- 1Department of Rheumatology, Renji Hospital, Shanghai Jiao Tong University School of Medicine, Shanghai, China
- 2Department of Pulmonology, Renji Hospital, Shanghai Jiao Tong University School of Medicine, Shanghai, China
- 3CT Scientific Collaboration, Siemens Healthineers, Shanghai, China
- 4State Key Laboratory of Respiratory Disease, National Clinical Center for Respiratory Disease, Guangzhou Institute of Respiratory Health, The First Affiliated Hospital of Guangzhou Medical University, Guangzhou, China
- 5Department of Radiology, Renji Hospital, Shanghai Jiao Tong University School of Medicine, Shanghai, China
Objectives: Anti-melanoma differentiation-associated gene 5-positive dermatomyositis-associated interstitial lung disease (MDA5+ DM-ILD) is a life-threatening disease. The current study aimed to quantitatively assess the pulmonary high-resolution computed tomography (HRCT) images of MDA5+ DM-ILD by applying the radiomics approach and establish a multidimensional risk prediction model for the 6-month mortality.
Methods: This retrospective study was conducted in 228 patients from two centers, namely, a derivation cohort and a longitudinal internal validation cohort in Renji Hospital, as well as an external validation cohort in Guangzhou. The derivation cohort was randomly divided into training and testing sets. The primary outcome was 6-month all-cause mortality since the time of admission. Baseline pulmonary HRCT images were quantitatively analyzed by radiomics approach, and a radiomic score (Rad-score) was generated. Clinical predictors selected by univariable Cox regression were further incorporated with the Rad-score, to enhance the prediction performance of the final model (Rad-score plus model). In parallel, an idiopathic pulmonary fibrosis (IPF)-based visual CT score and ILD-GAP score were calculated as comparators.
Results: The Rad-score was significantly associated with the 6-month mortality, outperformed the traditional visual score and ILD-GAP score. The Rad-score plus model was successfully developed to predict the 6-month mortality, with C-index values of 0.88 [95% confidence interval (CI), 0.79–0.96] in the training set (n = 121), 0.88 (95%CI, 0.71–1.0) in the testing set (n = 31), 0.83 (95%CI, 0.68–0.98) in the internal validation cohort (n = 44), and 0.84 (95%CI, 0.64–1.0) in the external validation cohort (n = 32).
Conclusions: The radiomic feature was an independent and reliable prognostic predictor for MDA5+ DM-ILD.
Introduction
Anti-melanoma differentiation-associated gene 5-positive dermatomyositis (MDA5+ DM) is a distinct subtype of dermatomyositis (DM) predominantly reported in East Asia, characterized by pathognomonic rashes, none or mild myositis, and, notably, rapidly progressive interstitial lung disease (ILD). Recent study had reported three subgroups of MDA5+ DM with different prognoses. Patients with rapidly progressive ILD had a very high mortality rate, compared to those with frequent signs of skin vasculopathy and with frequent arthralgia or arthritis (1). The overall prognosis of MDA5+ DM-ILD is poor with most fatalities occurring within the first half year since disease onset. A gravely 6-month mortality rate of around 50% was reported despite the intensive immunosuppressive therapy (2–5).
As a mainstream imaging tool for identifying ILD, pulmonary high-resolution computed tomography (HRCT) is also crucial in the prognostic prediction. Moreover, semi-quantitative HRCT score has been applied as a prognostic prediction measurement in MDA5+ DM-ILD (6, 7). However, currently available HRCT scoring methods are observer dependent, with considerable inter-observer bias and initially derived for idiopathic pulmonary fibrosis (IPF) (8–10). When referring to MDA5+ DM-ILD with a much more aggressive clinical course, the applicability of the scoring system is controversial (11). Therefore, a measurable and quantitative method to assess severity and predict prognosis of MDA5+ DM-ILD is urgently needed. The technological advance of “radiomics” has provided a promising solution for HRCT evaluation in a more comprehensive and objective perspective. The technological advance of “radiomics” is a conversion of digital medical images into mineable high-dimensional data. It may provide a promising solution for HRCT evaluation in a more comprehensive and objective perspective. Since the radiographic images contain information that reflects underlying pathophysiology, Radiomics could improve predictive or prognostic accuracy potentially. In the area of oncology, radiomics has enabled the non-invasive profiling of tumor heterogeneity and direct estimation of prognosis by high-throughput extraction of quantitative descriptors from radiographic images (12, 13). To the best of our knowledge, there was no radiomics study focusing on MDA5+ DM-ILD. Therefore, the current study aimed to quantitatively assess MDA5+ DM-ILD by applying the radiomics approach and further establish an applicable multidimensional risk prediction model by incorporating clinical factors for 6-month mortality to improve the prognosis of this disease.
