- 1Department of Nephrology, First Teaching Hospital of Tianjin University of Traditional Chinese Medicine, National Clinical Research Center for Chinese Medicine Acupuncture and Moxibustion, Tianjin, China
- 2Qilu Hospital, Shandong University, Shandong, China
- 3Institute of Traditional Chinese Medicine, Tianjin University of Traditional Chinese Medicine, Tianjin, China
- 4College of Animal Science and National Engineering Research Center for Breeding Swine Industry, South China Agricultural University, Guangzhou, Guangdong, China
- 5School of Pharmacy, Jiangxi University of Traditional Chinese Medicine, Nanchang, China
The global epidemic has been controlled to some extent, while sporadic outbreaks still occur in some places. It is essential to summarize the successful experience and promote the development of new drugs. This study aimed to explore the common mechanism of action of the four Chinese patent medicine (CPMs) recommended in the Medical Observation Period COVID-19 Diagnostic and Treatment Protocol and to accelerate the new drug development process. Firstly, the active ingredients and targets of the four CPMs were obtained by the Chinese medicine composition database (TCMSP, TCMID) and related literature, and the common action targets of the four TCMs were sorted out. Secondly, the targets of COVID-19 were obtained through the gene-disease database (GeneCards, NCBI). Then the Venn diagram was used to intersect the common drug targets with the disease targets. And GO and KEGG pathway functional enrichment analysis was performed on the intersected targets with the help of the R package. Finally, the results were further validated by molecular docking and molecular dynamics analysis. As a result, a total of 101 common active ingredients and 21 key active ingredients of four CPMs were obtained, including quercetin, luteolin, acacetin, kaempferol, baicalein, naringenin, artemisinin, aloe-emodin, which might be medicinal substances for the treatment of COVID-19. TNF, IL6, IL1B, CXCL8, CCL2, IL2, IL4, ICAM1, IFNG, and IL10 has been predicted as key targets. 397 GO biological functions and 166 KEGG signaling pathways were obtained. The former was mainly enriched in regulating apoptosis, inflammatory response, and T cell activation. The latter, with 92 entries related to COVID-19, was mainly enriched to signaling pathways such as Coronavirus disease—COVID-19, Cytokine-cytokine receptor interaction, IL-17 signaling pathway, and Toll-like receptor signaling pathway. Molecular docking results showed that 19/21 of key active ingredients exhibited strong binding activity to recognized COVID-19-related targets (3CL of SARS-CoV-2, ACE2, and S protein), even better than one of these four antiviral drugs. Among them, shinflavanone had better affinity to 3CL, ACE2, and S protein of SARS-CoV-2 than these four antiviral drugs. In summary, the four CPMs may play a role in the treatment of COVID-19 by binding flavonoids such as quercetin, luteolin, and acacetin to target proteins such as ACE2, 3CLpro, and S protein and acting on TNF, IL6, IL1B, CXCL8, and other targets to participate in broad-spectrum antiviral, immunomodulatory and inflammatory responses.
Introduction
Since the beginning of December 2019, COVID-19 has caused devastating consequences (1) and has been declared as the sixth public health emergency of international concern by the World Health Organization (WHO) (2). COVID-19 is highly contagious and spreads quickly, with broader impacts, and the population is generally susceptible (3). In addition, SARS-CoV-2 directly attacks the respiratory system and attacks the respiratory system, but also affects the immune, digestive, cardiovascular, neurological, genitourinary, and other extrapulmonary systems (4, 5). Moreover, related literature reports suggested that patients with COVID-19 might have multiple systemic sequelae during recovery (6). At present, the global epidemic has been controlled to some extent, while sporadic outbreaks still occur in some places. It is essential to summarize the successful experiences of various countries and promote the development of new drugs.
As one of the first countries affected by COVID-19, China has accumulated a lot of valuable experience in fighting COVID-19. It has been shown that Lianhua Qingwen (LHQW), Huoxiang Zhengqi (HXZQ), Jinhua Qinggan (JHQG), and Shufeng Jiedu (SFJD) are effective in treating COVID-19 (7). For COVID-19 patients in the medical observation period, JHQG, LHQW, and SFJD are recommended for fever and fatigue. For fatigue with gastrointestinal discomfort, HXZQ is recommended. In terms of clinical efficacy, in a multi-center randomized controlled study led by academician Nanshan Zhong, a total of 284 patients with COVID-19 were included. The results proved that combining LHQW with conventional treatment can significantly increase the symptom recovery rate, shorten the median time for symptom recovery, and improve the CT and clinical cure rates (8). Ling Chen et al. reviewed the clinical data of 200 patients with ordinary COVID-19 treated at Wuhan Third Hospital from January 27, 2020, to March 5, 2020. The results show that the combined application of SFJD can significantly improve the clinical symptoms of typical COVID-19 patients with cough, sputum, fatigue, chest tightness, and wheezing. The main symptoms are improved. It also can regulate the expression of related peripheral blood inflammation indicators and promote lungs inflammation absorption. At last, the cure rate are improved (9). Academician Boli Zhang and others reviewed 123 patients with mild COVID-19 who were admitted to the emergency department of Hubei Integrated Traditional Chinese and Western Medicine Hospital from February 1 to 5, 2020. The results demonstrated that JHQG combined with conventional western medicine treatment could significantly reduce the clinical symptoms of fever, cough, fatigue, and sputum in patients with mild COVID-19 and relieve anxiety of patients (10). HXZQ is an ancient anti-epidemic prescription. The Diagnosis and Treatment Scheme of Severe Acute Respiratory Syndrome (SARS) (Version 2004) recommends that HXZQ can be used for advanced lung closure, and it has shown initial results in the front line of anti-epidemic (11, 12).
Regarding basic experiments, corresponding evidence can be found for the treatment of COVID-19 by four CPMs. LHQW can work by inhibiting virus replication and reducing the release of cytokines from host cells. The team of academician Nanshan Zhong found in the African green monkey kidney cells Vero E6 cells that LHQW can inhibit the replication of SARS-CoV-2, affect the morphology of the virus, and inhibit pro-inflammatory cytokines at the mRNA level, such as TNF-α, IL-6, CCL2/MCP-1, and CXCL-10/IP-10 (13). SFJD has a broad spectrum of antiviral, anti-inflammatory, and antibacterial effects and can reduce lung injury (14), the levels of IL-1β and IL-18 in serum and bronchoalveolar perfusion fluid, and the expression levels of NLRP3-related components and virus titers in lung fluid (15–17). HXZQ has the functions of antiviral, anti-inflammatory, and immune regulation and could improve gastrointestinal discomfort (18). It can also reduce the inflammatory response in patients with COVID-19 by reducing pro-inflammatory factors, increasing the level of IL-10, and regulating the NF-kB pathway of inflammation (18). JHQG reduces lung inflammation, protects immune organs, regulates cytokine balance, and improves body immunity (19, 20).
Evidence can be discovered in clinical trials and basic experiments for four CPMs to treat COVID-19. Is there a common mechanism of action among them? To promote the development of new drugs and provide a reference for the following basic experiment, we explored the possible mechanism of the action of four CPMs in the treatment of COVID-19 from the perspective of network pharmacology. The workflow is shown in Figure 1.
Materials and methods
Identification of candidate compounds
There were four prescriptions, one from each group. According to the composition of the prescriptions, the traditional Chinese medicine system (TCMSP)1 (21) database was searched for the components of the four prescriptions using the pinyin name or latin name of each herb in each prescription. The composition and dosage of each prescription are shown in Table 1. The herb not found in the TCMSP were supplemented from the TCMID2 database and relevant published literature. The TCMSP database is the most commonly used database for the retrieval of Chinese medicine ingredients and describes the relationships between drugs, targets, and diseases (21, 22). This database includes 500 TCMs from the 2010 edition of the pharmacopeia and 3,069 compounds (23).
ADME screening
Oral bioavailability (OB) refers to the percentage of unmodified drug that is absorbed into the circulatory system after oral administration. The OB is a reliable indicator of the efficacy of oral administration (24). The higher the OB value of the drug is, the greater the clinical value is. After calculation and prediction by of the OBioavail1.1 software, herb components with an OB ≥ 30% were selected as candidate molecules for further analysis in this study (25).
The drug-likeness (DL) refers to the similarity of a compound to a known drug (26). The valuation of the DL coefficient is used in the early stages of drug development to help identify excellent compounds. Therefore, in this study, the tanimoto coefficient (27) was used to evaluate the DL index of the molecule of the four prescriptions. The calculation formula is as follows:
where “a” is the molecular property of the herbal compound on the basis of Dragon software3 (28) and “b” is the average molecular property of all the molecules in the DrugBank database.4 The average DL index of all the drugs in this database is 0.18. Therefore, compounds that met the conditions of OB ≥ 30% and DL ≥ 0.18 were defined as active ingredients in this study and were considered to have better pharmacologic effects (29, 30).
Identification of drug targets
In the previous two steps described, we identified the active compounds of the drugs. Then, the corresponding target proteins were identified with the TCMSP and ChemMapper databases, and the repeated targets were simultaneously eliminated. Finally, we used the UniProt KB database5 to standardize the target proteins and identify the confirmed human target genes.
Identification of common targets for four Chinese patent medicine
Jvenn diagrams6 are widely used in biology to illustrate the differences between gene lists originating from different differential analyses (31). The targets of the four prescriptions were intersected, and the selected targets were identified as their common targets by default.
Predicting putative targets of 2019-nCoV
The 2019-nCoV-related target proteins were screened from two databases: (1) the GeneCards (Ver. 4.14)7 database, the largest public platform, is used to effectively navigate gene–disease linkages (32, 33) and (2) the National Center for Biotechnology Information (NCBI)8 database is a popular choice for data deposition (34). We searched the two databases with the keyword “novel coronavirus” and set the species to “Homo sapiens.”
Protein–protein interaction network construction and hub gene analysis
First, we intersected the shared targets of the four prescriptions with the genes associated with 2019-nCoV and obtained a Venn diagram of the intersecting gene symbols. Then, the potential targets were imported into STRING,9 which is a database for predicting protein–protein interactions. The STRING database defines PPIs and provides confidence ranges for data scores (high > 0.7; medium > 0.4; low > 0.15) (35). In this study, we limited the species to “Homo sapiens” and selected a confidence score > 0.7. Finally, we exported the downloaded “string_interactions.tsv” file and imported it into the Cytoscape 3.7.210 platform to obtain and visualize the PPI network.
A hub gene is a gene that plays a crucial role in biological processes. In related pathways, the regulation of other genes is often affected by this gene. Therefore, the “cytoHubba”11 plug-in of Cytoscape was used to filter the hub nodes in the network according to the network topology parameters (36). The hub gene was further validated by cluster analysis using the MCODE plugin in Cytoscape.
Gene ontology and pathway enrichment
Gene Ontology (GO) is an international standard system for classifying gene function (37). GO was used to describe the gene functions and the relationships between these concepts. GO divided gene functions into three aspects: molecular function (MF), cellular component (CC), and biological process (BP) (38).
Kyoto Encyclopedia of Genes and Genomes (KEGG) is a set of artificially drawn pathway maps representing molecular interactions and reaction networks and includes seven major biological processes, including metabolism, cellular processes, organismal systems, and so on.
To further investigate the multiple mechanisms of the potential targets in 2019-nCoV, we conducted of GO and KEGG Enrichment analyses using the clusterProfiler R package (ver. 3.6.3), which is an R package that automates the process of biological term classification and the enrichment analysis of gene clusters. Relevant pathways with P-values < 0.05 were selected (39). In addition, the Hub gene enrichment pathway with GO-BP was enriched via ClueGO.
Network construction and analysis
To intuitively understand the common mechanism of the four recommended CPMs in the treatment of COVID-19 during the observation period, the identified active components, the potential targets, and the signal pathways with high scores were input into Cytoscape 3.7.2 software to construct the active ingredient—target—pathway network. At the same time, we constructed the relationship between the common active ingredients of four CPMs.
Molecular docking
Angiotensin-converting enzyme 2 (ACE2) is the entry receptor for 2019-nCoV entry into host cells (40); S protein, a type I glycoprotein on the surface of the virus, plays a crucial role during virus entry into the host cells (41); 3-C like protease (3CL pro)play an important role in post-translational processing of replicase polyproteins (42). The crystal structure of the ACE2, S protein, 3CLpro was taken from the RSCB Protein Data Bank (PDB ID: 1R42, 6VSB, 6LU7). PyMol (version 2.3.4) software package was used to erase all the hetero atoms, water molecules, and inhibitor present in the structure (43). the non-covalent interaction of phytochemicals-protease was calculated using autodock Vina (version 1.1.2) software package for the docking analysis (44). Using this method, binding affinities of ligand-protease were determined and reported in kcal/mol unit. For the ACE2, grid box (82 Å × 68 Å × 78 Å) centered at (53.788, 60.568, 30.881) Å, for the 3CLpro, grid box (52 Å × 64 Å × 66 Å) centered at (−26.283, 12.599, 58.965) Å, for the S protein, and grid box (126Å × 126 Å × 126 Å) centered at (225.616, 226.49, 225.337) Å.
