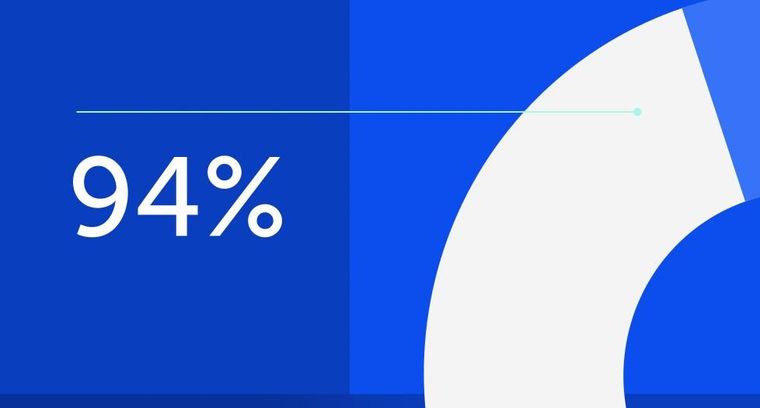
94% of researchers rate our articles as excellent or good
Learn more about the work of our research integrity team to safeguard the quality of each article we publish.
Find out more
METHODS article
Front. Med., 23 May 2022
Sec. Precision Medicine
Volume 9 - 2022 | https://doi.org/10.3389/fmed.2022.852529
This article is part of the Research TopicThe Path towards Precision Health: Prospects and ChallengesView all 10 articles
Background: Osteosarcoma (OS) is the most prevalent primary malignant bone cancer with poor prognosis. The aim of this study was to explore the prognostic factors that influence survival, and build up and validate a simple risk model to predict mortality in OS patients.
Materials and Methods: This was a single-center retrospective cohort study. A total of 153 patients with newly diagnosed OS were enrolled as the training group. We analyzed the clinical data and outcomes of the OS patients. Prognostic risk factors were identified and evaluated by a logistic regression model with Markov Chain Monte Carlo simulation. The risk score was constructed based on the training group and was further validated using each patient.
Results: Among the 153 patients, the mean (standard deviation) age was 21.6 (14.2) years, and 62 (40.5%) patients were females. The rate of in-hospital mortality of patients was 41.2% (95% CI, 31.6–50.7%). The candidate prognostic factors were selected and evaluated in relation to patient age, sex, tumor site (lower/upper extremity), tumor volume, intramedullary length of lesion, serum levels of alkaline phosphatase (ALP) and primary metastasis. However, only tumor size and primary metastasis were identified as independent prognostic indicators for patients with osteosarcoma. The risk model had a C-statistic of 0.7308 with a predictive range of 21.05–68.42%. Based on the distribution of the risk score, 24.8, 49.7 and 25.5% of patients were stratified into the high-, average- and low-risk groups for in-hospital mortality, with corresponding probabilities of 0.684, 0.329, and 0.210, respectively.
Conclusion: A simple risk model was developed and validated to predict the prognosis for patients with osteosarcoma of the extremity at primary diagnosis. The simple risk score system could be used to stratify patients into different risk groups of in-hospital mortality and may help clinicians judge the outcomes of prognosis and establish appropriate surveillance strategies.
Osteosarcoma (OS) is one of the most common primary malignant bone tumors in children and adolescents, accounting for approximately two-thirds of the bone cancers diagnosed in the second decade of life (1–3). The incidence of OS is approximately one to three cases annually per one million people worldwide (1–3). OS is characterized by the presence of an osteoid matrix or immature bone, mainly frequent sites in the metaphysis and diaphysis of long bones (femur, tibia) (2), and can be broadly classified into three histologic subtypes (intramedullary, surface, and extraskeletal) (4). The pathogenesis and etiology of OS are still unclear. In the past few decades, the 5-year survival of patients with localized OS has considerably improved to 78% with the development of neoadjuvant chemotherapy and surgical techniques. However, the 5-year survival rate drops to 25% in cases with metastasis at diagnosis or relapse (5). Moreover, another study showed that the 5-year survival rate showed no significant improvement in patients with localized disease and no improvement in metastatic patients over the past four decades (6). Thus, improving the survival of OS patients has proved challenging, although the therapy for osteosarcoma is on the precipice of advancement. The historical invariability of survival outcomes and the limited number of predictive risk factors known to be active in the development of this disease facilitate clinical trials designed to identify efficacious prognostic factors in patients with osteosarcoma.
