- 1College of Pharmacy and Graduate School of Pharmaceutical Sciences, Ewha Womans University, Seoul, South Korea
- 2Korea Center for Gendered Innovations for Science and Technology Research, Seoul, South Korea
This study aimed to assess the research on medical Artificial intelligence (AI) related to sex/gender and explore global research trends over the past 20 years. We searched the Web of Science (WoS) for gender-related medical AI publications from 2001 to 2020. We extracted the bibliometric data and calculated the annual growth of publications, Specialization Index, and Category Normalized Citation Impact. We also analyzed the publication distributions by institution, author, WoS subject category, and journal. A total of 3,110 papers were included in the bibliometric analysis. The number of publications continuously increased over time, with a steep increase between 2016 and 2020. The United States of America and Harvard University were the country and institution that had the largest number of publications. Surgery and urology nephrology were the most common subject categories of WoS. The most occurred keywords were machine learning, classification, risk, outcomes, diagnosis, and surgery. Despite increased interest, gender-related research is still low in medical AI field and further research is needed.
Introduction
Gender medicine investigates the influence of sex/gender on the pathophysiology, prevention and treatment of disease, and the social and psychological aspects of illness (1, 2). Although medical research has been performed dominantly on men both in preclinical and clinical studies (3), there have been continuous efforts to overcome this gender bias (4). Since Healy B proposed gender differences in clinical outcomes (5), the subject has been discussed extensively, including in medical fields such as cardiovascular and gastrointestinal disease and oncology (6–9).
Artificial intelligence (AI) is a branch of computer science in which machines are developed to mimic human intelligence, including cognition, perception, and problem-solving (10, 11). This field has developed quickly and been applied to many areas, including medicine (11, 12). With its sophisticated algorithms, AI assists doctors and health professionals with data management, image-based diagnostics, robotic surgery, prediction models, and decision-making support (13, 14).
The widespread application of AI has promoted research in related fields, supporting the implementation of AI technologies in health care (14). Guo et al. found that publications on health care related to AI increased an average of 17% per year since 1995, with a steep increase of 45% between 2014 and 2019 (15). Along with the increased number of publications in medical AI, gender differences are important in other research areas. As bibliometric analysis quantitatively analyzes scientific publications, it can provide researchers and stakeholders with a macroscopic overview of research trends and help develop further research direction and policy. This study aims to assess the research activity on medical AI related to sex/gender and explore the global research trends over the past 20 years.
Methods
We extracted bibliographic data on gender-related medical AI articles from Web of Science (WoS) Core Collection. WoS Core Collection, which contains over 20,000 peer-reviewed, high-quality journals published worldwide covering various fields (16), is one of the most well-established and commonly used databases for bibliometric analysis (17, 18). Articles from 2001 to 2020 were collected using the following search terms: {TS=(“artificial intelligence” OR “machine intelligence” OR “artificial neural network*” OR “machine learning” OR “deep learn*” OR “natural language process*” OR “robotic*” OR “thinking computer system” OR “fuzzy expert system*” OR “evolutionary computation” OR “hybrid intelligent system*”)} AND {TS=(disease* OR illness OR health-related OR medic* OR “medical diagnosis” OR treatment OR health* OR wellness OR well-being OR prescription OR drug)} AND {TS=(gender OR sex OR male OR female)}.
The inclusion criteria were: (i) articles, review articles, and editorial materials; (ii) publications from 2001 to 2020; and (iii) full texts published in English. Articles were excluded if they were a proceeding paper, meeting abstract, book review, book chapter, or correction.
For bibliometric analysis, we extracted the title, abstract, year of publication, journal name with impact factor, authors, institution, country, WoS subject category, keywords, and number of citations. We determined the annual publication growth, the relative research interest (RRI), and percentage of gender-related articles in the medical AI area. Four 5-year periods (2001–2005, 2006–2010, 2011–2015, and 2016–2020) were used to compare the progress of each country. Two bibliometric indicators, Specialization Index (SI) and Category Normalized Citation Impact (CNCI), were computed by InCites with the following equation (19, 20):
We also analyzed the publication distributions by institution, author, WoS subject category, and journal. We used VOSviewer (Leiden University, Leiden, The Netherlands; version 1.6.11) to draw network visualization maps and performed a citation analysis to identify the most cited articles.
Results
Publication Growth
We identified 3,261 papers during the search (Figure 1). After excluding 44 non-English papers and 107 non-articles, 3,110 papers met the inclusion criteria. The graphs of absolute number of publications (Figure 2A) and RRI (Figure 2B) showed that the overall trend of publication increased from 2001 to 2020. The growth rates from 2001 to 2005, from 2006 to 2010, from 2011 to 2015, and from 2016 to 2020 were 71.4, 115.8, 146.2, and 453.3%, respectively. The number of publications increased steeply between 2016 and 2020, accounting for 77.5% (2,410/3,110) of all included papers. Figure 2C shows the percentages of gender-related articles in medical AI researches, which doubled to 6.5% from 2001 to 2020. The linear regression analysis showed that the percentages increased significantly over the last 20 years (t = 12.978, P < 0.001).