Molecular dynamics simulation
To further investigate the stability of ligand small molecule binding proteins, molecular dynamics simulations (MDS) were performed using Gromacs 2020.6 software. Because the small ligand molecule is a multi-carbon ring skeleton structure, the Charmm36 force field with the TIP3P water model was chosen for simulation (see Supplementary Data Sheet 1 for specific parameters). The system temperature was set to 37°C for sodium chloride solution to simulate the real environment in the human body. During the MD simulation, all hydrogen bonds involved were constrained using the LINCS algorithm with an integration step of 2 fs. The electrostatic interactions were calculated using the Particle-mesh Ewald (PME) method with the cut-off value set to 1.2 nm. The non-key interaction truncation value is set to 10 Å and is updated every 10 steps. The protein-ligand complexes (hereafter referred to as complexes) are first pre-equilibrated for 100 ps to optimize the initial conformation of the protein in the solvent. After that, the NVT equilibrium of 100 ps with a coupling time constant of 0.1 ps was performed using a modified Berendsen temperature coupling algorithm to warm up the complex to 310 K with the solvent system. Subsequently, a 100 ps NPT equilibration was performed using a Berendsen constant pressure to equilibrate the solvent to the complex system to 1 bar. Ultimately, MDS of 50 ns duration was conducted to further investigate ligand binding relative to the receptor. Root Mean Square Deviation (RMSD) analysis was performed on the simulation results to analyze the stability of the ligand relative to the receptor during the simulation. The radius of gyration (Rg) can characterize the tightness of the protein structure and likewise relies on the radius of gyration to indicate the changes in the peptide chain relaxation of the protein during the simulation. In addition, hydrogen bond number analysis was performed for ligand and receptor binding to observe whether the binding is stable from the bonding perspective.
Results
The compounds, active ingredients and related targets of the four prescriptions
Based on the TCMSP database and the existing literature reports, 1,848, 1,818, 1,773, and 1,151 compounds of the four prescriptions HXZQ, JHQG, LHQW, and SFJD were retrieved, respectively. According to the criteria of OB ≥ 30% and DL ≥ 0.18, 203, 228, 241, and 179 active compounds and 269, 286, 246, and 259 predicted targets were identified, respectively. The number of the components, active components and potential targets of each prescription is shown in Table 2.
Common targets for four prescriptions
A total of 233 common targets of the four prescriptions were identified through jvenn diagrams, as shown in Figure 2.
Protein–protein interaction network and hub gene analysis
2019-nCoV gene dataset collection from the NCBI and GeneCards databases, 48 potential targets and 350 potential targets in 2019-nCoV were identified, respectively, and 46 duplicates were eliminated. A total of 352 targets related to 2019-nCoV were identified.
Among the above 352 2019-nCoV-related targets, the four prescriptions shared 51 common targets with the targets in 2019-nCoV (Figure 3A). The 51 putative therapeutic targets were imported into the STRING database to establish the putative therapeutic target PPI network, and then the “string_interaction.tsv” file was imported into Cytoscape 3.7.2 to perform network analysis. The screening of hub genes was performed using Cytoscape’s plugin cytoHubba and MCODE, respectively. The results of both screens were combined, and a total of 11 hub genes were obtained, namely TNF, IL6, IL1B, CXCL8, CCL2, IL2, IL4, ICAM1, IFNG, IL10, and MCL1. The hub genes screened by the plug-in cytoHubba and the plug-in MCODE are shown in Figures 3B, 4, respectively. The topological parameters of the 11 hub genes are shown in Table 3.
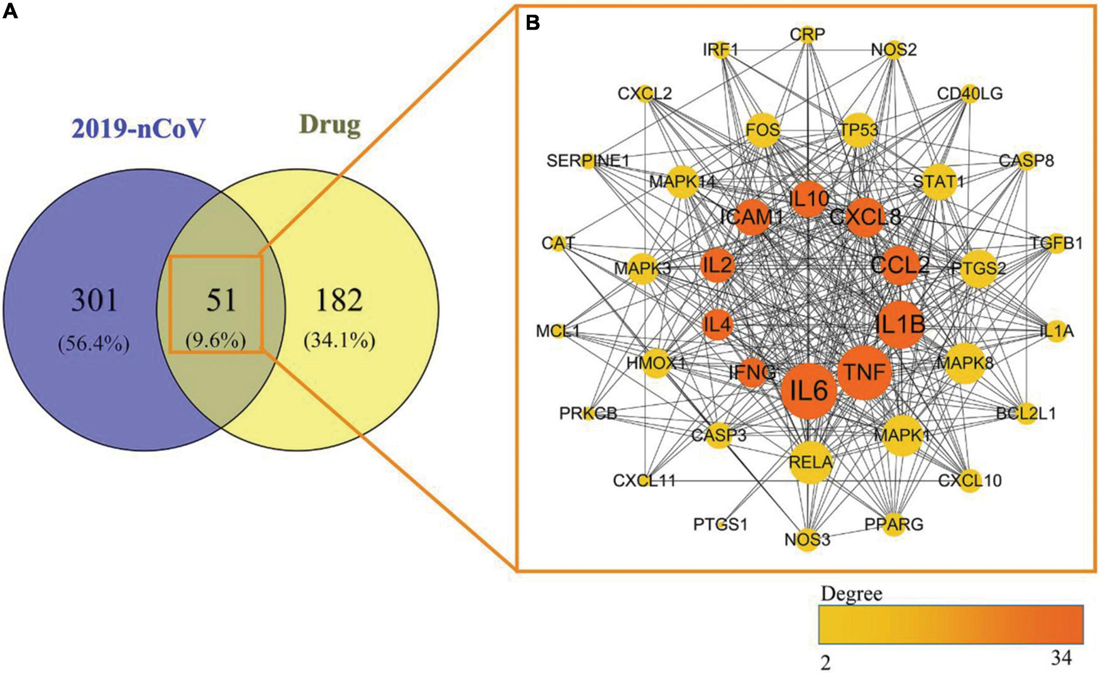
Figure 3. Mapping relationships between the four recommended CPMs and COVID-19. (A) Intersection targets of COVID-19 and four CPMs. (B) Protein interactions among 51 potential targets. The node’s size indicates the target’s degree, and the larger the node is, the higher the importance is. The thicker edges suggest stronger interactions. The degree value of nodes is positively correlated with node size and color brightness. The middle 10 targets are Hub genes screened based on the MCC method.
Pathway and gene ontology term enrichment analysis
Gene ontology enrichment
A total of 396 GO-BPs, 55 GO-MFs, and 33 GO-CCs were enriched by R package (version 3.6.3). The top 20 GO terms according to P-values < 0.05 are shown in Figure 5. BP was mainly enriched in the regulation of the apoptosis process (5 in total), response to oxidative stress (3 in total), response to lipopolysaccharide (2 in total), response to molecules of bacterial origin (2 in total), inflammatory response, T cell activation and so on (see Figure 5A for details); CC was mainly concentrated in membrane rafts, membrane microdomains, membrane regions, mitochondrial outer membranes, organelle outer membranes, outer membranes, plasma membrane rafts, RNA polymerase II transcription factor complexes, cell-substrate adherens junctions, cell-substrate junctions and so on, and the membrane had the largest proportion (see Figure 5C); MF was mostly involved in inflammatory mediator cytokines, MAP kinase activity, phosphatase binding, heme binding, and so on (see Figure 5B).
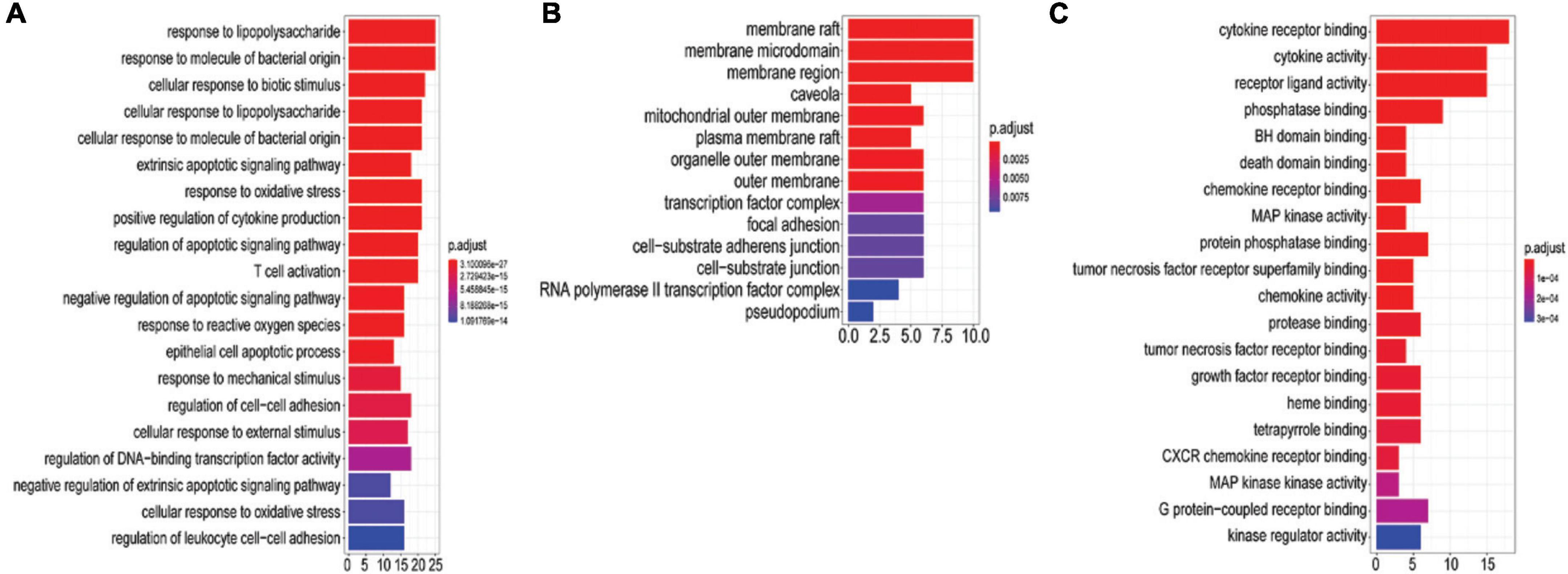
Figure 5. GO analysis of 51 potential targets genes. The y-axis shows the name of the GO entry, and the x-axis shows the value of gene number count, the value of which represents the number of targets enriched on the GO entry. The redder the color is, the smaller the value of p. adjust is. (A) The top 20 biological processes (BP). (B) GO-MF. (C) GO-CC.
Kyoto encyclopedia of genes and genomes enrichment
To elucidate the signaling pathways involved in the treatment of COVID-19 with four CPMs, we subjected 51 drug and disease intersection targets to pathway enrichment analysis by R 4.1.3. A total of 166 pathways were enriched (P < 0.05), 92 of which may be associated with COVID-19. The first 39 signaling pathways were visualized and analyzed in Figure 6A. Figure 6B shows the enriched signaling pathways hitting hub genes, showing 53 pathways associated with the 11 hub genes. It further indicates that hub genes and enrichment pathways have some credibility. The specific parameters of the hub genes and their associated pathways of the four CPMs are shown in Table 4.
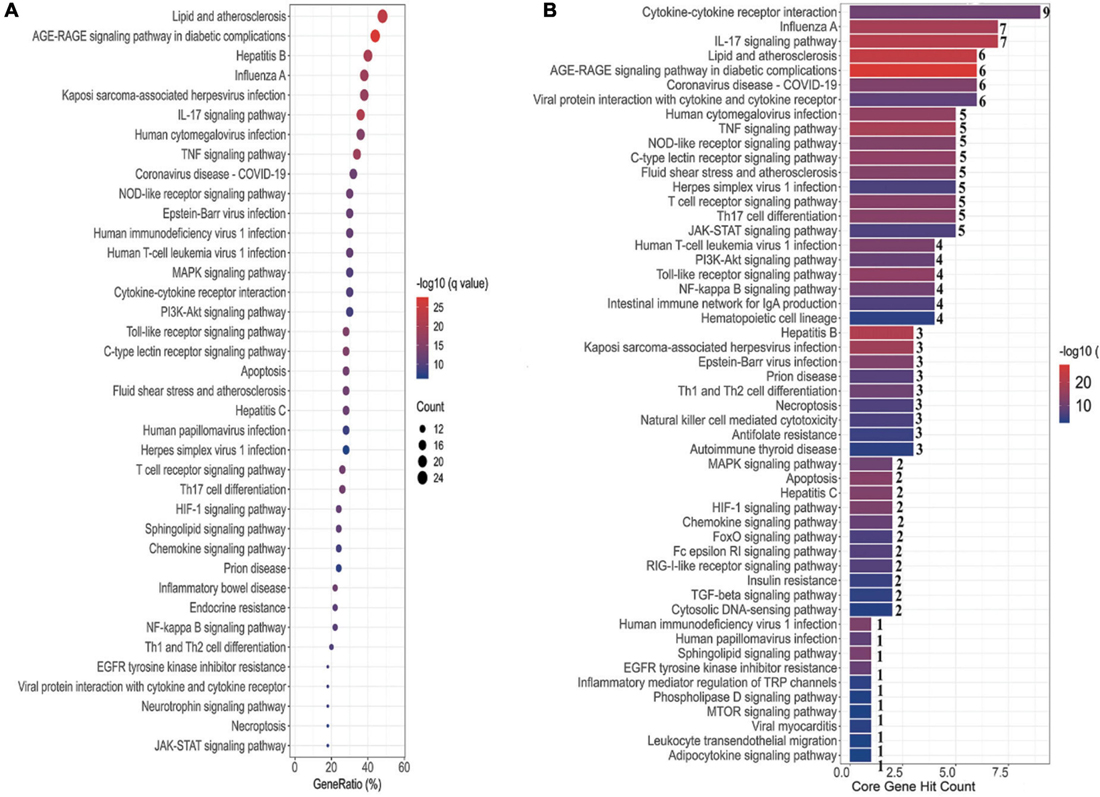
Figure 6. Pathway enrichment analysis and pathway hit to hub genes. (A) The top 39 signaling pathways associated with 51 potentially acting target genes. Pathways with a P-values < 0.05 were identified. The spot size represents the number of genes, and the color represents the P-value. The horizontal coordinate represents the generation, which is the ratio of the number of intersecting genes enriched in the pathway to the total intersecting genes; the vertical coordinate represents the name of the enriched pathway. (B) Signaling pathways associated with hub genes. The horizontal coordinate represents the number of hub gene hits on the pathway, and the vertical coordinate represents the pathway name.
