROC in Al-shamsi et al. (23) two models was 0.90 (multivariate full model) and 0.92 (multivariate stepwise model). They performed very well in their population, however, in the Mexican adult population AUROC was 0.78 and 0.76, respectively. Stepwise model had a lower AUROC than full model, this may be because the stepwise model includes eGFR, T2D, Cholesterol, and HbA1c, but not age, hypertension, triglycerides, and CVD variables, which does include full model and were more significant in Mexican adult population. Finally, Nelson et al. (25) used the CKD Prognosis Consortium (PC), which includes study cohorts from around the world, collecting more than five million patients, and developed two CKD predictive models, one for patients with T2D and the other without T2D. The median C statistic was 0.84 in the cohort without T2D and in his study’s validation, AUROC was 0.75.
It was observed that CKD risk predictive models are characterized by including the main risk factors for developing CKD (older age, T2D, and hypertension); in addition to renal variables (eGFR, proteinuria, albuminuria) that usually improve predictive capacity, and other variables (dyslipidemia, CVD, BMI, sex, etc.). It was considered that the inclusion or not of this group of variables can increase or decrease the predictive capacity depending on the population because they are not always significant.
The objective of creating CKD risk predictive models is to prevent, applied mainly in populations with risk factors for CKD susceptibility, initiation, or progression. The prevalence of this disease has been increasing in Mexico and Latin America, for this reason the need to evaluate CKD risk predictive models, to be used in Mexican adult population and facilitate surveillance of groups susceptible to risk for developing CKD (8).
There are numerous strengths to this study, including the representative based sample, rigorous and detailed assessment of risk factors including measures of renal function and proteinuria. The parsimonious list of variables in the final model is also a significant strength, enhancing the score’s utility and applicability. Some limitations should also be acknowledged. A very high coefficient of variation in creatinine concentrations was observed because creatinine was measured on a single occasion; however, multiple measurements in cross-sectional studies are not feasible. Furthermore, eGFR was estimated using the CKD-EPI equation, which may underestimate eGFR in both healthy individuals and those with CKD. However, a comparison of definitions of incident CKD in the setting of epidemiological research demonstrates that the present definition is the most sensitive (29), which is desirable in view of the potential application of the risk score for population screening. Finally, the cross-sectional design of the study.
Conclusion
The aims of this study were to evaluate different CKD risk predictive models in the Mexican adult population and develop our predictive risk model. The models evaluated showed a fair to good predictive capacity, however, adjusted in the Mexican adult population, this predictive capacity was diminished. The study’s model is a reliable tool for predict CKD risk among apparently healthy population. It was observed that the variables sex, age, BMI, FPG, SBP, and triglycerides satisfactorily predict the CKD risk, these variables are simple and common in the primary care attention. So, this model could help physicians to identify population at risk. The implementation of CKD risk predictive models will allow the prevention and control of CKD, applied in populations with risk factors for susceptibility, initiation, or progression of CKD. Communication and awareness of the risk to patients is the first step for prevention, it could motivate them to improve their lifestyle and adhere to prescribed therapies. Prevention by identifying patients at risk could also have an economic benefit in our health care system.
Data availability statement
The datasets used and/or analyzed during the current study are available from the corresponding author on reasonable request.
Ethics statement
The ENSANUT-MC 2016 protocols were approved by the research, ethics and biosafety committees of the National Institute of Public Health, strictly adhering to the principles set forth in the Declaration of Helsinki. The voluntary nature of participation was recorded in the informed consent and assent forms. The patients/participants provided their written informed consent to participate in this study.
Author contributions
VC wrote the manuscript (Introduction, Results, and Discussion). AG-R wrote the manuscript (Materials and methods and Discussion). DC contributed the analysis tools. CH-A conceived and designed the analysis. AP wrote the manuscript (Materials and methods) and contributed the data. MP-C contributed the analysis tools. SB conceived and designed the analysis. ED-G conceived and designed the analysis, contributed the analysis tools, and performed the analysis. All authors contributed to the article and approved the submitted version.
Funding
This study was supported by the CONACYT-PRONASE-SALUD study (project number 2256, CI:1767).
Acknowledgments
We would like to thank the survey’s team from the National Institute of Public Health for the elaboration of ENSANUT 2016 survey and database.
Conflict of interest
The authors declare that the research was conducted in the absence of any commercial or financial relationships that could be construed as a potential conflict of interest.
Publisher’s note
All claims expressed in this article are solely those of the authors and do not necessarily represent those of their affiliated organizations, or those of the publisher, the editors and the reviewers. Any product that may be evaluated in this article, or claim that may be made by its manufacturer, is not guaranteed or endorsed by the publisher.
