- 1Translational Cardiology, Center for Molecular Medicine, Karolinska Institutet, Stockholm, Sweden
- 2Department of Medicine Solna, Karolinska Institutet, Stockholm, Sweden
- 3INSERM, U1059, SAINBIOSE, Université de Lyon, Université Jean-Monnet, Saint-Etienne, France
- 4Department of Clinical and Exercise Physiology, University Hospital of Saint-Etienne, Saint-Etienne, France
- 5Department of Cardiology, Karolinska University Hospital, Stockholm, Sweden
- 6Department of Neurobiology, Care Sciences and Society, Karolinska Institutet, Stockholm, Sweden
- 7Women's Health and Allied Health Professionals Theme, Medical Unit Occupational Therapy and Physiotherapy, Karolinska University Hospital, Stockholm, Sweden
- 8Rheumatology, Inflammation and Ageing Theme, Karolinska University Hospital, Stockholm, Sweden
- 9Department of Medicine Solna, Division of Rheumatology, Karolinska Institutet, Stockholm, Sweden
Background: Chronic inflammation leads to autonomic dysfunction, which may contribute to the increased risk of cardiovascular diseases (CVD) in patients with rheumatoid arthritis (RA). Exercise is known to restore autonomic nervous system (ANS) activity and particularly its parasympathetic component. A practical clinical tool to assess autonomic function, and in particular parasympathetic tone, is heart rate recovery (HRR). The aim of this substudy from the prospective PARA 2010 study was to determine changes in HRR post-maximal exercise electrocardiogram (ECG) after a 2-year physical activity program and to determine the main predictive factors associated with effects on HRR in RA.
Methods: Twenty-five participants performed physiotherapist-guided aerobic and muscle-strengthening exercises for 1 year and were instructed to continue the unsupervised physical activity program autonomously in the next year. All participants were examined at baseline and at years 1 and 2 with a maximal exercise ECG on a cycle ergometer. HRR was measured at 1, 2, 3, 4, and 5 min following peak heart rate during exercise. Machine-learning algorithms with the elastic net linear regression models were performed to predict changes in HRR1 and HRR2 at 1 year and 2 years of the PARA program.
Results: Mean age was 60 years, range of 41–73 years (88% women). Both HRR1 and HRR2 increased significantly from baseline to year 1 with guided physical activity and decreased significantly from year 1 to year 2 with unsupervised physical activity. Blood pressure response to exercise, low BMI, and muscular strength were the best predictors of HRR1/HRR2 increase during the first year and HRR1/HRR2 decrease during the second year of the PARA program.
Conclusion: ANS activity in RA assessed by HRR was improved by guided physical activity, and machine learning allowed to identify predictors of the HRR response at the different time points. HRR could be a relevant marker of the effectiveness of physical activity recommended in patients with RA at high risk of CVD. Very inactive and/or high CVD risk RA patients may get substantial benefits from a physical activity program.
Background
The autonomic nervous system (ANS) plays a major role in cardiac and vascular adaptations to environmental stress and is thus a major health marker for the effects of aging and cardiovascular diseases (CVD) (1). Autonomic dysfunction, i.e., increased activity of sympathetic tone and/or less parasympathetic tone at rest is associated with chronic inflammation (2). Rheumatoid arthritis (RA) is the most common inflammatory joint disease affecting 1–2% of the population worldwide (3). Patients with RA exhibit signs of autonomic dysfunction (4, 5), which may contribute to the established vascular (6, 7) and myocardial (3, 8, 9) affection and increased risk of CVD in patients with RA (10–12).
Even if little is known yet about the mechanisms that associate (i) autonomic dysfunction, (ii) chronic inflammation, and (iii) CVD risk in patients with RA, regular physical activity counterbalances these three entities (3, 13). Physical activity is known to restore ANS activity, and particularly its parasympathetic component (14). Also, the vagus nerve, which represents parasympathetic activity, plays a key role in inflammation by regulating the production of cytokines and decreasing circulating inflammatory markers (13). Finally, the increase of parasympathetic activity has been shown to be a strong protective factor against cardiovascular events (1).
A practical clinical tool to assess autonomic function, and in particular parasympathetic tone, is heart rate recovery (HRR) (13, 15, 16). The decrease in heart rate (HR) immediately following a maximal exercise electrocardiogram (ECG) represent reactivation of the parasympathetic tone (17). Vagal reactivation plays an integral part in reducing HR after exercise, especially during the first 2 min (18). A reduced HRR (an indicator of autonomic dysfunction) has been associated with increased risks of both cardiac and all-cause mortality, whereas rapid HR recovery immediately after exercise is associated with a lower risk of CVD and CVD events (17, 19).
Even though autonomic activity has been explored in RA (20) and after an exercise ECG (21, 22), to our knowledge, no study has assessed HRR post-maximal exercise ECG following physical activity intervention in RA. Therefore, the aim of this work was to establish the effects of a 2-year physical activity program (23) on autonomic function in RA, assessed by HRR post-maximal exercise ECG, and to determine the main predictive factors associated with HRR in RA using machine learning algorithms.
Methods
Study Population
This is a substudy from the prospective PARA 2010 study (Trial registration number: ISRCTN255 39102) (23). Inclusion criteria were RA according to the American College of Rheumatology, without a history of CVD. For this substudy, 25 PARA 2010 participants were included. These inactive participants, since they did not meet the WHO guidelines on physical activity (24), benefited from a 2-year real-life intervention program. The study protocol was approved by the Stockholm regional ethics committee (reference number 2012/769-32).
Disease Activity
Disease activity score (DAS28) was measured at baseline from the erythrocyte sedimentation rate (ESR) level, the number of swollen (0–28) and tender joints (0–28), and self-reported general health perception (visual analog scale, VAS 0–10 mm). The DAS28 was scored 0–10 with scores below 3.2 indicating low disease activity and those above 5.1 as high (23). RA activity was also assessed from treatment: disease-modifying antirheumatic drugs (DMARDs), non-steroidal anti-inflammatory drugs, and cortisone. They were recommended to maintain their course of medical treatment during the study.
Physical Activity Support Program
During the first year, each participant was encouraged to take part in at least two weekly 45 min circuit training sessions (aerobic exercise and muscle strength) with a physiotherapy coach and to perform moderate-to-vigorous physical activity (MVPA) at least 30 min on most days of the week (MVPA ≥ 240 min/week, i.e., ≥12 MET-h/week) using a pedometer for monitoring their number of steps. Physiotherapy coaches initially instructed them on the desired performance of exercises and MVPA and then guided them into support group meetings 1 h every other week to facilitate learning of specific behavioral skills to enable incorporation of physical activity sessions in real life, according to social cognitive theory. Alternative types of physical activity, individually or together with group peers, were encouraged. To prevent relapse during holidays, challenge competitions were organized where participants reported their physical activity and could win simple prizes. Furthermore, they were provided with short message service (SMS) and weekly text messages to monitor and encourage their adherence to the program (23). During the second year, participants were expected to continue the program autonomously. They were encouraged to perform MVPA at least 30 min on most days of the week (MVPA ≥150 min/week, i.e., ≥7.5 MET-h/week) and to report adherence by SMS (23).
Resting ECG
A standard resting 12-lead ECG was performed after 10 min of rest. From this 10 s electrocardiographic recording, the duration of all normal sinus intervals (RR intervals) was measured using a digital compass application (Compass EP, EP studios, USA). ECGs with <10 normal intervals in a row were excluded. Two intervals following abnormal beats and intervals differing more than 20% from the preceding interval were also considered abnormal and were excluded (25).