As shown in Table 5, the pathways were mainly enriched in three areas: (i) viral infections, such as coronavirus disease-COVID-19, and human cytomegalovirus infection. (ii) immunity, such as Toll-like receptor signaling pathway, T cell receptor signaling pathway, NOD-like receptor signaling pathway, and other signaling pathways; (iii) inflammation, such as cytokine-cytokine receptor interaction, IL-17 signaling pathway, Th17 cell differentiation, C-type lectin receptor signaling pathway, etc. In addition, the relationship between the Hub gene enrichment pathway and the gene ontology biological process (G0-BP) is shown in Figure 7. As shown in Figure 7, the pathways enriched by the hub genes, such as coronavirus disease, cytokine-cytokine receptor interaction, and viral protein interaction with cytokine and cytokine receptor, are closely related to the production and regulation of molecular mediators of the immune response, the positive regulation of immune effector processes, and the regulation of leukocyte migration.
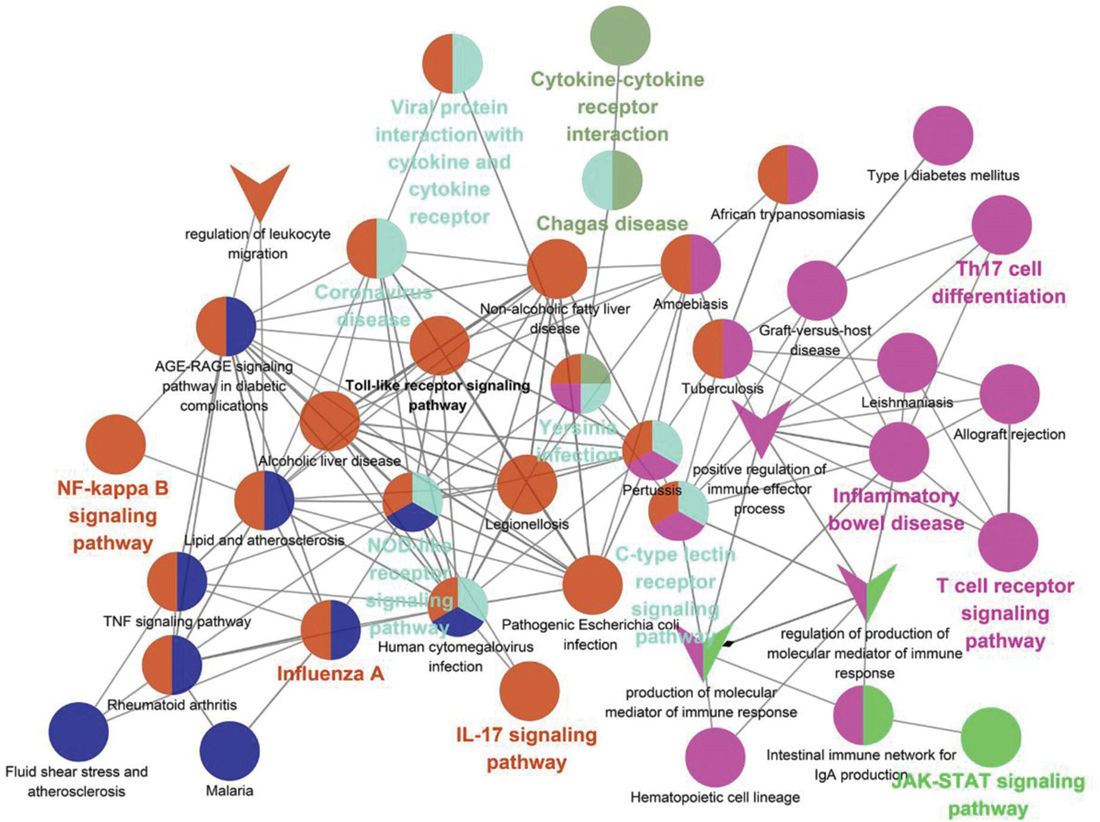
Figure 7. Correlation of KEGG enrichment of hub gene with GO-BP. The circle represents the pathway, and the “V” shape represents GO-BP. The node color means that the pathway is clustered into the same clusters with similar functions. The bolded pathway name means the p-value is smaller in that clusters with high confidence.
Network construction and analysis
Active component-common active component network of four prescriptions
There are 411 nodes and 850 edges in the active component-common active component network of the four prescriptions (Figure 8). The details of the 101 common active components are provided in Supplementary Table 1.
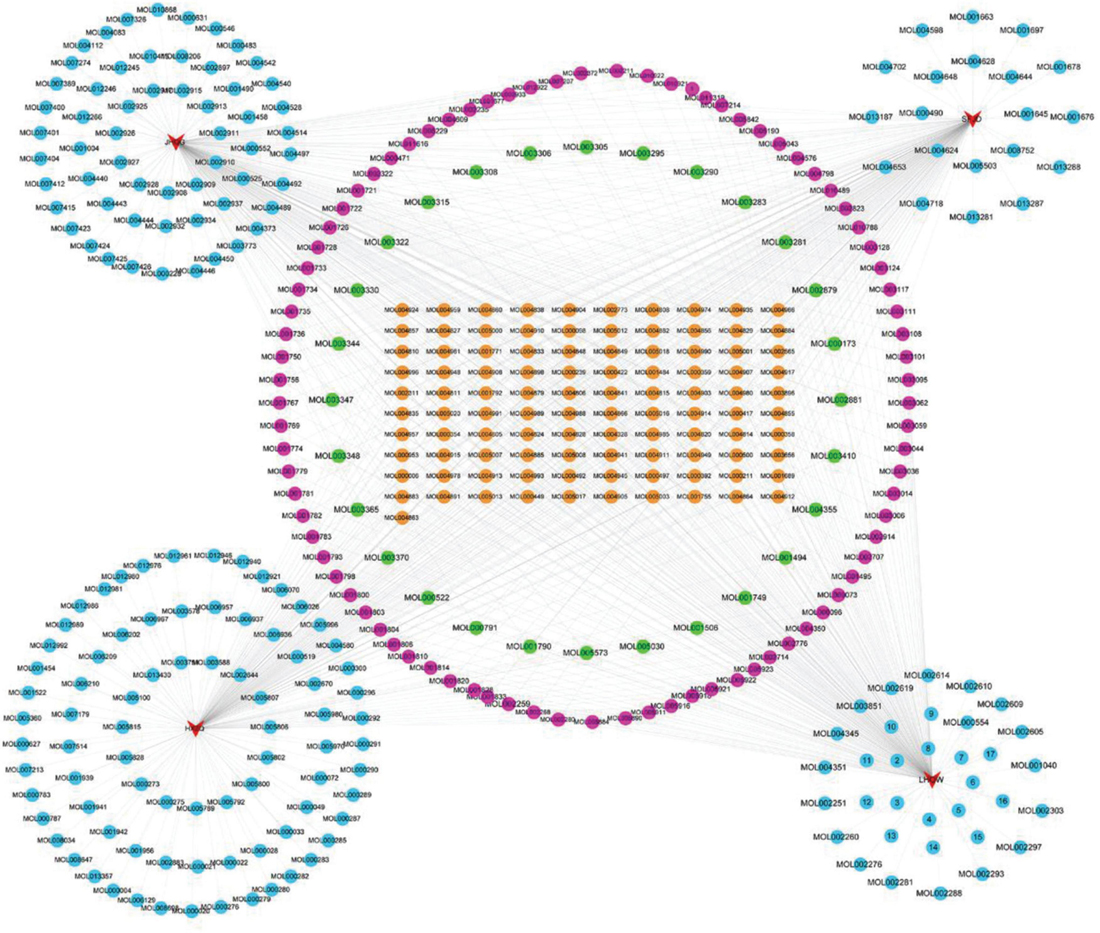
Figure 8. Active component-common active component network of the four prescriptions. The red triangles represent the four prescriptions; the Orange, green, rose red, and sky blue circles represent the common active ingredients of the four prescriptions, the common active ingredients of three prescriptions, two prescription common active ingredients, and each prescription unique active ingredient.
Active component-potential target–pathway network
The 53 signaling pathways associated with hub genes, potential targets, and active ingredients in the signaling pathways were entered into Cytoscape 3.7.2 software to construct the active ingredient-potential target-pathway network, as shown in Figure 9. According to the network diagram and topological parameters, it can be seen that the 21 active ingredients, 46 targets, and 56 signaling pathways are closely related to each other. The greater the nodal degree, the more biological functions it involves, indicating its biological importance. On the one hand, considering that fewer key components were screened by degree greater than the mean value, and on the other hand, we screened the pathway three times to further ensure the integrity and reliability of the study, all the active ingredients enriched this time were initially used as key active ingredients. Subsequently, key components of the initial screen were excluded if they had docking binding energies greater than −5 kcal/mol to the three potential action targets of COVID-19. Twenty-one key compounds were finally identified, and their specific parameters and sources are shown in Table 6.
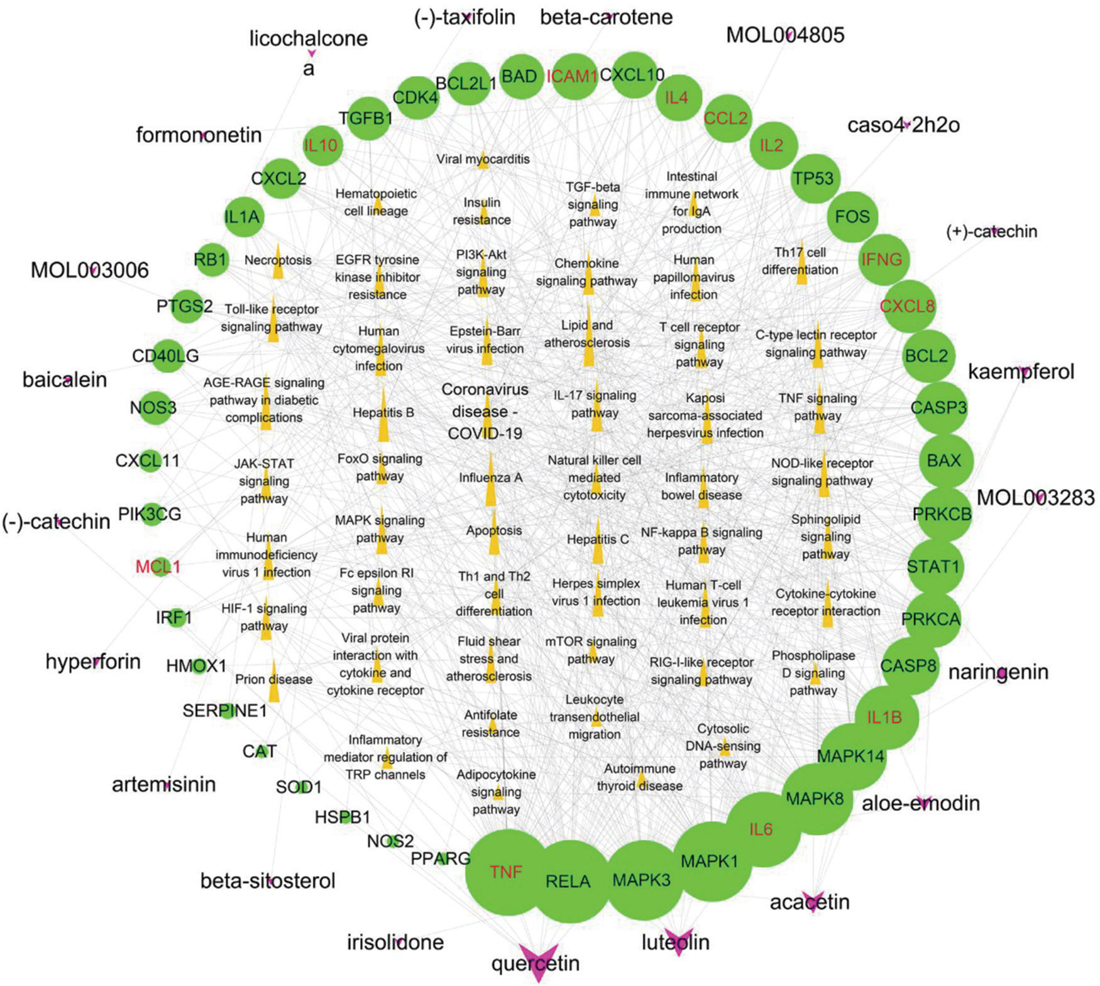
Figure 9. Potential active component-potential target–pathway network. Green circles represent action targets, yellow triangles represent the 53 signaling pathways associated with Hub genes, and rosy V shapes represent the four prescribed active ingredients. The node size is positively related to the degree, and the edges represent the interactions between the nodes.
Molecular docking
Lopinavir, remdesivir, ritonavir, ribavirin have been widely used in antiviral therapy (45–47), mainly through the control of their binding sites. In Autodock Vina scoring system, lower binding score indicates the better docking interaction between ligand and protein. According to the analysis of docking results (Table 7), binding affinities of the phytochemicals are distributed within the range of −7.0 to −9.1 kcal/mol, Similar to anti-viral drugs within the range of −6.1 to −10.4 kcal/mol. Molecular docking is performed to find out the best candidates among the 9 phytochemicals based on their binding scores. In docking with 3CLPro, ACE2, and S protein, the affinity of shinflavanone was in the order of −8.4, −9.7, and −10.4 kcal/mol, which were superior to these four antiviral drugs. In addition, the beta-carotene binding energy in docking with ACE2 was lower than that of Ritonavir and Ribavirin. In docking with S protein, beta-carotene and beta-sitosterol binding energy were lower than Remdesivir, Ribavirin, and Ritonavir; hyperforin and baicalein binding energy was lower than Ribavirin and Ritonavir.