Currently, some clinical studies have been reported regarding identifying the prognostic factors that influence survival in osteosarcoma, including patient age, sex, tumor site and size, histologic subtype, presence and location of metastases, histologic response to chemotherapy and type of surgery and surgical margins (7–14). However, there is no standardized system for evaluating the prognostic factors correlated with survival among these studies. Specifically, there were variations in the statistical methods and the study population. Consequently, the results are somewhat inconsistent and even contradictory in the published importance of some variables (such as patient age, tumor site, and tumor size) (8–10, 13). However, predictive models are important tools to provide estimates of patient outcome (15). Moreover, it is imperative to explore effective prognostic models to predict the mortality of patients with OS.
Herein, we mined the relevant clinical data of patients with osteosarcoma (OS) of the extremities spanning 3 years from 2013 to 2015. We subsequently identified prognostic risk factors related to poor outcomes and then developed and validated a risk model to stratify patients into different risk groups of in-hospital mortality and help clinicians provide patients with appropriate surveillance strategies.
Medical records of patients with OS of the extremities who were admitted to the Affiliated Cancer Hospital of Zhengzhou University between 1 January 2013 and 31 December 2015 with a minimum follow-up of 5 years were reviewed. The eligible patients for enrolment fulfilled the following criteria: typical radiographic and histologic features of primary, high-grade central osteosarcoma of the extremity. Exclusion criteria included non-extremity locations, low- or intermediate-grade osteosarcoma, treatment regimens that did not follow National Comprehensive Cancer Network (NCCN) guidelines, concomitant with previous history of cancer, and incomplete medical records. In total, we identified 153 patients who satisfied the prespecified study inclusion and exclusion criteria. The original 153 samples were used as the training group, and the bootstrap method was used as an internal test of the performance of the model (Figure 1). The ethics committee of the Affiliated Cancer Hospital of Zhengzhou University approved this study (No. 2017407).
Figure 1. The design of the study. The patient cohort including 153 patients with newly diagnosed OS after a strict pathological diagnosis and exclusion process was used to identify prognostic factors for in-hospital mortality and was used for further evaluation and validation analysis.
The 7 candidate risk factors were easily collected, reliable, clinically important, and potentially associated with the outcome, including patient age, sex, tumor site (lower/upper limbs), tumor volume, intramedullary length of lesion, ALP and primary metastasis. The outcome was in-hospital mortality.
Using the original sample with all candidate risk factors, we employ Markov Chain Monte Carlo (MCMC) simulation to select the primitive risk factors, which have a positive coefficient in more than 90% or <10% of the simulations and are regarded with a stable association with the outcome. The final risk model to predict the outcome was constructed by fitting a logistic regression model to the original sample using the selected risk factors by the MCMC simulation.
Due to the small sample size, the bootstrap method (16) was used to test model performance, and a total of 1,000 bootstrap samples were drawn with replacement of the same sample size as the original sample. Models were developed in the bootstrap samples and tested in the original sample.
We calculated the four indicators to evaluate the risk model performance. Discrimination was assessed with an internally validated c-statistic, and the distribution of the c-statistic for the bootstrap samples and the original sample (Figure 2A) are also presented. The internally validated calibration slope was used to measure calibration, and the observed in-hospital mortality in strata defined by quantiles of the predictive probabilities is presented (Figure 2B). We divided patients in the original sample into 3 mutually exclusive risk classes based on the quantiles of the predicted probability of in-hospital mortality, i.e., the lowest risk (class 1, <25% quantile), moderate risk (class 2, between 25 and 75% quantile), and highest risk (class 3, >75% quantile) for evaluation. We also calculated the internally validated Brier score to assess the overall fit of the model and internally validated Nagelkerke's R2 to measure the explained variation.