For the assessment of ultrashort heart rate variability parameters as surrogates of longer recordings, the square root of the mean squared differences of successive RR intervals (RMSSD in ms) was considered reliable down to 10 s ECG recordings (26, 27).
Exercise ECG
A maximal exercise ECG on the cycle ergometer (Ergomedic, Monark, Sweden) was performed by an experienced operator to rule out cardiac ischemia before starting the program and to assess physical capacity at each visit (baseline, year 1, and year 2). A starting load of 50 W was used with a 20 W/min increase in intensity until exhaustion or any of the relative or absolute contraindications of ACSM's guidelines (28) were met. The results were expressed in “W” and in percent of the predicted value. Continuous ECG was recorded, and blood pressure monitoring was measured throughout the exercise and recovery periods.
Exercise ECG was considered as a maximal cardiac level if participants achieved 90% of their maximal predicted HR (220-age for men and 210-age for women). HR exercise deltas between HR at 2 min from exercise and at rest and between maximal and resting were measured (bpm). The criteria for a positive exercise ECG were previously defined (29, 30).
Systolic Blood Pressure
Resting blood pressure was measured manually by an experienced nurse using a random-zero sphygmomanometer when the participant was sitting on the cycle ergometer immediately before the exercise phase. Systolic blood pressure (SBP) was measured every 2 min during exercise, and at 2 min and 5 min recovery from exercise. Maximal SBP was the highest value achieved during the exercise ECG. SBP exercise deltas between SBP at 2 min from exercise and at rest and between maximal and resting were measured (mmHg). SBP recovery deltas between maximal and at 2- and 5-min recovery from exercise were also measured (mmHg).
Heart rate recovery was measured at 1, 2, 3, 4, and 5 min following peak HR during exercise. Peak HR was identified as the maximum HR during the exercise protocol. HRR 1 min (HRR1) was defined as the absolute change from peak HR to HR 1 min post peak HR (HRR1 = peak HR – HR at 1 min post peak HR) (15, 16, 31). Similarly, HRs of recovery at 2 min (HRR2), 3 min (HRR3), 4 min (HRR4), and 5 min (HRR5) were calculated as the absolute change from peak HR to HRs 2, 3, 4, and 5 min, respectively, post-peak HR (31).
Variables Measurements
The variables measurements were obtained at each visit (baseline, year 1, and year 2).
Anthropometric Measures
Body mass index (BMI) was calculated by dividing body weight in kilograms by the square of body height in meters.
Muscular Strength Performance Tests
Lower limb function was assessed with the timed stands test (TST), measuring the time in seconds required for 10 full stands from a sitting position in an armless chair.
Upper limb function was assessed with the Grippit® (AB Detektor, Göteborg Sweden), measuring the maximum and average (three measures) handgrip strength with an electronic dynamometer in Newton (N).
Cardiovascular Assessment
Resting blood pressure was measured after 5–10 min of rest in a seated position before the bicycle ergometer test with a sphygmomanometer and a stethoscope.
Pulse wave velocity (PWV) and aortic augmentation index (AIx) were measured at a constant room temperature and calculated using an oscillometric arteriograph (TensioMed, Budapest, Hungary). It has been described in detail in previous reports from PARA 2010 (6).
Transthoracic echocardiography examinations were performed using a commercially available ultrasound system (Vivid E9, GE Healthcare, Milwaukee, WI, USA). Standard two-dimensional and Doppler echocardiography was performed in keeping with EACVI/ASE recommendations (32). Digital loops were stored and analyzed offline by an experienced reader. Speckle-tracking echocardiography was used to measure LV global longitudinal strain using the apical four-, three-, and two-chamber views. Left atrial (LA) reservoir strain was used to represent atrial deformation in keeping with expert consensus (33).
Biomarkers
Plasma high-sensitivity C-reactive protein (hs-CRP) concentrations were measured by a particle-enhanced immunoturbidimetric method (Roche, France) with a measuring interval of 0.1–20 mg/ml. ESR was measured at baseline using the Westergren method in mm/h.
Questionnaires
A short version of the International Physical Activity Questionnaire (IPAQ) is a self-administered questionnaire collecting information about (i) MVPA in weekly metabolic equivalent of task-h (MET-h/week) and (ii) sedentary time (ST in h/d), undertaken over the past 7 days before the assessment (34). It was used to measure the physical activity of participants at baseline and at 1 and 2 years.
Quality of life was assessed with the EuroQol (EQ-5D 3L) visual analog scale (VAS), which measured health state the actual day with a line drawn from a box to the appropriate point on a vertical VAS from “worst imaginable health state” (= 0) to “best imaginable health state” (= 100) (23).
Statistical Analysis
Data were expressed as mean (± SD) and frequencies (%). To determine statistically significant changes during the 2-year study protocol, a repeated-measured ANOVA on ranks was used with follow-up post-hoc comparisons. Wilcoxon's paired t-test was conducted for MVPA and ST data at years 1 and 2. Spearman rank correlation analyses were used to explore the relationship between HRR1/HRR2 and the different variables measured at baseline (Spearman correlogram). Machine learning models fit by elastic net linear regression provided regularized (shrunken) variable estimates and eliminated some variables from the set of predictors. These models were applied to predict HRR evolutions post-maximal exercise ECG using baseline, year 1, and 2 predictors chosen from significant associations in univariate analyses (Spearman correlograms). Eight-fold repeated crossvalidation was also applied to assess the performance of the model and to build a generalizable model. The predictive factors (magnitude ß-coefficient ≠ 0) were selected from each model. Correlations were determined between HRR1 (Models A and C)/HRR2 (Models B, D, and E) and (1) general characteristics of the participants: sex, age, anthropometric measures (BMI); (2) anaerobic and aerobic performances: TST (s), handgrip average and max (N), maximal aerobic power (W) (with % of expected); (3) cardiac parameters from resting and exercise ECG: resting HR (bpm), RMSSD (ms), % of expected maximal HR, HRs from exercise, recovery delta values (bpm), and cardiac parameters from echocardiography: LV strain (%) and LA reservoir strain (%); (4) vascular parameters from physical examination and exercise ECG: resting and mean blood pressures (mmHg), SBPs from exercise and recovery delta values (mmHg) and vascular parameters from arteriograph: PWV (m.s−1) and AIx (%); (5) inflammation biomarkers with CRP (mg.L−1); and (6) quality of life with EQ5D (/100).
Statistical analyses were performed using R (R Development Core Team, 2020) and SAS JMP Pro (JMP®, Version 16.1.0. SAS Institute Inc., Cary, NC, 1989–2021), where p < 0.05 was considered statistically significant. All tests were two-sided.
Results
Participants Characteristics
At baseline, the mean age was 60 ± 9.8 (41–73) years and 22 were women (88%). The mean DAS28 was 3.1 ± 1.2. Eighteen (72%) participants were taking biological RA medications, sixteen (64%) other DMARDs, eight (32%) were using non-steroidal anti-inflammatory drugs, and five (20%) cortisone. The mean values of ESR and LVEF were within normal ranges: 17 ± 11 mm and 59 ± 4% respectively. Out of the 25 participants, 22 (88%, 19 women) took part in the supported physical activity program (mean MVPA = 16.6 ± 5.4 MET-h/week and mean ST = 5.7 ± 2.3 h/d) in the first year; and 20 (80%, 18 women) continued the program autonomously (mean MVPA = 11.63 ± 4.6 MET-h/week and mean ST = 5.5 ± 2.6 h/d) during the second year. Exercise ECGs were all negative. Table 1 displays the general characteristics of the study population at baseline and at each yearly follow-up.
