- 1Centro de Educación Médica e Investigaciones Clínicas Norberto Quirno (CEMIC), Buenos Aires, Argentina
- 2Independent Researcher, São Paulo, Brazil
- 3Hospital Italiano de Buenos Aires, Buenos Aires, Argentina
The prevalence of chronic kidney disease (CKD) continues to increase worldwide, as well as the associated morbidity and mortality and the consequences on the patients' quality of life and countries' economies. CKD often evolves without being recognized by patients and physicians, although the diagnosis is based on two simple laboratory data: the estimated glomerular filtration rate (eGFR) and urine analysis. To measure GFR, the knowledge about the physiologic processes at the nephron level, the concept of clearance, and the identification of creatinine as a suitable endogenous marker for measuring the creatinine clearance (CrCl) had to be previously developed. On those bases, different equations to calculate CrCl (Cockcroft and Gault, 1976), or estimated GFR (four variables MDRD, 1999; CKD-Epi, 2009, among others) were generated. They all include creatinine and some demographic data, such as sex and age. However, to compare results throughout life or among laboratories, the creatinine determination must be standardized. In addition, the accuracy of these equations remains controversial in certain subgroups of patients. For these reasons, other mathematical models to improve CrCl estimation have been developed, such as when urine cannot be collected, in debilitated elderly patients and patients with trauma, diabetes, or obesity. Currently, eGFR in adults can be measured and reported immediately, using isotope dilution mass spectrometry traceable creatinine-based equations. In conclusion, based on knowledge obtained from renal physiology, eGFR can be used in the clinic for the diagnosis and early treatment of CKD, as well as a public instrument to estimate the prevalence.
Introduction
The prevalence of chronic kidney disease (CKD) continues to increase worldwide, as well as the associated morbidity and mortality and the consequences on patients' quality of life and countries' economies. In the year 2018, a joint document of the ASN, ERA-EDTA, and ISN societies estimated that over 850 million people worldwide (11% of the total population) lived with kidney disease, about twice the number of diabetic patients estimated by the IDF (422 million) (1–3). An analysis of the Global Burden of Disease Study stated that CKD as cause of death rose from position 25 in the year 1990 to the 17th in 2015 (4). Another publication from the same study estimated that CKD as a mortality cause would ascend to the 5th place by 2040 (5). Besides, CKD is an independent risk factor for cardiovascular disease, and a risk multiplier in other non-communicable chronic diseases such as hypertension, diabetes, and cardiovascular (6). These data confirm that CKD is a major public health problem.
Any clinical situation resulting from a reduction in the number of functioning nephrons can evolve to CKD, defined by KDIGO guidelines as “abnormalities in kidney structure or function, present for 3 months, with implications for health.” The same guideline classifies CKD based on the cause, glomerular filtration rate (GFR) category, and albuminuria (7).
Arterial hypertension, diabetes, obesity, proteinuric nephropathies, race, family history, genetic diseases, low birth weight, aging, among others, are risk factors for the CKD (8, 9). Early detection and treatment of potentially reversible risk factors and CKD allow to delay progression and its associated complications as well as reduce the risk of cardiovascular disease (10, 11).
In the real world, CKD is a silent disease that often evolves unrecognized by the patients and physicians, although the diagnosis is based on the two simple laboratory data: the estimated GFR (eGFR) and urine analysis (screening for albuminuria/proteinuria) (10). Early diagnosis of CKD by the general practitioners and generalists would contribute to retard progression, and reduce morbidity and mortality associated to CKD and its associated risk factors (12).
Glomerular filtration rate continues to be the best global index of kidney function, both in health and in disease, as it represents the excretory capacity of the kidney, correlates directly with the kidney functioning mass, to classify CKD in stages according to the risk of progression, and to calculate the drug dosing and preparing for the invasive studies. xx
Early diagnosis of CKD by the general practitioners and generalists would contribute to retard progression and reduce associated morbidity and mortality. Albuminuria, an important predictor of CKD progression, will not be analyzed in this article. Therefore, the evaluation of kidney function and the presence or absence of proteinuria/albuminuria should be part of any routine health evaluation, and desirable when conducting population health surveys.
The present manuscript, after a brief historical description on the milestones that paved the way since the emerging physiological concepts of filtration, reabsorption, and excretion at the nephron, will focus on the present concepts of eGFR, and how it can be applied in the clinic and as a public health tool. Finally, different eGFR equations derived from creatinine and cystatin C and demographic data used for the diagnosis in patients and as a public health instrument will be described.