Materials and Methods
Patients
The study population consisted of hospitalized patients from two centers: A derivation cohort from April 2014 to October 2019 and a longitudinal internal validation cohort from November 2019 to May 2020 were recruited in Renji Hospital (Shanghai). However, an external validation cohort from Guangzhou Institute of Respiratory Health was constructed during the same time period. Ethical approval was obtained for this retrospective analysis, and the need to obtain informed consent was waived for both institutions. Eligible patients for this study initially fulfilled Bohan and Peter's criteria for DM or Sontheimer's criteria for clinically amyopathic DM on admission (14, 15) and were retrospectively confirmed by the 239th European NeuroMuscular Center (ENMC) classification criteria for DM (16). All patients presented with imaging-confirmed ILD and positive anti-MDA5 antibody. The anti-MDA5 antibody was detected by immunoblotting assay (Euroimmun, Germany) and confirmed by enzyme-linked immunosorbent assay (Supplementary Material). ILD course was defined as time from the first abnormal pulmonary CT, which revealed ILD changes to admission. Patients with ILD course > 3 months or coexisting malignancy (within 3 years) or preexisting chronic obstructive pulmonary disease were excluded. The primary outcome was the 6-month all-cause mortality since the time of admission.
The derivation cohort was randomly divided into the training (n = 121) and testing (n = 31) sets. The internal and external validation cohorts comprised 44 and 32 patients, respectively (Figure 1).
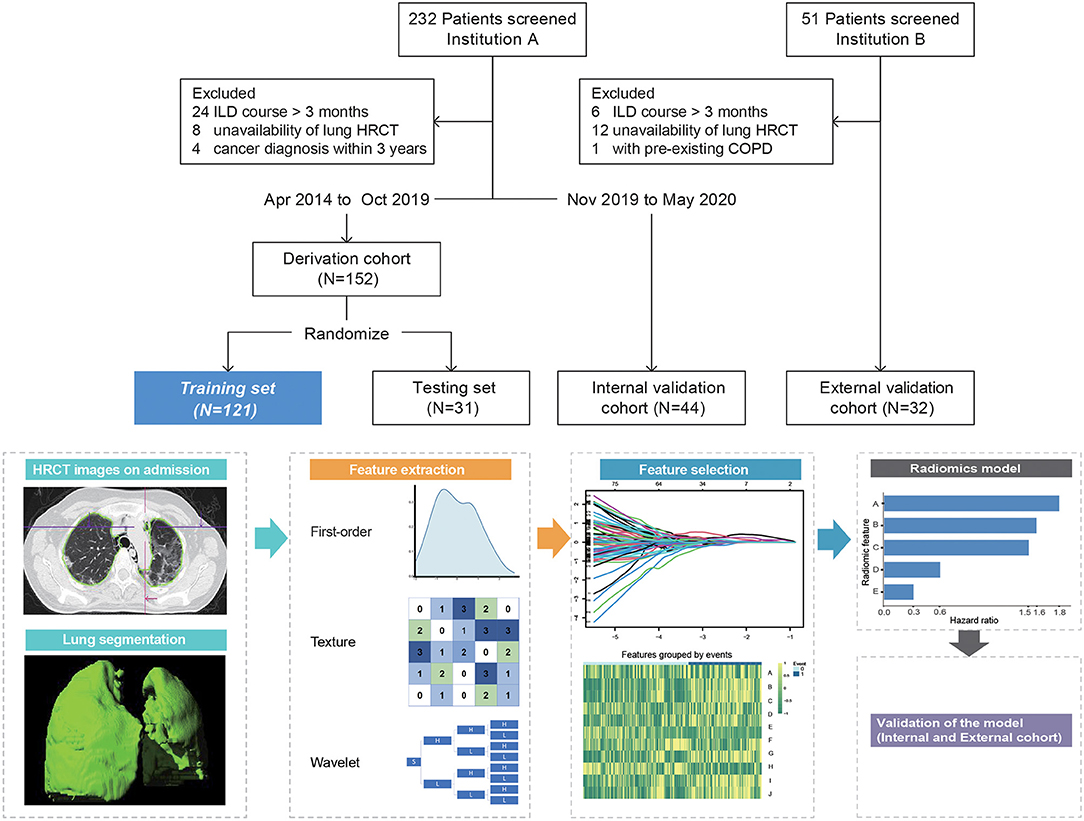
Figure 1. Overview of patient selection and workflow of radiomics model construction. Radiomic feature A = Original_Shape_Flatness (HR 1.82, 95%CI 1.28–2.59, p < 0.001), B = Wavelet.LLL_Glcm_MCC (HR 1.59, 95%CI 0.97–2.6, p = 0.068), C = Wavelet.LLH_Glcm_Ldmn (HR 1.5, 95%CI 1.02–2.22, p = 0.04), D = Wavelet.HLL_Glszm_LargeAreaHighGrayLevelEmphasis (HR 0.58, 95%CI 0.38–0.89, p < 0.001), and E = Wavelet.LLL_Firstorder_Skewness (HR 0.30, 95%CI 0.18–0.52, p < 0.001). ILD course, time from the first abnormal pulmonary CT that revealed ILD changes to admission; HRCT, high-resolution computed tomography; COPD, chronic obstructive pulmonary disease.