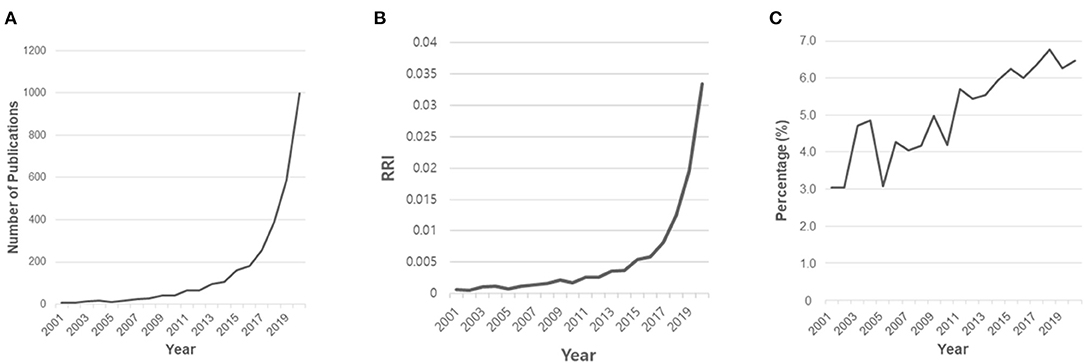
Figure 2. Annual growth of gender-related publications in medical AI. (A) The number of publications by year. (B) Relative research interest by year. (C) The percentage of gender-related publications in medical AI.
Distribution by Country
Table 1 lists the top 20 countries which published gender-related articles in medical AI between 2001 and 2020. The United States of America (USA) had the most publications on gender-related medical AI (n = 1,377; 44.3%), followed by People's Republic of China (Peoples R China, n = 305; 9.8%), United Kingdom (n = 241; 7.7%), Italy (n = 211; 6.8%), and the Republic of Korea (South Korea, n = 201; 6.5%). Across the four five-year periods from 2001 to 2020, there was a 43.6% increase in the number of publications worldwide from the first to the last period. Canada had the greatest percentage increase in the number of publications (+134.0%), followed by Peoples R China (+127.5%), the South Korea (+77.5%), and United Kingdom (+65.0%). There was no country where the number of publications decreased.
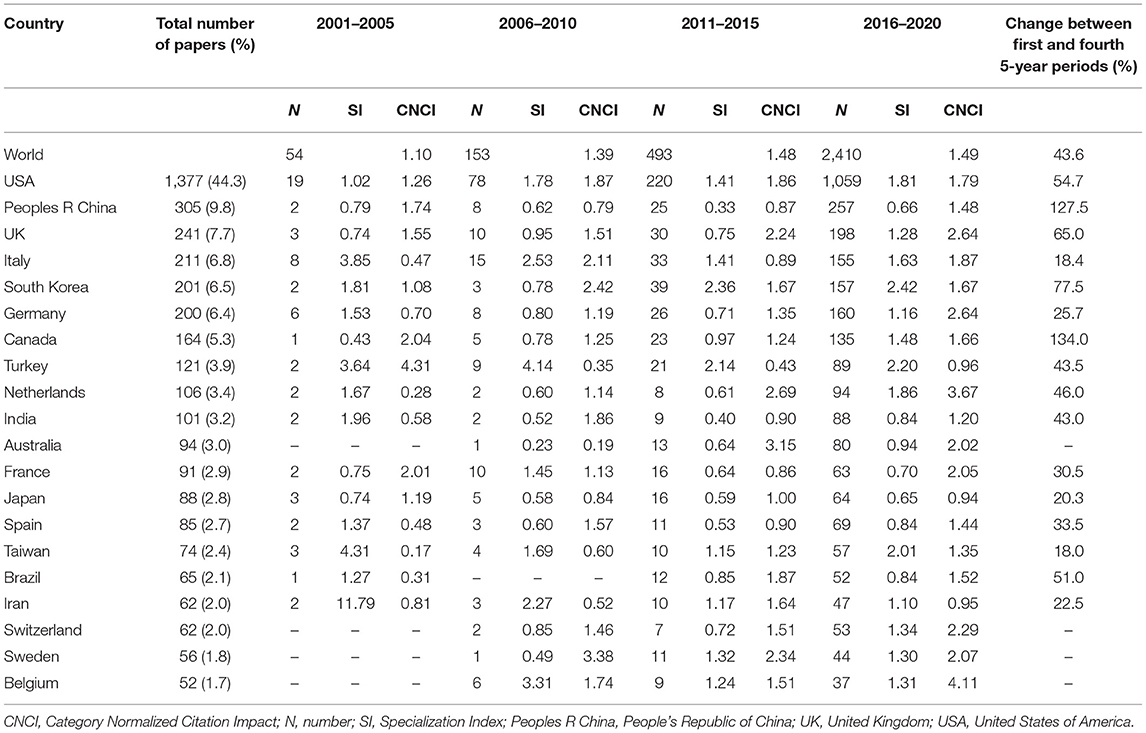
Table 1. The 20 countries contributing the most gender-related articles in medical artificial intelligence.
The SIs and CNCIs varied across countries and over time. The global CNCI increased steadily from 1.1 to 1.49 over the last 20 years. Compared to the first period (2001–2005), the USA, United Kingdom, South Korea, and Netherland showed an increase in both SI and CNCI in the fourth period (2016–2020), whereas Peoples R China, Turkey, and Japan showed a decrease in their SIs and CNCIs. From 2016 through 2020, South Korea had the highest SI (2.42), whereas Belgium had the highest CNCI (4.11).
Distribution by Institution
Table 2 shows the top 10 institutions for gender-related articles in medical AI fields. The top 10 institutions contributed to 26.5% (824/3,110) of the total number of publications. Harvard University had the largest number of publications (n = 142; 4.6%), followed by the University of California System (n = 136; 4.4%), the University of Texas System (n = 84; 2.7%), Harvard Medical School (n = 84; 2.7%), and University of London (n = 81; 2.6%). Almost 90% of the top 10 institutions were located in the USA.