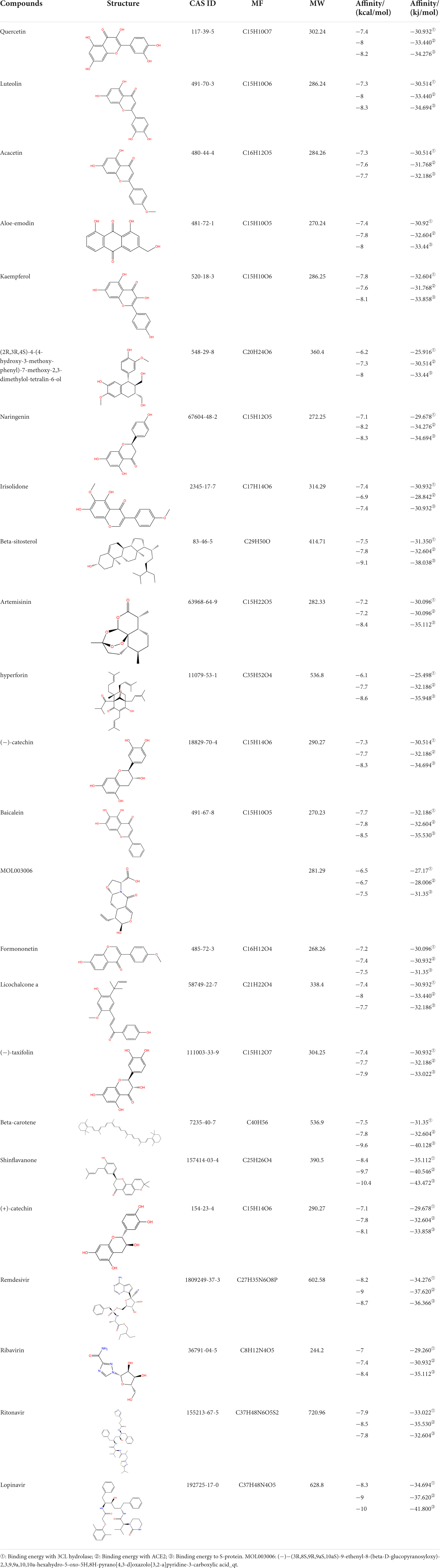
Table 7. Chemical information and binding energy of four CPM main potential active ingredients and antiviral drugs.
Additionally, the docking analysis between the selected compounds and the target proteins was shown in Figure 10. In docking with 3CLPro, the compound shinflavanone formed hydrogen bonds with GLY-143 to interact with each other (Figure 10A). In docking with S proteins, β-sitosterol formed three hydrogen bonds with ARG-1039 (A), ARG-1039 (B), and ARG-1039 (C), respectively (Figure 10F). Hyperforin forms two hydrogen bonds with LEU-1024 (B) and THR-1027 (B), respectively (Figure 10G). Baicalein formed two hydrogen bonds with GLN-1036(C) and HIS-1048(C), respectively (Figure 10H). These 21 phytochemicals bind to the active site of the major protease of 2019-nCOV with lower binding energy than the crystal structured ligand, implying that they may have strong antiviral activity.
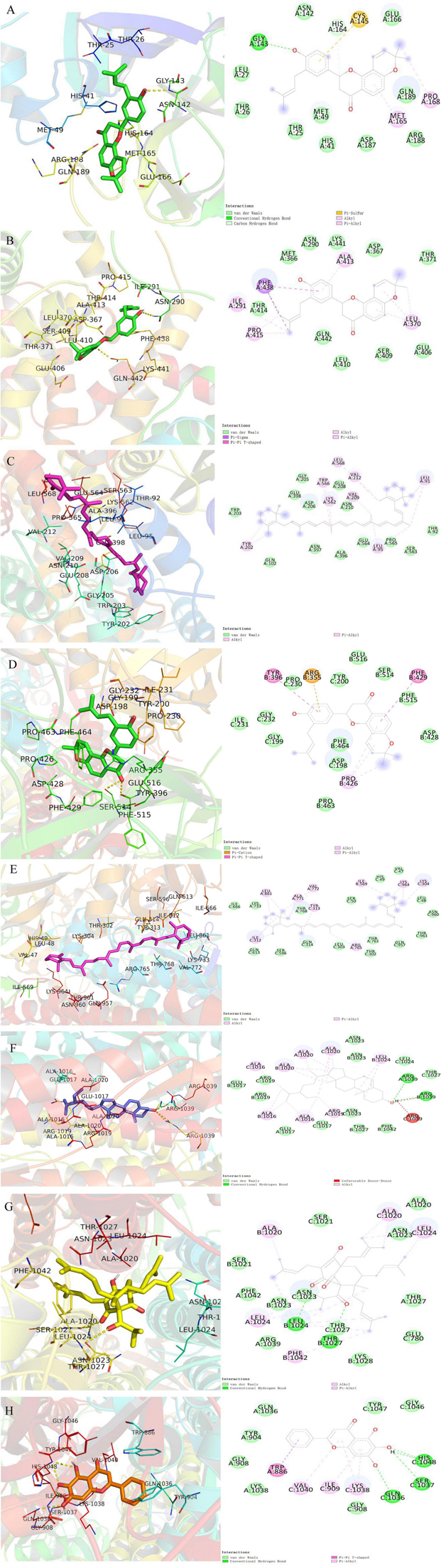
Figure 10. Molecular models of the selected compounds binding to the target proteins. (A) The docking mode and interactions between shinflavanone and 3CL. (B–C) Shinflavanone, beta-carotene and ACE2. (D–H) Shinflavanone, beta-carotene, beta_sitosterol, hyperforin, baicalein and S protein.
Molecular dynamics simulation
From the RMSD plot analysis (Figure 11A), it is observed that the complex formed by the compound and ACE2 protein was more stable during the simulated 50 ns. The RMSD values of the ligand relative to the receptor were maintained in a low range and fluctuated less, suggesting that the ligand did not move much relative to the receptor and the binding was more stable. The RMSD of the compound relative to 3-C like protease maintained an increasing trend in the first 25 ns or so, and showed a trend of decreasing and then increasing to the RMSD value at 25 ns from 25 to 40 ns, and basically maintained equilibrium with less fluctuation in the last 10 ns. The possible reason was that the ligand shifted the binding cavity of the protein as the simulation proceeded. After entering the new binding cavity and undergoing particular rotation, it maintained a stable conformation and conformation in the last 10 ns, forming a more stable binding. The stability of the binding can be further verified by extending the duration of the kinetic simulation later. The RMSD values of the compounds relative to the S proteins kept fluctuating widely during the simulation, suggesting that the binding between the ligand and the receptor may not be very stable. Therefore, further extension of the kinetic time is needed to investigate the binding stability.
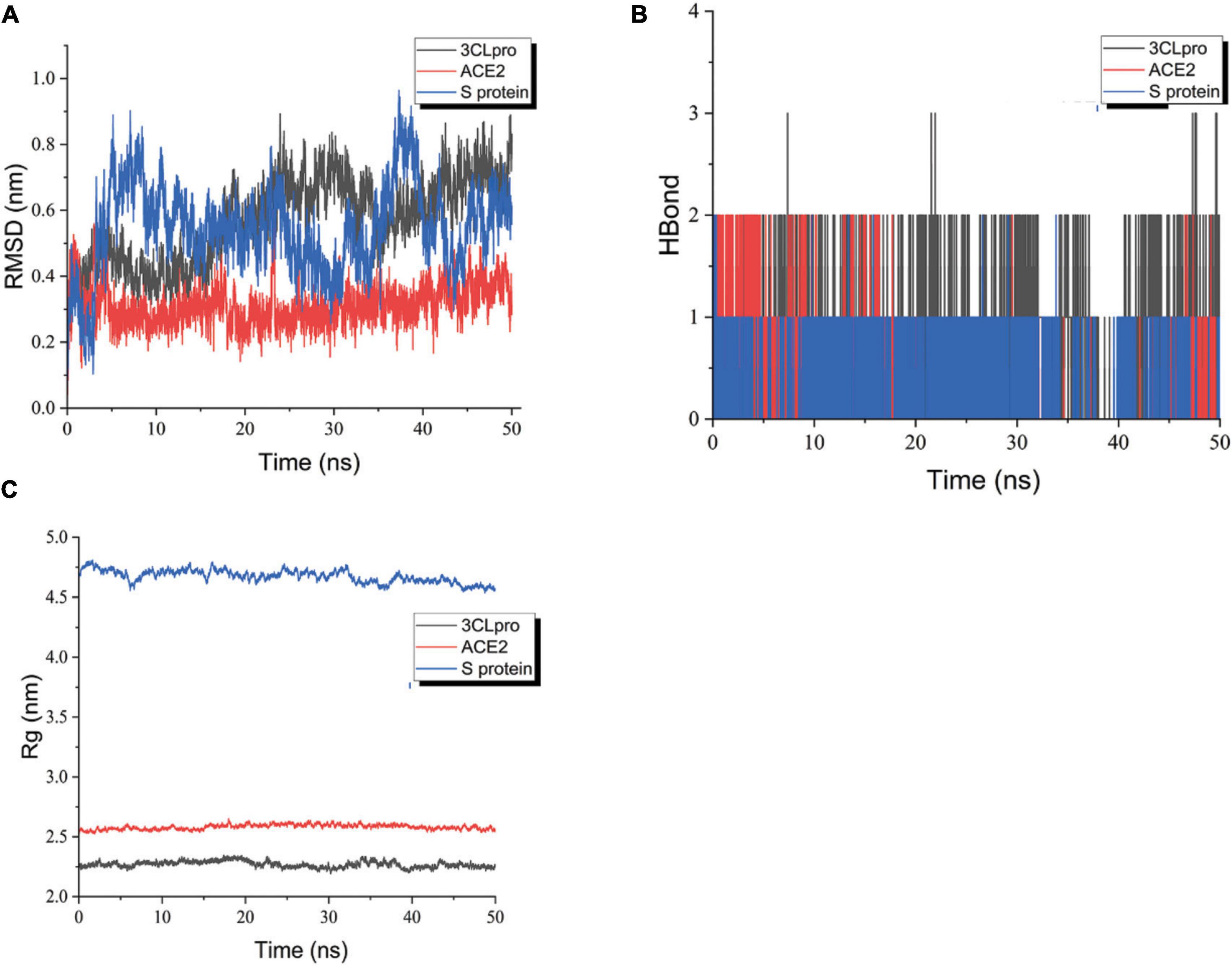
Figure 11. RMSD plot during molecular dynamics simulations. (A) The RMSD of ligand-receptor. (B) The H-Bond of ligand-receptor. (C) The Rg of ligand-receptor. Blue polygonal line means shinflavanone and S protein, red polygonal line means shinflavanone and ACE2, black polygonal line means shinflavanone and 3-C like protease.
Rg can characterize the tightness of the protein structure, and the same radius of gyration can be relied on to distinguish the changes in the peptide chain relaxation of the protein during the simulation. As shown in Figure 11C, the Rg of both 3CLpro and ACE2 proteins remained relatively stable throughout the simulation. The Rg of 3CLpro protein was basically maintained around 2.25 nm, and that of ACE2 protein was basically maintained around 2.5 nm, suggesting that the complexes formed by the proteins and ligands were more stable. However, the Rg of S protein shows some fluctuations, which may be related to the fact that the small molecule is constantly undergoing conformational and conformational transitions, which in turn have some effects on the protein structure. It is in agreement with the previous analysis of RMSD and RMSF.
The number of hydrogen bonds between the protein and the ligand varies with time during the simulated 50 ns. As can be seen in Figure 11B, the 3-C like protease maintains the formation of more than two hydrogen bonds between the ligand and the receptor for nearly half of the simulation. Several hydrogen bonds between the ACE2 protein and the ligand were always formed. The overall situation was similar to that of 3-C like protease. The S protein basically maintains a hydrogen bond with the ligand during the simulation, suggesting that the ligand may always keep a part of the molecule relatively static in conformation and conformation during the simulation. In contrast, the rest of the molecule undergoes conformational and conformational transitions.
In general, the ligand maintains a more stable binding to the 3-C like protease and ACE2 protein throughout the simulation. In the complexes formed with S proteins, the ligands kept undergoing conformational and conformational transitions. The stability of S protein-ligand binding can be further explored later by extending the kinetic simulation time.
Discussion
Traditional Chinese medicine (TCM) and herbal medicines (HMs) have a long history and play an indispensable role in the prevention and treatment of several epidemic diseases (48). Based on network pharmacology and systems biology, this paper discusses the possible common mechanisms of the four CPMs recommended by the Diagnosis and Treatment Protocol for COVID-19 during the Medical Observation period.
The material basis of the medicinal effect of traditional Chinese medicine
These four CPMs have been widely used and well-established in the Chinese protocol for treating COVID-19. At the same time, the specific content and proportion of the ancient books from which the CPM is derived are described for convenience of reference. The research on the material basis of the efficacy of TCM is gradually rising from molecular biology to the level of systems biology. Although there is no clear definition of the material basis of pharmacological efficacy in Chinese medicine, different theories and hypotheses exist, such as the all-component theory, the active ingredient theory, the metabolic component, and the multi-component synergism theory (49). However, most scholars believe that TCM’s effectiveness is reflected in the overall biological effect of the entire chemical composition, which is the synergistic effect of multiple components, not a single chemical entity. It is because a single ingredient does not represent an herb’s drug properties and efficacy, nor is the efficacy the sum of all ingredients (50). In drug compatibility, there will be physical or chemical reactions, which will lead to the formation of new compounds, affect the dissolution rate of effective and toxic components in the formula, or have no noticeable effect. In addition, the chemical components that act on multiple targets and produce overall effects after entering the human body may originate from inherent components of the herbs, products formed during the preparation process, or metabolites produced by the interaction between the drug and the body after entering the body (51). Lan Wang et al. demonstrated synergistic effects between three active compounds isolated from three components of a TCM formulation in an animal model of acute promyelocytic leukemia (APL) and an APL cell line (52). At present, the existing methods to study the synergistic effect of Chinese herbal medicines include mathematical modeling (such as CI) and computer technology (such as systems biology analysis, S2S), fractionation technology combined with CI models, and combination of effective components of traditional Chinese medicines (50). In addition, in 2008, China launched the 15-year “Herbalome Project,” which aims to elucidate the chemical composition, structure, and function of commonly used Chinese herbal medicines and Chinese medicine formulations, establish a standard resource library and explain the synergistic and complementary mechanisms of multi-component Chinese medicines at multiple targets (53, 54). Although the synergistic research of Chinese herbal medicine is still in its infancy, we believe that it will develop better and better with the efforts of everyone.