Radiomics Analysis of HRCT Images
Patients underwent non-contrast pulmonary HRCT within a week of admission (median, 2 days; range, 1–6 days) using a multidetector CT scanner. CT slice thickness was 1.0–1.5 mm at 10-mm interval with standard reconstruction. Radiomics analysis was performed using a dedicated software (Radiomics, syngo.via Frontier 1.2.2, Siemens Healthineers, Forchheim, Germany). This platform integrated an open-source library named “PyRadiomics” (version 2.0.1). First, the Digital Imaging and Communications in Medicine files of CT images were imported; segmentation of the volumetric lung was performed automatically with deep learning algorithm and adjusted manually by ZC and YZ (both with more than 5 years' experience in chest CT imaging). The medical image series were resampled to 1 1 1 mm voxel size with B-spline interpolator before subsequent feature extraction steps. Bin width was set at 25 to create histograms for discretization of the image gray levels. The extracted features are as follows: (i) 18 first-order features, which describe the distribution of voxel intensities in region of interest (ROI); (ii) 18 shape and size features, which include descriptors of 2D and 3D size and shape of ROI; (iii) 75 texture features, describing patterns or the spatial distribution of voxel intensities. In addition to the original images, wavelet-transformed images were generated using eight different high- to low-frequency band combinations according to three directions.
Thus, a total of 855 features, namely, 111 from the original image and 744 from the wavelet-transformed images, were outputted. Then, the intra- and inter-observer reliabilities were evaluated using Spearman's correlation coefficients in derivation cohort; features with rho>0.8 were included for further analysis.
Radiomics Model Construction and Radiomic Score (Rad-Score) Generation
The radiomics model was derived from the training set and tuned in the testing set. The least absolute shrinkage and selection operator (LASSO) and Cox regression model was used to select features, with penalty parameter tuning conducted by ten-fold cross-validation (17). Then, optimal features for radiomics model were determined by backward stepdown analysis with Akaike's information criterion (AIC) applied as the stopping rule.
Radiomic score for each patient was calculated from a linear combination of selected radiomic features weighted by respective coefficients.
Visual HRCT Scoring and ILD-GAP Model
All CT images were reviewed by ZC and YZ who were blinded to patients' outcome. Inter-observer variability was evaluated by intraclass correlation coefficient (ICC). The results were agreed upon by consensus between the two observers. HRCT findings were graded on a scale of 1–6 based on the classification system that corresponded to consecutive pathologic phases as previously reported (8): 1, normal attenuation; 2, ground-glass attenuation (GGA); 3, consolidation; 4, GGA associated with traction bronchiolectasis or bronchiectasis; 5, consolidation associated with traction bronchiolectasis or bronchiectasis; and 6, honeycombing.
The lungs were divided into six zones (upper, middle, and lower on both sides); each zone was evaluated separately. The score was based on the percentage of the abnormal lung parenchyma and was estimated to the nearest 5% of parenchymal involvement. The overall CT visual score was calculated by adding the average score of six zones (6).
In addition, an ILD-GAP score without lung imaging information was also calculated in our analysis as a comparator, which was modified from the IPF-derived GAP staging system and had been validated in some non-IPF ILD cohorts for prognosis prediction (18, 19).
Finally, a subgroup analysis was performed to show in which cases the radiomics model outperformed the visual scoring model.