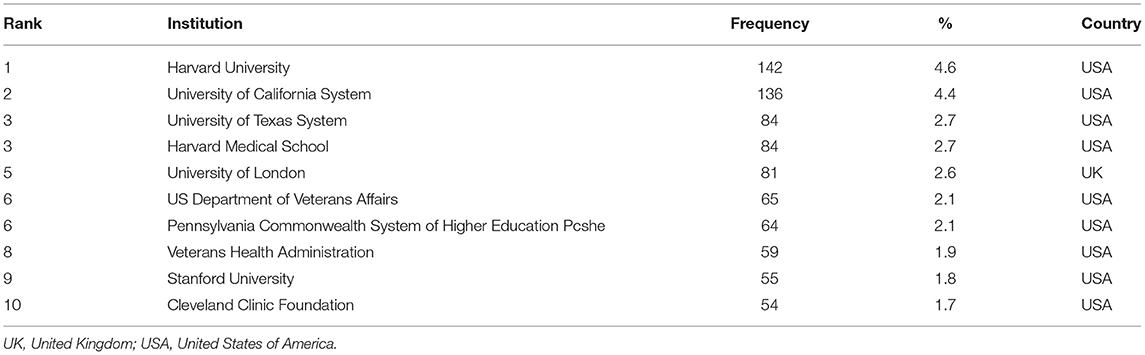
Table 2. The institutions contributing the most gender-related articles in medical artificial intelligence.
Figure 3 shows the collaboration network between institutions. The network map of institutions that had at least 20 publications showed seven clusters. Among these, the four biggest clusters were (i) the cluster (red) on Stanford University and University of Pittsburgh; (ii) the cluster (green) on the Cleveland Clinic and the University of Michigan; (iii) the cluster (blue) on Yonsei University and Seoul National University; and (iv) the cluster (yellowish-green) on Yale University and the University of California (UC) San Diego.
Distribution by Author
A total of 18,247 authors accounted for all publications for gender-related medical AI in 2001–2020. Dey D, Kaouk JH, and Grossie E contributed the most, with 10 publications, followed by Slomka PJ and Kaouk J, with nine publications (Table 3). In terms of first-author publications, Lin E ranked first with five publications, whereas Lee BJ ranked second with four. Most of the high-ranked authors by publications were from the USA, except for two from Europe. For the high-ranked first-authors, six were from Asia, four from the USA, and two from Europe. In addition, the results of co-citation, bibliometric coupling, and co-authorship analysis were shown in Supplementary Figure 1.
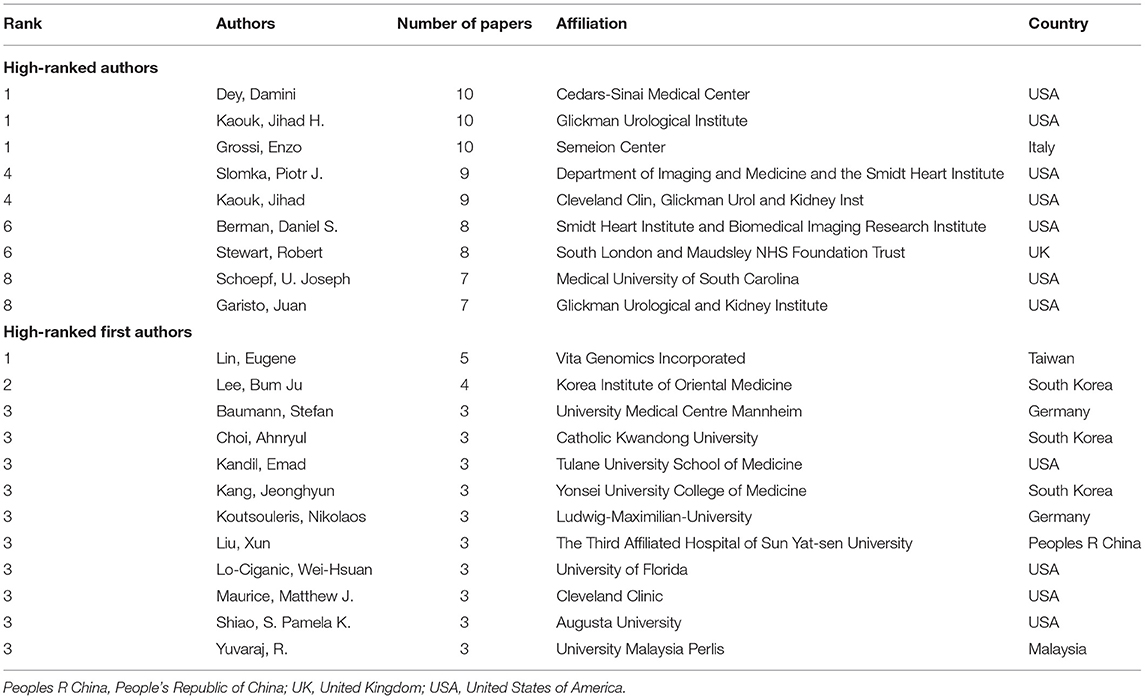
Table 3. The authors and first-authors contributing the most gender-related articles in medical artificial intelligence.
Distribution by Topic
Table 4 shows the 10 most common WoS subject categories. Surgery ranked first, with 496 publications (15.9%), followed by Urology and Nephrology (n = 241; 7.7%), Medicine, General and Internal (n = 212; 6.8%), Neuroscience (n = 204; 6.6%), and Radiology, Nuclear Medicine and Medical Imaging (n = 172; 5.5%).