Active compounds analysis
According to the “active ingredient-potential target-pathway,” 21 essential compounds were identified, followed by quercetin, luteolin, acacetin, aloe-emodin, kaempferol, naringenin, irisolidone, beta-sitosterol, artemisinin, hyperforin, etc. Most of them are flavonoids. Flavonoids are natural antiviral compounds with a wide range of antiviral activity, anti-inflammatory, antioxidant, and immunomodulatory effects (55–57). Molecular docking results showed that shinflavanone had a better affinity for SARS-CoV-2 3CL hydrolase, ACE2, and S protein than all four commonly used antiviral drugs, suggesting that it may be superior in interfering with virus entry into host cells and viral replication. In addition, 19 compounds also showed better affinity to SARS-CoV-2 3CL hydrolase or ACE2 or S protein than one of these four commonly used antiviral drugs, such as quercetin, luteolin, acacetin, aloe-emodin, kaempferol, naringenin, beta- sitostero, artemisinin, hyperforin, baicalein, formononetin, licochalcone A, (−)-taxifolin, beta-carotene, (+)-catechin, irisolidone, and (−)-catechin, suggesting that TCM has excellent potential in the treatment of SARS-CoV-2.
(I). Flavonoids
Quercetin has many effects, such as anti-inflammatory, immunomodulatory, antioxidant, and free radical scavenging, which can play a prominent therapeutic role in lung injury (58–63). Quercetin can block the enzymatic activity of coronavirus 3cl protease in treating viral infections such as Sars-CoV infection. Quercetin also blocks the entry of the viral receptor complex into the cell and interrupts its life cycle, leading to viral death (64). In addition, as an essential consideration in developing new antiviral drugs (65, 66), quercetin can increase the bioavailability of antiviral drugs in the body. Luteolin has potent anti-inflammatory, antiviral, antibacterial, and other effects (67, 68). At the lowest concentration (1 mol/L), it can effectively reduce the expression of NF-KB P65, IL-1β, IL 6, and TNF-α in anti-inflammatory (69). Kaempferol has antiviral, anti-inflammatory, antioxidant, and other effects (70, 71). In addition, some studies reported that quercetin, luteolin, and kaempferol could inhibit the proteolytic activity of Sars-CoV 3CLpro (52–54). Naringenin is famous for its antiviral effect and has anti-inflammatory, antioxidant, and immunomodulatory effects (72–75). In addition, naringenin can inhibit inflammatory factors through the NF-κB pathway and reduce LPS-induced lung inflammation. Licochalcone A has anti-inflammatory, immune-promoting, and other biological effects (76, 77). It has been reported in the Lancet that the main active ingredient of licorice (glycyrrhizic acid) effectively inhibits the replication of clinical isolates of sars viruses, and licochalcone A may have the same function (78). Shinflavanone is also derived from Glycyrrhiza glabra, and its antiviral may be superior according to molecular docking, but it is relatively little studied at present and needs further research.
Baicalein and acacetin are flavonoids in scutellaria baicalensis, with anti-inflammatory, antioxidant, and ameliorative effects on acute lung injury (79). Baicalein strongly inhibits virus replication and improves the acute lung injury induced by influenza A and B viruses (80). The synergistic activity of baicalein and ribavirin against influenza virus infections in cell culture and mice was investigated by Chen, and it was discovered that, compared with ribavirin alone, the combination of baicalein and ribavirin increased the survival rate in mice infected with a lethal dose of H1N1 influenza virus (81). Furthermore, baicalein and acacetin can significantly reduce airway hyperresponsiveness, inflammation, and mucus production (82, 83). Acacia can resist plasmodium and regulate immunity (84). Most key compounds have antiviral, anti-inflammatory, and immune-regulating effects. Therefore, it can be inferred that they treat 2019-nCoV mainly through anti-inflammatory, antiviral, and immune-regulating activities.
(II). Others
Flavonoids and terpenoids are natural antiviral compounds. Artemisinin is a commonly used anti-malarial terpenoid compound with a broad-spectrum antiviral activity that has recently been repurposed as a potential COVID-19 drug. Recent studies have confirmed the antiviral activity of artemisinin and its derivatives against SARS-2-CoV-2 at micromolar concentrations (85, 86). Several clinical trials have shown that artemisinin-related agents can help improve SARS-CoV-2 clearance, shorten the time to conversion of SARS-CoV-2 tests to negative, improve the patient condition, and contribute to faster recovery of COVID-19 patients (87, 88). In addition, artemisinin has anti-inflammatory activity and reduces systemic levels of inflammatory cytokines that contribute to cytokine storm and inflammatory organ damage in high-risk COVID-19 patients (89). Aloe-emodin can impede coronaviruses’ entry, replication, and release (90–92). In molecular docking, aloe-emodin has an excellent binding ability to the main protease (6LU7) and nucleocapsid phosphoprotein of COVID-19. It can be used as a potential inhibitor of anti-COVID-19 main protease and a potential drug to cure SARS-CoV-2 infection (93, 94).
Target analysis of traditional inhibitors and four Chinese patent medicines
According to the current status of COVID-19 therapy, the targets of COVID-19 inhibitors involve four main areas, which are briefly described as follows.
(1) Antiviral drugs: SARS-CoV-2 main protease inhibitors (95–98), HIV protease inhibitors (99), RNA-dependent RNA polymerase inhibitors (100).
(2) Cytokine monoclonal antibodies/inhibitors: IL6 monoclonal antibodies, complement component 5 inhibitors, PD-1 blocking antibody, human granulocyte-macrophage colony-stimulating factor receptor inhibitors, IL17A antagonist, IL1β antibody, vascular endothelial-derived growth factor antibody, IL1 receptor antagonist, anti-C5a receptor antibody, and tumor necrosis factor-α inhibitor (101).
(3) Interferons: IFN-α1b Eye Drops, IFN-β1b, IFN-β1a, IFN atomization, IFN-α1b spray, recombinant super-compound IFN, and IFN aerosol inhalation (101).
(4) Others: RAAS inhibitors (102, 103), JAK inhibitors (104), cathepsin L-selective inhibitors, and proton pump inhibitors (105).
At the same time, TCM differs from chemical drugs in that they usually have simple and straightforward targets of action, while CPMs consist of multiple herbs with multiple targets, and their specific targets of action are relatively complex (106). However, the therapeutic targets of the four CPM in the treatment of COVID-19 protocal are consistent with traditional COVID-19 inhibitors, which further confirms its feasibility. The targets of action of four CPMs for the treatment of COVID-19 are reviewed below. Some of them may still be in the theoretical stage, pending further basic experiments.
➀ LHQW
Nanshan Zhong et al. infected Vero-E6 cells and Huh-7 cells with clinically isolated SARS-CoV-2 virus and assessed the antiviral activity of LHQW against SARS-CoV-2 using CPE and plaque reduction assays, and measured the expression levels of pro-inflammatory cytokines in Huh-7 cells by real-time quantitative PCR. The results showed that LHQW significantly inhibited SARS-CoV-2 replication in Vero E6 cells and reduced the production of pro-inflammatory cytokines such as IL-6, TNF-α, CCL-2/MCP-1, and CXCL-10/IP-10 (13).
➁ JHQG
JHQG is the first TCM prescription for the treatment of H1N1 infection. Its potential mechanism of action for the treatment of COVID-19 is anti-inflammatory, anti-SARS-CoV-2 (107, 108), mainly through the regulation of interleukin receptors (IL-1A, IL-1B, IL-2, IL-4, IL-6, IL-10), mitogen-activated protein kinase receptors (MAPK1, MAPK3, MAPK8, MAPK14), TNF and other significant targets, thus achieving the treatment of COVID-19 (109–112). JHQG is derived from the combined formula of Yin Qiao San (YQS) and Ma Xing Shi Gan Tang (MXSG) with addition and reduction. Cellular experiments have shown that MXSG can regulate the expression and activation level of the Toll-like receptor (TLR) in the immune response pathway, which regulates the secretion and protein expression of IFN and effectively fights against viruses (113). Animal experiments showed that YQS could antagonize the function of influenza virus non-structural protein NS1, inhibit the expression of the JAK/STAT signaling pathway and promote the production of antiviral protein (Mx A) induced by IFN-α (114).
➂ HXZQ
The potential mechanism of action of HXZQ for COVID-19 is inhibiting SARS-CoV-2 replication, improving immune, and anti-inflammatory, mainly by acting on 3CLpro (115), PTGS2, AR, HSP90AB1, CAMSAP2, PPARG, NOS2, and other targets (116–118).
➃ SFJD
The potential mechanism of action of SFJD for the treatment of COVID-19 is anti-inflammatory, anti-SARS-CoV-2, mainly acting on mitogen-activated protein kinase receptors (MAPK1, MAPK3, MAPK8, MAPK14), interleukin receptors (IL-6, IL-10), TNF, PTGS2, CASP3, and other multiple targets to achieve the therapeutic effect on COVID-19 (119, 120). In SFJD alone or combined with oseltamivir for the treatment of chronic obstructive pulmonary disease induced by influenza A virus, SFJDC alone and combined with oseltamivir were found to control airway inflammation and lung injury in IAV-infected rats by modulating NLRP3 inflammatory vesicles and subsequently downregulating IL-1β and IL-18 (15). The results of experiments in yeast thermogenesis model rats confirmed the significant antipyretic effect of SFJD, which can significantly inhibit the production of inflammatory factors PGE2, thus considerably inhibiting the production of thermogenic cytokines TNF-α, IL-1α, IL-1β, IL-6. It reduces the amount of thermogenic factors cAMP, Na+, K+-ATPase, decreases cAMP/cGMP, reduces heat production, and increases the amount of endogenous antipyretic mediator AVP, thus exerting its antipyretic effect (121). The immunomodulatory effect of SFJD on rats with pneumonia was further verified in a rat model of pneumonia caused by streptococcus pneumonia. It has a therapeutic impact on pneumonia model rats by reducing B lymphocytes, CD8+ ratio, and elevated levels of IL-1α, IL-1β, IL-2, IL-10, TNF-α, IFN-α, IFN-γ, IgM, IgG, CD4+/CD8+, and NK cells (122).
Analysis of the common action targets of four Chinese patent medicines
In the post-genetic era, researchers have recognized that many high-incidence diseases involve multifactorial physiological and pathological factors (123). Multi-target or multi-component therapeutics will receive increasing attention, and traditional Chinese medicine may become an essential resource for multi-component multi-target drug design (124). In this study, a total of 11 hub genes were identified through two plugins in cytoscape, cytoHubba, and Cluego. These hub genes are primarily inflammatory mediator cytokines, which are consistent with four CPM targets, including interleukins (IL-2, IL-4, IL-6, CXCL8, IL-10, and IL-1B), interferon (IFNG), tumor necrosis factor (TNF), chemokine (CCL2), and adhesion molecule (ICAM1). A study published in the Lancet notes that the occurrence and development of 2019-nCoV are closely related to cytokine storms. Cytokine storms are severe systemic reactions caused by the overactivation of the immune system by infection, drugs, or certain diseases, which can lead to multiple organ failure and even death (125). In patients with severe and critical COVID-19, the immune system is disrupted, thereby producing large amounts of inflammatory cytokines, which leads to life-threatening cytokine storms (126). Therefore, it is necessary to detect and interfere with inflammatory cytokines as soon as possible and initiate immunotherapy, aiming to avoid the progression of mild and common infections to severe and critical disease. This necessity further demonstrates the feasibility of the four prescriptions for the treatment of 2019-nCoV.
Pathway analysis
According to the KEGG enrichment analysis results of the potential targets, it can be inferred that the four prescriptions can achieve therapeutic effects against COVID-19 by targeting antiviral, inflammatory response, immune regulation, cell apoptosis, and other pathways. The signaling pathways mainly focus on three aspects: (1) Antivirus. Among the top 39 signaling pathways, 12 are related to antiviral, such as Coronavirus disease—COVID-19. (2) Regulation of the immune system. There are six pathways associated with immune regulation. The overactivation of the immune system leads to a cytokine storm, an essential step in transforming from mild to severe diseases. The four prescriptions may play a role in modulating immunity, avoiding or mitigating cytokine storms by acting on the T cell receptor signaling pathway, Toll-like receptor signaling pathway, NOD-like receptor signaling pathway, Th1, and Th2 cell differentiation, Chemokine signaling pathway, and Fc epsilon RI signaling pathway by interfering with a range of pathophysiological processes, including the recruitment, activation, and secretion of immune cells. (3) Inflammatory response. There are four signaling pathways involved in inflammation, including the IL-17 signaling pathway, Th17 cell differentiation, C-type lectin receptor signaling pathway, and TNF signaling pathway. To sum up, the four CPMs mainly treat COVID-19 through anti-virus, immune regulation, and anti-inflammatory. Twenty-two of the top 39 signaling pathways can be attributed to these three areas, as shown in Table 5. The specific mechanisms of action of the four CPMs on the Coronavirus disease—COVID-19 pathway are shown in Figure 12.