Statistical Analysis
The differences of clinical parameters between the derivation and internal validation and external validation cohorts were assessed by the Mann–Whitney U-test, chi-square test, or Fisher exact test, as appropriate. Post hoc comparisons were made with Bonferroni's correction for adjustments of multiple comparisons. The optimal cutoff values for significant continuous variables were identified by X-tile (20). The association between Rad-score and outcome was assessed by Kaplan–Meier survival plot and log-rank test.
An improved multidimensional prediction model based upon Rad-score (Rad-score plus model) was constructed by integrating clinically significant predictors from univariable analysis into the multivariable Cox proportional hazards model (backward stepdown selection with AIC).
Correlation analysis between visual score, Rad-score, and other variables were applied with Spearman's coefficients. Model discrimination was quantified by the Harrell concordance index (C-index) with 95%CI. The change in overall log-likelihood ratio was used to assess the increase in predictive power. Additionally, the model calibration was assessed using the calibration curve, Greenwood–Nam–D'Agostino (GND) test, and Brier score (13, 21, 22). A decision curve analysis was built to determine and compare the clinical usefulness of each model (23). Significance was defined as p-value <0.05.
Statistical analyses were performed on SPSS software version 25 (IBM Corp., Armonk, NY, United States) and R software version 3.6.1. All R codes used were available at GitHub (https://github.com/tomato08217/MDA-ILD5).
Results
The all-cause 6-month mortality was 62/152 (40.8%), 17/44 (38.6%), and 14/32 (43.8%) for derivation, internal validation, and external validation cohorts, respectively. Table 1 presents the comparable clinical characteristics of the three cohorts in detail. Comparisons between the training and testing sets of the derivation cohort are also listed in Supplementary Table 1. A total of 17.8% patients lacked the data of the forced vital capacity percentage of predicted (FVC%), since they were in severe condition unable to complete either routine or bedside spirometry. For the remaining patients, the optimal cutoff values calculated for FVC% were 49.7% (rounding to 50%). Therefore, we classified FVC% into three categories: FVC% ≥ 50%, FVC% <50%, and unable to perform pulmonary function tests.