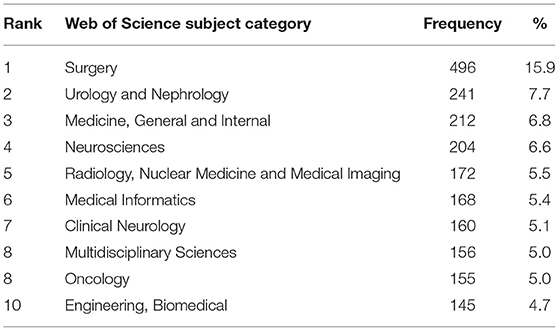
Table 4. The most productive Web of Science subject categories in gender-related articles in medical artificial intelligence.
Figure 4 shows the network visualization map of keywords with a minimum occurrence of 20. Five clusters with 177 terms were obtained from the analysis: (i) a red cluster with 56 items focused on machine learning, classification, diagnosis, children, deep learning, and meta-analysis; (ii) a green cluster with 55 items focused on items focused on surgery, outcomes, robotic surgery, cancer, and management; (iii) a blue cluster with 47 items focused on risk, prediction, disease, mortality, health, artificial intelligence, and validation; (iv) a yellowish-green cluster with 13 items focused on stroke, therapy, rehabilitation, and reliability; and (v) a purple cluster with six items focused on index, guidelines, coronary artery disease, and intervention. Network visualization maps for keywords across the time periods were shown in Supplementary Figure 2.
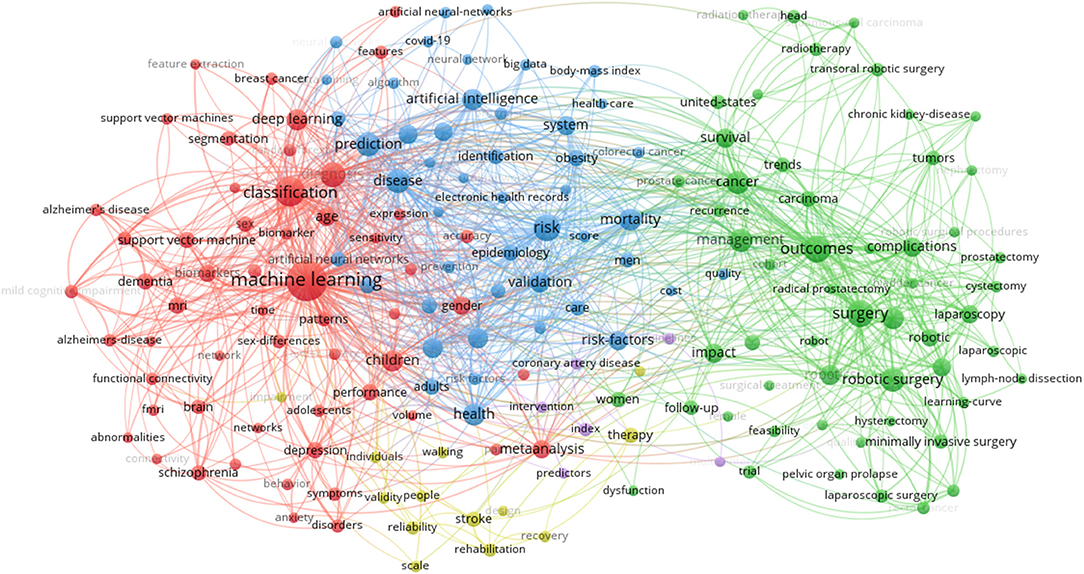
Figure 4. Network visualization map of keywords. Keywords included the author's keywords and keywords plus.
Distribution by Journal
The 3,110 papers were published in 1,281 journals. Table 5 lists the top 10 journals by the number of publications within the study period. The top 10 journals contributed 13.0% (403/3,110) of the total publications. PLoS One published the most articles on gender-related medical AI (n = 81; 2.6%), followed by Surgical Endoscopy and Other Interventional Techniques (n = 48; 1.5%), Asian Journal of Surgery (n = 44; 1.4%), and Scientific Reports (n = 43; 1.4%). Among the top 10 journals by publication number, Surgical Endoscopy and Other Interventional Techniques had the highest H-index (15), whereas the Journal of Urology had the largest number of citations per paper (55).
Characteristics of Top 9 Papers Most Frequently Cited
There were 44,711 citations in 3,110 publications. Table 6 shows the top 9 papers with the highest citation frequency. The top 9 papers accounted for 7.0 % (3,112/44,711) of the total citations and were cited 346 times, on average. The work of Wynants et al. (21) was the most cited paper (n = 567; 1.3%), followed by the study by Poplin et al. (22) (n = 382; 0.9%) and Aarts et al. (23) (n = 369; 0.8%). Among the top 9 papers, three were published in journals with an impact factor (IF) > 20, one in a journal with an IF between 10 and 20, three in journals with IFs between 5 and 10, and two in journals with an IF < 5.