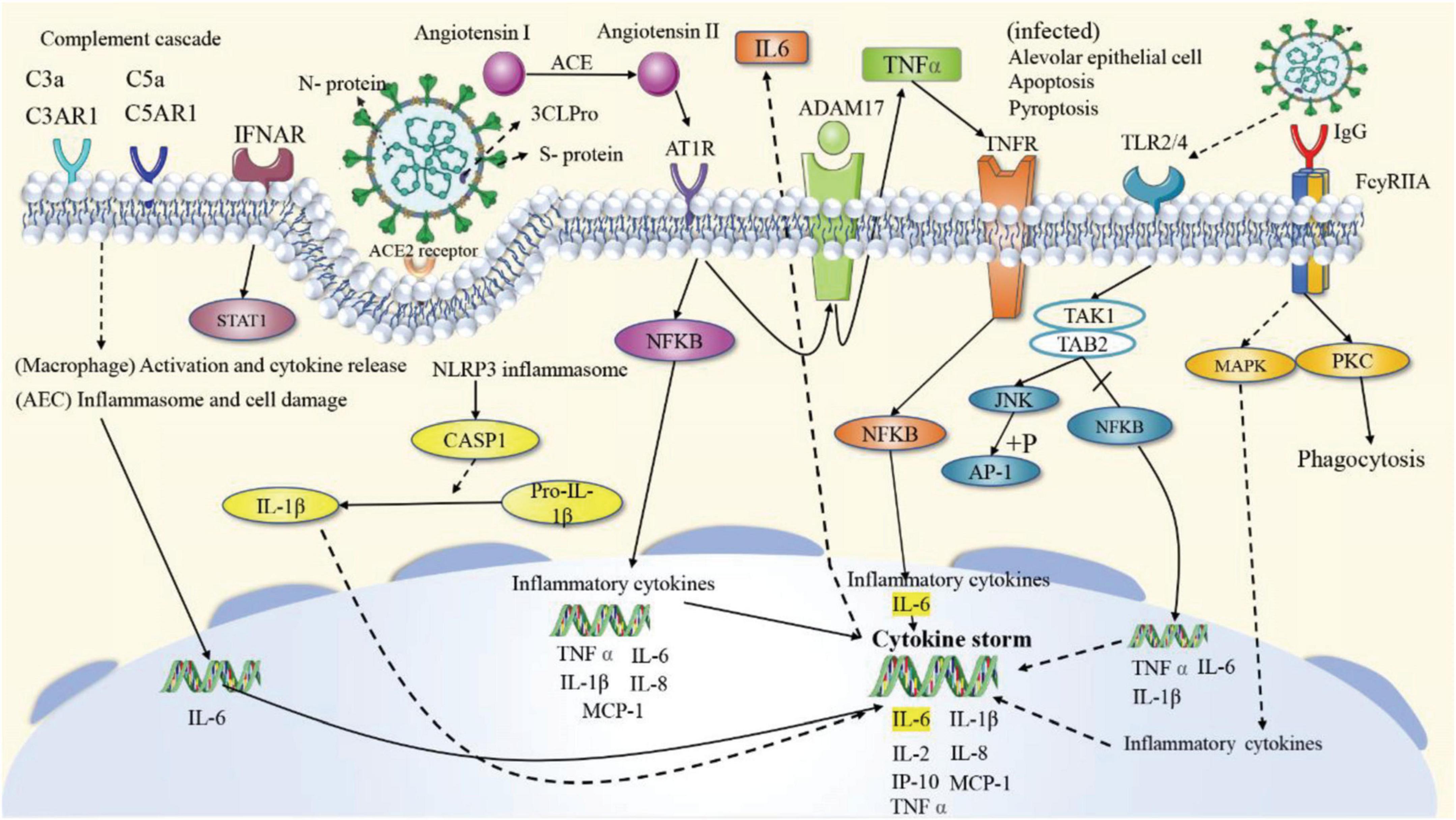
Figure 12. The specific mechanisms of action of the four CPMs on the Coronavirus disease—COVID-19 pathway. The solid arrows represent activation, the dashed arrows represent indirect effects, and the vertical lines above the arrows represent inhibition.
Conclusion
TCM is the strength and highlight of China’s COVID-19 prevention and control plan. In the fight against COVID-19, TCM has been involved in the prevention, treatment, and rehabilitation. TCM and Western medicine complement and cooperate, which makes an essential contribution to the comprehensive control of COVID-19. In this study, the possible common mechanism of the action of four classic CPMs in treating COVID-19 has been unearthed. Consequently, 101 common active ingredients and 233 common targets were screened out. There were 51 potential targets and 92 related pathways associated with COVID-19. Further analysis revealed that the active ingredients might be flavonoids, such as quercetin, luteolin, and acacetin. The enrichment analysis of 51 potential targets indicated that these targets could be applied to treat COVID-19 by activating broad spectrum antiviral, immune regulatory, and inflammatory responses through the regulation of the Coronavirus disease—COVID-19, cytokine-cytokine receptor interaction, IL-17 signaling pathway, and others. Due to intra-laboratory and multiple conditions limitations, this study explored the common mechanism of action of four PCMs for treating COVID-19 through network pharmacology and molecular dynamics with some limitations. In the future, we will further validate the candidate targets and potential signaling pathways predicted in this study by in vivo and in vitro experiments. China is willing to unite people from all countries to fight the epidemic and share the experience and achievements of Chinese medicine.
Data availability statement
The datasets presented in this study can be found in online repositories. The names of the repository/repositories and accession number(s) can be found in the article/Supplementary material.
Author contributions
HY directed the research and revised the manuscript. LW and ZW performed the research and wrote the manuscript. XW modified the tables and figures and revised the manuscript. LY, JW, and YL revised the manuscript. All authors have read and approved the manuscript.
Funding
This work was supported by the National Natural Science Foundation of China (81873263).
Acknowledgments
We are thankful to Tianjin University of Traditional Chinese Medicine for the help in conducting this study.
Conflict of interest
The authors declare that the research was conducted in the absence of any commercial or financial relationships that could be construed as a potential conflict of interest.
Publisher’s note
All claims expressed in this article are solely those of the authors and do not necessarily represent those of their affiliated organizations, or those of the publisher, the editors and the reviewers. Any product that may be evaluated in this article, or claim that may be made by its manufacturer, is not guaranteed or endorsed by the publisher.
Supplementary material
The Supplementary Material for this article can be found online at: https://www.frontiersin.org/articles/10.3389/fmed.2022.874611/full#supplementary-material
Footnotes
- ^ http://tcmspw.com/tcmsp.php, Ver. 2.3.
- ^ http://119.3.41.228:8000/tcmid/
- ^ http://www.talete.mi.it/products/dragon_description.htm
- ^ https://go.drugbank.com/
- ^ https://www.uniprot.org/
- ^ http://genoweb.toulouse.inra.fr:8091/app/example.html
- ^ https://www.genecards.org/
- ^ https://www.ncbi.nlm.nih.gov/
- ^ http://string-db.org/, ver. 11.0.
- ^ www.cytoscape.org/
- ^ http://apps.cytoscape.org/apps/cytohubba
References
1. Cohen MS, Wohl DA, Fischer WA II, Smith DM, Eron JJ. Outpatient treatment of severe acute respiratory syndrome coronavirus 2 infection to prevent coronavirus disease 2019 progression. Clin Infect Dis. (2021) 73:1717–21. doi: 10.1093/cid/ciab494
2. Lai C-C, Shih T-P, Ko W-C, Tang H-J, Hsueh P-R. Severe acute respiratory syndrome coronavirus 2 (SARS-CoV-2) and corona virus disease-2019 (Covid-19): the epidemic and the challenges. Int J Antimicrob Agents. (2020) 55:105924. doi: 10.1016/j.ijantimicag.2020.105924
3. Vabret N, Britton GJ, Gruber C, Hegde S, Kim J, Kuksin M, et al. Immunology of Covid-19: current state of the science. Immunity. (2020) 52:910–41. doi: 10.1016/j.immuni.2020.05.002
4. Dong M, Zhang J, Ma X, Tan J, Chen L, Liu S, et al. Ace2, Tmprss2 distribution and extrapulmonary organ injury in patients with Covid-19. Biomed Pharmacother. (2020) 131:110678. doi: 10.1016/j.biopha.2020.110678
5. Wang K, Chen W, Zhang Z, Deng Y, Lian J-Q, Du P, et al. Cd147-spike protein is a novel route for SARS-CoV-2 infection to host cells. Signal Transduct Target Ther. (2020) 5:1–10. doi: 10.1038/s41392-020-00426-x
6. Zhao Y-M, Shang Y-M, Song W-B, Li Q-Q, Xie H, Xu Q-F, et al. Follow-up study of the pulmonary function and related physiological characteristics of Covid-19 survivors three months after recovery. EClinicalMedicine. (2020) 25:100463. doi: 10.1016/j.eclinm.2020.100463
7. Luo H, Gao Y, Zou J, Zhang S, Chen H, Liu Q, et al. Reflections on treatment of Covid-19 with traditional Chinese medicine. Chin Med. (2020) 15:1–14. doi: 10.1186/s13020-020-00375-1
8. Hu K, Guan W-J, Bi Y, Zhang W, Li L, Zhang B, et al. Efficacy and safety of lianhuaqingwen capsules, a repurposed Chinese herb, in patients with coronavirus disease 2019: a multicenter, prospective, randomized controlled trial. Phytomedicine. (2021) 85:153242.
9. Ling C, Fang L, Jinhu W, Yan SJ, Jinshen X, Bi S, et al. Clinical efficacy of Shufeng Jiedu capsule combined with western medicine in treatment of common Covid-19 patients by retrospective analysis. Chin J Exp Tradit Med Formul. (2020) 26:14–20.
10. Can D, Wenguang X, Chanjuan Z, Guobing S, Zhengliang L, Qinglin L, et al. Clinical observation on Jinhua Qinggan granule combined with conventional western medicine therapy in treating mild cases of coronavirus. J Tradit Chin Med. (2020) 61:1473–7.
11. Wenguang X, Changging A, Chanjuan Z, Jixian Z, Min H, Yu W, et al. Clinical observation on 34 patients with novel coronavirus pneumonia (Covid-19) treated with intergrated traditional Chinese and Western medicine. J Tradit Chin Med. (2020) 61:375–82.
12. Zhuang W, Fan Z, Chu Y, Wang H, Yang Y, Wu L, et al. Chinese patent medicines in the treatment of coronavirus disease 2019 (Covid-19) in China. Front Pharmacol. (2020) 11:1066. doi: 10.3389/fphar.2020.01066
13. Runfeng L, Yunlong H, Jicheng H, Weiqi P, Qinhai M, Yongxia S, et al. Lianhuaqingwen exerts anti-viral and anti-inflammatory activity against novel coronavirus (SARS-CoV-2). Pharmacol Res. (2020) 156:104761. doi: 10.1016/j.phrs.2020.104761
14. Yanyan B, Yingjie G, Yujing S, Lei B, Rongmei Y, Xin M, et al. Study on broad-spectrum antiviral effect of Shufeng Jiedu capsules. J New Chin Med. (2019) 51:5–8.
15. Ji S, Bai Q, Wu X, Zhang D-W, Wang S, Shen J-L, et al. Unique synergistic antiviral effects of Shufeng Jiedu capsule and oseltamivir in influenza a viral-induced acute exacerbation of chronic obstructive pulmonary disease. Biomed Pharmacother. (2020) 121:109652. doi: 10.1016/j.biopha.2019.109652
16. Jing L, Li M, Jie L, Xingmei T, Jun X, Xiuping S, et al. Study on the antipyretic mechanism of Shufeng Jiedu capsules. Chin Tradit Herb Drugs. (2016) 47:2040–3.
17. Li M, Yan H, Yanbao H, Dongdong Z, Yanpeng Z, Jing L, et al. Study on mechanism for immunoregulation of Shufeng Jiedu capsule. Drug Eval Res. (2019) 42:1763–8.
18. Zhi-peng H, Yuan-xue L, Lel H, Yu W, Yi H, Shui-ping Z, et al. Research progress on potential application of Huoxiang Zhengqi in prevention and treatment of coronavirus disease 2019. Drugs Clin. (2020) 35:405–10.
19. Ling L, Ke W, Fang-guo L, Liang C, Bo Z, Shi-ying Z, et al. Effect of maxing Shigan decoction against type A influenza virus infection in mice induced by viral lung injury based on Tlr4-Myd88-Traf6 signal pathways. Chin Tradit Herb Drugs. (2017) 48:1591–6.
20. Ling L, Jia-Min W, Jian-Jun OY, Jue H, Can L, Pin Y, et al. Study on screening and mechanism of effective Chinese medicine for influenza virus pneumonia. Chin J Immunol. (2018) 34:1168–73.
21. da Hao C, Xiao PG. Network pharmacology: a rosetta stone for traditional Chinese medicine. Drug Dev Res. (2014) 75:299–312. doi: 10.1002/ddr.21214
22. Zhu B, Zhang W, Yang L, Hu S, Rui G, Sun Z, et al. Network pharmacology-based identification of protective mechanism of Panax notoginseng saponins on aspirin induced gastrointestinal injury. Biomed Pharmacother. (2018) 105:159–66. doi: 10.1016/j.biopha.2018.04.054
23. Huang J, Fan C, Tan HY, Hong M, Zheng Q. Identification of the active compounds and significant pathways of Yinchenhao decoction based on network pharmacology. Mol Med Rep. (2017) 16:4583–92. doi: 10.3892/mmr.2017.7149
24. Liu H, Wang J, Zhou W, Wang Y, Yang LJ. Systems approaches and polypharmacology for drug discovery from herbal medicines: an example using licorice. J Ethnopharmacol. (2013) 146:773–93. doi: 10.1016/j.jep.2013.02.004
25. Xu X, Zhang W, Huang C, Li Y, Yu H, Wang Y, et al. A novel chemometric method for the prediction of human oral bioavailability. Int J Mol Sci. (2012) 13:6964–82. doi: 10.3390/ijms13066964
26. Walters WP, Murcko MAJ. Prediction of ‘drug-likeness’. Adv Drug Deliv Rev. (2002) 54:255–71. doi: 10.1016/S0169-409X(02)00003-0
27. Yoshihiro Y, Masaaki K, Minoru K, Susumu G. Drug-target interaction prediction from chemical, genomic and pharmacological data in an integrated framework. Bioinformatics. (2010) 26:i246–54. doi: 10.1093/bioinformatics/btq176
28. Mauri A, Consonni V, Pavan M, Todeschini R. Dragon software: an easy approach to molecular descriptor calculations. Match. (2006) 56:237–48.