Notably, the treatment regimens differed between the two centers, with significantly higher intensity of glucocorticoid and immunosuppressant exposure in the external validation cohort (Table 1). And, in the derivation cohort, the deceased received significantly higher dose of glucocorticoid than survivors, while the intensity of immunosuppressant use was comparable between the two groups (Table 2).
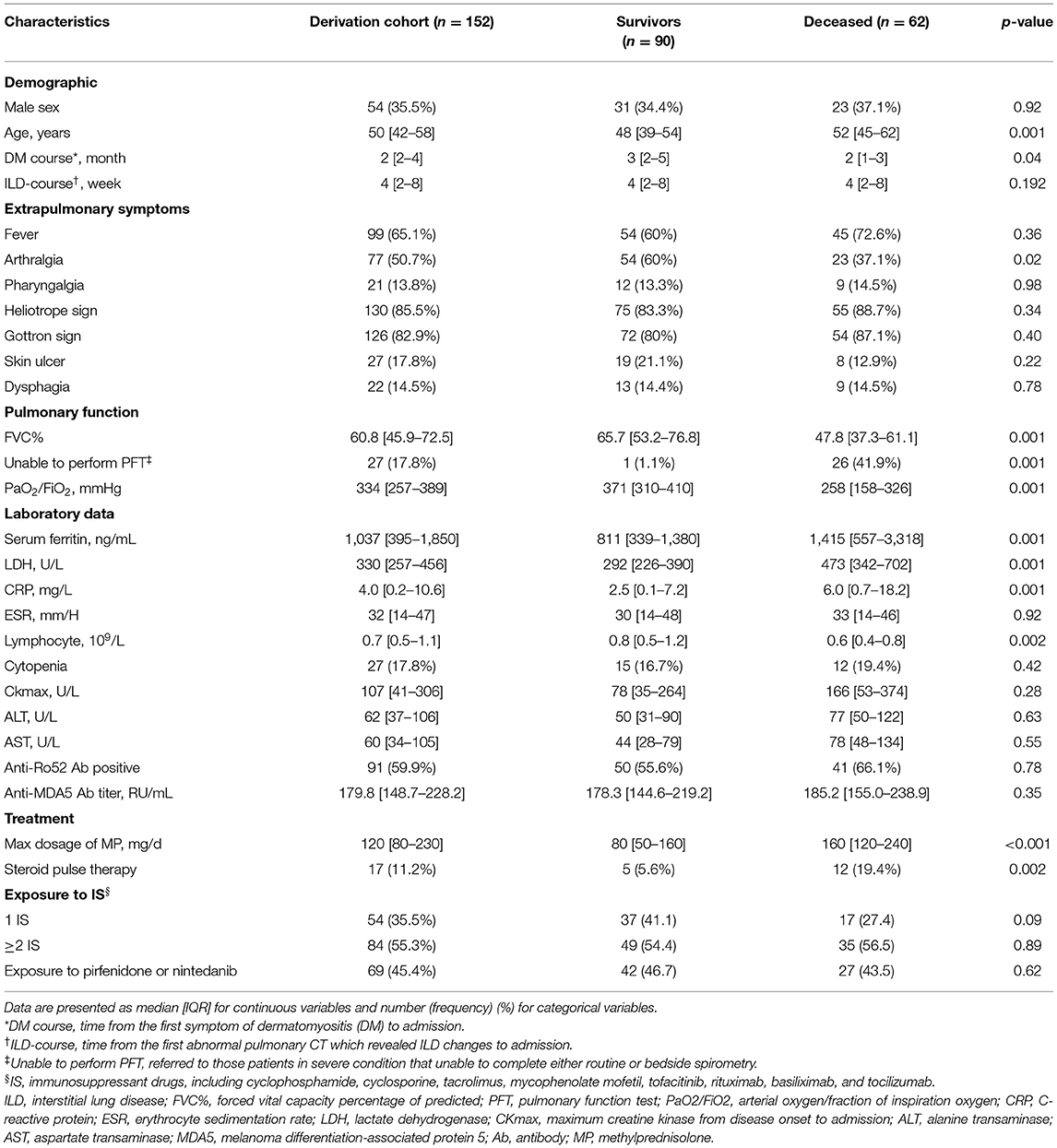
Table 2. Univariable Cox regression results of baseline clinical characteristics and treatment between the survivors and deceased in the derivation cohort.
Radiomics Model and Rad-Score
Eight hundred and twenty-five features remained after reproducibility analysis. Five radiomic features were finally selected for modeling as follows: Original_Shape_Flatness (A), Wavelet.LLL_Glcm_MCC (B), Wavelet.LLH_Glcm_Ldmn (C), Wavelet.HLL_Glszm_LargeAreaHighGrayLevelEmphasis (D), and Wavelet.LLL_Firstorder_Skewness (E).
The contribution of the selected parameters with their regression coefficients was presented in the form of a histogram in Figure 1. Then, the Rad-score was computed using the following formula: A*(0.599) +B*(0.461) +C*(0.408) +D*(−0.543) +E*(−1.19). The C-index values of the radiomics model were 0.83 (95%CI, 0.74–0.91), 0.82 (95%CI, 0.65–0.99), 0.78 (95%CI, 0.64–0.93), and 0.76 (95%CI, 0.59–0.92) for the training, testing, internal validation, and external validation sets, respectively. The GND test showed good calibration abilities in the four datasets. The prediction errors were low, attested by Brier score ranging from 0.12 to 0.19.
The optimal cutoff value for Rad-score was determined as 1, dividing patients into the high-risk group (Rad-score > 1) and low-risk group (Rad-score ≤ 1) with distinct 6-month mortality (Figure 2A). The representative patients' CT images of the two groups are presented in Figures 2B,C, respectively, with corresponding Rad-scores.