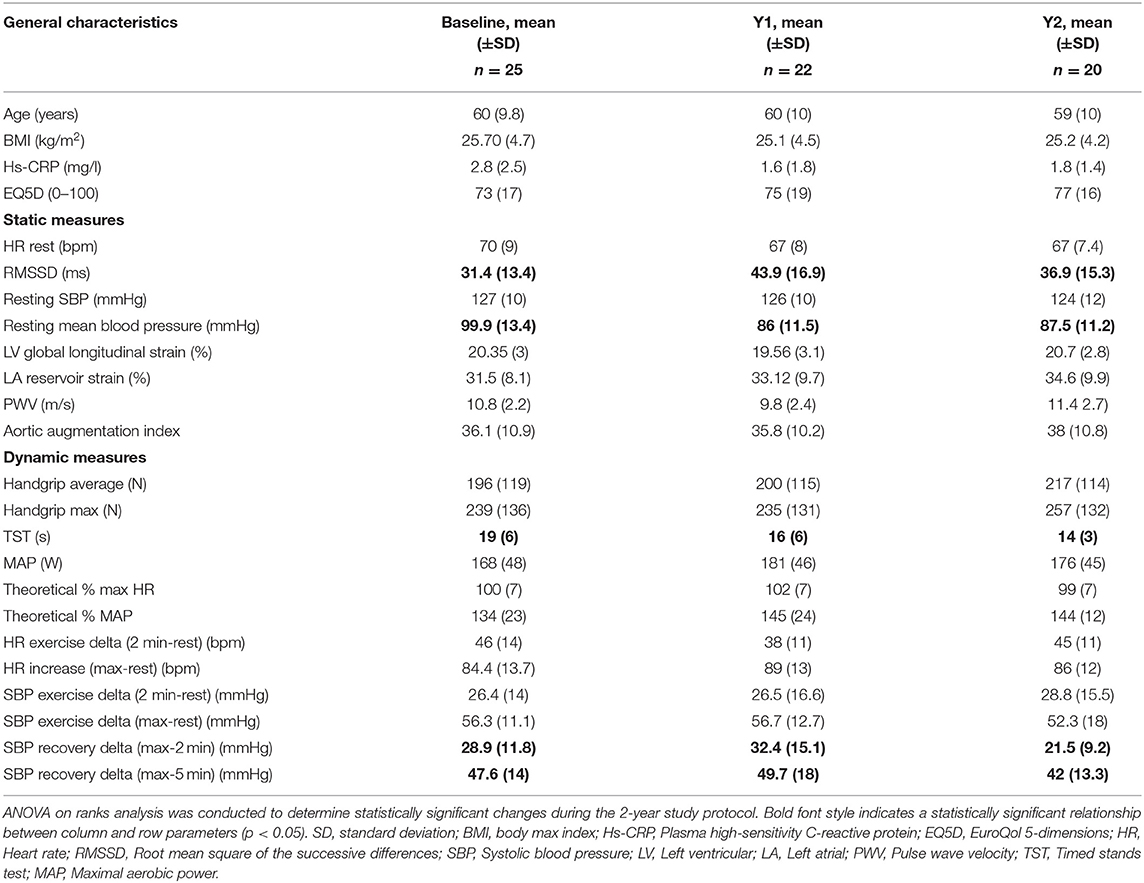
Table 1. General characteristics, static, and dynamic measures of the study cohort at baseline and yearly follow-ups.
A statistically significant increase was observed in the first year (compared to baseline), then a statistically significant decrease was observed in the second year (compared to year 1) for HRR1 (p = 0.02 and p = 0.01), HRR2 (p = 0.03) (Table 2; Figure 1; Supplementary Figure 1), RMSSD (p = 0.03 and p = 0.04), SBP recovery index (p = 0.01 for 2- and 5-min recovery from exercise) (Table 1; Supplementary Figure 2) and MVPA (p = 0.04 between years 1 and 2).
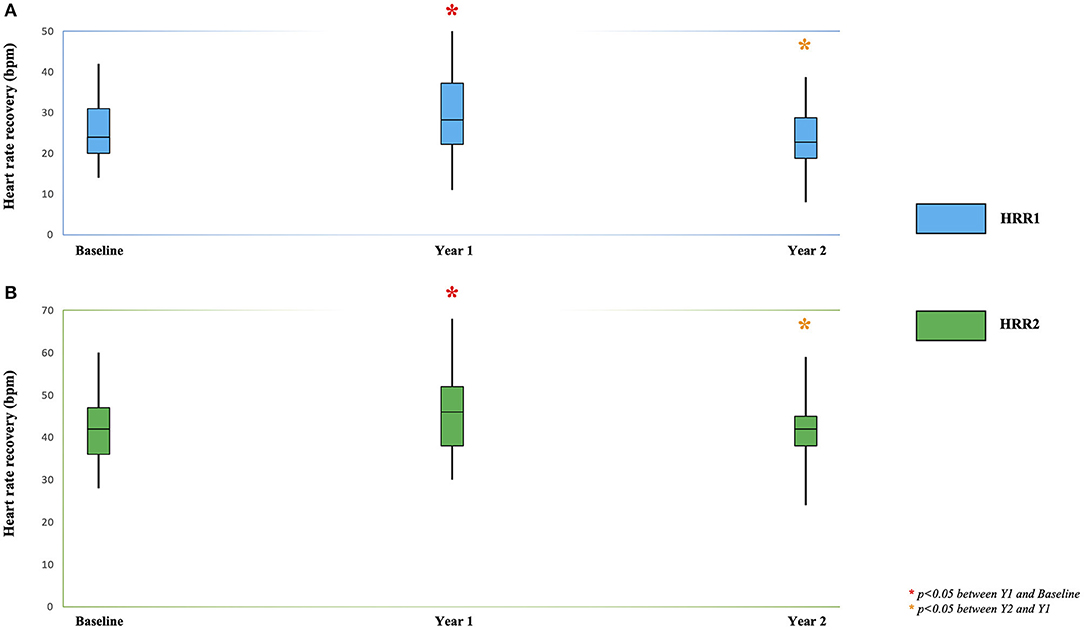
Figure 1. Heart rate recovery at 1 min: HRR1 (A) and 2 min: HRR2 (B) during the 2-year physical activity program in participants with RA. ANOVA on ranks analysis was conducted to determine statistically significant changes during the 2-year study protocol. *p < 0.05 between Y1 and Baseline. *p < 0.05 between Y2 and Y1.
A significant improvement in resting mean blood pressure (p = 0.03) and TST (p = 0.04) was observed over the entire study period. All these associations remained significant even after adjusting for the non-sphericity of the data (Table 1).
Predictions
In order to predict (1) the significant increase of HRR1 and HRR2 at 1 year (from baseline) and (2) the significant decrease of HRR1 and HRR2 at 2 years (from year 1) of the PARA program (Table 2; Figure 1; Supplementary Figure 1), the most clinically relevant variables were subsequently entered in elastic net linear regression models (Table 3; Supplementary Figure 3).
