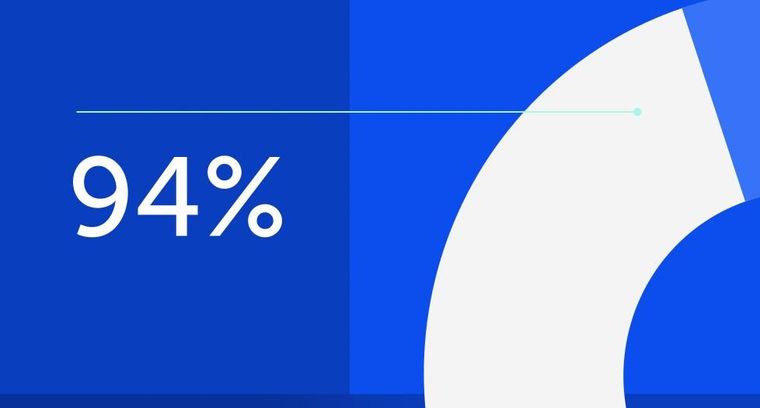
94% of researchers rate our articles as excellent or good
Learn more about the work of our research integrity team to safeguard the quality of each article we publish.
Find out more
ORIGINAL RESEARCH article
Front. Med., 22 December 2021
Sec. Nuclear Medicine
Volume 8 - 2021 | https://doi.org/10.3389/fmed.2021.792677
This article is part of the Research TopicArtificial Intelligence in Positron Emission TomographyView all 13 articles
Purpose: Hemophagocytic lymphohistiocytosis (HLH) is a rare and severe disease with a poor prognosis. We aimed to determine if 18F-fluorodeoxyglucose (18F-FDG) PET/CT-derived radiomic features alone or combination with clinical parameters could predict survival in adult HLH.
Methods: This study included 70 adults with HLH (training cohort, n = 50; validation cohort, n = 20) who underwent pretherapeutic 18F-FDG PET/CT scans between August 2016 and June 2020. Radiomic features were extracted from the liver and spleen on CT and PET images. For evaluation of 6-month survival, the features exhibiting p < 0.1 in the univariate analysis between non-survivors and survivors were selected. The least absolute shrinkage and selection operator (LASSO) regression analysis was used to develop a radiomics score (Rad-score). A nomogram was built by the multivariate regression analysis to visualize the predictive model for 3-month, 6-month, and 1-year survival, while the performance and usefulness of the model were evaluated by calibration curves, the receiver operating characteristic (ROC) curves, and decision curves.
Results: The Rad-score was able to predict 6-month survival in adult HLH, with area under the ROC curves (AUCs) of 0.927 (95% CI: 0.878–0.974) and 0.869 (95% CI: 0.697–1.000) in the training and validation cohorts, respectively. The radiomics nomogram combining the Rad-score with the clinical parameters resulted in better performance for predicting 6-month survival than the clinical model or the Rad-score alone. Moreover, the nomogram displayed superior discrimination, calibration, and clinical usefulness in both the cohorts.
Conclusion: The newly developed Rad-score is a powerful predictor for overall survival (OS) in adults with HLH. The nomogram has great potential for predicting 3-month, 6-month, and 1-year survival, which may timely guide personalized treatments for adult HLH.
Hemophagocytic lymphohistiocytosis (HLH) is a syndrome of severe immune activation and dysregulation characterized by hyperactive cytotoxic T lymphocytes, natural killer (NK) cells, and macrophages leading to cytokine storm and immune-mediated multiple organ failure (1, 2). Historically, HLH has been classified as primary or familial HLH driven by underlying genetic defects in cytotoxic immune function or as secondary or reactive HLH caused by infections [e.g., Epstein-Barr virus (EBV), cytomegalovirus (CMV), HIV, and coronavirus disease 2019 (COVID-19)], malignancies (e.g., hematologic malignancies), and autoimmune diseases (e.g., macrophage activation syndrome) (1). Emerging evidence demonstrated that HLH may occur in patients of any age and is most often driven by an integration of genetic defects and acquired exposures (3, 4). Primary HLH occurs in 1/50,000–1/100,000 live-born children, while secondary HLH occurs in older children and adults (1). The precise incidence of adult HLH is still unknown, but it accounts for ~40% of all HLH (1, 5). The frequent manifestations are intermittent fever, hepatosplenomegaly, lymphadenopathy, liver injury, cytopenia, hypertriglyceridemia, hyperferritinemia, and hemophagocytosis (1). Because of few data and/or no prospective studies for adult HLH, pediatric data are often generalized to guide diagnostic, therapeutic, and prognostic decision-making in adults (1, 6). In general, adults have poorer outcome than children even with aggressive therapy, with a median survival of 4 months (1). The principal reasons for mortality are multiorgan failure, hemorrhage, and sepsis, which can be treated properly if diagnosed early (7). Therefore, identifying poor prognosis in adult HLH is crucial for risk stratification and therapeutic decision-making. Recently, it has been reported that clinical and laboratory markers are correlated with survival in adult HLH including age, platelet, fibrinogen, albumin, serum ferritin, alanine aminotransferase (ALT), and malignancy (1, 2, 8, 9). But none of them can be a single effective prognostic factor as a result of poor sensitivity and/or specificity. Hence, it would be of great utility to build a predictive model to precisely evaluate the prognosis in adult HLH based on multiple indictors.
18F-fluorodeoxyglucose (18F-FDG) PET/CT has been employed for detecting underlying malignancy and predicting prognosis of adult HLH (10–12). One of the most common PET/CT finding is hepatosplenomegaly with diffusely increased FDG uptake, which contains a great deal of information reflecting disease status in adult HLH (13, 14). Radiomics can convert medical images into quantitative data and subsequently analyze these data for prognosis prediction by high-throughput computing. PET/CT radiomic features have been explored to predict outcome in malignancies such as lymphoma and lung cancer (15–17). It has been suggested that the quantitative PET parameters of spleen are independent prognostic factors (11, 12), but whether PET/CT radiomic features extracted from liver and spleen can be applied for outcome prognostication in adult HLH is unclear yet. Therefore, the first aim of this study was to establish a PET/CT radiomics score (Rad-score) for predicting 6-month survival in adult HLH and the second aim was to combine the Rad-score with clinical parameters, in order to develop a nomogram for predicting individual prognosis accurately and reliably.