The Identification of the Process of Glomerular Filtration to Measuring GFR in the Clinic
Knowledge of kidney physiology began in the mid-19th century, when Carl Ludwig (1816–1895) developed the concept of glomerular filtration. In his thesis, he identified the glomerulus as a filter, where urine formation began; this filter was submitted to physical and chemical forces, driven by the hydrostatic pressure generated by the heart, and regulated by the contraction and vasodilatation of the afferent and efferent arterioles. He went further, speculating that the filtered volume decreased along the tubules due to reabsorption, in order to concentrate the final products at the urine (13, 14).
In 1874, Rudolf Heidenhain (1834–1897) injected a dye, indigo carmine, in hypotensive anuric rabbits; after 15 min he removed the kidneys and identified the dye in tubular cells. He deduced that secretion from blood into the tubule occurred that meant an active tubular transport mechanism (15).
Arthur Cushny (1866–1926) in 1917 reasoned that Ludwig's theory (the glomerulus as a filter) implied a large volume of water, and near all the filtered glucose, amino acids, sodium, and other solutes should be in the ultrafiltrate. And, as these solutes are present at different concentrations in the plasma, the reabsorption of glucose, amino acids, and others dissolved substances present in urine should happen according to their respective blood levels. He concluded that there was a threshold for differential reabsorption of some solutes (16).
In 1924, Alfred Richards (1876–1966) and Joseph Wearns (1893–1984) published their results for the filtration process, infusing epinephrine into the glomerulus of an anesthetized frog, and observing the hemodynamic effects on the afferent and efferent arterioles and the resulting ultrafiltrate. They confirmed that the free-protein ultrafiltrate was due to filtration at the glomerular tuft, the solutes were filtered and reabsorbed at the tubular level, and there was a threshold for glucose reabsorption, corroborating the differential reabsorption of filtered solutes in the tubule (17).
At this point, the mechanism of filtration, secretion, and reabsorption in the nephron had been proved, but to transfer the concept of GFR to the clinic, it was still necessary to find a solute removed only by filtration, and not reabsorbed or secreted in the tubule. In 1926, Paul Rehberg identified creatinine as that solute, as it was produced by the body itself, filtered and, presumably, it was not reabsorbed or excreted (18).
Donald Van Slyke (1883–1971), in 1928, introduced the concept of “clearance,” regarding urea, as the volume of blood that would be totally cleared of it in a minute when urine flow exceeded 2 ml/min. The clearance technique was quickly applied to different solutes and became itself a fundamental tool in kidney physiology (19). Applying this concept, in 1937, Homer Smith measured GFR using inulin, a substance he had proved previously was excreted exclusively by glomerular filtration. After that, for many years, inulin was the gold standard to measure true GFR (20).
The clearance concept was fundamental, not only for studying the formation of urine or kidney physiology, but because it provided a simple tool to be used in the clinic, as GFR could be measured as creatinine clearance (CrCl). Since then, over many years, CrCl has been used in the clinic to evaluate GFR.
Creatinine and Creatinine Clearance as Estimated GFR
Creatinine is a waste product of muscle metabolism, generated relatively constantly. It is almost eliminated by the glomerular filtration as it is a small molecule (113 Daltons) not bound to proteins. However, as its concentration depends on muscle mass, it is different in men and women, and may change according to the protein diet and muscle mass (21).
In 1886, Max Jaffe (1841–1911) noticed that creatinine in contact with picric acid in an alkaline solution developed an orange-red color, proportional to creatinine concentration (22). Years later, in 1914, based on the Jaffe's experiment, Otto Folin measured the creatinine in deproteinized blood, incorporating Jaffe's reaction to the clinical diagnosis (23). By this time, creatinine had been identified as a substance removed only by filtration, the concept of clearance was introduced and widely applied in his studies by Homer Smith, and the determination of serum creatinine was available. All the conditions were met for using CrCl as a proxy for GFR in clinical practice.
At present, creatinine is one of the most frequent laboratory determinations: easy to perform, available almost everywhere, and cheap. It can be determined by enzymatic or colorimetric methods. However, as every analyte, serum creatinine measure is exposed to random error (performed by the operator) and systematic error (depending on the material, the instrument, and the process). Standardizing its determination as a method with traceable calibration to isotope dilution mass spectrometry (IDMS) reduces biases, improving the accuracy of creatinine determination (24, 25).