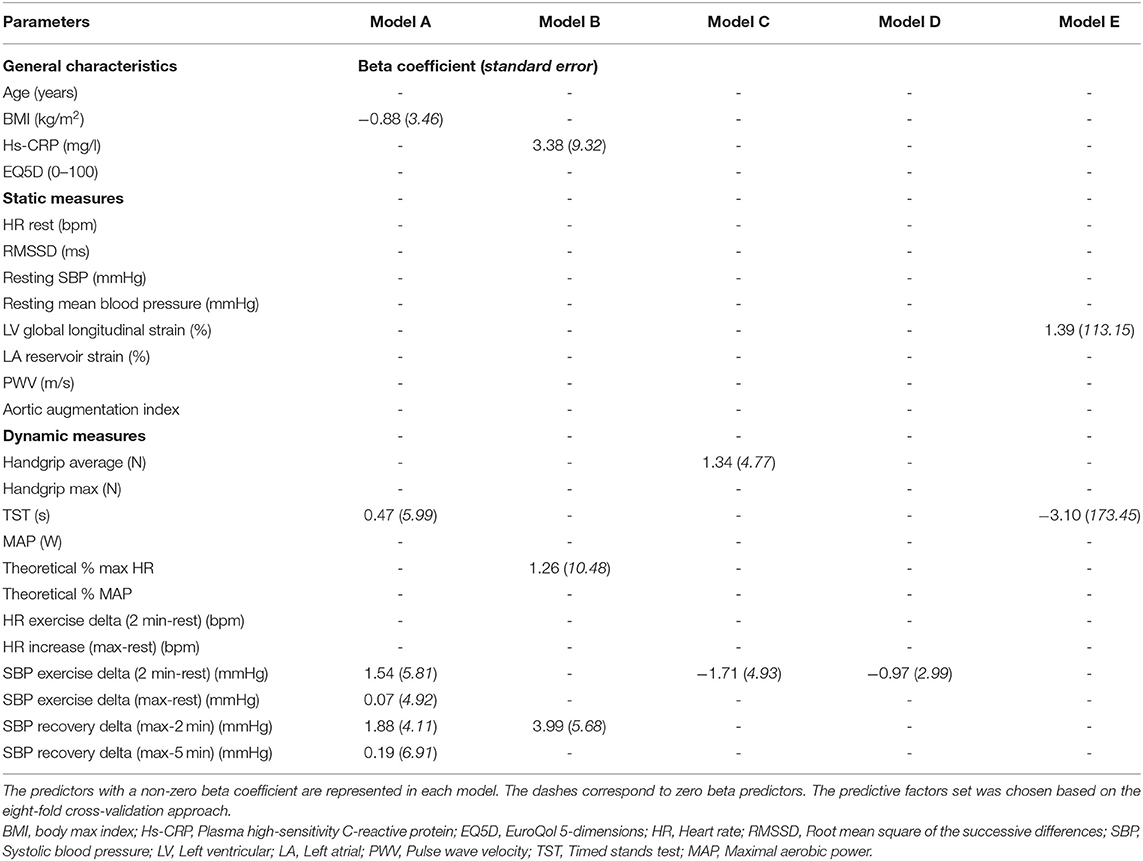
Table 3. The results of five elastic net linear regression models applied to predict heart rate recovery (HRR) 1 and 2 increases (Models A and B) and decreases (Models C, D, and E) post-maximal exercise ECG using the baseline, year 1, and year 2 predictors as independent variables.
Five models were built for predicting five types of outcomes: (1)- the increase of HRR1 (Model A) and (2)- HRR2 (Model B) during the first year of the program, from predictors obtained at baseline, (3)- the decrease of HRR1 (Model C), and (4)- HRR2 (Model D) during the second year of the program, from predictors obtained at year 1 and year 2 for HRR2 (Model E). These five elastic net regression models were retained in a total of 10 of 24 candidate predictors for HRR changes (Table 3; Figure 2; Supplementary Figure 4).
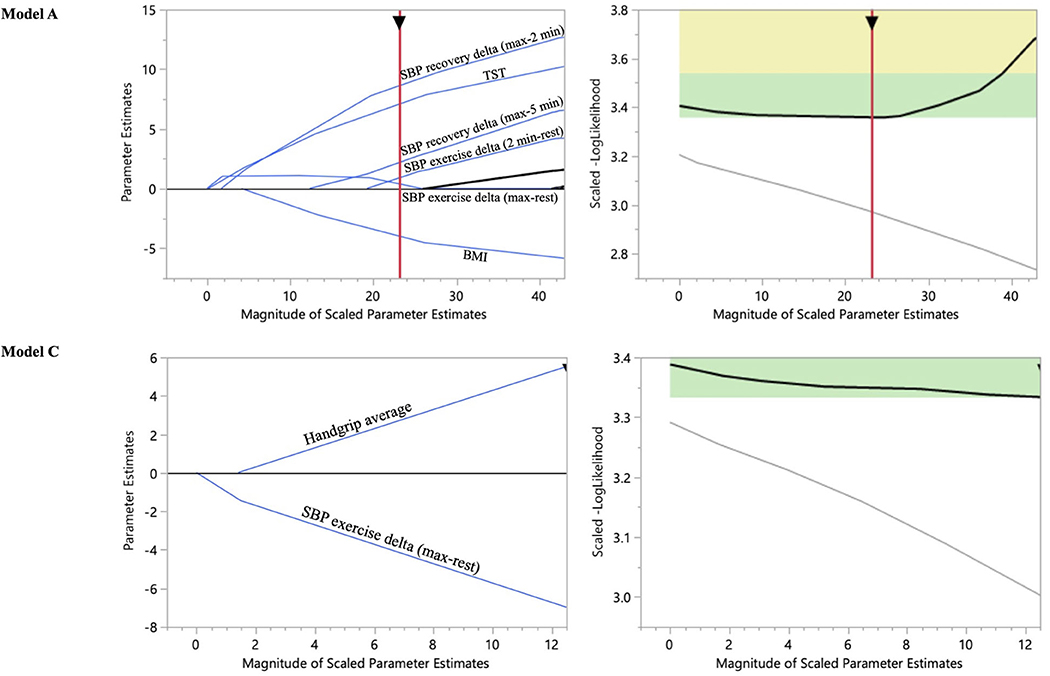
Figure 2. Solution path trace plot applied to predict HRR1 increase and decrease post-maximal exercise ECG using the baseline and year 1 predictors as independent variables: Models A and C. Each line corresponds to a predictor variable. As regularization is applied to the model (from right to left in the trace plot), less important coefficients get smaller, and some are completely removed from the model (the ones that have a beta coefficient of 0 and where the line for the predictor intersects with the x-axis before the optimal solution is reached). A generalizable model was built with an eight-fold cross validation to determine the model which provides a global minimum (within the green rectangle) for the scaled-log likelihood value among the validation folds.
The SBP response to exercise at baseline was the best predictor for an increase of HRR1 (Model A) and HRR2 (Model B). Indeed, SBP recovery at 2 min was positively correlated with HRR1 (Model A: ß-coefficient = 1.88) and HRR2 (Model B: ß-coefficient = 3.99) increase (Table 3; Figure 2; Supplementary Figure 4).
For the changes at year 1, SBP increase at baseline exercise, lower BMI, and higher TST were the other predictive covariates of HRR1 increase (Model A: ß-coefficient = 1.54, −0.88, and 0.47 respectively) (Table 3; Figure 2). Hs-CRP and % theoretical max were the other predictive covariates of HRR2 increase (Model B: ß-coefficient = 3.38 and 1.26, respectively) (Table 3; Supplementary Figure 4).
For the changes at year 2, SBP response to exercise at year 1 was the best predictor of HRR1 (Model C) and HRR2 (Model D) decrease (Table 3; Figure 2; Supplementary Figure 4). Indeed, the SBP exercise delta (at year 1) was negatively correlated with HRR1 (Model C: ß-coefficient = −1.71) and HRR2 (Model D: ß-coefficient = −0.96) decrease (at year 2) (Table 3; Figure 2; Supplementary Figure 4). Handgrip was the only other covariate of HRR1 decrease (Model C: ß-coefficient = 1.34) (Table 3; Figure 2). TST and LV strain were the predictors at year 2 of HRR2 decrease during the second part of the program (Model E: ß-coefficient = −3.1 and 1.39, respectively) (Table 3; Supplementary Figure 4).