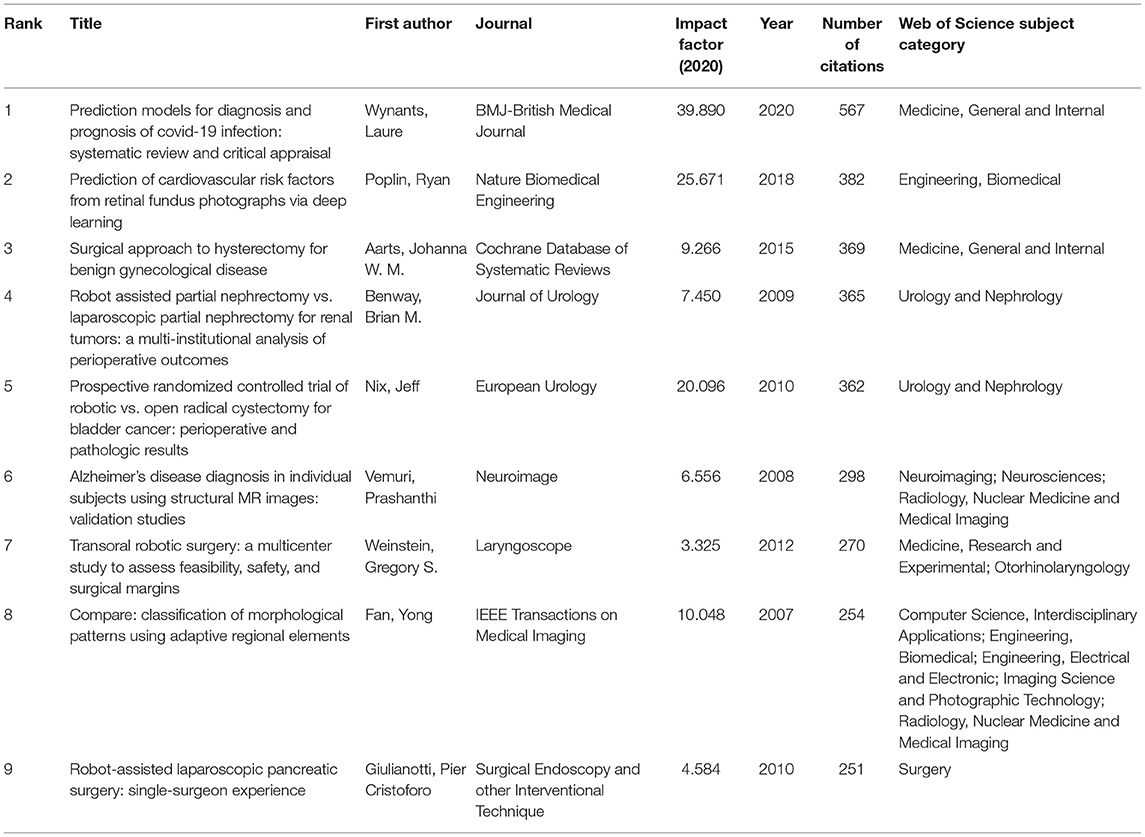
Table 6. The papers with the most frequent citations of gender-related medical artificial intelligence.
Discussion
Our bibliometric analysis of the gender-related articles in medical AI revealed major changes over the last 20 years. The number of publications and percentage of gender-related articles in medical AI fields continuously increased from 2001 to 2020, with a steep increase in the past 5 years. This change can be explained by both increased interest of AI and awareness of gender medicine. Due to the technological development including computing power and data storage, AI has been developed (24), leading to advances in researches and collaborative works in medical AI fields (15, 25). In addition, there have been continuous efforts to overcome this gender bias (4), although women used to be underrepresented in clinical research (26).
After the National Institute of Health (NIH) Revitalization Act of 1993 mandated the enrollment of women and ethnic minorities in clinical research in the USA (27), funding agencies such as the Canadian Institutes of Health Research (28), European Commission (29), and NIH (30) required consideration of sex and gender in study design, analysis, and reporting for grant applications. In addition, several editorial guidelines included gender-specific work [e.g., Animal Research: Reporting In Vivo Experiments (ARRIVE) (31), Sex and Gender Equity in Research (SAGER) (32), and International Committee of Medical Journal Editors (ICMJE) recommendations (33)].
Both the number of publications and RRI on gender-related medical AI have steadily increased for 20 years, showing the increase of research interests in related fields. The percentage of gender-related articles in medical AI doubled in the last 20 years to 6.5%, although this figure remains small. According to Sugimoto et al., in 2016, two-thirds of articles were gender-related reporting articles of clinical medicine and public health research, whereas one-third of such articles were for biomedical research (34). Geller et al. showed that 26% of NIH-funded randomized control trials in 2018 included sex as a covariate (35). Compared to other fields, medical AI had a low percentage of gender-related articles. This requires further study.
As the number of publications can only provide volumetric information, our analysis showed SI and CNCI across countries and over time. These two parameters can provide different perspectives on research trends (36). SI, the ratio of the percentage of publications related to the specific area in a given country to those worldwide, evaluates specialization. CNCI, which is the ratio of the observed to the expected number of citations in the same WoS category, shows the citation impact. For example, although Canada and Peoples R China had the highest percentage increase in the number of publications over the previous 20 years, Canada showed overspecialization and citation impact specifically in gender-related medicine AI research compared to the worldwide figures, whereas Peoples R China did not.
The USA had the most publications on gender-related medical AI between 2001 and 2020, with overall high CNCIs and SIs. As expected, the top 10 institutions and high-ranked authors were from the USA. According to the network visualization plot, most of the top 10 institutions were also well-connected through research networks. According to the bibliographic analysis of authors, it was possible to understand the relationships between authors. Author co-citation analysis visualized the intellectual structure of the scientific knowledge domain by calculating how often the author's work is cited with other authors (37), whereas bibliographic coupling showed the similarity relationships by calculating how often two papers are cited together (38). In addition, co-authorship analysis showed the cooperative and interactive relationships between authors, indicating the authors' willingness to write a paper together (39).