This retrospective study was approved by Institutional Review Board of Beijing Friendship Hospital of Capital Medical University and the requirement of a written informed consent was waived. The medical records of 185 consecutive adult patients (age ≥ 18 years) with a diagnosis of HLH were reviewed from August 2016 to June 2020. The diagnostic criteria of HLH were in accordance with HLH-2004 protocol, which requires five of the following eight criteria: (1) fever; (2) splenomegaly; (3) cytopenia affecting ≥ 2 lineages (Hemoglobin (HGB) < 9 g/dl, platelets < 100 × 109/L, neutrophils < 1.0 × 109/L); (4) serum triglyceride ≥ 265 mg/dl and/or fibrinogen ≤ 150 mg/dl; (5) hemophagocytosis in bone marrow, spleen, lymph nodes, or liver; (6) low or absent NK cell activity; (7) Ferritin ≥ 500 μg/l; and (8) soluble interleukin-2 receptor (soluble CD25) ≥ 2,400 U/ml (18). The exclusion criteria included: patients with receiving chemotherapy before 18F-FDG PET/CT scan (n = 114) or incomplete follow-up (n = 1). Consequently, a total of 70 patients were included in this study. All the patients received personalized treatments in the Department of Hematology and were followed-up for at least 180 days with a median of 353 days. These cases were randomly divided into the training (n = 50) and validation cohorts (n = 20) with a ratio of 5:2.
Clinical parameters including age, gender, malignancy, EBV infection, hemophagocytosis, and laboratory variables [white blood cell, absolute neutrophil, hemoglobin, platelet, C-reactive protein (CRP), ALT, aspartate aminotransferase (AST), triglycerides, serum ferritin, fibrinogen, erythrocyte sedimentation rate, and lactate dehydrogenase] were obtained from medical records (Table 1). All the laboratory and radiological data were collected before initial HLH-specific therapy. The most likely trigger of secondary HLH (malignancy, infection, autoimmune, and idiopathic) was determined by assessment and medical evidence of physician.
18F-fluorodeoxyglucose PET/CT was performed on a Siemens biography mCT PET/CT scanner (Siemens Healthineers, Erlangen, Germany). Patients were instructed to fast for at least 6 h, accompanied by blood glucose <11.1 mmol/l. Then, 18F-FDG (4.4 MBq/kg) was injected intravenously. After a 60-min uptake time, low-dose CT scan was executed for visualization of anatomic structures and attenuation correction, with 140 keV, automatic mAs, and a slice thickness of 3 mm. The whole-body PET scan was carried out with 2.5 min per bed position using three-dimensional (3D) mode immediately after a whole-body CT scan. Images were reconstructed with an iterative reconstruction algorithm.
The entire liver and spleen on CT images were defined as the regions of interest (ROIs), which were delineated by two experienced nuclear radiologists with a validated semi-automatic approach using (3D SlicerTM software, Boston, Massachusetts, United States) (version 4.10.0, http://www.slicer.org) (Figure 1) (19). Moreover, the ROIs were resampled exploiting B-spline interpolation in order for mapping those onto the PET images. In consequence, the ROIs had the matching pixel spacing with the PET images.
Radiomic features were extracted from 18F-FDG PET and CT images separately, using pyradiomics that is an open-source Python package (20). These included first-order features (n = 18), shape features (n = 14), gray level co-occurrence matrix (GLCM) features (n = 24), gray level run length matrix (GLRLM) features (n = 16), gray level size zone matrix (GLSZM) features (n = 16), neighboring gray tone difference matrix (NGTDM) features (n = 5), and gray level dependence matrix (GLDM) features (n = 14). Image processing utilized wavelet filtering, square, square root, logarithm, exponential, and gradient. Overall, a total of 5,264 radiomic features (4 × 1,317) were obtained from liver and spleen on PET and CT images.
Our workflow is shown in Figure 1. Firstly, the univariate analysis (t-test for normally distributed variables or the Mann–Whitney U test for skewed distributed variables) was used to compare differences of radiomic features between non-survivors and survivors at 180 days in the training set. The total of 384 features with p-values < 0.1 were retained for further analysis. Next, the least absolute shrinkage and selection operator (LASSO) algorithm was applied to select the optimal features among 384 features in the training set, adding L1 regularization term to a least square algorithm for data dimension reduction. Because of imbalanced datasets, the synthetic minority oversampling technique (SMOTE) was used to improve random oversampling in the training set. An individualized Rad-score was calculated from a linear combination of the selected features weighted by their respective coefficients. The receiver operating characteristic (ROC) curve was employed to evaluate the prediction accuracy and determine the optimal threshold of the Rad-score. All the patients were divided into high- and low-risk groups according to the maximum Youden index of the ROC curve. The potential association of the Rad-score with overall survival (OS) was evaluated by the Kaplan–Meier survival analysis and the log-rank test in the training and validation cohorts.
To build a powerful model and a robust nomogram for the survival prediction, the clinical prognostic factors were chosen by the univariate Cox regression analyses (p < 0.05). Then, the Rad-score and the strong clinical indicators were incorporated to establish the multivariate Cox regression model that was visualized by a nomogram. The Harrell's concordance-index (C-index) was employed to assess the model performance and calibration curves were plotted to enhance the predictive precision of nomogram. Similarly, a clinical model was established with clinical information alone by the multivariate Cox regression analysis. Three different types of predictive models (clinical variables, the Rad-score, and their combinations) were evaluated by the C-index. Decision curve analysis (DCA) was utilized to assess the clinical usefulness of the models.