Supplementary material
The Supplementary Material for this article can be found online at: https://www.frontiersin.org/articles/10.3389/fmed.2022.903090/full#supplementary-material
Abbreviations
AIC, Akaike information criterion; AUROC, area under the receiver operating characteristic curve; BMI, body mass index; CKD, chronic kidney disease; CKD-EPI, chronic kidney disease epidemiology collaboration; CVD, cardiovascular disease; DALYs, disability-adjusted life years; DBP, diastolic blood pressure; eGFR, estimated glomerular filtration rate; ENSANUT, National Health and Nutrition Survey; FPG, fasting plasma glucose; FPI, fasting plasma insulin; HOMA-IR, homeostasis model assessment-estimated insulin resistance; IDMS, isotope dilution mass spectrometry; SBP, systolic blood pressure; T2D, type 2 diabetes; UACR, urinary albumin-creatinine ratio; WHO, World Health Organization; YLDs, years of healthy life lost due to disability; YLLs, years of life lost.
References
1. GBD Chronic Kidney Disease Collaboration. Global, regional, and national burden of chronic kidney disease, 1990–2017: a systematic analysis for the global burden of disease study 2017. Lancet. (2020) 395:709–33. doi: 10.1016/S0140-6736(20)30045-3
2. Agudelo-Botero M, Valdez-Ortiz R, Giraldo-Rodríguez L, González-Robledo MC, Mino-León D, Rosales-Herrera MF, et al. Overview of the burden of chronic kidney disease in Mexico: secondary data analysis based on the global burden of disease study 2017. BMJ Open. (2020) 10:e035285. doi: 10.1136/bmjopen-2019-035285
3. Cockwell P, Fisher L-A. The global burden of chronic kidney disease. Lancet. (2020) 395:662–4. doi: 10.1016/S0140-6736(19)32977-0
4. Gómez-Dantés H, Fullman N, Lamadrid-Figueroa H, Cahuana-Hurtado L, Darney B, Avila-Burgos L, et al. Dissonant health transition in the states of Mexico, 1990–2013: a systematic analysis for the global burden of disease study 2013. Lancet. (2016) 388:2386–402. doi: 10.1016/S0140-6736(16)31773-1
5. Basto-Abreu A, López-Olmedo N, Rojas-Martínez R, Aguilar-Salinas CA, De la Cruz-Góngora V, Rivera-Dommarco J, et al. Prevalence of diabetes and glycemic control in Mexico: national results from 2018 and 2020. Salud Publica Mex. (2021) 63:725–33. doi: 10.21149/12842
6. Campos-Nonato I, Hernández-Barrera L, Oviedo-Solís C, Ramírez-Villalobos D, Hernández B, Barquera S. Epidemiología de la hipertensión arterial en adultos mexicanos: diagnóstico, control y tendencias. Ensanut 2020. Salud Publica Mex. (2021) 63:692–704. doi: 10.21149/12851
7. INSP.Encuesta Nacional de Salud y Nutrición 2018 - Presentación de resultados. (2020). Available online at: https://ensanut.insp.mx/encuestas/ensanut2018/doctos/informes/ensanut_2018_presentacion_resultados.pdf [accessed on Jul 2021, 31].
8. Tamayo y Orozco J, Lastiri Quirós HS. La enfermedad renal crónica en México. Hacia una politica nacional para enfrentarla. Intersistemas. México: Academia Nacional de Medicina de México (2016) 82.
9. Figueroa-Lara A, Angel Gonzalez-Block M, Alarcon-Irigoyen J. Medical expenditure for chronic diseases in Mexico: the case of selected diagnoses treated by the largest care providers. PLoS One. (2016) 11:e0145177. doi: 10.1371/journal.pone.0145177
10. Aldrete-Velasco JA, Chiquete E, Rodríguez-García JA, Rincón-Pedrero R, Correa-Rotter R, Peña-García R, et al. Mortalidad por enfermedad renal crónica y su relación con la diabetes en México. Med Interna Mex. (2018) 34:536–50.
11. Ávila-Saldivar MN. Enfermedad renal cronica: prevencion y deteccion temprana en el primer nivel de atencion. Med Interna Méx. (2013) 29:148–53.
12. Romero-Martínez M, Shamah-Levy T, Cuevas-Nasu L, Gómez-Humarán IM, Gaona-Pineda EB, Gómez-Acosta LM, et al. Diseño metodológico de la encuesta nacional de salud y nutrición de medio camino 2016. Salud Publica Mex. (2017) 59:299–305. doi: 10.21149/8593
13. Hernández-Alcaraz C, Shamah-Levy T, Romero-Martínez M, Sepúlveda-Amor J, Aguilar-Salinas CA, Rivera-Dommarco J, et al. Submuestra para el análisis de enfermedades crónicas con biomarcadores, encuesta nacional de salud y nutrición 2016. Salud Publica Mex. (2020) 62:504–10. doi: 10.21149/11306
14. Campos-Nonato I, Hernández-Barrera L, Pedroza-Tobías A, Medina C, Barquera S. Hypertension in Mexican adults: prevalence, diagnosis and type of treatment. Ensanut MC 2016. Salud Publica Mex. (2018) 60:233–43. doi: 10.21149/8813
15. Unger T, Borghi C, Charchar F, Khan NA, Poulter NR, Prabhakaran D, et al. 2020 international society of hypertension global hypertension practice guidelines. Hypertension. (2020) 75:1334–57.