Discussion
The results of this study identified a parallel relation between the changes in levels of physical activity with ANS activity in RA. In particular, a significant improvement after 1 year of supervised physical activity and a decrease toward baseline during the following year with less intense physical activity is noted, as illustrated in Supplementary Figure 5. Integrating all plausible factors affecting ANS activity, the present machine learning elastic net regression models identified BMI, CRP, and TST as well as HR and SBP responses to exercise as baseline predictors of the exercise associated ANS improvement in RA (Supplementary Figure 5). Taken together, these findings extend the well-established positive effects of exercise in RA to measures and predictors of ANS, with potential clinical implications for CVD risk.
The first 2 min of HRR is validated and commonly used ANS measures (31). A slow decrease of HRR after exercise indicates autonomic dysfunction or at least a non-optimal ANS and is an independent predictor of CVD and all-cause mortality (18). Also, an impaired HRR from the first minute after exercise ECG is a predictor of overall mortality (19, 35). In this study, the HRRs 1–5 were largely above the normal cut-off of 12 beats per minute (17), but lower than the HRR observed in larger population-based cohorts (36). The absolute HRR 1 and 2 in this work were similar to previous work in patients with RA after maximal treadmill testing (20) supporting an autonomic dysfunction in RA. Cardiac rehabilitation increases HRR, which is associated with improved outcomes (37). The reversibility of the autonomic dysfunction in RA has hitherto remained unexplored. Therefore, we next assessed HRR after a 2-year physical activity program in RA participants (20) to establish the possibility to improve HRR and to determine the main predictive factors associated with HRR in RA.
Importantly, an improved ANS function in RA after the first year of supervised physical activity was demonstrated in this study by significant increases of HRR at 1 and 2 min after maximal exercise. Beneficial cardiovascular effects of exercise interventions in RA are well-established (6, 8–11, 20), and our results extend these findings to a potential link via the enhancement of parasympathetic modulation, which was further supported by the significant increase in RMSSD in the present study.
Elastic regression modeling with eight-fold cross validation allowed us to identify blood pressure response to exercise, lower BMI, and higher muscular strength at baseline as predictors of HRR increase during the first year of the PARA 2010 physical activity program. These findings point to the possibility to identify responders and non-responders to physical activity intervention programs in RA.
Both the SBP increase at exercise and the decrease at recovery predicted the HRR increase in response to the PARA2010 intervention in RA. Previous studies have established a relationship between HRR and SBP, but provided contradictory conclusions if SBP changes during exercise are a consequence or cause of the observed HRR (38–42). In this study, SBP recovery followed the pattern of HRR and increased in the first year. The rise and recovery of SBP with exercise is predominantly caused by an increase in cardiac output, being dependent on HR and systolic function (28, 42), which was normal in this study. In RA, increased arterial stiffness (7, 43), diastolic dysfunction (9), and a non-optimal ventricular-arterial coupling (6) may affect the SBP increase and recovery after exercise (28, 44). In addition to SBP responses, positive predictive factors for improved HRR by exercise intervention identified by elastic net regression analysis included also strength and a low BMI at baseline. These observations indicate conditions giving the optimal conditions for improving ANS function by exercise. In contrast, higher CRP predicted an increase in HRR. Exposure to inflammation affects the cardiovascular system, as demonstrated by the disease duration as a major determinant of vascular function in RA (7). There is, in addition, a close relation and possible interregulation between inflammation and ANS activity. The observation that participants with higher CRP exhibited a larger improvement in HRR supports that inflammatory conditions indicate a larger beneficial potential of physical activity.
The observed ANS improvement at year 1 was not preserved over time since HRR was significantly decreased at year 2 compared with year 1 in this study, despite a level of physical activity that continued to overreach the WHO recommendations (>2x in the first year and 1.5x in the second year) (24). These results suggested a high efficient threshold of exercise training to obtain an adapted autonomic response, potentially optimizing the cardiovascular response and improving cardiovascular outcomes. The slightest decrease in physical activity (even over physical activity recommendations) leads to a sympathovagal balance shifting toward less parasympathetic activity. Finally, assessment of the last available cardiovascular characteristics identified a negative association of HRR increase with TST decrease and a positive association with normal-to-high values of LV strain at year 2. Thus, a substantial gain of muscular strength (lower limb with TST and upper limb with handgrip) and a normal LV systolic deformation (absence of subclinical cardiac dysfunction) predicted a better response to exercise after the 2-year physical activity program. Both are strong cardiovascular prognostic factors (9, 45–47), associated with a reduction in resting blood pressure (48, 49). In addition to being significantly correlated at baseline, the significant correlations between autonomic and vascular functions at 1 and 2 years indicate that these two variables are closely related to each other and maybe part of the indirect effects of physical activity increase during the first year and physical activity decrease in the second year.
The bidirectional changes in HRR over increasing and decreasing physical activity may be used to follow a physical activity intervention in RA to identify non-responder and non-adherent participants with potential hypertension risk, despite normal resting blood pressure and normal exercise ECG findings. From a practical standpoint, exercising ECG and extending it for only several minutes into exercise recovery provides a unique, minimally invasive means to assess HR and blood pressure responses to exercise to indirectly determine participant's response or adherence to physical activity and to predict the CVD risk (28, 50), and also mainly to detect hypertension early (39, 42, 51). It was already reported in the literature that a delayed SBP decrease post-exercise was more accurate than ST segment depression for the diagnosis of coronary artery disease (CAD) (39, 52, 53). Early identification of high cardiovascular risk could improve the effectiveness of prevention in RA by targeting patients who will benefit the most from lifestyle interventions (exercise, diet, and antihypertensive drugs). The present results suggest that ANS upregulation by a high (≥7.5 MET-h/week) and regular (≥5 days/7) MVPA training level may be key for successful aging. Obviously, the support group sessions according to social cognitive theory improved adherence of RA participants in the first year (supervised physical activity). The outcomes of this study could provide new insight into the relationship between SBP response to exercise and ANS modulation.
Major strengths of this study include the prospective longitudinal design with close follow-up of the participant's adherence to the physical activity program and with the repeated physiological parameter assessments (23). The longitudinal follow-up allowed to assess causality between physiological parameters according to the steps of the PARA cohort study, which are 0–1 year: whole program of physical activity; and 1–2 years: autonomously physical activity (23). No subject of the study had medication that could affect autonomic function (anticholinergics, cholinesterase antagonists, adrenoreceptor agonists, and adrenoreceptor antagonists). Most of the participants were able to perform the exercise ECG to their maximum cardiorespiratory ability, i.e., until exhaustion (the mean theoretical % of MAP was exceeded by 34–45% each time), despite problems with their joints, which could have influenced their HRR.
Certain limitations should also be acknowledged. First, the relatively small sample size is mainly made up of Swedish women (88%). It is the same population described in the two previous studies from the team, but four participants were excluded due to incomplete data concerning exercise ECG (6, 9). However, the use of a machine-learning algorithm with the elastic net linear regression models permits the identification of predictive factors among an important number of parameters (p) in relation to a small sample (n) of participants (p > n). Nested repeated crossvalidation was used for the models in the study, which provides an unbiased estimation of the performance of the prediction model (54). Compared with a classical linear regression method, the elastic net linear regression model increases the robustness, simplicity, and accuracy of such results in this context (55, 56). However, standard errors are large with the small sample. Second, the study did not have a comparison group, but the aim of the work was to examine the effects of a physical activity intervention on HRR in this population. Third, difficulties, even with experience, to define the values of diastolic blood pressure when it comes to defining or recognizing the fifth Korotkoff sound during exercise ECG (57). Also, a common measure of SBP recovery is defined in the literature as the ratio of the SBP obtained in the third minute of the recovery period to either the peak-exercise SBP or the SBP in the first minute of the recovery period after exercise ECG (39, 42, 58). Unfortunately, SBP was measured at 2 and 5 min recovery from exercise in the PARA study, which is generally performed in clinical research in practice (51, 57). Finally, as participants were tasked to report their level of physical activity, the reporting bias should be acknowledged even if it was the most validated physical activity questionnaire (59). Future studies should be to use objective methods such as accelerometry to assess physical activity, mainly when this activity is practiced in autonomy. A more precise evaluation of physical activity and biological data could determine if exercise leads to a sympathovagal balance shifting toward a more parasympathetic activity or less sympathetic activity (60).