Continuous variables are presented as medians with interquartile ranges and categorical variables are presented as frequencies and percentages. OS was defined as the time from the initial diagnosis of HLH to the date of death from any cause or deadline of follow-up. All the p-values were two-sided, with a significant level of <0.05. Statistical analyses were performed with Python (version 3.7.8, www.python.org) and R (version 4.0.3, www.r-project.org). The Python packages “sklearn,” “numpy,” and “pandas” were used for the LASSO binary logistic regression and the ROC curve; the “scipy” was for analyzing statistical properties; and the “imblearn” was for analyzing SMOTE. The R package “rms” was employed to create nomograms.
A total of 70 adults with HLH were included in this study who fulfilled the inclusion criteria. There were 36 males and 34 females and the median age at diagnosis was 38 years (range: 18–79 years). The baseline characteristics of all the patients are shown in Table 1.
The possible triggers of HLH in these patients were as follows: 35 (50.0%) infections, 22 (31.4%) malignancies, 7 (10%) autoimmune diseases, and 6 (8.6%) unknown disorders. In the 35 cases with infectious disorders, viral infections were the most common cause with 23 (65.7%) EBV, 3 (8.6%) CMV, and 5 (14.3%) other viruses. Bacterial infections were identified in 4 (11.4%) patients. Among 22 malignancy-associated HLH cases, diffuse large B-cell lymphoma (n = 6, 27.3%) and NK/T-cell lymphoma (n = 6, 27.3%) were the most frequent triggers. The other malignancy-associated patients with HLH were two classical Hodgkin's lymphoma, two peripheral T-cell lymphoma, two unclassified T-cell lymphoma, one follicular lymphoma, one non-Hodgkin B cell lymphoma, one anaplastic large cell lymphoma, and one acute lymphocytic leukemia. Concomitant malignancies and EBV infection were found in 11 (15.7%) patients. Adult Still's disease (n = 5, 71.4%) was the most common diagnosis among autoimmune diseases and the other two patients were diagnosed with systemic lupus erythematosus and undifferentiated systemic rheumatic disease, respectively.
The baseline characteristics in the training and validation cohorts are also given in Table 1. Obviously, the clinical variables had no differences between the two cohorts (p > 0.05). After a median follow-up of 353 days (range: 9–1,216 days), 30 patients (42.8%) had died.
The optimal radiomic features were selected by the LASSO algorithm and 10-fold cross-validation (Figure 2). Eventually, six features were extracted to construct the Rad-score in the training set, which included 3 CT features and 3 PET features. The selected features were spleen_CT_wavelet-HHH_GLSZM_gray level non-uniformity normalized, spleen_CT_wavelet-LHL_NGTDM_contrast, liver_CT_wavelet-HHH_GLCM_informational measure of correlation (IMC) 2, spleen_PET_square root_first order_kurtosis, spleen_PET_wavelet-LHL_GLSZM_size zone non-uniformity normalized, and liver_PET_wavelet-HHL_GLSZM_small area emphasis. Among these features, there were four from spleen and two were from liver. The Rad-score for each patient was calculated by the following formula:
Figure 2. Feature selection for the prediction using the least absolute shrinkage and selection operator (LASSO) regression model, tuning parameter (λ) selection in the LASSO model involved the use of tenfold cross-validation (A). In the coefficient profiles of the radiomics features for OS prediction, a value of Lambda = 0.044668 was selected as the optimal value (B).
Rad-score = 13.762 + 300.60 × spleen_CT_wavelet-LHL_NGTDM_contrast – 9.1753 × spleen_CT_wavelet-HHH_GLSZM_gray level non-uniformity normalized – 0.17938 × spleen_PET_square root_first order_kurtosis + 13.305 × spleen_PET_wavelet-LHL_GLSZM_size zone non-uniformity normalized – 246.77 × liver_CT_wavelet-HHH_GLCM_IMC 2 + 4.2599 × liver_PET_wavelet-HHL_GLSZM_small area emphasis.
The median and the interquartile range for the selected radiomics features in the training cohort are shown in Table 2. The Rad-score in the training and validation cohorts are shown in Table 3. Not surprisingly, the Rad-score had notable difference between non-survivors and survivors in the training (p < 0.001) and validation cohorts (p = 0.011). Particularly, non-survivors had the higher Rad-score than survivors in the training (Rad-score = 1.6386 vs. −1.0608) and validation cohorts (Rad-score = 1.3763 vs. −1.3595). The Rad-score for individuals in the both the cohorts is shown in Figures 3A,B.
Table 2. Comparison of the radiomics features between 180-day survivors and non-survivors in the training cohort.
Table 3. Comparison of the Rad-score between 180-day survivors and non-survivors in both the training and validation cohorts.
Figure 3. Rad-score of patients in the training and validation cohorts (A,B) and time-dependent ROC analysis of Rad-score at 180 days (C). Kaplan-Meier survival analysis with the best cutoff value of the Rad-score in the training cohort (D) and validation cohort (E). We calculated p values using the log-rank test.
In addition, the Rad-score had good predictive power for survival forecast at 180 days and its area under the ROC curves (AUCs) in distinguish high-risk status were 0.927 (95% CI: 0.879–0.975) in the training set and 0.869 (95% CI: 0.684–1.000) in the validation set (Figure 3C). The best cutoff with maximum Youden index was −0.3 and, therefore, patients were divided into high- and low-risk groups according to the Rad-score in the both the cohorts. The Kaplan–Meier curves and the log-rank test found that patients in low-risk category had a better prognosis than those in high-risk category in the training and validation cohorts (p < 0.05) (Figures 3D,E).