Isolated creatinine is not a good marker to evaluate kidney function, as it increases when GFR is around 50 ml/min or below. Creatinine clearance is better to estimate GFR, but it has some disadvantages when evaluating kidney function. Creatinine is not excreted only by glomerular filtration, as a small fraction is secreted at the tubular level. This fraction increases as kidney function decreases and cannot be calculated individually (21). Also, in advanced CKD, the intestinal microbiota contributes to degrading creatinine, and this proportion also cannot be estimated (26). Therefore, when CKD is present, CrCl tends to overestimate GFR, and the difference increases as kidney function decline. This fact moved to search mathematical formulas based on creatinine and demographic factors, such as age, sex, body mass index, and race, to estimate GFR (eGFR).
Despite the limitations described, creatinine continues to be the most frequent marker used in the clinic to assess the function of kidney.
In some situations, a 24-h urine collection is mandatory, and the measured creatinine clearance is preferred in some patient groups to avoid misinterpretations. This is the case of a very low protein intake, such as vegetarians, high protein intake, creatine supplementation, diet rich in meat, some muscle mass abnormalities (malnutrition, amputation, and loss of muscle mass), rapid change in kidney function, before starting dialysis or in children and pregnant women (27, 28).
The Development of Equations, Based on Creatinine, for Measuring eGFR
Equations to estimate GFR are widely used in day-to-day practice. More than 70 have been developed. In this publication, the most used and recent will be detailed.
One of the first ones was the Cockcroft–Gault (C–G) equation, available since the mid-1970s, that (or which) includes creatinine, sex, and weight, and is not adjusted for the body surface area (Table 1) (29). However, this equation correlates more to CrCl than to GFR. Besides that, the creatinine method used in the development of the C–G equation is no longer in use, and samples from the study are not available to compare the results to standardized creatinine values (30). Anyway, this equation has been and continues to be widely utilized, in part because many pharmacokinetic studies had been performed in the previously used C–G equation, before the standardization of serum creatinine traceable to IDMS (31, 32).
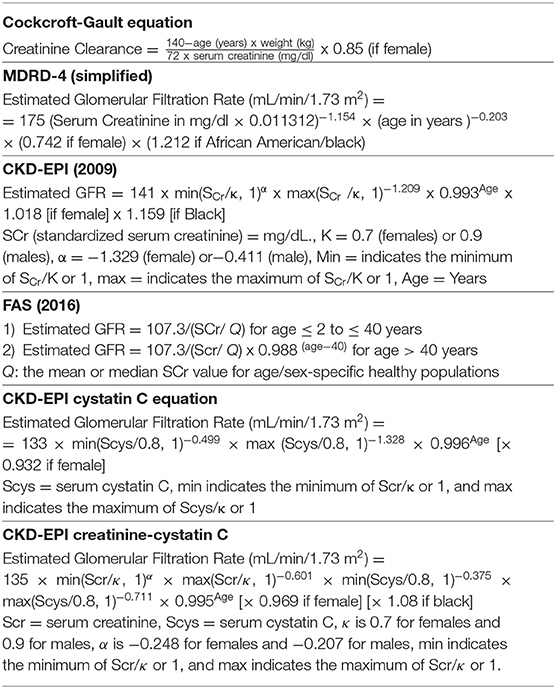
Table 1. Most used creatinine and cystatin C equations to estimate glomerular filtration rate (eGFR).
Similar equations require other data like patient height and/or weight; many times this information is not recorded or correctly recorded, favoring erroneous results.
In 1999, Levey and associates developed seven equations applying a regression model to predict eGFR, using data of 1,628 patients enrolled in the baseline period of the Modification of Diet in Renal Disease (MDRD) study. The equation that gave the best agreement with iothalamate-measured GFR was the six variable equation, valid for a standard body surface of 1.73 m2 (33):
GFR = 170 × [PCr]-0.999 × [Age]-0.176 × [0.762 if women] × [1.180 if African American/black] × [SUN]-0.170 × [Alb]+0.318
In 2000, Levey and coworkers proposed the simplified four-variable MDRD equation that correlates very well with the six-parameter equation proposed before (Table 1) (34). This formula, originally defined for serum creatinine measured by the old Jaffe method, was re-expressed with the serum creatinine calibrated to an assay traceable to IDMS in 2006 (35). A recommendation to convert Jaffe-measured creatinine into reference method-based procedures (SCr_Jaffe ×0.95 @ SCr_enzyme) if the reference procedure used for the calibration was IDMS has been proposed but a full agreement for doing so has not arisen (25).