Conclusion
Autonomic nervous system activity via HRR measurement can be a relevant marker of the effectiveness of physical activity recommended in patients with RA at high risk of CVD. Exercise ECG can serve as a simple minimally invasive means to assess blood pressure responses to exercise and recovery, which are the main predictive factors of HRR evolutions during a physical activity program. HRR and SBP recovery, associated with muscular strength performance tests, can mainly be used to assess sympathovagal balance and to quantify the degree of autonomic dysfunction in order to detect CVD risk early. These results can guide future recommendations for RA patients and may improve adherence to regular physical activity programs and thus their cardiovascular health.
Data Availability Statement
The original contributions presented in the study are included in the article/Supplementary Material, further inquiries can be directed to the corresponding author.
Ethics Statement
The studies involving human participants were reviewed and approved by the Stockholm Regional Ethics Committee (Reference Number 2012/769-32). The patients/participants provided their written informed consent to participate in this study.
Author Contributions
DH, PS, CO, IL, and MB: conception and design of the work, interpretation, and draft of the manuscript and substantial revision. DH, AV, CF, BN, and MB: acquisition. DH, PS, and MB: analysis. All authors have approved the manuscript.
Funding
PARA 2010 was supported by grants from AFA Insurance (Grant Number 100172), The Swedish Research Council, Combine Sweden, The Swedish Rheumatism Foundation, and The KI National Postgraduate School of Health Care Sciences. DH was supported by the Foundation for Geriatric Diseases at Karolinska Institutet (Grant Number FS-2021:0006), the Auvergne-Rhone-Alpes Region (Grant Number 20 006296 01), the GHT-Loire public hospital funds, and the European Society of Cardiology Council on Basic Cardiovascular Science (Grant Number 000085188), French Institute in Sweden (TOR scholarship SCIEN 23/21). PS was supported by the Clinical Scientist Training Programme (CSTP) at Karolinska Institutet. MB was supported by the Swedish Research Council (Grant Number 2019-01486), the Swedish Heart and Lung Foundation (Grant Number 20180571), and King Gustaf V and Queen Victoria Freemason Foundation. The funding sources had no role in the design and conduct of the study, collection, management, analysis, and interpretation of the data, preparation, review, or approval of the manuscript, and decision to submit the manuscript for publication.
Conflict of Interest
The authors declare that the research was conducted in the absence of any commercial or financial relationships that could be construed as a potential conflict of interest.
Publisher's Note
All claims expressed in this article are solely those of the authors and do not necessarily represent those of their affiliated organizations, or those of the publisher, the editors and the reviewers. Any product that may be evaluated in this article, or claim that may be made by its manufacturer, is not guaranteed or endorsed by the publisher.
Acknowledgments
The authors thank Eva Wallgren and Linda Nilson for excellent assistance with exercise testing, arteriographic measures, and echocardiography.
Supplementary Material
The Supplementary Material for this article can be found online at: https://www.frontiersin.org/articles/10.3389/fmed.2021.788243/full#supplementary-material
References
1. Raffin J, Barthélémy J-C, Dupré C, Pichot V, Berger M, Féasson L, et al. Exercise frequency determines heart rate variability gains in older people: a meta-analysis and meta-regression. Sports Med. (2019) 49:719–29. doi: 10.1007/s40279-019-01097-7
2. Lanza GA, Pitocco D, Navarese EP, Sestito A, Sgueglia GA, Manto A, et al. Association between cardiac autonomic dysfunction and inflammation in type 1 diabetic patients: effect of beta-blockade. Eur Heart J. (2007) 28:814–20. doi: 10.1093/eurheartj/ehm018
3. Chen J, Norling LV, Cooper D. Cardiac dysfunction in rheumatoid arthritis: the role of inflammation. Cells. (2021) 10:881. doi: 10.3390/cells10040881
4. Goldstein RS, Bruchfeld A, Yang L, Qureshi AR, Gallowitsch-Puerta M, Patel NB, et al. Cholinergic anti-inflammatory pathway activity and High Mobility Group Box-1 (HMGB1) serum levels in patients with rheumatoid arthritis. Mol Med. (2007) 13:210–5. doi: 10.2119/2006-00108
5. Adlan AM. Veldhuijzen van Zanten JJCS, Lip GYH, Paton JFR, Kitas GD, Fisher JP. Cardiovascular autonomic regulation, inflammation and pain in rheumatoid arthritis. Auton Neurosci. (2017) 208:137–45. doi: 10.1016/j.autneu.2017.09.003
6. Sarajlic P, Fridén C, Lund LH, Manouras A, Venkateshvaran A, Larsson SC, et al. Enhanced ventricular-arterial coupling during a 2-year physical activity programme in patients with rheumatoid arthritis: a prospective substudy of the physical activity in rheumatoid arthritis 2010 trial. J Intern Med. (2018) 284:664–73. doi: 10.1111/joim.12715
7. Vázquez-Del Mercado M, Gomez-Bañuelos E, Chavarria-Avila E, Cardona-Muñoz E, Ramos-Becerra C, Alanis-Sanchez A, et al. Disease duration of rheumatoid arthritis is a predictor of vascular stiffness: a cross-sectional study in patients without known cardiovascular comorbidities: a STROBE-compliant article. Medicine (Baltimore). (2017) 96:e7862. doi: 10.1097/MD.0000000000007862
8. Zegkos T, Kitas G, Dimitroulas T. Cardiovascular risk in rheumatoid arthritis: assessment, management and next steps. Ther Adv Musculoskelet Dis. (2016) 8:86–101. doi: 10.1177/1759720X16643340
9. Venkateshvaran A, Sarajlic P, Lund LH, Fridén C, Nordgren B, Opava CH, et al. Impaired left atrial dynamics and its improvement by guided physical activity reveal left atrial strain as a novel early indicator of reversible cardiac dysfunction in rheumatoid arthritis. Eur J Prev Cardiol. (2018) 25:1106–8. doi: 10.1177/2047487318777775
10. Jagpal A, Navarro-Millán I. Cardiovascular co-morbidity in patients with rheumatoid arthritis: a narrative review of risk factors, cardiovascular risk assessment and treatment. BMC Rheumatol. (2018) 2:10. doi: 10.1186/s41927-018-0014-y
11. Chodara AM, Wattiaux A, Bartels CM. Managing cardiovascular disease risk in rheumatoid arthritis: clinical updates and three strategic approaches. Curr Rheumatol Rep. (2017) 19:16. doi: 10.1007/s11926-017-0643-y
12. Baghdadi LR, Woodman RJ, Shanahan EM, Mangoni AA. The impact of traditional cardiovascular risk factors on cardiovascular outcomes in patients with rheumatoid arthritis: a systematic review and meta-analysis. PLoS ONE. (2015) 10:e0117952. doi: 10.1371/journal.pone.0117952