16. American Diabetes Association. Classification and diagnosis of diabetes: standards of medical care in diabetes—2018. Diabetes Care. (2018) 41(Suppl 1.):S13–27.
17. Qu HQ, Li Q, Rentfro AR, Fisher-Hoch SP, McCormick JB. The definition of insulin resistance using HOMA-IR for Americans of Mexican descent using machine learning. PLoS One. (2011) 6:e21041. doi: 10.1371/journal.pone.0021041
18. Levey AS, Eckardt K-U, Dorman NM, Christiansen SL, Hoorn EJ, Ingelfinger JR, et al. Nomenclature for kidney function and disease: report of a kidney disease: improving global outcomes (KDIGO) consensus conference. Kidney Int. (2020) 97:1117–29. doi: 10.1016/j.kint.2020.02.010
19. Arreola-Guerra J, Rincón-Pedrero R, Cruz-Rivera C, Belmont-Pérez T, Correa-Rotter R, Niño-Cruz J. Performance of MDRD-IDMS and CKD-EPI equations in Mexican individuals with normal renal function. Nefrologia. (2014) 34:591–8. doi: 10.3265/Nefrologia.pre2014.jun.12538
20. Alberti KGMM, Eckel RH, Grundy SM, Zimmet PZ, Cleeman JI, Donato KA, et al. Harmonizing the metabolic syndrome. Circulation. (2009) 120:1640–5.
21. Kwon KS, Bang H, Bomback AS, Koh DH, Yum JH, Lee JH, et al. A simple prediction score for kidney disease in the Korean population. Nephrology. (2012) 17:278–84. doi: 10.1111/j.1440-1797.2011.01552.x
22. O’Seaghdha CM, Lyass A, Massaro JM, Meigs JB, Coresh J, D’Agostino R, et al. A risk score for chronic kidney disease in the general population. Am J Med. (2012) 125:270–7. doi: 10.1016/j.amjmed.2011.09.009
23. Al-Shamsi S, Oulhaj A, Regmi D, Govender RD. Use of estimated glomerular filtration rate to predict incident chronic kidney disease in patients at risk of cardiovascular disease: a retrospective study. BMC Nephrol. (2019) 20:325. doi: 10.1186/s12882-019-1494-8
24. Lee C, Yun HR, Joo YS, Lee S, Kim J, Nam KH, et al. Framingham risk score and risk of incident chronic kidney disease: a community-based prospective cohort study. Kidney Res Clin Pract. (2019) 38:49–59. doi: 10.23876/j.krcp.18.0118
25. Nelson RG, Grams ME, Ballew SH, Sang Y, Azizi F, Chadban SJ, et al. Development of risk prediction equations for incident chronic kidney disease. JAMA J Am Med Assoc. (2019) 322:2104–14. doi: 10.1001/jama.2019.17379
26. Wen J, Hao J, Zhang Y, Cao K, Zhang X, Li J, et al. Risk scores for predicting incident chronic kidney disease among rural Chinese people: a village-based cohort study. BMC Nephrol. (2020) 21:120. doi: 10.1186/s12882-020-01787-9
27. Saranburut K, Vathesatogkit P, Thongmung N, Chittamma A, Vanavanan S, Tangstheanphan T, et al. Risk scores to predict decreased glomerular filtration rate at 10 years in an Asian general population. BMC Nephrol. (2017) 18:240. doi: 10.1186/s12882-017-0653-z
28. Echouffo-Tcheugui JB, Kengne AP. Risk models to predict chronic kidney disease and its progression: a systematic review. Remuzzi G, editor. PLoS Med. (2012) 9:e1001344. doi: 10.1371/journal.pmed.1001344
Keywords: risk score, chronic kidney disease, Mexican, prediction, validation
Citation: Colli VA, González-Rocha A, Canales D, Hernández-Alcáraz C, Pedroza A, Pérez-Chan M, Barquera S and Denova-Gutierrez E (2022) Chronic kidney disease risk prediction scores assessment and development in Mexican adult population. Front. Med. 9:903090. doi: 10.3389/fmed.2022.903090
Received: 23 March 2022; Accepted: 07 October 2022;
Published: 20 October 2022.
Edited by:
Kathrin Eller, Medical University of Graz, AustriaReviewed by:
Zeid Khitan, Marshall University, United StatesNeftali Eduardo Antonio-Villa, Universidad Nacional Autónoma de México, Mexico
Copyright © 2022 Colli, González-Rocha, Canales, Hernández-Alcáraz, Pedroza, Pérez-Chan, Barquera and Denova-Gutierrez. This is an open-access article distributed under the terms of the Creative Commons Attribution License (CC BY). The use, distribution or reproduction in other forums is permitted, provided the original author(s) and the copyright owner(s) are credited and that the original publication in this journal is cited, in accordance with accepted academic practice. No use, distribution or reproduction is permitted which does not comply with these terms.
*Correspondence: Edgar Denova-Gutierrez, ZWRnYXIuZGVub3ZhQGluc3AubXg=