The univariate Cox regression analysis showed that 6 parameters were significantly associated with OS including the Rad-score, T-cell neoplasms, white blood cell, hemoglobin, platelet count, and CRP (p < 0.05; Table 4). The multivariate analysis displayed that the Rad-score, white blood cell, and CRP were consistently strong predictors (Table 4), which were used to build the combined model. When the Rad-score was excluded, three variables (T-cell neoplasms, hemoglobin, and platelet count) were independent prognostic factors among clinical parameters (Table 5). Likewise, these three prognostic factors were used to build the clinical model.
Table 4. The univariate and multivariate Cox hazards regression analysis of OS in the training cohort.
Table 5. The multivariate Cox hazards regression analysis of OS in the training cohort without the Rad-score.
To assess the performance of models in predicting prognosis, the C-indices of three types of models were shown in Table 6. The Rad-score model had acceptable predictive ability with C-indices of 0.795 (95% CI: 0.695–0.895) and 0.752 (95% CI: 0.591–0.913) in the training and validation cohorts, respectively. The C-indices of the clinical model were of 0.765 (95% CI: 0.665–0.865) and 0.762 (0.527–0.997) in the training and validation cohorts, respectively. It was noticeable that the combined radiomics model had the highest C-indices, with 0.831 (95% CI: 0.749–0.913) and 0.810 (95% CI: 0.657–0.963) in both the training and validation cohorts, sequentially. The curves of decision-curve analysis (DCAs) indicated that the combined radiomics model provided more net clinical benefit than clinical model with a threshold > 0.25 (Figure 4).
Figure 4. Decision-curve analysis for the radiomics model and clinical model. The threshold probability represents the predicted 180-day risk of death for recommending aggressive treatment.
Given that the combined model possessed synergetic power for survival prediction, the personalized nomogram was constructed by incorporating all the three independent prognostic factors (the Rad-score, white blood cell, and CRP) (Figure 5A), which can visualize the prediction outcome and the proportion of each factor. The calibration curves demonstrated good agreements between the predicted and observed values in the training and validation cohorts, indicating that the nomogram was able to precisely predict 6-month survival (Figures 5B,C).
Figure 5. The radiomics nomogram for predicting overall survival for adult HLH patients (A). Points for Rad-score, WBC, and CRP can be obtained by calibrating with the point caliper, and then combined to obtain a total score that can be calibrated with the patient's probability of survival at different time. Calibration curves of the radiomics nomogram in the training cohort (B) and validation cohort (C). Nomogram-estimated OS is plotted on the x-axis; the observed OS is plotted on the y-axis. The diagonal dotted line is a perfect estimation by an ideal model, in which the estimated outcome perfectly corresponds to the actual outcome. The solid red line represents performance of the nomogram: A smaller distance of the scatter points from the dotted line indicates better calibration.
Timely diagnosis and prognosis are critical for HLH considering that the early and proper administration of an efficacious therapy can improve survival. In this study, the novel prognostic factors and predictive models associated with 6-month survival in adult patients with HLH are reported via pretherapeutic 18F-FDG PET/CT radiomics analysis. The Rad-score and the combined prediction model (the Rad-score and clinical variable combination) have been developed for quantitative identification of the adults with HLH at high risk of death within 6 months in 70 patients.
18F-fluorodeoxyglucose PET/CT is a whole-body scan containing both the metabolic and anatomical information, which has been recommended for identifying possible triggers and suitable biopsy sites in secondary HLH (6). However, 18F-FDG PET/CT findings are non-specific, since inflammatory response and malignant lesions have the same manifestation that is hypermetabolism. In HLH, 18F-FDG PET/CT often shows diffusely increased FDG uptake in spleen, liver, and bone marrow with or without focal lesions and hypermetabolic lymph nodes. Increasing evidence demonstrated that these non-specific presentations can be used to assess systemic inflammatory response and have potential for prognosis prediction in HLH (21). For instance, the FDG uptake of spleen and bone marrow has been considered as prognostic factors in adult patients with HLH (11, 12, 22). More importantly, spleen and liver, components of the reticuloendothelial system, are the most frequent abnormal signs in HLH (23). Our data proved that 6 radiomic features from spleen and liver were linked with the prognosis of adult HLH, thus utilized for establishment of the Rad-score. Among six radiomic features, two-thirds (4/6) were derived from spleen including GLSZM size zone non-uniformity normalized feature and kurtosis of spleen PET and GLSZM gray level non-uniformity normalized feature and NGTDM contrast of spleen CT. It is well-known that spleen is the largest secondary lymphoid organ and a site where immune responses can be controlled by activated immune cells. As splenomegaly is one of the diagnostic criteria of HLH, the hypermetabolic spleen has been discovered to be correlated with high inflammatory response and cytokine activity (24, 25). One recent report suggested that spleen FDG uptake may provide useful information for predicting in-hospital mortality in autoimmune diseases including HLH (26). Another study of 43 patients with secondary HLH found that the ratio of spleen to mediastinum in the average standardized uptake value (SUV) was an independent predictor for survival (12). In consistent with these statements, our findings indicated that radiomic features of spleen possessed a powerful predictive ability for 6-month survival in adult patients with HLH. The rest of two radiomic features were extracted from liver including GLCM IMC2 (Informational Measure of Correlation) of liver CT and GLSZM small area emphasis of liver PET. Radiomics have showed great value in characterization of diffuse liver diseases such as non-alcoholic steatohepatitis and chronic hepatitis B (27). In addition, the well-known liver enzymes, AST and ALT, are identified as indicators of various diseases including HLH. High ALT and AST/ALT ratio have been found to act as adverse prognostic factors in adult HLH (8, 28). Furthermore, hepatic involvement and hepatomegaly reveal poor prognostic indicators and early death predictors in HLH (8, 28). In line with these studies, our results illustrated that the radiomic features of spleen and liver presented great prognostic values for adult HLH.