Surgery and Urology and Nephrology was the most common WoS subject category in our analysis. Similarly, Surgical Endoscopy and Other Interventional Techniques, Asian Journal of Surgery, Urology, and Journal of Urology were the journals that ranked high in the number of gender-related publications in medical AI. Surgery is one of the most developed areas in medical AI. AI can be applied pre-, intra-, and post-surgery, such as for preoperative risk prediction, imaging, 3D reconstruction, and robotic intervention (40, 41). As several studies reported the sex differences in prognosis after surgery (42–44), sex should be considered in AI surgery research. Urology is another area of interest in gender-related medical AI. There are anatomical, physiological, and pathophysiological urological differences between men and women (45). Hormones and metabolisms differ by sex, thereby affecting medical conditions (46). Furthermore, environmental and occupational exposures may differ by gender, which should be considered in gender medicine (47).
The network visualization map of keywords across the time periods showed that research topics have continuously expanded and changed over past two decades. In the first period (2001–2005), there was only two clusters; one was disease and the other was artificial neural networks and cancer. In the last period (2016–2020), there was 6 clusters including machine learning, risk, and surgery. These results can be used to guide future studies by listing the trending topics.
The citation analysis showed that gender-related medical AI had a high influence, with an average of 15 citations. The topics covered in the top 9 articles with the highest citations were surgery, imaging, and prediction models. The most cited article was the study of Wynants et al. (21), which systematically reviewed and critically evaluated all 232 predictive models for diagnosis and prognosis of COVID-19 including 169 studies. This study showed that gender is one of the frequent prognostic factors of COVID-19. As the COVID-19 pandemic has posed a threat to the global economic and health systems with high morbidity and mortality (48), COVID-19-related articles have recently dominated medical publishing in the last 2 years (49). The study of Poplin et al., the second most cited article, developed deep learning models using retinal fundus images to predict multiple cardiovascular risk factors including age and gender (22). The third most cited article was the article by Aarts et al. (23), which reviewed the effectiveness and safety of four types of hysterectomy surgeries in women with benign gynecological diseases. Interestingly, most of the top 9 articles were published in journals with an IF < 10. This demonstrates an increased interest in this field.
This bibliometric study has some limitations. First, like other bibliometric studies, the results can be affected by the search term and databases used. As we only used the WoS, we could not include publications in other electronic databases (e.g., PubMed or Embase). However, we selected the WoS covering a broad range of articles (50) and applied search strategies with high sensitivity. Second, there was a possibility of the inclusion of studies that had little to do with our topics. As we focused on showing macroscopic tendencies, studies were identified through search if they had AI-, medication-, and gender-related terms in titles, abstracts, or keywords, regardless of their topics. For example, the article by Roberts et al. (51), the originally identified as the second most cited article, suggested the structural topic models for surveys in political sciences; it was not presented in Table 6 for qualitative interpretation. Despite this, including gender-related words is meaningful because it covers gender in any way. Third, the number of citations can be biased by self-citations and time elapsed since publication. Lastly, non-English publications were not included.
To the best of our knowledge, this is the first bibliometric study to investigate the worldwide research output of gender-related medical AI by bibliometric analysis. This study concluded that gender-related research in medical AI increased over the past 20 years. Despite increased interest, gender-related research is still low in medical AI field and further research is needed.
Data Availability Statement
The raw data supporting the conclusions of this article will be made available by the authors, without undue reservation.
Author Contributions
JY and HG contributed to designing the study. HY contributed to acquisition and analysis of data. HY, JY, and HG contributed to interpretation of data. HY and HL contributed to drafting of the manuscript. JY and HG contributed to critical revision of the manuscript. All authors contributed to the conception and design and read and approved the final manuscript.
Funding
The research leading to these results received funding from Ministry of Science and ICT under WISET202103GI01.
Conflict of Interest
The authors declare that the research was conducted in the absence of any commercial or financial relationships that could be construed as a potential conflict of interest.
Publisher's Note
All claims expressed in this article are solely those of the authors and do not necessarily represent those of their affiliated organizations, or those of the publisher, the editors and the reviewers. Any product that may be evaluated in this article, or claim that may be made by its manufacturer, is not guaranteed or endorsed by the publisher.
Supplementary Material
The Supplementary Material for this article can be found online at: https://www.frontiersin.org/articles/10.3389/fmed.2022.868040/full#supplementary-material
References
1. Baggio G, Corsini A, Floreani A, Giannini S, Zagonel V. Gender medicine: a task for the third millennium. Clin Chem Lab Med. (2013) 51:713–27. doi: 10.1515/cclm-2012-0849
2. Mauvais-Jarvis F, Merz NB, Barnes PJ, Brinton RD, Carrero JJ, DeMeo DL, et al. Sex and gender: modifiers of health, disease, and medicine. Lancet. (2020) 396:565–82. doi: 10.1016/S0140-6736(20)31561-0
3. Gochfeld M. Sex differences in human and animal toxicology: toxicokinetics. Toxicol Pathol. (2017) 45:172–89. doi: 10.1177/0192623316677327
4. Lee SK. Sex as an important biological variable in biomedical research. BMB Rep. (2018) 51:167. doi: 10.5483/BMBRep.2018.51.4.034
6. Arain FA, Kuniyoshi FH, Abdalrhim AD, Miller VM. Sex/gender medicine. The biological basis for personalized care in cardiovascular medicine. Circ J. (2009) 73:1774–82. doi: 10.1253/circj.CJ-09-0588
7. Freire AC, Basit AW, Choudhary R, Piong CW, Merchant HA. Does sex matter? The influence of gender on gastrointestinal physiology and drug delivery. Int J Pharm. (2011) 415:15–28. doi: 10.1016/j.ijpharm.2011.04.069
8. Wagner AD, Oertelt-Prigione S, Adjei A, Buclin T, Cristina V, Csajka C, et al. Gender medicine andoncology:report and consensus of an ESMO workshop. Ann Oncol. (2019) 30:1914–24. doi: 10.1093/annonc/mdz414
9. Safdar B, Greenberg MR. Organization, execution and evaluation of the 2014 academic emergency medicine consensus conference on gender-specific research in emergency care–an executive summary. Acad Emerg Med. (2014) 21:1307–17. doi: 10.1111/acem.12530