The MDRD GFR has little bias compared with measured GFR with urinary clearance of iothalamate under 60 ml/min/1.73 m2, but underestimated the measured GFR at higher levels (36). These results are expected, as any equation reflects the characteristics of the population from which it derives; and the MDRD study was performed on the population with CKD.
In the year 2009, an improved creatinine-based equation, the CKD–EPI Collaboration (Chronic Kidney Disease Epidemiology Collaboration) was published, based on new methods for measuring creatinine, valid for all stages of renal insufficiency (Table 1). To work out the CKD–EPI equation, the authors took data from 8,254 participants of 10 studies (development set), 3,896 subjects from 16 studies (validation set) and, 16,032 individuals from NHANES (National Health and Nutritional Survey) for prevalence. The new equation performed significantly better than the MDRD study equation, especially at higher GFR, with lesser bias, improved precision, and greater accuracy (37).
This equation is still the most accurate GFR estimating equation evaluated in large diverse populations, applicable for the general clinical use. It provides lower estimates of the prevalence of decreased eGFR, and is useful as a trial measure for decreased eGFR and to replace the MDRD Study equation for routine reporting of serum creatinine-based eGFR by clinical laboratories (38).
A systematic search of MEDLINE, between 1999 and 21 October 2011, was performed by Earley et al. to review the GFR estimating equations performance; 12 studies were selected. In those from North America, Europe, and Australia, the authors corroborated, once again, that the CKD–EPI equation performed better at higher GFRs (> 60 ml/min/1.73 m2) and the MDRD equation behaved better at lower GFRs (<60 ml/min/1.73 m2). In Asian or African populations, neither equation worked as well as in the other regions (39).
KDIGO CKD Guidelines recommend clinical laboratories to report eGFR in adults using the 2009 CKD–EPI equation (7), provided creatinine determination is traceable to IDMS.
The inclusion of race in the eGFR equation has been questioned, even though, in adults, age, sex, weight, height, and race are surrogates of muscle mass. In the original MDRD and CKD–EPI equations, the inclusion of race (Black/non-Black) improved accuracy (40). It has been argued that to exclude race from the eGFR equations would provoke a systematic under interpretation of measured GFR. To disclosure about the use of race when estimating GFR, or to accept denial to identify race from the patient, or to use a cystatin C confirmatory test has been proposed as a way to overcome the conflict (41, 42). In September 2021, the National Kidney Foundation and the American Society of Nephrology Joint Task Force on Reassessing the Inclusion of Race in Diagnosing Kidney Diseases recommended a new 2021 CKD-EPI creatinine eGFR equation which does not include race to estimate GFR (Table 1). They recommend for the United States immediate implementation of the CKD-EPI creatinine equation refit without the race variable in all laboratories, and to facilitate increased, routine and timely use of cystatin C, as combining creatinine and cystatin C is more accurate (43).
Finally, in 2016, Pottel et al. developed a novel equation, the full age spectrum (FAS) equation, to estimate the GFR across all over the age spectrum since available equations lack continuity with aging (the Schwartz equation for pediatrics, the CKD–EPI equation for adults under 70 years age, and the BIS-1 for older than 70 years old). This new equation is normalized on serum Cr (SCr/Q) for age (children and adolescents) and gender (adolescents and adults), being Q the median serum Cr from a specific healthy subpopulation. In the validation study, 6,870 healthy and kidney disease caucasian and from the non-African origin individuals, of whom 765 were children and adolescents <18 years old and 1,748 elderly higher than 70 years old, participated. For validation, measured GFR was performed using inulin or iothalamate or iohexol clearance (Table 1) (44). The FAS equation that can be used in ages <2–100 years old, resulted less biased and more accurate than the Schwartz equation for children and adolescents, and less biased and as accurate as the CKD–EPI equation for adults under or over 70 years old.
The Development of Equations Using Cystatin C
Cystatin C was described for the first time in 1961 (45). As creatinine, it is an endogenous marker. It is a low molecular weight protein (13 kD) and consists of a chain of 120 amino acids. Produced constantly by all nucleated cells of the body, it filters freely through the glomerulus and is totally reabsorbed and catabolized by the proximal tubular cells. Muscle mass, age, sex, or diet do not affect its concentration; these characteristics make Cystatin C useful in groups with reduced muscle mass (46, 47).
To improve the accuracy of eGFR several equations have now been developed using either cystatin C alone or cystatin C in combination with creatinine. Cystatin C-based equations have advantages over the creatinine-based equation as they are less influenced by age, sex, and race (48). The 2012 CKD–EPI creatinine-cystatin C equation is more accurate than the 2009 CKD–EPI creatinine and 2012 CKD–EPI cystatin C equations and it is useful as a confirmatory test for decreased eGFR as determined by serum creatinine-based eGFR (Table 1) (49).