Radiomics is a high-throughput extraction of quantitative information from medical images as well as subtle manifestations that are difficult to recognize or quantify by human eyes. Compared with the traditional PET/CT metrics, the radiomic features may reflect the pathological process much more sufficiently in the spleen and liver of patients with HLH. In this study, the majority of the selected radiomic features (5/6) were derived from wavelet decomposition images, indicating that wavelet transforms emphasize image details. It is very likely that wavelet decomposition images contain inconspicuous prognostic information (29, 30). GLSZM quantifies the number of groups of interconnected neighboring voxels with the same gray level intensity. NGTDM represents contrast, quantifying the difference between the gray level of a voxel and the average level of its neighbors within a distance. GLCM captures spatial relationships of pairs of voxels, while kurtosis is a first-order feature expressing the peak of the distribution of values in the ROI. All the selected features describe the texture of the spleen and liver quantitatively, reflecting uniformity or heterogeneity in both the organs (31). Previous studies found that intratumor heterogeneity was associated with poor outcome in various malignancies (15, 17, 30, 32, 33). HLH is a heterogeneous disease with various etiological components and complex underlying genetic variant types (3). Each possible etiology has distinct clinical characteristics and prognosis. Even in lymphoma-associated HLH that has the worst prognosis, the treatment response is diverse (34). HLH could occur in EBV-associated T-/NK-cell lymphoproliferative disorders, which is a spectrum of disease from infection to malignancy. The histological features and immunophenotype are markedly heterogeneous. As in children, multiple gene mutations are linked to the development of HLH in adults, especially with the EBV-driven lymphoma, which requires hematopoietic cell transplant (6, 35, 36). The 18F-FDG appearance of the liver and spleen came in various sizes, densities, and metabolisms, which reflected the heterogeneity of HLH. Radiomics quantified the spatial complexity of them. This study exhibited that the heterogeneity of spleen and liver may reveal overproliferation of immune cells accompanied with inflammatory infiltration triggered by EBV infection (37–40). On the other hand, the heterogeneous distribution of metabolism or density may also suggest the involvement of tumor cells (41–43). Both the malignancy and EBV infection seemed to link an inferior prognosis in adult HLH (9, 34, 44, 45). Additionally, the radiomic features have the possibilities associated with genetic signatures (3, 46); however, the underlying biological significance of these radiomic features has not been fully studied and the relationships among radiomic features, genetic signatures, and prognosis need further exploration in HLH.
Recent studies pointed out that a number of clinical parameters play a role in the prognosis of HLH such as lymphoid malignancy, hemoglobin, platelets, CRP, and cytopenia [(8, 9, 50, 51)]. It is well-documented that lymphoid malignancy is negatively associated with survival. Typically, T-cell lymphoma is acknowledged to have a more severe survival due to poor response to chemotherapy, in comparison with B-cell lymphoma (47). In a large-scale Japanese study, the 5-year OS was the worst in T-/NK-cell lymphoma-associated HLH compared with other types of HLH including primary HLH, B-cell lymphoma-associated HLH, and infection-associated HLH (48). Lower hemoglobin and platelet have been reported to be the more consistent negative prognostic biomarkers in HLH (8, 49). This study consistently showed that these 3 clinical parameters were involved in the clinical prediction model. However, the two clinical variables incorporated in the nomogram were white blood cell and CRP. Cytopenia is one of the major presentation in HLH. Serious cytopenia may mark the severity of a cytokine storm and lead to hemorrhage and sepsis, suggesting to be an inferior factor (7). CRP is the prototypical acute phase serum protein, increasing rapidly during inflammation (50). It has been highlighted that CRP is markedly enhanced in patients with secondary HLH compared to primary ones. High CRP levels have been correlated with increased risk of infection and overall mortality in HLH, suggested to be indices of disease severity (51). CRP probably serves as a predictor of 18F-FDG PET/CT effectiveness due to the fact that the diagnostic accuracy of PET/CT is positively linked with CRP > 60 mg/l in HLH (12).
Interestingly, T-cell neoplasms were not retained in the predictive model when the Rad-score was incorporated. A possible explanation was that the Rad-score contained partial pathological information. The inclusion of the Rad-score not only improved the prognostic performance, but also simplified the prediction model. DCA demonstrated that the nomogram with the Rad-score and two clinical parameters was superior to the clinical model in terms of clinical application. Overall, the nomogram was successfully built to predict 3-month, 6-month, and 1-year survival of adults with HLH and the accuracy and clinical applicability of the model were verified through C-index, calibration curve, and DCA.
This study has several limitations. First, patients may have been missed for inclusion in a single-center study and selection bias may occur because of the retrospective nature of the study design. Second, the heterogeneity of the patients and treatments may affect our results. Third, gene, transcript, and protein signatures become increasingly important for the prognosis of adult HLH (3), but these data were not collected. Finally, the Rad-score was calculated using ROIs that were manually delineated in 3D slicer. It was time-consuming and inconvenient for clinical practice, so automatic or semi-automatic image segmentation will be needed. Notably, a multicenter and prospective study with larger cohort will be required to validate our findings in the future.
This preliminary study indicated that the pretherapeutic 18F-FDG PET/CT radiomic features of spleen and liver are independent prognostic factors in adult HLH, with the heterogeneity of spleen and liver associated with inferior prognosis. Integrating radiomic features with clinical parameters show synergetic power for 6-month survival prediction compared to other models with radiomics features or clinical parameters alone. The nomogram has great potential for predicting individualized 3-month, 6-month, and 1-year survival, which may timely guide personalized treatments for adult HLH.
The raw data supporting the conclusions of this article will be made available by the authors, without undue reservation.
The studies involving human participants were reviewed and approved by Institutional Review Board of Beijing Friendship Hospital of Capital Medical University. Written informed consent for participation was not required for this study in accordance with the national legislation and the institutional requirements. No potentially identifiable human images or data are presented in the manuscript.