Figure 2. Evaluation of the risk model. (A) Distribution of c-statistic in bootstrap and original samples. Internally validated c-statistic is 0.7308. (B) Distribution of observed in-hospital mortality by stratified predictive probability.
To facilitate the use of the selected risk factors and the risk model, we constructed a simple risk score for each patient based on the regression coefficients estimated from the risk model with the original sample. Points for each risk factor were calculated by dividing the risk factor's coefficient by summing the absolute value of coefficients in the model, multiplying by 100, and rounding to the nearest integer. We stratified patients into three risk groups based on the distribution of the risk score: low (<25th percentile), average (25th−75th percentile), and high (>75th percentile).
Analysis was conducted using SAS statistical software version 9.4 (SAS Institute Inc.). The study followed the Transparent Reporting of a Multivariable Prediction Model for Individual Prognosis or Diagnosis (TRIPOD) reporting guideline. Each of the 22 items of the TRIPOD statement was addressed.
A total of 153 eligible patients were enrolled. The general characteristics of the 153 patients are shown in the Table 1. The mean age at diagnosis was 21.6 years (standard deviation 14.2) and 62 (40.5%) patients were female. Fifty-nine patients died and 94 remained continuously survival during follow-up phase. The rate of in-hospital mortality was 41.2% (95% CI, 31.6–50.7%) for the original sample. According to the outcome of patients with OS, these patients were divided into two groups named the death and survival groups.
Of the extremity tumors, 141 (92.2%) were situated in the lower extremities (femur, fibula, tibia), and 12 (7.8%) were situated in the upper extremities (humerus, radius). The primary tumors involved lower limbs tumors for the death and survival groups were 57 (96.6%) patients and 84 patients (89.4%), respectively. As to tumor lesions, the median length of lesion was 10.1 centimeter [interquartile range (IQR) 7–13.2] in the death group and 9.0 centimeter (IQR 6.7–12) in the survival group. There were no significant differences in age, sex, tumor site, and tumor lesion between the two groups, while tumor volume and serum levels of alkaline phosphatase were markedly increased in OS patients with death vs. survival and primary metastasis was significantly related to the overall survival of patients (Table 1).
The MCMC method selected three primitive factors with a posterior probability of at least 0.90, including lower limbs, tumor volume and primary metastasis. The final model was developed with a logistic model, and primary metastasis and tumor volume were selected.
The risk model based on the two risk factors demonstrated good discrimination, calibration, overall fit, and explained variation. The internally validated c-statistic was 0.7308. For calibration, the validated calibration slope was 0.9660. The mean observed in-hospital mortality rate ranged from 21.05% in the lowest predicted quantile to 68.42% in the highest predicted quantile, a range of 47.4%. The validated Brier score was 0.2021, and the validated explained variation was 0.2006.
The risk stratification of in-hospital mortality is demonstrated in Table 2. Based on the distribution of the risk score, 24.8, 49.7, and 25.5% of patients were stratified into the high- (risk score ≥ 67.5+), average- (risk score 4.7–67.5) and low-risk (risk score 0–4.7) groups for in-hospital mortality, with corresponding probabilities of 0.684, 0.329, and 0.210, respectively (Figure 3).
Osteosarcoma is the most common primary malignant bone tumor with higher rates of invasion and metastasis. Although these therapeutic regimens for osteosarcoma have been unprecedentedly advanced, the prognosis is still poor in patients with OS (17). The 5-year overall survival rate of OS patients has remained virtually unchanged in recent decades, especially for metastatic osteosarcomas (6, 17). This may be due to the rarity and heterogeneity of the tumor, together with the lack of pathognomonic mutations identified and the limited targeted treatments thus far (3, 7, 17, 18). Thus, a novel and innovative risk model predicting mortality is urgently needed to increase the understanding of factors identified to exert prognostic effects in patients with OS.