29. Huang J, Tang H, Cao S, He Y, Feng Y, Wang K, et al. Molecular targets and associated potential pathways of Danlu capsules in hyperplasia of mammary glands based on systems pharmacology. Evid Based Complement Alternat Med. (2017) 2017:1930598. doi: 10.1155/2017/1930598
30. Yang L, Liu W, Hu Z, Yang M, Li J, Fan X, et al. A systems pharmacology approach for identifying the multiple mechanisms of action of the Wei Pi Xiao decoction for the treatment of gastric precancerous lesions. Evid Based Complement Alternat Med. (2019) 2019:1562707. doi: 10.1155/2019/1562707
31. Bardou P, Mariette J, Escudié F, Djemiel C, Klopp C. Jvenn: an interactive Venn diagram viewer. BMC Bioinformatics. (2014) 15:293. doi: 10.1186/1471-2105-15-293
32. Piñero J, Queralt-Rosinach N, Bravo A, Deu-Pons J, Bauer-Mehren A, Baron M, et al. Disgenet: a discovery platform for the dynamical exploration of human diseases and their genes. Database. (2015) 2015:bav028. doi: 10.1093/database/bav028
33. Stelzer G, Rosen N, Plaschkes I, Zimmerman S, Twik M, Fishilevich S, et al. The GeneCards suite: from gene data mining to disease genome sequence analyses. Curr Protoc Bioinformatics. (2016) 54:1.30.1–33. doi: 10.1002/cpbi.5
34. Feindt W, Oppenheim SJ, DeSalle R, Mehr S. Good citizenship made easy: a step−by−step guide to submitting Rna−Seq data to Ncbi. Curr Protoc Bioinformatics. (2018) 64:e67. doi: 10.1002/cpbi.67
35. Szklarczyk D, Morris JH, Cook H, Kuhn M, Wyder S, Simonovic M, et al. The string database in 2017: quality-controlled protein–protein association networks, made broadly accessible. Nucleic Acids Res. (2016) 45:D362–8. doi: 10.1093/nar/gkw937
36. Chin C-H, Chen S-H, Wu H-H, Ho C-W, Ko M-T, Lin C-Y. Cytohubba: identifying hub objects and sub-networks from complex interactome. BMC Syst Biol. (2014) 8:S11. doi: 10.1186/1752-0509-8-S4-S11
37. Ashburner M, Ball CA, Blake JA, Botstein D, Cherry JM. Gene ontology: tool for the unification of biology. Nat Genet. (2000) 25:25–9. doi: 10.1038/75556
38. The Gene Ontology Consortium. Expansion of the gene ontology knowledgebase and resources. Nucleic Acids Res. (2017) 45:D331–8. doi: 10.1093/nar/gkw1108
39. Yu G, Wang L-G, Han Y, He Q-Y. Clusterprofiler: an R package for comparing biological themes among gene clusters. OMICS. (2012) 16:284–7. doi: 10.1089/omi.2011.0118
40. Luan J, Lu Y, Jin X, Zhang L. Spike protein recognition of mammalian Ace2 predicts the host range and an optimized Ace2 for SARS-CoV-2 infection. Biochem Biophys Res Commun. (2020) 526:165–9. doi: 10.1016/j.bbrc.2020.03.047
41. Ibrahim IM, Abdelmalek DH, Elshahat ME, Elfiky AA. Covid-19 spike-host cell receptor Grp78 binding site prediction. J Infect. (2020) 80:554–62. doi: 10.1016/j.jinf.2020.02.026
42. Boopathi, S, Poma AB, Kolandaivel P. Novel 2019 coronavirus structure, mechanism of action, antiviral drug promises and rule out against its treatment. J Biomol Struct Dyn. (2020) 39:3409–18. doi: 10.1080/07391102.2020.1758788
44. Trott O, Olson AJ. Autodock Vina: improving the speed and accuracy of docking with a new scoring function, efficient optimization, and multithreading. J Comput Chem. (2010) 31:455–61. doi: 10.1002/jcc.21334
45. Cvetkovic RS, Goa KL. Lopinavir/Ritonavir. Drugs. (2003) 63:769–802. doi: 10.2165/00003495-200363080-00004
46. Loustaud-Ratti V, Debette-Gratien M, Jacques J, Alain S, Marquet P, Sautereau D, et al. Ribavirin: past, present and future. World J Hepatol. (2016) 8:123. doi: 10.4254/wjh.v8.i2.123
47. Holshue ML, DeBolt C, Lindquist S, Lofy KH, Wiesman J, Bruce H, et al. First case of 2019 novel coronavirus in the United States. New Engl J Med. (2020) 382:929–36. doi: 10.1056/NEJMoa2001191
48. Xiong Y, Gao M, van Duijn B, Choi H, van Horssen F, Wang M. International policies and challenges on the legalization of traditional medicine/herbal medicines in the fight against Covid-19. Pharmacol Res. (2021) 166:105472. doi: 10.1016/j.phrs.2021.105472
49. Du W, Zhu M, Xiao X, Jiang M, Luo G, Zhang S, et al. Research on the pharmacodynamic material basis of compound traditional Chinese medicine and the problems that should be paid attention to in the future. Lishizhen Med Materia Med Res. (2013) 24:692–4. doi: 10.1016/j.jconrel.2009.05.008
50. Zhou X, Seto SW, Chang D, Kiat H, Razmovski-Naumovski V, Chan K, et al. Synergistic effects of Chinese herbal medicine: a comprehensive review of methodology and current research. Front Pharmacol. (2016) 7:201. doi: 10.3389/fphar.2016.00201
51. Yi H, Duan L, Geng Z. Research progress on the material basis of pharmacodynamics of traditional Chinese medicine compounds. Asia Pac Tradit Med. (2021) 17:210–3.
52. Wang L, Zhou GB, Liu P, Song JH, Liang Y, Yan XJ, et al. Dissection of mechanisms of Chinese medicinal formula realgar-indigo naturalis as an effective treatment for promyelocytic leukemia. Proc Natl Acad Sci U.S.A. (2008) 105:4826–31. doi: 10.1073/pnas.0712365105
53. Stone R. Biochemistry. Lifting the veil on traditional Chinese medicine. Science. (2008) 319:709–10. doi: 10.1126/science.319.5864.709
54. Zhang X, Liu Y, Guo Z, Feng J, Dong J, Fu Q, et al. The herbalome–an attempt to globalize Chinese herbal medicine. Anal Bioanal Chem. (2012) 402:573–81. doi: 10.1007/s00216-011-5533-y
55. Rengasamy KR, Khan H, Gowrishankar S, Lagoa RJ, Mahomoodally FM, Khan Z, et al. The role of flavonoids in autoimmune diseases: therapeutic updates. Pharmacol Therap. (2019) 194:107–31. doi: 10.1016/j.pharmthera.2018.09.009
56. Gandhi GR, Neta MTSL, Sathiyabama RG, Quintans JDSS, e Silva AMDO, de Souza Araujo AA, et al. Flavonoids as Th1/Th2 cytokines immunomodulators: a systematic review of studies on animal models. Phytomedicine. (2018) 44:74–84. doi: 10.1016/j.phymed.2018.03.057
57. Jo S, Kim S, Shin DH, Kim M-S. Inhibition of sars-Cov 3cl protease by flavonoids. J Enzyme Inhibit Med Chem. (2020) 35:145–51. doi: 10.1080/14756366.2019.1690480
58. Lin W, Wang W, Wang D, Ling W. Quercetin protects against atherosclerosis by inhibiting dendritic cell activation. Mol Nutr Food Res. (2017) 61:1700031. doi: 10.1002/mnfr.201700031
59. Jo S, Kim H, Kim S, Shin DH, Kim MS. Characteristics of flavonoids as potent Mers−Cov 3c−like protease inhibitors. Chem Biol Drug Design. (2019) 94:2023–30. doi: 10.1111/cbdd.13604
60. Gerin F, Sener U, Erman H, Yilmaz A, Aydin B, Armutcu F, et al. The effects of quercetin on acute lung injury and biomarkers of inflammation and oxidative stress in the rat model of sepsis. Inflammation. (2016) 39:700–5. doi: 10.1007/s10753-015-0296-9
61. Li Y, Yao J, Han C, Yang J, Chaudhry MT, Wang S, et al. Quercetin, inflammation and immunity. Nutrients. (2016) 8:167. doi: 10.3390/nu8030167
62. Casas−Grajales S, Vázquez−Flores LF, Ramos−Tovar E, Hernández−Aquino E, Flores−Beltrán RE, Cerda−García−Rojas CM, et al. Quercetin reverses experimental cirrhosis by immunomodulation of the proinflammatory and profibrotic processes. Fundament Clin Pharmacol. (2017) 31:610–24. doi: 10.1111/fcp.12315
63. Valentová K, Šíma P, Rybková Z, Køížan J, Malachová K, Køen V. (Anti) mutagenic and immunomodulatory properties of quercetin glycosides. J Sci Food Agric. (2016) 96:1492–9. doi: 10.1002/jsfa.7251
64. Wu SN, Chiang HT, Shen AY, Lo YK. Differential effects of quercetin, a natural polyphenolic flavonoid, on L−type calcium current in pituitary tumor (Gh3) cells and neuronal Ng108−15 cells. J Cell Physiol. (2003) 195:298–308. doi: 10.1002/jcp.10244
65. Wu CP, Calcagno AM, Hladky SB, Ambudkar SV, Barrand MA. Modulatory effects of plant phenols on human multidrug−resistance proteins 1, 4 and 5 (Abcc1, 4 and 5). FEBS J. (2005) 272:4725–40. doi: 10.1111/j.1742-4658.2005.04888.x
66. Thilakarathna SH, Rupasinghe H. Flavonoid bioavailability and attempts for bioavailability enhancement. Nutrients. (2013) 5:3367–87. doi: 10.3390/nu5093367
67. Gutiérrez-Venegas G, Torras-Ceballos A, Gómez-Mora JA, Fernández-Rojas B. Luteolin, quercetin, genistein and quercetagetin inhibit the effects of lipopolysaccharide obtained from Porphyromonas gingivalis in H9c2 cardiomyoblasts. Cell Mol Biol Lett. (2017) 22:1–12. doi: 10.1186/s11658-017-0047-z
68. Tu D-G, Lin W-T, Yu C-C, Lee S-S, Peng C-Y, Lin T, et al. Chemotherapeutic effects of luteolin on radio-sensitivity enhancement and interleukin-6/signal transducer and activator of transcription 3 signaling repression of oral cancer stem cells. J Formos Med Assoc. (2016) 115:1032–8. doi: 10.1016/j.jfma.2016.08.009
69. Abdallah HM, Esmat A. Antioxidant and Anti-inflammatory activities of the major phenolics from Zygophyllum simplex L. J Ethnopharmacol. (2017) 205:51–6. doi: 10.1016/j.jep.2017.04.022
70. Zhao Y, Xu Y, Li Y, Jin Q, Sun J, Zhiqiang E, et al. Supplementation of kaempferol to in vitro maturation medium regulates oxidative stress and enhances subsequent embryonic development in vitro. Zygote. (2020) 28:59–64. doi: 10.1017/S0967199419000674
71. Behbahani M, Sayedipour S, Pourazar A, Shanehsazzadeh M. In vitro anti-Hiv-1 activities of kaempferol and kaempferol-7-o-glucoside isolated from Securigera securidaca. Res Pharm Sci. (2014) 9:463.
72. Mir IA, Tiku AB. Chemopreventive and therapeutic potential of “naringenin,” a flavanone present in citrus fruits. Nutr Cancer. (2015) 67:27–42. doi: 10.1080/01635581.2015.976320
73. Lyu S-Y, Rhim J-Y, Park W-B. Antiherpetic activities of flavonoids against herpes simplex virus type 1 (Hsv-1) and type 2 (Hsv-2) in vitro. Arch Pharm Res. (2005) 28:1293–301. doi: 10.1007/BF02978215
74. Liu A-L, Wang H-D, Lee SM, Wang Y-T, Du G-H. Structure–activity relationship of flavonoids as influenza virus neuraminidase inhibitors and their in vitro anti-viral activities. Bioorg Med Chem. (2008) 16:7141–7. doi: 10.1016/j.bmc.2008.06.049
75. Evers DL, Chao C-F, Wang X, Zhang Z, Huong S-M, Huang E-S. Human cytomegalovirus-inhibitory flavonoids: studies on antiviral activity and mechanism of action. Antiviral Res. (2005) 68:124–34. doi: 10.1016/j.antiviral.2005.08.002
76. Huang B, Liu J, Ju C, Yang D, Chen G, Xu S, et al. Licochalcone a prevents the loss of dopaminergic neurons by inhibiting microglial activation in lipopolysaccharide (Lps)-induced Parkinson’s disease models. Int J Mol Sci. (2017) 18:2043. doi: 10.3390/ijms18102043
77. Lien L-M, Lin K-H, Huang L-T, Tseng M-F, Chiu H-C, Chen R-J, et al. Licochalcone a prevents platelet activation and thrombus formation through the inhibition of Plcγ2-Pkc, Akt, and Mapk pathways. Int J Mol Sci. (2017) 18:1500. doi: 10.3390/ijms18071500
78. Cinatl J, Morgenstern B, Bauer G, Chandra P, Rabenau H, Doerr H. Glycyrrhizin, an active component of liquorice roots, and replication of Sars-associated coronavirus. Lancet. (2003) 361:2045–6. doi: 10.1016/S0140-6736(03)13615-X
79. Wu D, Wang Y, Zhang H, Du M, Li TJI. Acacetin attenuates mice endotoxin-induced acute lung injury via augmentation of heme oxygenase-1 activity. Inflammopharmacology. (2018) 26:635–43. doi: 10.1007/s10787-017-0398-0
80. Seong R, Kim J, Shin O. Wogonin, a flavonoid isolated from Scutellaria baicalensis, has anti-viral activities against influenza infection via modulation of Ampk pathways. Acta Virol. (2018) 62:78–85. doi: 10.4149/av_2018_109
81. Chen L, Dou J, Su Z, Zhou H, Wang H, Zhou W, et al. Synergistic activity of baicalein with ribavirin against influenza a (H1n1) virus infections in cell culture and in mice. Antiviral Res. (2011) 91:314–20. doi: 10.1016/j.antiviral.2011.07.008
82. Liou C-J, Wu S-J, Chen L-C, Yeh K-W, Chen C-Y, Huang W-C. Acacetin from traditionally used saussurea involucrata Kar. Et Kir. Suppressed adipogenesis in 3t3-L1 adipocytes and attenuated lipid accumulation in obese mice. Front Pharmacol. (2017) 8:589. doi: 10.3389/fphar.2017.00589
83. Xu T, Ge X, Lu C, Dai W, Chen H, Xiao Z, et al. Baicalein attenuates ova-induced allergic airway inflammation through the inhibition of the Nf-K b signaling pathway. Aging. (2019) 11:9310–27. doi: 10.18632/aging.102371
84. Zhao N, Dong Q, Fu X-X, Du L-L, Cheng X, Du Y-M, et al. Acacetin blocks Kv1. 3 channels and inhibits human T cell activation. Cell Physiol Biochem. (2014) 34:1359–72. doi: 10.1159/000366343
85. Cao R, Hu H, Li Y, Wang X, Xu M, Liu J, et al. Anti-SARS-CoV-2 potential of artemisinins in vitro. ACS Infect Dis. (2020) 6:2524–31.