Despite its greater usefulness, cystatin C has not displaced creatinine for GFR estimation in clinical practice, possibly due to its higher cost and lower availability.
Clinical Situations Where mGFR Is Needed
In some clinical situations, such as patients with anorexia nervosa, cirrhosis, obesity, evaluation of living kidney donors, prescribing nephrotoxic drugs with a narrow therapeutic window, pharmacokinetic studies of drugs excreted by the kidney, or any situation in which eGFR is unreliable, it is reasonable to measure GFR (mGFR), despite the added cost and time and resources consuming (7, 50–52). At present, iothalamate or 51Cr-EDTA or 99Tcm-DTPA urinary clearance or 99Tcm-DTPA or iohexol plasma clearance are the accurate methods for determining mGFR (52–55). In patients with large edema or ascites, urinary clearance should be employed (55).
Iohexol, a low-cost non-toxic non-ionic contrast agent, has some advantages for the plasma clearance such as simplicity, low cost, stability, and low interlaboratory variation. Besides that, it is not radioactive, is excreted almost exclusively by the kidney, is neither secreted nor reabsorbed at the tubular level, has low protein binding, and correlates with inulin renal clearance (56, 57). It is contraindicated in patients with allergy to iodine.
Conclusion
From the knowledge that emerges from renal physiology, laboratory medicine, epidemiology, and biostatistics, have emerged equations that constitute tools not only for the clinical care of patients, but also to establish the prevalence of CKD, and consequently implementing the public health policies aimed to reduce it.
The inclusion of the automatic calculation of GFR in the laboratory reports of creatinine determinations constitutes a useful tool for daily practice, and from public health perspectives for population screening for CKD.
CKD–EPI Study equation continues to be the most used in general practice and from public health perspective. Recently, the FAS equation emerged as a promising option to estimate eGFR for all ages (from 2 to 100 years old).
Further improvement in GFR estimating equations will require development in more broadly representative populations, such as diverse racial and ethnic groups, use of multiple filtration markers, and evaluation using statistical techniques to compare eGFR to mGFR.
Author Contributions
All authors listed have made a substantial, direct, and intellectual contribution to the work and approved it for publication.
Conflict of Interest
The authors declare that the research was conducted in the absence of any commercial or financial relationships that could be construed as a potential conflict of interest.
Publisher's Note
All claims expressed in this article are solely those of the authors and do not necessarily represent those of their affiliated organizations, or those of the publisher, the editors and the reviewers. Any product that may be evaluated in this article, or claim that may be made by its manufacturer, is not guaranteed or endorsed by the publisher.
References
1. American Society of Nephrology (ASN) European Renal Association – European Dialysis and Transplant Association (ERA-EDTA) International Society of Nephrology (ISN). The Hidden Epidemic: Worldwide, Over 850 Million People Suffer From Kidney Diseases. (2018). Available online at: https://www.asn-online.org/news/2018/0626-Joint_Hidden_Epidem.pdf (accessed July 22, 2021).
2. The World bank Total Population. Available online at: https://data.worldbank.org/indicator/SP.POP.TOTL (accessed July 22, 2021).
3. Who Fact sheets Diabetes. Available online at: http://www.who.int/news-room/fact-sheets/detail/diabetes (accessed July 22, 2021).
4. GBD 2015 Mortality and Causes of Death Collaborators. Global, regional, and national life expectancy, all-cause mortality, and cause-specific mortality for 249 causes of death, 1980-2015: a systematic analysis for the Global Burden of Disease Study 2015. Lancet. (2016) 388:1459–544. doi: 10.1016/S0140-6736(16)31012-1
5. Foreman KJ, Marquez N, Dolgert A, Fukutaki K, Fullman N, McGaughey M. Forecasting life expectancy, years of life lost, and all-cause and cause-specific mortality for 250 causes of death: reference and alternative scenarios for 2016-40 for 195 countries and territories. Lancet. (2018) 392:2052–90. doi: 10.1016/S0140-6736(18)31694-5
6. Matsushita K, Coresh J, Sang Y, Chalmers J, Fox C, Guallar E, et al. Estimated glomerular filtration rate and albuminuria for prediction of cardiovascular outcomes; a collaborative meta-analysis of indivividual participant data. Lancet Diabetes Endocrinol. (2015) 3:514–25. doi: 10.1016/S2213-8587(15)00040-6
7. Kidney Disease: Improving Global Outcomes (KDIGO) CKD Work Group. KDIGO 2012 clinical practice guideline for the evaluation and management of chronic kidney disease. Kidney Int Suppl. (2013) 3:1–150. doi: 10.1038/kisup.2012.73
8. National Kidney Foundation. K/DOQI clinical practice guidelines for chronic kidney disease: evaluation, classification, and stratification. Am J Kidney Dis. (2002) 39(2 Suppl. 1): S1–266.