13. Peçanha T, Lima AHR de A. Inflammation and cardiovascular autonomic dysfunction in rheumatoid arthritis: a bidirectional pathway leading to cardiovascular disease. J Physiol. (2017) 595:1025–6. doi: 10.1113/JP273649
14. Hupin D, Edouard P, Gremeaux V, Garet M, Celle S, Pichot V, et al. Physical activity to reduce mortality risk. Eur Heart J. (2017) 38:1534–7. doi: 10.1093/eurheartj/ehx236
15. Arduini A, Gomez-Cabrera M-C, Romagnoli M. Reliability of different models to assess heart rate recovery after submaximal bicycle exercise. J Sci Med Sport. (2011) 14:352–7. doi: 10.1016/j.jsams.2011.02.012
16. Shetler K, Marcus R, Froelicher VF, Vora S, Kalisetti D, Prakash M, et al. Heart rate recovery: validation and methodologic issues. J Am Coll Cardiol. (2001) 38:1980–7. doi: 10.1016/S0735-1097(01)01652-7
17. Cole CR, Blackstone EH, Pashkow FJ, Snader CE, Lauer MS. Heart-rate recovery immediately after exercise as a predictor of mortality. N Engl J Med. (1999) 341:1351–7. doi: 10.1056/NEJM199910283411804
18. Lipinski MJ, Vetrovec GW, Froelicher VF. Importance of the first two minutes of heart rate recovery after exercise treadmill testing in predicting mortality and the presence of coronary artery disease in men. Am J Cardiol. (2004) 93:445–9. doi: 10.1016/j.amjcard.2003.10.039
19. Jouven X, Empana J-P, Schwartz PJ, Desnos M, Courbon D, Ducimetière P. Heart-rate profile during exercise as a predictor of sudden death. N Engl J Med. (2005) 352:1951–8. doi: 10.1056/NEJMoa043012
20. Osailan A, Metsios GS, Rouse PC, Ntoumanis N, Duda JL, Kitas GD, et al. Factors associated with parasympathetic activation following exercise in patients with rheumatoid arthritis: a cross-sectional study. BMC Cardiovasc Disord. (2016) 16:86. doi: 10.1186/s12872-016-0264-9
21. Sydó N, Sydó T, Gonzalez Carta KA, Hussain N, Farooq S, Murphy JG, et al. Prognostic performance of heart rate recovery on an exercise test in a primary prevention population. J. Am. Heart Assoc. 7:e008143. doi: 10.1161/JAHA.117.008143
22. Davrath LR, Akselrod S, Pinhas I, Toledo E, Beck A, Elian D, et al. Evaluation of autonomic function underlying slow postexercise heart rate recovery. Med Sci Sports Exerc. (2006) 38:2095–101. doi: 10.1249/01.mss.0000235360.24308.c7
23. Nordgren B, Fridén C, Demmelmaier I, Bergström G, Opava CH. Long-term health-enhancing physical activity in rheumatoid arthritis–the PARA 2010 study. BMC Public Health. (2012) 12:397. doi: 10.1186/1471-2458-12-397
24. Bull FC, Al-Ansari SS, Biddle S, Borodulin K, Buman MP, Cardon G, et al. World Health Organization 2020 guidelines on physical activity and sedentary behaviour. Br J Sports Med. (2020) 54:1451–62. doi: 10.1136/bjsports-2020-102955
25. Dekker JM, Schouten EG, Klootwijk P, Pool J, Swenne CA, Kromhout D. Heart rate variability from short electrocardiographic recordings predicts mortality from all causes in middle-aged and elderly men. The Zutphen Study. Am J Epidemiol. (1997) 145:899–908. doi: 10.1093/oxfordjournals.aje.a009049
26. Wehler D, Jelinek HF, Gronau A, Wessel N, Kraemer JF, Krones R, et al. Reliability of heart-rate-variability features derived from ultra-short ECG recordings and their validity in the assessment of cardiac autonomic neuropathy. Biomed Signal Process Control. (2021) 68:102651. doi: 10.1016/j.bspc.2021.102651
27. Munoz ML, van Roon A, Riese H, Thio C, Oostenbroek E, Westrik I, et al. Validity of ultra-short recordings for heart rate variability measurements. PLoS ONE. (2015) 10:e0138921. doi: 10.1371/journal.pone.0138921
28. Fletcher GF, Ades PA, Kligfield P, Arena R, Balady GJ, Bittner VA, et al. Exercise standards for testing and training: a scientific statement from the American Heart Association. Circulation. (2013) 128:873–934. doi: 10.1161/CIR.0b013e31829b5b44
29. Hupin D, Edouard P, Oriol M, Laukkanen J, Abraham P, Doutreleau S, et al. Exercise electrocardiogram in middle-aged and older leisure time sportsmen: 100 exercise tests would be enough to identify one silent myocardial ischemia at risk for cardiac event. Int J Cardiol. (2018) 257:16–23. doi: 10.1016/j.ijcard.2017.10.081
30. Puelacher C, Wagener M, Abächerli R, Honegger U, Lhasam N, Schaerli N, et al. Diagnostic value of ST-segment deviations during cardiac exercise stress testing: systematic comparison of different ECG leads and time-points. Int J Cardiol. (2017) 238:166–72. doi: 10.1016/j.ijcard.2017.02.079
31. Peçanha T, Bartels R, Brito LC, Paula-Ribeiro M, Oliveira RS, Goldberger JJ. Methods of assessment of the post-exercise cardiac autonomic recovery: a methodological review. Int J Cardiol. (2017) 227:795–802. doi: 10.1016/j.ijcard.2016.10.057
32. Lang RM, Bierig M, Devereux RB, Flachskampf FA, Foster E, Pellikka PA, et al. Recommendations for chamber quantification: a report from the American Society of Echocardiography's Guidelines and Standards Committee and the Chamber Quantification Writing Group, developed in conjunction with the European Association of Echocardiography, a branch of the European Society of Cardiology. J Am Soc Echocardiogr. (2005) 18:1440–63. doi: 10.1016/j.echo.2005.10.005
33. Badano LP, Kolias TJ, Muraru D, Abraham TP, Aurigemma G, Edvardsen T, et al. Standardization of left atrial, right ventricular, and right atrial deformation imaging using two-dimensional speckle tracking echocardiography: a consensus document of the EACVI/ASE/Industry Task Force to standardize deformation imaging. Eur Heart J Cardiovasc Imaging. (2018) 19:591–600. doi: 10.1093/ehjci/jey042
34. Craig CL, Marshall AL, Sjöström M, Bauman AE, Booth ML, Ainsworth BE, et al. International physical activity questionnaire: 12-country reliability and validity. Med Sci Sports Exerc. (2003) 35:1381–95. doi: 10.1249/01.MSS.0000078924.61453.FB
35. Maeder MT, Ammann P, Rickli H, Brunner-La Rocca HP. Impact of the exercise mode on heart rate recovery after maximal exercise. Eur J Appl Physiol. (2009) 105:247–55. doi: 10.1007/s00421-008-0896-2
36. Van de Vegte YJ, van der Harst P, Verweij N. Heart rate recovery 10 seconds after cessation of exercise predicts death. J Am Heart Assoc. (2018) 7:e008341. doi: 10.1161/JAHA.117.008341
37. Jolly MA, Brennan DM, Cho L. Impact of exercise on heart rate recovery. Circulation. (2011) 124:1520–6. doi: 10.1161/CIRCULATIONAHA.110.005009
38. Westover AN, Nakonezny PA, Barlow CE, Adinoff B, Brown ES, Halm EA, et al. Heart rate recovery and systolic blood pressure recovery after maximal exercise in prevalent users of stimulant medications. J Clin Psychopharmacol. (2016) 36:295–7. doi: 10.1097/JCP.0000000000000485