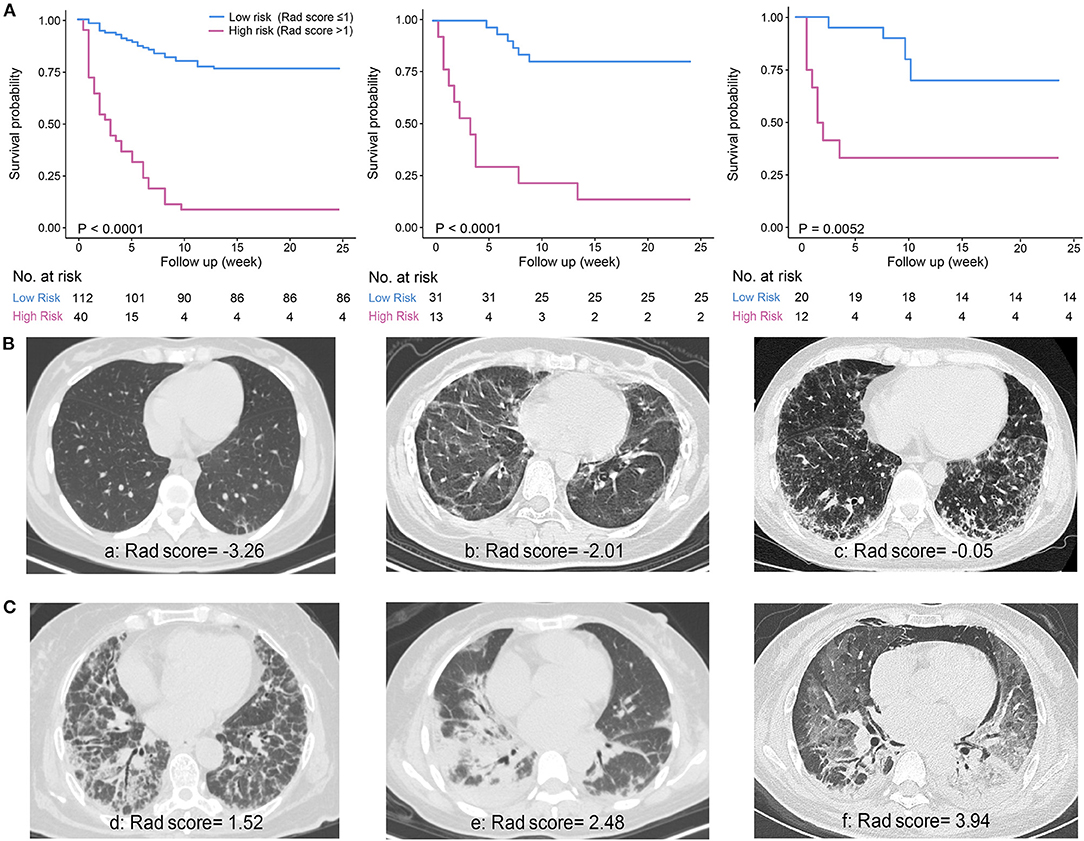
Figure 2. Survival curves of the Rad-score and representative CT images. (A) Significant difference between low-risk (Rad-score ≤ 1) and high-risk (Rad-score > 1) groups was shown in the derivation cohort (n = 152, left) and verified in the internal validation (n = 44, middle) and external validation cohort (n = 32, right). (B) Representative patients' CT images of low-risk group. a: A 32-year-old female patient with discrete subpleural reticulation and ground-glass attenuation (GGA); b: a 23-year-old female patient with typical subpleural lines and GGA; c: a 39-year-old male patient showing a number of GGA and reticulation with some basal consolidation. (C) Representative patients' CT images of high-risk group. d: A 62-year-old female patient with a large amount of reticulation pattern accompanied by GGA and consolidation; e: a 47-year-old female patient with predominant consolidation; f: a 41-year-old female patient with diffuse GGA and consolidation accompanied by pneumomediastinum.
Rad-Score Plus Model
The univariable Cox regression results of baseline clinical characteristics and treatment between the survivors and deceased in the derivation cohort are presented in Table 2. Then, 10 candidate predictors, i.e., with significant differences in univariable analyses, were incorporated into a multivariable Cox proportional hazards model with Rad-score, indicated as follows: age on admission, course of DM, arthralgia, three-category FVC% (FVC% ≥ 50%, FVC% <50%, and unable to perform pulmonary function tests), arterial oxygen/fraction of inspiration oxygen (PaO2/FiO2), lactate dehydrogenase (LDH), serum ferritin (ng/mL), C-reactive protein, lymphocyte, and maximum dosage of methylprednisolone (Table 3).
The optimal combination obtained by the backward stepwise algorithm with minimum AIC was as follows: Rad-score, FVC% (three categories), and age (on admission). The respective hazard ratios and β-regression coefficients are presented in Table 4. The final Rad-score plus model was developed to predict the 6-month mortality with C-index values of 0.88 (95%CI, 0.79–0.96) in the training set, 0.88 (95%CI, 0.71–1.0) in the testing set, 0.83 (95%CI, 0.68–0.98) in the internal validation cohort, and 0.84 (95%CI, 0.64–1.0) in the external validation cohort, respectively. Nomogram was presented online to provide individualized risk estimates (https://mda5-ild.shinyapps.io/MDA_ILD5_test/).