9. Wang Y, Chen X, Caballero B, Cheskin LJ. Association between obesity and kidney disease: a systematic review and meta-analysis. Kidney Int. (2008)73:19–33. doi: 10.1038/sj.ki.5002586
10. Vassalotti JS, Stevens LA, Levey AS. Testing for chronic kidney disease: a position statement from the National Kidney Foundation. Am J Kidney Dis. (2007) 50:169–80. doi: 10.1053/j.ajkd.2007.06.013
11. Levin A, Stevens PE. Early detection of CKD: the benefits, limitations and effects on prognosis. Nat Rev Nephrol. (2011) 7:446–57. doi: 10.1038/nrneph.2011.86
12. Schlipak MG, Tummalapalli SL, Boulware LE, Winkelmayer WC, Zoungas S. for Conference Participants. The case for early identification and intervention of chronic kidney disease: conclusions from a Kidney Disease: Improving Global Outomes (KDIGO) Controversies Conference. Kidney Int. (2021) 99:34–47. doi: 10.1016/j.kint.2020.10.012
14. Davies J, Gottschalk CW, Haberle DA, Thurau K. Carl Ludwig's revolutionary concept of renal function. Kidney Int. (1994) 46:1–23.
15. Jamison RL. Resolving an 80-yr. -old controversy: the beginning of the modern era of renal physiology. Adv Physiol Educ. (2014) 38:286–95. doi: 10.1152/advan.00105.2014
17. Wearn JT, Richards AN. Observations of the composition of glomerular urine, with particular reference to the problem of reabsorption in the renal tubules. Am J Physiol. (1924) 71:0209–227. doi: 10.1152/ajplegacy.1924.71.1.209
18. Rehberg PB. Studies on kidney function. I The rate of filtration and reabsorption in the human kidney. Biochem J. (1926) 20:447–60. doi: 10.1042/bj0200447
19. Moller E, McIntosh JF, Van Slike DD. Studies on urea excretion. II. Relationship between urine volume and the ratio of urea excretion by normal adults. J Clin Invest. (1928) 6:427–65. doi: 10.1172/JCI100206
20. Smith HW. Note on the interpretation of clearance methods in the diseased kidney. J Clin Invest. (1941) 20:631–5. doi: 10.1172/JCI101256
21. Perrone RD, Madias NE, Levey AS. Serum Creatinine as an Index of Renal Function: New Insights into Old Concepts. Clin Chem. (1992) 38:1933–53. doi: 10.1093/clinchem/38.10.1933
22. Jaffe M. Über den Niederschlag, welchen Pikrinsäre in normalem Harn erzeugt und über eine neue Reaction des Kreatinins. Zeitschrift für physiologische Chemie. (1886) 10:391–400. doi: 10.1515/bchm1.1886.10.5.391
23. Shaffer PA. Otto Folin 1867-1934. Washington, DC: National Academy of Sciences (1952). p. 47–82.
24. Cook JG. Factors influencing the assay of creatinine. Ann Clin Biochem. (1975) 12:219–32. doi: 10.1177/000456327501200162
25. Myers GL, Miller WG, Coresh J, Fleming J, Greenberg N, Greene T, et al. Recommendations for improving serum creatinine measurement: a report from the laboratory working group of the National kidney disease Education program. Clin Chem. (2006) 52:5–18. doi: 10.1373/clinchem.2005.0525144
26. Papadakis MA, Arieff AI. Unpredictability of clinical evaluation of renal function in cirrhosis. Prospective study. Am J Med. (1987) 82:945–52. doi: 10.1016/0002-9343(87)90156-2
27. Stevens LA, Coresh J, Greene T, Levey AS. Assessing kidney function: measured and estimated glomerular filtration rate. N Engl J Med. (2006) 354:2473–83. doi: 10.1056/NEJMra054415
28. Lopes van Balen VA, van Gansewinkel TAG, de Haas S, Spaan JJ, Ghossein-Doha C, van Kuijk SMJ, et al. Maternal kidney function during pregnancy: systematic review and meta-analysis. Ultrasound Obstet Gynecol. (2019) 54:297–307. doi: 10.1002/uog.20137
29. Cockcroft DW, Gault MH. Prediction of creatinine clearance from serum creatinine. Nephron. (1976) 16:31–41. doi: 10.1159/000180580
31. National Kidney Disease Education Program: NKDEP CKD and Drug Dosing: Information for Providers. Available online at: http://www.nkdep.nih.gov/professionals/drug-dosing-information.htm#cockcroft-gault (accessed August 31, 2021).