JY, YK, and HZ contributed to the study design, decision-making, and coordination of the study. XY, JLiu, XL, WW, and SZ contributed to the management of registration of cases and collected PET/CT image data. XY, JLiu, XL, WW, and YK contributed to the image quality control, analysis, and data interpretation. LL and HZ contributed to the statistical analysis. XY, JLi, and JY contributed to the drafting and revising the manuscript. All the authors read, revised, and approved the final version of the manuscript.
This study was supported by the National Natural Science Foundation of China (Nos: 81971642 and 81771860), the Beijing Natural Science Foundation (No: 7192041), and the National Key Research and Development Plan (No: 2020YFC0122000).
LL was employed by Sinounion Medical Technology (Beijing) Corporation, Ltd.
The remaining authors declare that the research was conducted in the absence of any commercial or financial relationships that could be construed as a potential conflict of interest.
All claims expressed in this article are solely those of the authors and do not necessarily represent those of their affiliated organizations, or those of the publisher, the editors and the reviewers. Any product that may be evaluated in this article, or claim that may be made by its manufacturer, is not guaranteed or endorsed by the publisher.
1. Al-Samkari H, Berliner N. Hemophagocytic lymphohistiocytosis. Annu Rev Pathol. (2018) 13:27–49. doi: 10.1146/annurev-pathol-020117-043625
2. Birndt S, Schenk T, Heinevetter B, Brunkhorst FM, Maschmeyer G, Rothmann F, et al. Hemophagocytic lymphohistiocytosis in adults: collaborative analysis of 137 cases of a nationwide German registry. J Cancer Res Clin Oncol. (2020) 146:1065–77. doi: 10.1007/s00432-020-03139-4
3. Zhang J, Sun Y, Shi X, Zhang R, Wang Y, Xiao J, et al. Genotype characteristics and immunological indicator evaluation of 311 hemophagocytic lymphohistiocytosis cases in China. Orphanet J Rare Dis. (2020) 15:112. doi: 10.1186/s13023-020-01390-z
4. Canna SW, Marsh RA. Pediatric hemophagocytic lymphohistiocytosis. Blood. (2020) 135:1332–43. doi: 10.1182/blood.2019000936
5. Ramos-Casals M, Brito-Zeron P, Lopez-Guillermo A, Khamashta MA, Bosch X. Adult haemophagocytic syndrome. Lancet. (2014) 383:1503–16. doi: 10.1016/S0140-6736(13)61048-X
6. La Rosée P, Horne A, Hines M, von Bahr Greenwood T, Machowicz R, Berliner N, et al. Recommendations for the management of hemophagocytic lymphohistiocytosis in adults. Blood. (2019) 133:2465–77. doi: 10.1182/blood.2018894618
7. Merrill SA, Naik R, Streiff MB, Shanbhag S, Lanzkron S, Braunstein EM, et al. A prospective quality improvement initiative in adult hemophagocytic lymphohistiocytosis to improve testing and a framework to facilitate trigger identification and mitigate hemorrhage from retrospective analysis. Medicine (Baltimore). (2018) 97:e11579. doi: 10.1097/MD.0000000000011579
8. Zhou J, Zhou J, Wu ZQ, Goyal H, Xu HG. A novel prognostic model for adult patients with hemophagocytic lymphohistiocytosis. Orphanet J Rare Dis. (2020) 15:215. doi: 10.1186/s13023-020-01496-4
9. Yoon SE, Eun Y, Huh K, Chung CR, Yoo IY, Cho J, et al. A comprehensive analysis of adult patients with secondary hemophagocytic lymphohistiocytosis: a prospective cohort study. Ann Hematol. (2020) 99:2095–104. doi: 10.1007/s00277-020-04083-6
10. Yuan L, Kan Y, Meeks JK, Ma D, Yang J. 18F-FDG PET/CT for identifying the potential causes and extent of secondary hemophagocytic lymphohistiocytosis. Diagn Interv Radiol. (2016) 22:471–5. doi: 10.5152/dir.2016.15226
11. Kim J, Yoo SW, Kang SR, Bom HS, Song HC, Min JJ. Clinical implication of F-18 FDG PET/CT in patients with secondary hemophagocytic lymphohistiocytosis. Ann Hematol. (2014) 93:661–7. doi: 10.1007/s00277-013-1906-y
12. Zheng Y, Hu G, Liu Y, Ma Y, Dang Y, Li F, et al. The role of (18)F-FDG PET/CT in the management of patients with secondary haemophagocytic lymphohistiocytosis. Clin Radiol. (2016) 71:1248–54. doi: 10.1016/j.crad.2016.05.011
13. Shieh AC, Guler E, Smith DA, Tirumani SH, Beck RC, Ramaiya NH. Hemophagocytic lymphohistiocytosis: a primer for radiologists. AJR Am J Roentgenol. (2020) 214:W11–W9. doi: 10.2214/AJR.19.21788
14. Abou Shaar R, Eby CS, van Dorp S, de Witte T, Otrock ZK. Increasing ferritin predicts early death in adult hemophagocytic lymphohistiocytosis. Int J Lab Hematol. (2021) 43:1024–31. doi: 10.1111/ijlh.13489
15. Wang H, Zhao S, Li L, Tian R. Development and validation of an (18)F-FDG PET radiomic model for prognosis prediction in patients with nasal-type extranodal natural killer/T cell lymphoma. Eur Radiol. (2020) 30:5578–87. doi: 10.1007/s00330-020-06943-1
16. Cottereau AS, Nioche C, Dirand AS, Clerc J, Morschhauser F, Casasnovas O, et al. (18)F-FDG PET dissemination features in diffuse large B-cell lymphoma are predictive of outcome. J Nucl Med. (2020) 61:40–5. doi: 10.2967/jnumed.119.229450