39. Alihanoglu YI, Yildiz BS, Kilic ID, Saricopur A, Oncu M, Buber I, et al. A new method for evaluation of the autonomic nervous system in patients with idiopathic hyperhidrosis: systolic blood pressure and heart rate recovery after graded exercise. Clin Exp Dermatol. (2016) 41:847–51. doi: 10.1111/ced.12915
40. Tanindi A, Ugurlu M, Tore HF. Blood pressure morning surge, exercise blood pressure response and autonomic nervous system. Scand Cardiovasc J. (2015) 49:220–7. doi: 10.3109/14017431.2015.1045934
41. Nieminen T, Leino J, Maanoja J, Nikus K, Viik J, Lehtimäki T, et al. The prognostic value of haemodynamic parameters in the recovery phase of an exercise test. The finnish cardiovascular study. J Hum Hypertens. (2008) 22:537–43. doi: 10.1038/jhh.2008.38
42. Ellis K, Pothier CE, Blackstone EH, Lauer MS. Is systolic blood pressure recovery after exercise a predictor of mortality? Am Heart J. (2004) 147:287–92. doi: 10.1016/j.ahj.2003.08.009
43. Van Hout MJ, Dekkers IA, Westenberg JJ, Schalij MJ, Widya RL, de Mutsert R, et al. Normal and reference values for cardiovascular magnetic resonance-based pulse wave velocity in the middle-aged general population. J Cardiovasc Magn Reson. (2021) 23:46. doi: 10.1186/s12968-021-00739-y
44. Mäki-Petäjä KM, Barrett SML, Evans SV, Cheriyan J, McEniery CM, Wilkinson IB. The role of the autonomic nervous system in the regulation of aortic stiffness. Hypertension. (2016) 68:1290–7. doi: 10.1161/HYPERTENSIONAHA.116.08035
45. Celis-Morales CA, Welsh P, Lyall DM, Steell L, Petermann F, Anderson J, et al. Associations of grip strength with cardiovascular, respiratory, and cancer outcomes and all cause mortality: prospective cohort study of half a million UK Biobank participants. BMJ. (2018) 361:k1651. doi: 10.1136/bmj.k1651
46. Argyridou S, Zaccardi F, Davies MJ, Khunti K, Yates T. Walking pace improves all-cause and cardiovascular mortality risk prediction: a UK Biobank prognostic study. Eur J Prev Cardiol. (2019) 27:1036–44. doi: 10.1177/2047487319887281
47. Zaccardi F, Franks PW, Dudbridge F, et al. Mortality risk comparing walking pace to handgrip strength and a healthy lifestyle: a UK Biobank study. Eur J Prev Cardiol. (2019) 28:704–12. doi: 10.1177/2047487319885041
48. Hendriks T, Said MA, Janssen LMA, van der Ende MY, van Veldhuisen DJ, Verweij N, et al. Effect of systolic blood pressure on left ventricular structure and function: a mendelian randomization study. Hypertension. (2019) 74:826–32. doi: 10.1161/HYPERTENSIONAHA.119.12679
49. Bentley DC, Nguyen CH and Thomas SG. Resting blood pressure reductions following handgrip exercise training and the impact of age and sex: a systematic review and narrative synthesis. Syst Rev. (2018) 7:229. doi: 10.1186/s13643-018-0876-5
50. Yeckel CW, Gulanski B, Zgorski ML, Dziura J, Parish R, Sherwin RS. Simple exercise recovery index for sympathetic overactivity is linked to insulin resistance. Med Sci Sports Exerc. (2009) 41:505–15. doi: 10.1249/MSS.0b013e31818afa2f
51. Laukkanen JA, Willeit P, Kurl S, Mäkikallio TH, Savonen K, Ronkainen K, et al. Elevated systolic blood pressure during recovery from exercise and the risk of sudden cardiac death. J Hypertens. (2014) 32:659–66. doi: 10.1097/HJH.0000000000000066
52. McHam SA, Marwick TH, Pashkow FJ, Lauer MS. Delayed systolic blood pressure recovery after graded exercise: an independent correlate of angiographic coronary disease. J Am Coll Cardiol. (1999) 34:754–9. doi: 10.1016/S0735-1097(99)00269-7
53. Amon KW, Richards KL, Crawford MH. Usefulness of the postexercise response of systolic blood pressure in the diagnosis of coronary artery disease. Circulation. (1984) 70:951–6. doi: 10.1161/01.CIR.70.6.951
54. Tseng Y-J, Wang H-Y, Lin T-W, Lu J-J, Hsieh C-H, Liao C-T. Development of a machine learning model for survival risk stratification of patients with advanced oral cancer. JAMA Netw Open. (2020) 3:e2011768. doi: 10.1001/jamanetworkopen.2020.11768
55. Yedinak JL Li Y, Krieger MS, Howe K, Ndoye CD, Lee H, et al. Machine learning takes a village: assessing neighbourhood-level vulnerability for an overdose and infectious disease outbreak. Int J Drug Policy. (2021) 31:103395. doi: 10.1016/j.drugpo.2021.103395
56. Jardillier R, Chatelain F, Guyon L. Benchmark of lasso-like penalties in the Cox model for TCGA datasets reveal improved performance with pre-filtering and wide differences between cancers. bioRxiv [Preprint]. (2020) doi: 10.1101/2020.03.09.984070
57. Laukkanen JA, Kurl S. Blood pressure responses during exercise testing-is up best for prognosis? Ann Med. (2012) 44:218–24. doi: 10.3109/07853890.2011.560180
58. Moradi M, Farhadian M, Kazemi M. Assessing post-cardiac stress test heart rate recovery and systolic blood pressure recovery in patients suffering from vasovagal syncope. Electron J Gen Med. (2019) 16:em118. doi: 10.29333/ejgm/93476
59. Lee PH, Macfarlane DJ, Lam TH, Stewart SM. Validity of the International Physical Activity Questionnaire Short Form (IPAQ-SF): a systematic review. Int J Behav Nutr Phys Act. (2011) 8:115. doi: 10.1186/1479-5868-8-115
Keywords: heart rate recovery, autonomic nervous system, physical activity, rheumatoid arthritis, inflammation, cardiovascular disease, blood pressure, muscular strength
Citation: Hupin D, Sarajlic P, Venkateshvaran A, Fridén C, Nordgren B, Opava CH, Lundberg IE and Bäck M (2021) Cardiovascular Autonomic Function Changes and Predictors During a 2-Year Physical Activity Program in Rheumatoid Arthritis: A PARA 2010 Substudy. Front. Med. 8:788243. doi: 10.3389/fmed.2021.788243
Received: 01 October 2021; Accepted: 09 November 2021;
Published: 15 December 2021.
Edited by:
Alberto Gullo, Centro Neurolesi Bonino Pulejo (IRCCS), ItalyReviewed by:
Anastasios Lymperopoulos, Nova Southeastern University, United StatesHuangHsi Chen, Chung Shan Medical University Hospital, Taiwan
Copyright © 2021 Hupin, Sarajlic, Venkateshvaran, Fridén, Nordgren, Opava, Lundberg and Bäck. This is an open-access article distributed under the terms of the Creative Commons Attribution License (CC BY). The use, distribution or reproduction in other forums is permitted, provided the original author(s) and the copyright owner(s) are credited and that the original publication in this journal is cited, in accordance with accepted academic practice. No use, distribution or reproduction is permitted which does not comply with these terms.
*Correspondence: David Hupin, ZGF2aWQuaHVwaW4mI3gwMDA0MDtraS5zZQ==