32. Matzke GR, Aronoff GR, Atkinson AJ, Bennett WM, Decker BS, Eckardt KW, et al. Drug dosing consideration in patients with acute and chronic kidney disease-a clinical update from Kidney Disease: Improving Global Outcomes (KDIGO). Kidney Int. (2011) 80:1122–37. doi: 10.1038/ki.2011.322
33. Levey AS, Bosch JP, Lewis JB, Greene T, Rogers N, Roth D, et al. more accurate method to estimate glomerular filtration rate from serum creatinine: a new prediction equation. Modification of Diet in Renal Disease Study Group. Ann Intern Med. (1999) 130:461–70. doi: 10.7326/0003-4819-130-6-199903160-00002
34. Levey AS, Green T, Kusek J, Beck GA. simplified equation to predict glomerular filtration rate from serum creatinine. J Am Soc Nephrol. (2000) 11:155A.
35. Levey AS, Coresh J, Greene T, Stevens LA, Zhang YL, Hendriksen S et al. Using standardized serum creatinine values in the modification of diet in renal disease study equation for estimating glomerular filtration rate. Ann Intern Med. (2006) 145:247–54. doi: 10.7326/0003-4819-145-4-200608150-00004
36. Stevens LA, Coresh J, Feldman HI, Greene T, Lash JP, Nelson RJ, et al. Evaluation of the modification of diet in renal disease study equation in a large diverse population. J Am Soc Nephrol. (2007) 18:2749–57. doi: 10.1681/ASN.2007020199
37. Levey AS, Stevens LA, Schmid CH, Zhang Y, Castro AF, Feldman HI, et al. New equation to estimate glomerular filtration rate. Ann Intern Med. (2009) 150:604–12. doi: 10.7326/0003-4819-150-9-200905050-00006
38. Levey AS, Stevens LA. Estimating GFR using the CKD Epidemiology Collaboration (CKD-EPI) creatinine equation: more accurate GFR estimates, lower CKD prevalence estimates, and better risk predictions. Am J Kidney Dis. (2010) 55:622–7. doi: 10.1053/j.ajkd.2010.02.337
39. Earley A, Miskulin D, Lamb EJ. S Levey AS, Uhlig K. Estimating equations for glomerular filtration rate in the era of creatinine standardization: a systematic review. Ann Intern Med. (2012) 156:785–95. doi: 10.7326/0003-4819-156-11-201203200-00391
40. Levey AS, Titan SM, Powe NR, Coresh J, Inker LA. Kidney disease, race, and GFR estimation. Clin J Am Soc Nephrol. (2020) 15:1203–12. doi: 10.2215/CJN.12791019
41. Duggal V. Thomas I-Chun, Montez-Rath ME, Chertow GM, Tamura MK. National Estimates of CKD prevalence and potential impact of estimating glomerular filtration rate without race. J Am Soc Nephrol. (2021) 32:1454–63. doi: 10.1681/ASN.2020121780
42. Hsu C, Yang W, Parikh RV, Anderson AH, Chen TK, Cohen DL, et al. Race, genetic ancestry, and estimating kidney function in CKD. N Engl J Med. (2021) 385:1750–60. doi: 10.1056/NEJMoa2103753
43. Delgado C, Baweja M, Crews DC, Eneanya ND, Gadegbeku CA, Inker LA, et al. A Unifying Approach for GFR Estimation: Recommendations of the NKF-ASN Task Force on Reassessing the Inclusion of Race in Diagnosing Kidney Disease. Am J Kid Dis. (2021) 22:S0272-6386(21)00828–3. doi: 10.1053/j.ajkd.2021.08.003
44. Pottel H, Hoste L, Dubourg L, Ebert N, Schaeffner E, Eriksen BO, et al. An estimated glomerular filtration rate equation for the full age spectrum. Nephrol Dial Transplant. (2016) 31:798–806. doi: 10.1093/ndt/gfv454
45. Butler EA, Flynn FV. The occurrence of part-gamma protein in urine: a new protein abnormality. J Clin Path. (1961) 14:172–8. doi: 10.1136/jcp.14.2.172
46. Abrahamson M, Olafsson I, Palsdottir A, Ulvsbäck M, Lundwall A, Jensson O, et al. Structure and expression of the human cystatin C gene. Biochem J. (1990) 268:287–94. doi: 10.1042/bj2680287