17. Arshad MA, Thornton A, Lu H, Tam H, Wallitt K, Rodgers N, et al. Discovery of pre-therapy 2-deoxy-2-(18)F-fluoro-D-glucose positron emission tomography-based radiomics classifiers of survival outcome in non-small-cell lung cancer patients. Eur J Nucl Med Mol Imaging. (2019) 46:455–66. doi: 10.1007/s00259-018-4139-4
18. Henter JI, Horne A, Arico M, Egeler RM, Filipovich AH, Imashuku S, et al. HLH-2004: diagnostic and therapeutic guidelines for hemophagocytic lymphohistiocytosis. Pediatr Blood Cancer. (2007) 48:124–31. doi: 10.1002/pbc.21039
19. Kikinis R, Pieper SD, Vosburgh KG. 3D Slicer: a platform for subject-specific image analysis, visualization, and clinical support. In: Jolesz FA, editor. Intraoperative Imaging and Image-Guided Therapy. New York, NY: Springer New York (2014). p. 277-89. doi: 10.1007/978-1-4614-7657-3_19
20. van Griethuysen JJM, Fedorov A, Parmar C, Hosny A, Aucoin N, Narayan V, et al. Computational radiomics system to decode the radiographic phenotype. Cancer Res. (2017) 77:e104–e7. doi: 10.1158/0008-5472.CAN-17-0339
21. Liu J, Yang X, Yang J. Prognosis predicting value of semiquantitative parameters of visceral adipose tissue and subcutaneous adipose tissue of (18)F-FDG PET/CT in newly diagnosed secondary hemophagocytic lymphohistiocytosis. Ann Nucl Med. (2021) 35:386–96. doi: 10.1007/s12149-021-01577-9
22. Yang YQ, Ding CY, Xu J, Fan L, Wang L, Tian T, et al. Exploring the role of bone marrow increased FDG uptake on PET/CT in patients with lymphoma-associated hemophagocytic lymphohistiocytosis: a reflection of bone marrow involvement or cytokine storm? Leuk Lymphoma. (2016) 57:291–8. doi: 10.3109/10428194.2015.1048442
23. Bronte V, Pittet MJ. The spleen in local and systemic regulation of immunity. Immunity. (2013) 39:806–18. doi: 10.1016/j.immuni.2013.10.010
24. Pijl JP, Kwee TC, Slart R, Yakar D, Wouthuyzen-Bakker M, Glaudemans A. Clinical implications of increased uptake in bone marrow and spleen on FDG-PET in patients with bacteremia. Eur J Nucl Med Mol Imaging. (2020) 48:1467–77. doi: 10.1007/s00259-020-05071-8
25. Kalkanis A, Kalkanis D, Drougas D, Vavougios GD, Datseris I, Judson MA, et al. Correlation of spleen metabolism assessed by 18F-FDG PET with serum interleukin-2 receptor levels and other biomarkers in patients with untreated sarcoidosis. Nucl Med Commun. (2016) 37:273–7. doi: 10.1097/MNM.0000000000000431
26. Ahn SS, Hwang SH, Jung SM, Lee SW, Park YB, Yun M, et al. Evaluation of spleen glucose metabolism using (18)F-FDG PET/CT in patients with febrile autoimmune disease. J Nucl Med. (2017) 58:507–13. doi: 10.2967/jnumed.116.180729
27. Wei J, Jiang H, Gu D, Niu M, Fu F, Han Y, et al. Radiomics in liver diseases: current progress and future opportunities. Liver Int. (2020) 40:2050–63. doi: 10.1111/liv.14555
28. Yin G, Man C, Liao S, Qiu H. The prognosis role of AST/ALT (De Ritis) ratio in patients with adult secondary hemophagocytic lymphohistiocytosis. Mediators Inflamm. (2020) 2020:5719751. doi: 10.1155/2020/5719751
29. Chaddad A, Daniel P, Niazi T. Radiomics evaluation of histological heterogeneity using multiscale textures derived from 3D wavelet transformation of multispectral images. Front Oncol. (2018) 8:96. doi: 10.3389/fonc.2018.00096
30. Jiang Y, Yuan Q, Lv W, Xi S, Huang W, Sun Z, et al. Radiomic signature of (18)F fluorodeoxyglucose PET/CT for prediction of gastric cancer survival and chemotherapeutic benefits. Theranostics. (2018) 8:5915–28. doi: 10.7150/thno.28018
31. Mayerhoefer ME, Materka A, Langs G, Häggström I, Szczypiński P, Gibbs P, et al. Introduction to radiomics. J Nucl Med. (2020) 61:488–95. doi: 10.2967/jnumed.118.222893
32. Lv W, Yuan Q, Wang Q, Ma J, Feng Q, Chen W, et al. Radiomics analysis of PET and CT components of PET/CT imaging integrated with clinical parameters: application to prognosis for nasopharyngeal carcinoma. Mol Imaging Biol. (2019) 21:954–64. doi: 10.1007/s11307-018-01304-3
33. Lue KH, Wu YF, Liu SH, Hsieh TC, Chuang KS, Lin HH, et al. Prognostic value of pretreatment radiomic features of 18F-FDG PET in patients with hodgkin lymphoma. Clin Nucl Med. (2019) 44:e559–e65. doi: 10.1097/RLU.0000000000002732
34. Li B, Guo J, Li T, Gu J, Zeng C, Xiao M, et al. Clinical characteristics of hemophagocytic lymphohistiocytosis associated with non-Hodgkin B-cell lymphoma: a multicenter retrospective study. Clin Lymphoma Myeloma Leuk. (2021) 21:e198–205. doi: 10.1016/S2152-2650(21)02106-6
35. Jin Z, Wang Y, Wei N, Wang Z. Adult primary hemophagocytic lymphohistocytosis associated with lymphoma. Ann Hematol. (2020) 99:663–5. doi: 10.1007/s00277-020-03924-8
36. Ghosh S, Köstel Bal S, Edwards ESJ, Pillay B, Jiménez Heredia R, Erol Cipe F, et al. Extended clinical and immunological phenotype and transplant outcome in CD27 and CD70 deficiency. Blood. (2020) 136:2638–55. doi: 10.1182/blood.2020006738