10. Rich E. Artificial intelligence and the humanities. Comput Humanit. (1985) 19:117–22. doi: 10.1007/BF02259633
11. Pannu A. Artificial intelligence and its application in different areas. Artificial Intellig. (2015) 4:79–84.
12. Mintz Y, Brodie R. Introduction to artificial intelligence in medicine. Minim Invasive Ther Allied Technol. (2019) 28:73–81. doi: 10.1080/13645706.2019.1575882
13. Amisha PM, Pathania M, Rathaur VK. Overview of artificial intelligence in medicine. J Family Med Prim Care. (2019) 8:2328–31. doi: 10.4103/jfmpc.jfmpc_440_19
14. Hamet P, Tremblay J. Artificial intelligence in medicine. Metabolism. (2017) 69:S36–40. doi: 10.1016/j.metabol.2017.01.011
15. Guo Y, Hao Z, Zhao S, Gong J, Yang F. Artificial intelligence in health care: bibliometric analysis. J Med Internet Res. (2020) 22:e18228. doi: 10.2196/18228
16. Clarivate Analytics. Web of Science Core Collection. (2022). Available online at: https://clarivate.com/webofsciencegroup/solutions/web-of-science-core-collection. (accessed April 5, 2022).
17. Li K, Rollins J, Yan E. Web of Science use in published research and review papers 1997-2017: a selective, dynamic, cross-domain, content-based analysis. Scientometrics. (2018) 115:1–20. doi: 10.1007/s11192-017-2622-5
18. Meho LI, Yang K. Impact of data sources on citation counts and rankings of LIS faculty: Web of science versus scopus and google scholar. J Am Soc Inf Sci Technol. (2007) 58:2105–25. doi: 10.1002/asi.20677
19. UNESCO Institute for Statistics. What Do Bibliometric Indicators Tell Us About World Scientific Output? UIS Bulletin on Science and Technology Statistics (2005). p. 1–6.
20. Normalized Indicators (2020). Available online at: https://incites.help.clarivate.com/Content/Indicators-Handbook/ih-normalized-indicators.htm# (accessed April 5, 2022).
21. Wynants L, Van Calster B, Collins GS, Riley RD, Heinze G, Schuit E, et al. Prediction models for diagnosis and prognosis of covid-19: systematic review and critical appraisal. BMJ. (2020) 369:m1328. doi: 10.1136/bmj.m1328
22. Poplin R, Varadarajan AV, Blumer K, Liu Y, McConnell MV, Corrado GS, et al. Prediction of cardiovascular risk factors from retinal fundus photographs via deep learning. Nat Biomed Eng. (2018) 2:158–64. doi: 10.1038/s41551-018-0195-0
23. Aarts JW, Nieboer TE, Johnson N, Tavender E, Garry R, Mol BWJ, et al. Surgical approach to hysterectomy for benign gynaecological disease. Cochrane Database Syst Rev. (2015) 2015:CD003677. doi: 10.1002/14651858.CD003677.pub5
24. Tipol EJ. High-performance medicine: the convergence of human and artificial intelligence. Nat Med. (2019) 25:44–56. doi: 10.1038/s41591-018-0300-7
25. Ho YS, Wang MH. A bibliometric analysis of artificial intelligence publications from 1991 to 2018. COLLNET J Scientometr Inform Manag. (2020) 14:369–92. doi: 10.1080/09737766.2021.1918032
26. Vidaver RM, Lafleur B, Tong C, Bradshaw R, Marts SA. Women subjects in NIH-funded clinical research literature: lack of progress in both representation and analysis by sex. J Womens Health Gender Based Med. (2000) 9:495–504. doi: 10.1089/15246090050073576
28. Johnson JL, Beaudet A. Sex and gender reporting in health research: why Canada should be a leader. Can J Public Health. (2013) 104:e80–81. doi: 10.1007/BF03405660
29. European Commission. Fact Sheet: Gender Equality in Horizon 2020. (2013). Available online at: https://ec.europa.eu/programmes/horizon2020/sites/default/files/FactSheet_Gender_2.pdf
30. Clayton JA, Collins FS. Policy: NIH to balance sex in cell and animal studies. Nature News. (2014) 509:282–3. doi: 10.1038/509282a
31. Kilkenny C, Browne WJ, Cuthill IC, Emerson M, Altman DG. Improving bioscience research reporting: the ARRIVE guidelines for reporting animal research. PLoS Biol. (2010) 8:e1000412. doi: 10.1371/journal.pbio.1000412
32. De Castro P, Heidari S, Babor TF. Sex and gender equity in research (SAGER): reporting guidelines as a framework of innovation for an equitable approach to gender medicine. Ann Ist Super Sanita. (2016) 52:154–57. doi: 10.4415/ANN_16_02_05
33. Intermational Committee of Medical Journal Editors (ICMJE). Recommendations for the Conduct, Reporting, Editing, and Publication of Scholarly Work in Medical Journals. (2019). Available online at: www.icmje.org/icmje-recommendations.pdf
34. Sugimoto CR, Ahn YY, Smith E, Macaluso B, Larivière V. Factors affecting sex-related reporting in medical research: a cross-disciplinary bibliometric analysis. Lancet. (2019) 393:550–59. doi: 10.1016/S0140-6736(18)32995-7
35. Geller SE, Koch AR, Roesch P, Filut A, Hallgren E, Carnes M. The more things change, the more they stay the same: a study to evaluate compliance with inclusion and assessment of women and minorities in randomized controlled trials. Acad Med. (2018) 93:630. doi: 10.1097/ACM.0000000000002027
36. Devos P, Ménard J. Trends in worldwide research in hypertension over the period 1999–2018: a bibliometric study. Hypertension. (2020) 76:1649–55. doi: 10.1161/HYPERTENSIONAHA.120.15711
37. Bayer AE, Smart JC, McLaughlin GW. Mapping intellectual structure of a scientific subfield through author cocitations. J Am Soc Inform Sci. (1990) 41:444.