47. Filler G, Bokenkamp A, Hofmann W, Bricon TL, Martinez-Bru C, Grubb A. Cystatin C as a marker of GFR – history, indications, and future research. Clin Biochem. (2005) 38:1–8. doi: 10.1016/j.clinbiochem.2004.09.025
48. Stevens LA, Coresh J, Schmid CH, Feldman HI, Froissart M, Kusek J et al. Estimating GFR using serum cystatin C alone and in combination with serum creatinine: a pooled analysis of 3,418 individuals with CKD. Am J Kidney Dis. (2008) 51:395–406. doi: 10.1053/j.ajkd.2007.11.018
49. Inker LA, Schmid CH, Tighiouart H, Eckfeldt JH, Feldman HI, Greene T et al. Estimating glomerular filtration rate from serum creatinine and cystatin C. N Engl J Med. (2012) 367:20–9. doi: 10.1056/NEJMoa1114248
50. Ebert E, Bevc S, Bökenkamp A, Gaillard F, Hornum M, Jager KJ, et al. Assessment of kidney function: clinical indications for measured GFR. Clin Kidney J. (2021) 14:1861–70. doi: 10.1093/ckj/sfab042
51. European Medicines Agency. Guideline on the Evaluation of the Pharmacokinetics of Medicinal Products in Patients With Decreased Renal Function. London: European Medicines Agency (2014). Available online at: http://www.ema.europa.eu/docs/en_GB/document_library/Scientific_guideline/2014/02/WC500162133.pdf (accessed November 11, 2021).
52. Soveri I, Berg UB, Björk J, Elinder CG, Grubb A, Mejare I, et al. Measuring GFR: a systematic review. Am J Kidney Dis. (2014) 64:411–24. doi: 10.1053/j.ajkd.2014.04.010
53. Agarwal R, Delanaye P. Glomerular filtration rate: when to measure and in which patients? Nephrol Dial Transpl. (2019) 34:2001–7. doi: 10.1093/ndt/gfy363
54. Vidal-Petiot E, Courbebaisse M, Livrozet M, Corrégé G, Rusu T, Montravers F, et al. Comparison of 51Cr-EDTA and 99mTc-DTPA for glomerular filtration rate measurement. J Nephrol. (2021) 34:729–37. doi: 10.1007/s40620-020-00932-9
55. Delanaye P, Ebert N, Melsom T, Gaspari F, Mariat C, Cavalier E, et al. Iohexol plasma clearance for measuring glomerular filtration rate in clinical practice and research: a review. Part 1: How to measure glomerular filtration rate with iohexol? Clin Kidney J. (2016) 9:682–99. doi: 10.1093/ckj/sfw070
56. Gaspari F, Perico N, Ruggenenti P, Mosconi L, Amuchastegui CS, Guerini E, et al. Plasma clearance of nonradioactive lohexol as a measure of glomerular filtration pate1. J Am Soc Nephrol. (1995) 6:257–63. doi: 10.1681/ASN.V62257
Keywords: glomerular filtration rate, chronic kidney disease, MDRD study equation, CKD-EPI equation, cystatin C, creatinine clearance
Citation: Cusumano AM, Tzanno-Martins C and Rosa-Diez GJ (2021) The Glomerular Filtration Rate: From the Diagnosis of Kidney Function to a Public Health Tool. Front. Med. 8:769335. doi: 10.3389/fmed.2021.769335
Received: 01 September 2021; Accepted: 25 October 2021;
Published: 25 November 2021.
Edited by:
Sergey Brodsky, Ohio State University Hospital, United StatesReviewed by:
Sebastjan Bevc, Maribor University Medical Centre, SloveniaLena Jafri, Aga Khan University, Pakistan
Copyright © 2021 Cusumano, Tzanno-Martins and Rosa-Diez. This is an open-access article distributed under the terms of the Creative Commons Attribution License (CC BY). The use, distribution or reproduction in other forums is permitted, provided the original author(s) and the copyright owner(s) are credited and that the original publication in this journal is cited, in accordance with accepted academic practice. No use, distribution or reproduction is permitted which does not comply with these terms.
*Correspondence: Ana Maria Cusumano, anacusumano@yahoo.com.ar