37. Lu J, Fang Q, Ma C, Su F, Chen G, Huang M, et al. Atypical Epstein-Barr virus-associated hemophagocytic lymphohistiocytosis simulating lymphadenitis on (18)F-FDG PET/CT and its differential diagnosis. Hell J Nucl Med. (2017) 20:254–7. doi: 10.1967/s002449910612
38. Hao R, Yang X, Liu Z, Yang J. EBV-Associated T-cell lymphoproliferative disorders demonstrated on FDG PET/CT in a patient with hemophagocytic lymphohistiocytosis. Clin Nucl Med. (2019) 44:829–30. doi: 10.1097/RLU.0000000000002649
39. Pan Q, Luo Y, Wu H, Ma Y, Li F. Epstein-Barr Virus-associated hemophagocytic lymphohistiocytosis mimicking lymphoma on FDG PET/CT. Clin Nucl Med. (2018) 43:125–7. doi: 10.1097/RLU.0000000000001923
40. Thomas DL, Syrbu S, Graham MM. Epstein-Barr virus mimicking lymphoma on FDG-PET/CT. Clin Nucl Med. (2009) 34:891–3. doi: 10.1097/RLU.0b013e3181bed135
41. Suga K, Kawakami Y, Hiyama A, Matsunaga N, Imoto S, Fukuda N, et al. F-18 FDG PET/CT findings in a case of T-cell lymphoma-associated hemophagocytic syndrome with liver involvement. Clin Nucl Med. (2010) 35:116–20. doi: 10.1097/RLU.0b013e3181c7bf20
42. Harada S, Shinohara T, Naruse K, Machida H. Diffuse 18F-fluorodeoxyglucose accumulation in the bone marrow of a patient with haemophagocytic lymphohistiocytosis due to Hodgkin lymphoma. BMJ Case Rep. (2016) 2016:bcr2016217555. doi: 10.1136/bcr-2016-217555
43. Donald JS, Barnthouse N, Chen DL. Rare variant of intravascular large B-cell lymphoma with hemophagocytic syndrome. Clin Nucl Med. (2018) 43:e125–e6. doi: 10.1097/RLU.0000000000001969
44. Otrock ZK, Eby CS. Clinical characteristics, prognostic factors, and outcomes of adult patients with hemophagocytic lymphohistiocytosis. Am J Hematol. (2015) 90:220–4. doi: 10.1002/ajh.23911
45. Wang J, Wang D, Zhang Q, Duan L, Tian T, Zhang X, et al. The significance of pre-therapeutic F-18-FDG PET-CT in lymphoma-associated hemophagocytic lymphohistiocytosis when pathological evidence is unavailable. J Cancer Res Clin Oncol. (2016) 142:859–71. doi: 10.1007/s00432-015-2094-z
46. Tang Y, Tan H, Hu S. Is there any potential of FDG PET/CT in monitoring disease activity in familial hemophagocytic lymphohistiocytosis? Clin Nucl Med. (2018) 43:296–8. doi: 10.1097/RLU.0000000000002008
47. Jaffe ES. The 2008 WHO classification of lymphomas: implications for clinical practice and translational research. Hematology Am Soc Hematol Educ Program. (2009) 2009:523–31. doi: 10.1182/asheducation-2009.1.523
48. Ishii E, Ohga S, Imashuku S, Yasukawa M, Tsuda H, Miura I, et al. Nationwide survey of hemophagocytic lymphohistiocytosis in Japan. Int J Hematol. (2007) 86:58–65. doi: 10.1532/IJH97.07012
49. Pan H, Huo Y, Sun L. Comparison between clinical features and prognosis of malignancy- and non-malignancy-associated pediatric hemophagocytic lymphohistiocytosis. BMC Pediatr. (2019) 19:468. doi: 10.1186/s12887-019-1702-5
50. Marnell L, Mold C, Du Clos TW. C-reactive protein: ligands, receptors and role in inflammation. Clin Immunol. (2005) 117:104–11. doi: 10.1016/j.clim.2005.08.004
Keywords: hemophagocytic lymphohistiocytosis, radiomics, prognosis, 18F-FDG PET/CT, nomogram
Citation: Yang X, Liu J, Lu X, Kan Y, Wang W, Zhang S, Liu L, Zhang H, Li J and Yang J (2021) Development and Validation of a Nomogram Based on 18F-FDG PET/CT Radiomics to Predict the Overall Survival in Adult Hemophagocytic Lymphohistiocytosis. Front. Med. 8:792677. doi: 10.3389/fmed.2021.792677
Received: 11 October 2021; Accepted: 22 November 2021;
Published: 22 December 2021.
Edited by:
Xiaoli Lan, Huazhong University of Science and Technology, ChinaReviewed by:
Alberto Miceli, Università di Genova, ItalyCopyright © 2021 Yang, Liu, Lu, Kan, Wang, Zhang, Liu, Zhang, Li and Yang. This is an open-access article distributed under the terms of the Creative Commons Attribution License (CC BY). The use, distribution or reproduction in other forums is permitted, provided the original author(s) and the copyright owner(s) are credited and that the original publication in this journal is cited, in accordance with accepted academic practice. No use, distribution or reproduction is permitted which does not comply with these terms.
*Correspondence: Jigang Yang, eWFuZ2ppZ2FuZ0BjY211LmVkdS5jbg==; Jixia Li, ai5saUBhdWNrbGFuZC5hYy5ueg==
Disclaimer: All claims expressed in this article are solely those of the authors and do not necessarily represent those of their affiliated organizations, or those of the publisher, the editors and the reviewers. Any product that may be evaluated in this article or claim that may be made by its manufacturer is not guaranteed or endorsed by the publisher.
Research integrity at Frontiers
Learn more about the work of our research integrity team to safeguard the quality of each article we publish.