Several clinical trials have been performed to identify the prognostic indicators relevant to the mortality of OS patients, which have been mostly conducted in developed countries. However, the outcomes vary among these studies. The common clinically detected tumor size is widely evaluated in prognostic modeling, whereas the predictive performance of tumor size in predicting outcome was inconsistent among different studies. Some studies indicated that tumor size was one of the significant prognostic factors to predict in-hospital mortality in OS patients (9, 19–22), while tumor size lost its significance in another study; it did not appear to be a significant prognostic factor at all (13). Moreover, worldwide, there is still no consensus as to the standardized risk model that can be used to evaluate the prognosis of OS. Thus, in the absence of availability of these clinical data (such as histologic response to chemotherapy and type of surgery and surgical margins) before the treatment of OS cases, it is difficult to determine whether the prognostic factor evaluated is the true prognostic effect or not and to apply the proposed risk factors to stratify patients at diagnosis before treatment.
We conducted this study based on the patient cohort with OS. First, we analyzed the clinical data of OS cases and selected these potential prognostic variables associated with the outcome. These clinical data, as predictive factors, are conveniently collected, widely reliable, and clinically important during hospitalization and could be applied to predict the outcomes of OS cases by statistical algorithms. Then, we employed MCMC simulation to explore the strength of the correlation between these factors and the prognosis of patients with OS. Two risk factors (metastasis, tumor volume) were identified with the aggressive regimens, which were independent predictive indicators of 5-year survival in OS cases. Additionally, their prognostic value has been recognized in many studies (8–10, 23, 24). Finally, a simple risk model was constructed and evaluated based on the two factors, which reflected good discrimination, calibration, overall fit, and explained variation. We further constructed a simple risk score to stratify patients into three risk groups of in-hospital mortality. Through the risk stratification, we found that 24.8% of the patients were at high risk of in-hospital mortality, which emphasized the importance of identifying these patients to provide them with targeted and systemic treatment and establish appropriate surveillance strategies. Moreover, on the foundation of the risk model, we are able to offer OS cases useful prognostic information and predict survival at diagnosis. Thus, the results of this study demonstrated that not only this risk model but also the risk score had an important potential application in clinical work.
Despite the advantages outlined above, our study has several limitations. First, the study did not have sufficient OS patients at new diagnosis. The main reason is the rarity of the disease and the difficulty in accumulating adequate cases. Second, this was a single-center retrospective study in China, and the performance of the risk model lacked validation in more independent regions and different races. Third, our results were based on the foundation of the existing medical records database and lacked the independent and external validity of this study. Finally, further studies are needed to estimate and confirm the generality of our results.
In summary, in this study, we established a novel prognostic risk model based on clinical data from OS patients at primary diagnosis in China. It may help clinicians stratify patients into different risk groups of in-hospital mortality, provide them with targeted and systemic treatment and establish appropriate surveillance strategies. Hence, these findings offer a direction to predict the prognosis of OS cases.
The original contributions presented in the study are included in the article/supplementary material, further inquiries can be directed to the corresponding author/s.
The studies involving human participants were reviewed and approved by the Ethics Committee of Affiliated Cancer Hospital of Zhengzhou University. Written informed consent for participation was not provided by the participants' legal guardians/next of kin because: The clinical study was retrospective involving human participants. Only the clinical data (gender, age, tumor site, etc.) of hospitalized patients with osteosarcoma are collected, it does not involve intervention in the treatment and prognosis of patients, and does not bring risks to the physiology of patients. Meanwhile, we will do our best to protect patient information and not disclose patient privacy. The Ethics Committee waived the requirement for written informed consent for participation.
YC and ZR designed the study. YC, CL, XW, and ZL retrieved references and analyzed data. YC and CL wrote the manuscript. ZR revised the manuscript. All authors reviewed and approved the manuscript.
This study was sponsored by grants from National Key Research and Development Program of China (2018YFC2000501), National Natural Science Foundation of China (U2004121), and China Postdoctoral Science Foundation (2020T130609).
The authors declare that the research was conducted in the absence of any commercial or financial relationships that could be construed as a potential conflict of interest.
All claims expressed in this article are solely those of the authors and do not necessarily represent those of their affiliated organizations, or those of the publisher, the editors and the reviewers. Any product that may be evaluated in this article, or claim that may be made by its manufacturer, is not guaranteed or endorsed by the publisher.