38. Zhao D, Strotmann A. Evolution of research activities and intellectual influences in information science 1996–2005: introducing author bibliographic-coupling analysis. J Am Soc Inform Sci Technol. (2008) 59:2070–86. doi: 10.1002/asi.20910
39. Huang MH, Chang YW. A study of interdisciplinarity in information science: using direct citation and co-authorship analysis. J Inform Sci. (2011) 37:369–78. doi: 10.1177/0165551511407141
40. Hashimoto DA, Ward TM, Meireles OR. The role of artificial intelligence in surgery. Adv Surg. (2020) 54:89–101. doi: 10.1016/j.yasu.2020.05.010
41. Zhou XY, Guo Y, Shen M, Yang GZ. Application of artificial intelligence in surgery. Front Med. (2020) 14:1–7. doi: 10.1007/s11684-019-0733-5
42. Hogue CW Jr, Barzilai B, Pieper KS, Coombs LP, DeLong ER, Kouchoukos NT, et al. Sex differences in neurological outcomes and mortality after cardiac surgery: a society of thoracic surgery national database report. Circulation. (2001) 103:2133–7. doi: 10.1161/01.CIR.103.17.2133
43. de Perrot M, Licker M, Bouchardy C, Usel M, Robert J, Spiliopoulos A. Sex differences in presentation, management, and prognosis of patients with non–small cell lung carcinoma. J Thorac Cardiovasc Surg. (2000) 119:21–6. doi: 10.1016/S0022-5223(00)70213-3
44. Tighe PJ, Riley JL III, Fillingim RB. Sex differences in the incidence of severe pain events following surgery: a review of 333,000 pain scores. Pain Med. (2014) 15:1390–404. doi: 10.1111/pme.12498
45. Abelson B, Sun D, Que L, Nebel RA, Baker D, Popiel P, et al. Sex differences in lower urinary tract biologyand physiology. Biol Sex Differ. (2018) 9:1–13. doi: 10.1186/s13293-018-0204-8
46. Moon JY, Choi MH, Kim J. Metabolic profiling of cholesterol and sex steroid hormones to monitor urological diseases. Endocr Relat Cancer. (2016) 23:R455–67. doi: 10.1530/ERC-16-0285
47. Fajkovic H. Editorial comment: gender medicine in urology. Curr Opin Urol. (2019) 29:247–8. doi: 10.1097/MOU.0000000000000597
48. World Health Organization (WHO). Coronavirus Disease (COVID-19) Weekly Epidemiological Update and Weekly Operational Update. (2022). Available online at: https://www.who.int/emergencies/diseases/novel-coronavirus-2019/situation-reports. (accessed April 5, 2022).
49. Kousha K, Thelwall M. Covid-19 refereeing duration and impact in major medical journals. Quan Sci Stud. (2022) 3:1–17. doi: 10.1162/qss_a_00176
50. Yang K, Meho LI. Citation analysis: a comparison of Google Scholar, Scopus, and Web of Science. Proc Am Soc Inform Sci Technol. (2006) 43:1–15. doi: 10.1002/meet.14504301185
Keywords: artificial intelligence, bibliometric analysis, gender, medicine, medical research
Citation: Yoon HY, Lee H, Yee J and Gwak HS (2022) Global Research Trends of Gender-Related Artificial Intelligence in Medicine Between 2001–2020: A Bibliometric Study. Front. Med. 9:868040. doi: 10.3389/fmed.2022.868040
Received: 02 February 2022; Accepted: 27 April 2022;
Published: 17 May 2022.
Edited by:
Mette Due Theilade Thomsen, PIP Adviser, DenmarkReviewed by:
Elizabeth Pollitzer, Portia, United KingdomMohamed Abouzid, Poznan University of Medical Sciences, Poland
Copyright © 2022 Yoon, Lee, Yee and Gwak. This is an open-access article distributed under the terms of the Creative Commons Attribution License (CC BY). The use, distribution or reproduction in other forums is permitted, provided the original author(s) and the copyright owner(s) are credited and that the original publication in this journal is cited, in accordance with accepted academic practice. No use, distribution or reproduction is permitted which does not comply with these terms.
*Correspondence: Hye Sun Gwak, hsgwak@ewha.ac.kr; Jeong Yee, jjjhello1@naver.com