Visual Score and ILD-GAP Model
The agreement of visual CT score between two readers was good with ICC of 0.821 (95%CI, 0.711–0.898). The visual score model yielded the C-index values of 0.75 (95%CI, 0.66–0.84) for the training set, 0.81 (95%CI, 0.65–0.98) for the testing set, and 0.79 (95%CI, 0.65–0.94) for the internal validation cohort, when predicting the 6-month mortality of MDA5+ DM-ILD. However, the discriminative power of visual score dramatically declined in the external validation cohort with the C-index of 0.66 (95%CI, 0.49–0.82) and poor calibration ability. However, the C-index values of ILD-GAP model were relatively even in each dataset (see Table 5 for details).
Comparison of the Predictive Performance of Each Model
The prediction accuracy and robustness of the ILD-GAP score, visual score, radiomics model, and Rad-score plus model are compared in Table 5, with respective calibration curves (Figure 3). The final Rad-score plus model had the best performance surpassing the radiomics model, visual score, and ILD-GAP score (p < 0.001). Finally, the decision curve analysis demonstrated that, in terms of clinical applicability, the Rad-score plus model also had a higher overall net benefit than other models across the majority of the ranges of threshold probabilities (Figure 4).
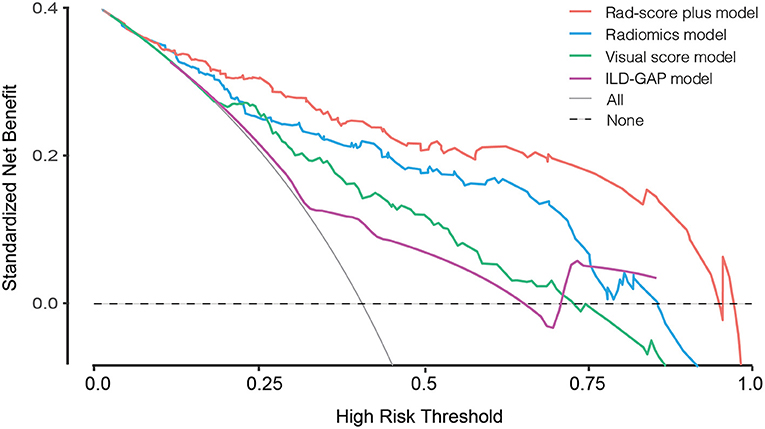
Figure 3. Calibration curves for the visual score model (green line), radiomics model (blue line), Rad-score plus model (red line), and ILD-GAP model (purple line). Calibration curves showed the calibration of each model in terms of the agreement between the predicted and observed 6-month outcomes. Nomogram-predicted outcome was plotted on the x-axis; the observed 6-month survival probability was plotted on the y-axis. Diagonal dotted line referred to perfect estimation by an ideal model, in which the predicted outcome perfectly corresponded to the actual outcome. Solid line referred to performance of the nomogram, a closer lining of which with the diagonal dotted line indicated better estimation.
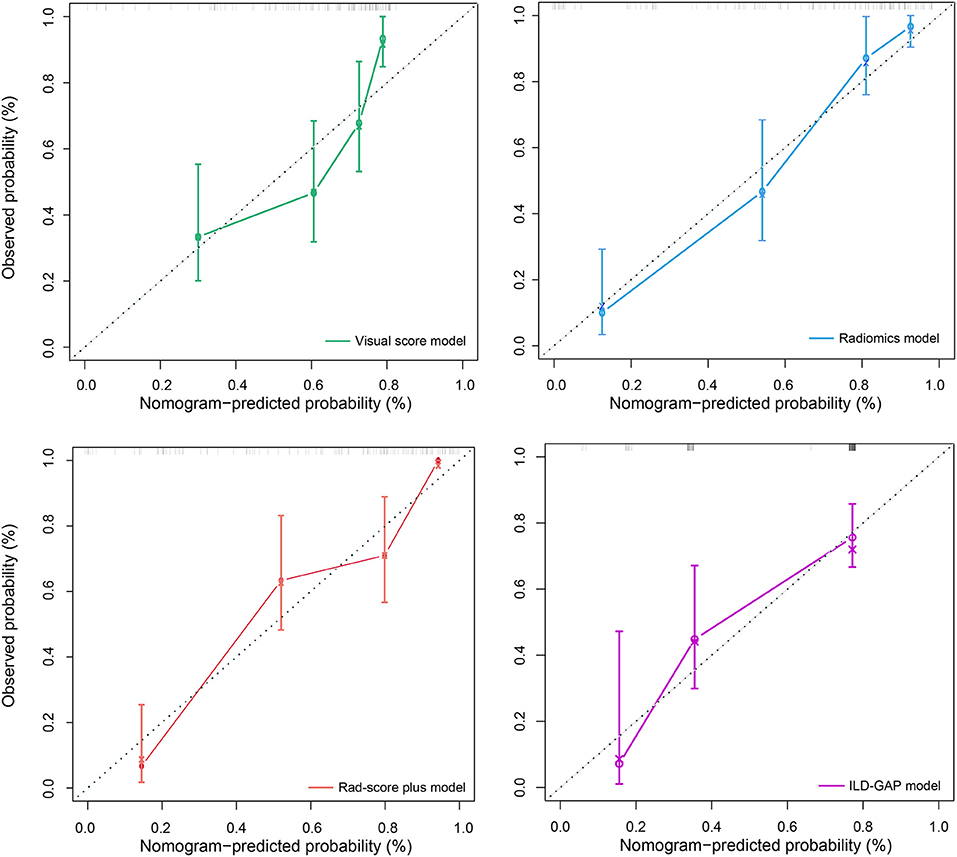
Figure 4. Decision curve analysis for ILD-GAP model, visual score model, Radiomics model, and Rad-score plus model. The concept of population net benefit (NB) is fundamental to decision curves (measured in the y-axis) and referred to classification accuracy of a model. Suppose high risk is defined as risk above some risk threshold R (x-axis), an intervention is recommended in such high-risk patients. The NB of using the risk model was calculated by the true-positive rate (TPRR), the proportion of cases with risk above risk threshold R, and the false-positive rate (FPRR), the proportion of controls with risk above risk threshold R. The horizontal dotted line at NB = 0 indicated a simple policy of no intervention to all patients (treat none); the gray curve in the plot depicted the NB of another simple policy: the intervention was recommended to everyone regardless of risk. In our result, the Rad-score plus model had the highest net benefit compared to others, almost across the full range of threshold probabilities.