We thank the colleagues from Department of Orthopedic Surgery and Medical Record Department of Affiliated Cancer Hospital of Zhengzhou University. We thank all the participants who have involved in this study.
1. Ottaviani G, Jaffe N. The epidemiology of osteosarcoma. Cancer Treat Res. (2009) 152:3–13. doi: 10.1007/978-1-4419-0284-9_1
2. Mirabello L, Troisi R, Savage SA. Osteosarcoma incidence and survival rates from 1973 to 2004: data from the surveillance, epidemiology, and end results program. Cancer. (2009) 115:1531–43. doi: 10.1002/cncr.24121
3. Kansara M, Teng M, Smyth M, Thomas DJ. Translational biology of osteosarcoma. Nat Rev Cancer. (2014) 14:722–35. doi: 10.1038/nrc3838
4. Klein M, Siegal GP. Osteosarcoma: anatomic and histologic variants. Am J Clin Pathol. (2006) 125:555–81. doi: 10.1309/UC6KQHLD9LV2KENN
5. Gaspar N, Occean B, Pacquement H, Bompas E, Bouvier C, Brisse H, et al. Results of methotrexate-etoposide-ifosfamide based regimen (M-Ei) in osteosarcoma patients included in the French Os2006/Sarcome-09 Study. Eur J Cancer. (2018) 88:57–66. doi: 10.1016/j.ejca.2017.09.036
6. Allison D, Carney S, Ahlmann E, Hendifar A, Chawla S, Fedenko A, et al. A meta-analysis of osteosarcoma outcomes in the modern medical era. Sarcoma. (2012) 2012:704872. doi: 10.1155/2012/704872
7. Davis A, Bell R, Goodwin PJ. Prognostic factors in osteosarcoma: a critical review. J Clin Oncol. (1994) 12:423–31. doi: 10.1200/JCO.1994.12.2.423
8. Ferrari S, Bertoni F, Mercuri M, Picci P, Giacomini S, Longhi A, et al. Predictive factors of disease-free survival for non-metastatic osteosarcoma of the extremity: an analysis of 300 patients treated at the Rizzoli Institute. Ann Oncol. (2001) 12:1145–50. doi: 10.1023/A:1011636912674
9. Bielack S, Kempf-Bielack B, Delling G, Exner G, Flege S, Helmke K, et al. Prognostic factors in high-grade osteosarcoma of the extremities or trunk: an analysis of 1,702 patients treated on neoadjuvant cooperative osteosarcoma study group protocols. J Clin Oncol. (2002) 20:776–90. doi: 10.1200/JCO.2002.20.3.776
10. Bacci G, Longhi A, Ferrari S, Briccoli A, Donati D, De Paolis M, et al. Prognostic significance of serum lactate dehydrogenase in osteosarcoma of the extremity: experience at Rizzoli on 1421 patients treated over the last 30 years. Tumori. (2004) 90:478–84. doi: 10.1177/030089160409000507
11. Bacci G, Longhi A, Versari M, Mercuri M, Briccoli A, Picci P. Prognostic factors for osteosarcoma of the extremity treated with neoadjuvant chemotherapy: 15-year experience in 789 patients treated at a single institution. Cancer. (2006) 106:1154–61. doi: 10.1002/cncr.21724
12. Whelan J, Jinks R, McTiernan A, Sydes M, Hook J, Trani L, et al. Survival from high-grade localised extremity osteosarcoma: combined results and prognostic factors from three european osteosarcoma intergroup randomised controlled trials. Ann Oncol. (2012) 23:1607–16. doi: 10.1093/annonc/mdr491
13. Ogura K, Fujiwara T, Yasunaga H, Matsui H, Jeon D, Cho W, et al. Development and external validation of nomograms predicting distant metastases and overall survival after neoadjuvant chemotherapy and surgery for patients with nonmetastatic osteosarcoma: a multi-institutional study. Cancer. (2015) 121:3844–52. doi: 10.1002/cncr.29575
14. Bertrand T, Cruz A, Binitie O, Cheong D, Letson GD. Do surgical margins affect local recurrence and survival in extremity, nonmetastatic, high-grade osteosarcoma? Clin Orthop Relat Res. (2016) 474:677–83. doi: 10.1007/s11999-015-4359-x