86. Gilmore K, Zhou Y, Ramirez S, Pham LV, Fahnøe U, Feng S, et al. In vitro efficacy of artemisinin-based treatments against SARS-CoV-2. Sci Rep. (2020) 11:1–14. doi: 10.1038/s41598-021-93361-y
87. Li G, Yuan M, Li H, Deng C, Wang Q, Tang Y, et al. Safety and efficacy of artemisinin-piperaquine for treatment of Covid-19: an open-label, non-randomised and controlled trial. Int J Antimicrob Agents. (2021) 57:106216. doi: 10.1016/j.ijantimicag.2020.106216
88. Lin Y, Wu F, Xie Z, Song X, Zhu Q, Wei J, et al. Clinical study of artesunate in the treatment of coronavirus disease 2019. Zhonghua Wei Zhong Bing Ji Jiu Yi Xue. (2020) 32:417–20.
89. Uckun FM, Saund S, Windlass H, Trieu VJ. Repurposing anti-malaria phytomedicine artemisinin as a Covid-19 drug. Front Pharmacol. (2021) 12:649532. doi: 10.3389/fphar.2021.649532
90. El Mazouri S, Aanniz T, Touhtouh J, Kandoussi I, Hakmi M, Belyamani L, et al. Anthraquinones: a promising multi-target therapeutic scaffold to treat Covid-19. Int J Appl Biol Pharm Technol. (2021) 12:338–55. doi: 10.26502/ijabpt.202104
91. Chang S-J, Huang S-H, Lin Y-J, Tsou Y-Y, Lin C-W. Antiviral activity of rheum palmatum methanol extract and chrysophanol against Japanese encephalitis virus. Arch Pharm Res. (2014) 37:1117–23. doi: 10.1007/s12272-013-0325-x
92. Lin C-W, Tsai F-J, Tsai C-H, Lai C-C, Wan L, Ho T-Y, et al. Anti-Sars coronavirus 3c-like protease effects of isatis indigotica root and plant-derived phenolic compounds. Antiviral Res. (2005) 68:36–42. doi: 10.1016/j.antiviral.2005.07.002
93. Rolta R, Yadav R, Salaria D, Trivedi S, Imran M, Sourirajan A, et al. In silico screening of hundred phytocompounds of ten medicinal plants as potential inhibitors of nucleocapsid phosphoprotein of Covid-19: an approach to prevent virus assembly. J Biomol Struct Dyn. (2021) 39:7017–34. doi: 10.1080/07391102.2020.1804457
94. Kumar D, Chandel V, Raj S, Rathi B. In silico identification of potent FDA approved drugs against coronavirus Covid-19 main protease: a drug repurposing approach. Chem Biol Lett. (2020) 7:166–75.
95. Hashemian SMR, Pourhanifeh MH, Hamblin MR, Shahrzad MK, Mirzaei H. Rdrp inhibitors and Covid-19: is molnupiravir a good option? Biomed Pharmacother. (2022) 146:112517. doi: 10.1016/j.biopha.2021.112517
96. Pant S, Singh M, Ravichandiran V, Murty USN, Srivastava HK. Peptide-like and small-molecule inhibitors against Covid-19. J Biomol Struct Dyn. (2021) 39:2904–13. doi: 10.1080/07391102.2020.1757510
97. Bojadzic D, Alcazar O, Chen J, Chuang ST, Condor Capcha JM, Shehadeh LA, et al. Small-molecule inhibitors of the coronavirus spike: ace2 protein-protein interaction as blockers of viral attachment and entry for SARS-CoV-2. ACS Infect Dis. (2021) 7:1519–34. doi: 10.1021/acsinfecdis.1c00070
98. Chen CC, Yu X, Kuo CJ, Min J, Chen S, Ma L, et al. Overview of antiviral drug candidates targeting coronaviral 3c-like main proteases. FEBS J. (2021) 288:5089–121. doi: 10.1111/febs.15696
99. Mirzaei M, Harismah K, Da’i M, Salarrezaei E, Roshandel Z. Screening efficacy of available HIV protease inhibitors on Covid-19 protease. J Mil Med. (2020) 22:100–7.
100. Tian L, Qiang T, Liang C, Ren X, Jia M, Zhang J, et al. RNA-dependent RNA polymerase (Rdrp) inhibitors: the current landscape and repurposing for the Covid-19 pandemic. Eur J Med Chem. (2021) 213:113201. doi: 10.1016/j.ejmech.2021.113201
101. Liu T, Luo S, Libby P, Shi GP. Cathepsin L-selective inhibitors: a potentially promising treatment for Covid-19 patients. Pharmacol Ther. (2020) 213:107587. doi: 10.1016/j.pharmthera.2020.107587
102. Zheng YY, Ma YT, Zhang JY, Xie X. Reply to: ‘Interaction between Raas inhibitors and Ace2 in the context of Covid-19’. Nat Rev Cardiol. (2020) 17:313–4. doi: 10.1038/s41569-020-0369-9
103. Sun ML, Yang JM, Sun YP, Su GH. [Inhibitors of Ras might be a good choice for the therapy of Covid-19 pneumonia]. Zhonghua Jie He He Hu Xi Za Zhi. (2020) 43:219–22.
104. Chen CX, Wang JJ, Li H, Yuan LT, Gale RP, Liang Y. Jak-inhibitors for coronavirus disease-2019 (Covid-19): a meta-analysis. Leukemia. (2021) 35:2616–20.
105. Wu S, Jin Z, Peng C, Li D, Cheng Y, Zhu R, et al. Use of proton pump inhibitors are associated with higher mortality in hospitalized patients with Covid-19. J Glob Health. (2022) 12:05005. doi: 10.7189/jogh.12.05005
106. Choudhry N, Zhao X, Xu D, Zanin M, Chen W, Yang Z, et al. Chinese therapeutic strategy for fighting Covid-19 and potential small-molecule inhibitors against severe acute respiratory syndrome coronavirus 2 (SARS-CoV-2). J Med Chem. (2020) 63:13205–27. doi: 10.1021/acs.jmedchem.0c00626
107. Li, LJCT, Drugs H. Effect of maxing shigan decoction against type A influenza virus infection in mice induced by viral lung injury based on Tlr4-Myd88-Traf6 signal pathways. Chin Tradit Herb Drugs. (2017) 48:1591–6.
108. Qi J, Qi X, Wang X. Clinical efficacy of different doses of Jinhuaqinggan granule on influenza and serum levels of cytokines. Mod Med. (2016) 44:1664–9.
109. Xia Y, Zhao Y, Wu YR, Kuang GY, Shen F, Chen W, et al. Network-based pharmacology and bioinformatics study on the potential targets and mechanisms of “Jinhua Qingsense granules” in the treatment of novel coronavirus pneumonia. Liaoning J Tradit Chin Med. (2021) 48:18–23+222–3.
110. Gao, J, Xiao G, Fan G, Zhang H, Zhu Y, Ming L. Clinical and pharmacological advances in the treatment of Covid-19 with the “three-drug triad”. Chin Sci Bull. (2022) 67:3296–10.
111. Lin J, Zheng W, Zeng G, Lin Q. Study on network pharmacology of Jinhua Qinggan granules in the treatment of novel coronavirus pneumonia. J Chin Med Mater. (2020) 43:2070–6.
112. Mao Y, Su Y, Xue P, Li L, Zhu S. Exploring the mechanism of action of Jinhua Qingxiang granules in the treatment of novel coronavirus pneumonia. J Chin Med Mater. (2020) 43:2842–8.
113. Zhang S, He GL, Lu F, Li L, Zhang B, Dai B, et al. Exploring the anti-influenza virus mechanism of Ma Heng Shi Gan Tang based on Tlr7/8-mediated Ifn-A/B protein expression levels of ephedra first decoction. China J Tradit Chin Med Pharm. (2019) 34:1188–93.
114. Wang S, Wang W, Nan C, Wang X. Effects of silver forsythia on Jak/Stat signaling pathway and expression of Ns1 protein and Mx a protein in lung tissue of mice with Fm1-induced pneumonia. China Pharmacist. (2020) 23:213–7.
115. Du H, Wang P, Ma Q, Li N, Ding J, Sun TF, et al. A preliminary investigation on the effective components and mechanism of Huo Xiang Zheng Qi tang to inhibit the replication process of novel coronavirus. Modern Tradit Chin Med Materia Med World Sci Technol. (2020) 22:645–51.
116. Ren W, Liang P, Ma Y, Sun Q, Pu Q, Dong L, et al. Research progress of traditional Chinese medicine against Covid-19. Biomed Pharmacother. (2021) 137:111310. doi: 10.1016/j.biopha.2021.111310
117. Deng Y-J. Study on active compounds from Huoxiang Zhengqi oral liquid for prevention of coronavirus disease 2019 (Covid-19) based on network pharmacology and molecular docking. Chin Tradit Herb Drugs. (2020) 51:1113–22.
118. Deng Y, Liu B, He Z, Liu T, Zheng R, Yang A, et al. Exploration of active compounds of Huoxiang Zhengqi oral liquid for the prevention of novel coronavirus pneumonia (Covid-19) based on network pharmacology and molecular docking method. Chin Tradit Herb Drugs. (2020) 51:1113–22.
119. Liu L, Xu Y, Jiang S, Xu Y. Exploring the possible mechanism of action of sparse wind relief capsules for the treatment of novel coronavirus pneumonia based on network pharmacology and molecular docking. Chin J Pharmacovigilance. (2021) 18:115–20.
120. Li K, Chen F, Li J, Chen X, Li M, He G. A network pharmacological study on the treatment of novel coronavirus pneumonia with Shu Feng Jie toxin capsule. J Chin Med Mater. (2020) 43:3080–7.
121. Liu J, Ma L, Lu J, Tian X, Xu J, Shen X, et al. Study on the mechanism of antipyretic effect of Shu Feng Jie toxin capsule. Chin Tradit Herb Drugs. (2016) 47:2040–3.
122. Ma L, Huang Y, Yanbao H, Zhang D, Zhang Y, Liu J, et al. Study on the mechanism of immunomodulatory effect of Shu Feng Jie toxin capsule. Drug Eval Res. (2019) 42:1763–8.
123. Loktionov A. Common gene polymorphisms and nutrition: emerging links with pathogenesis of multifactorial chronic diseases (review). J Nutr Biochem. (2003) 14:426–51. doi: 10.1016/s0955-2863(03)00032-9
124. Wang Y, Fan X, Qu H, Gao X, Cheng Y. Strategies and techniques for multi-component drug design from medicinal herbs and traditional Chinese medicine. Curr Top Med Chem. (2012) 12:1356–62. doi: 10.2174/156802612801319034
125. Chen N, Zhou M, Dong X, Qu J, Gong F, Han Y, et al. Epidemiological and clinical characteristics of 99 cases of 2019 novel coronavirus pneumonia in Wuhan, China: a descriptive study. Lancet. (2020) 395:507–13. doi: 10.1016/S0140-6736(20)30211-7
Keywords: COVID-19, Chinese patent medicines, active components, common mechanism of action, network pharmacology, ACE2, 3CL pro
Citation: Wang L, Wang Z, Yang Z, Wang X, Yan L, Wu J, Liu Y, Fu B and Yang H (2022) Potential common mechanism of four Chinese patent medicines recommended by diagnosis and treatment protocol for COVID-19 in medical observation period. Front. Med. 9:874611. doi: 10.3389/fmed.2022.874611
Received: 12 February 2022; Accepted: 10 October 2022;
Published: 26 October 2022.
Edited by:
Ying Zhang, Zhejiang University, ChinaReviewed by:
S. Udhaya Kumar, Vellore Institute of Technology, IndiaSriroopreddy Ramireddy, VIT University, India
Copyright © 2022 Wang, Wang, Yang, Wang, Yan, Wu, Liu, Fu and Yang. This is an open-access article distributed under the terms of the Creative Commons Attribution License (CC BY). The use, distribution or reproduction in other forums is permitted, provided the original author(s) and the copyright owner(s) are credited and that the original publication in this journal is cited, in accordance with accepted academic practice. No use, distribution or reproduction is permitted which does not comply with these terms.
*Correspondence: Hongtao Yang, dGpjbWh0QDEyNi5jb20=; Lin Wang, VGlhbkppbldhbmdMaW5AMTI2LmNvbQ==
†These authors have contributed equally to this work