15. Harrell FE Jr, Lee KL, Mark DB. Multivariable prognostic models: issues in developing models, evaluating assumptions and adequacy, and measuring and reducing errors. Stat Med. (1996) 15:361–87. doi: 10.1002/(SICI)1097-0258(19960229)15:4<361::AID-SIM168>3.0.CO;2-4
16. Steyerberg E, Harrell F, Borsboom G, Eijkemans M, Vergouwe Y, Habbema JD. Internal validation of predictive models: efficiency of some procedures for logistic regression analysis. J Clin Epidemiol. (2001) 54:774–81. doi: 10.1016/S0895-4356(01)00341-9
17. Gill J, Gorlick G. Advancing therapy for osteosarcoma. Nat Rev Clin Oncol. (2021) 18:609–24. doi: 10.1038/s41571-021-00519-8
18. Corre I, Verrecchia F, Crenn V, Redini F, Trichet VJC. The osteosarcoma microenvironment: a complex but targetable ecosystem. Cells. (2020) 9. doi: 10.3390/cells9040976
19. Wuisman P, Enneking W, Roesner A. Local growth and the prognosis of osteosarcoma. Int Orthop. (1992) 16:55–8. doi: 10.1007/BF00182986
20. Spanier S, Shuster J, Vander Griend RA. The effect of local extent of the tumor on prognosis in osteosarcoma. J Bone Joint Surg. (1990) 72:643–53. doi: 10.2106/00004623-199072050-00002
21. Mankin H, Hornicek F, Rosenberg A, Harmon D, Gebhardt MC. Survival data for 648 patients with osteosarcoma treated at one institution. Clin Orthopaed Relat Res. (2004) 429:286–91. doi: 10.1097/01.blo.0000145991.65770.e6
22. Petrilli A, Gentil F, Epelman S, Lopes L, Bianchi A, Lopes A, et al. Increased survival, limb preservation, and prognostic factors for osteosarcoma. Cancer. (1991) 68:733–7. doi: 10.1002/1097-0142(19910815)68:4<733::AID-CNCR2820680412>3.0.CO;2-0
23. Kager L, Zoubek A, Pötschger U, Kastner U, Flege S, Kempf-Bielack B, et al. Primary metastatic osteosarcoma: presentation and outcome of patients treated on neoadjuvant cooperative osteosarcoma study group protocols. J Clin Oncol. (2003) 21:2011–8. doi: 10.1200/JCO.2003.08.132
Keywords: osteosarcoma, clinical data, outcome, prognostic factors, risk model
Citation: Chen Y, Li C, Wang X, Liu Z and Ren Z (2022) Development of a Simple Risk Model to Predict Mortality in Patients With Osteosarcoma of the Extremity. Front. Med. 9:852529. doi: 10.3389/fmed.2022.852529
Received: 11 January 2022; Accepted: 21 April 2022;
Published: 23 May 2022.
Edited by:
Muntaser Eltayeb Ibrahim, University of Khartoum, SudanCopyright © 2022 Chen, Li, Wang, Liu and Ren. This is an open-access article distributed under the terms of the Creative Commons Attribution License (CC BY). The use, distribution or reproduction in other forums is permitted, provided the original author(s) and the copyright owner(s) are credited and that the original publication in this journal is cited, in accordance with accepted academic practice. No use, distribution or reproduction is permitted which does not comply with these terms.
*Correspondence: Zhigang Ren, fccrenzg@zzu.edu.cn
Disclaimer: All claims expressed in this article are solely those of the authors and do not necessarily represent those of their affiliated organizations, or those of the publisher, the editors and the reviewers. Any product that may be evaluated in this article or claim that may be made by its manufacturer is not guaranteed or endorsed by the publisher.
Research integrity at Frontiers
Learn more about the work of our research integrity team to safeguard the quality of each article we publish.