In the correlation analysis, the visual score, Rad-score, and Rad-score plus mentioned above all showed strong correlation with FVC and PaO2/FiO2 rather than other clinical predictors (for details, please see Supplementary Table 3).
Subgroup Analysis
Across all datasets, radiomics performed better than visual score in 35 cases, which were defined as Rad-score better group. This subgroup presented a significantly higher mortality, a shorter disease course, a worse pulmonary function, and a higher dosage requirement of steroid (Supplementary Table 2), possibly implying that the radiomics model has a better performance in the patients with more progressive disease course.
Discussion
In the current study, we first applied radiomics analysis in MDA5+ DM-ILD based on a large multicenter cohort. This radiomics approach could not only quantitatively assess the ILD on HRCT, but also excellently predict the prognosis of this tricky disease, surpassing the traditional visual score. Furthermore, the final Rad-score plus model, integrating Rad-score and clinical parameters, showed a superior discriminative performance.
MDA5+ DM-ILD remains to be a great challenge for us despite early diagnosis and recent treatment advances (4, 24). Immunosuppressive therapy has been widely accepted as the cornerstone of treatment for this tricky disease. However, the therapeutic effect of a specific regimen has not been demonstrated depending on risk or severity stratification. Accumulative prognostic factors of the disease have been reported involving aspects of respiratory physiology, laboratory markers, and radiology (2, 5, 7, 25). To the best of our knowledge, the current study is the first attempt to quantitatively assess MDA5+ DM-ILD by applying the computer-based radiomics approach, which enriches the panorama of prognostic prediction for this disease.
Our data illustrated that the Rad-score derived from multiple radiomic features could provide a reliable predictor for the 6-month mortality of MDA5+ DM-ILD, and outperform the visual CT score. It implied that radiomics enhanced the quantification of chest CT images as it provided more comprehensive features to describe the distribution, heterogeneity of CT value, and texture information behind the visual image. Shape and texture features were the predominant components of the Rad-score. The selected shape feature of flatness, which indicated the change in the lung shape during the progression of the disease, had a negative impact on prognosis. The texture features of MCC, IDMN, and Large AreaHighGrayLevelEmphasis, describing the complexity of texture or the distribution of gray level value, implied that the survival outcome was worse with the increasing heterogeneity of the texture. Such heterogeneity might impute to the lesions of GGA, consolidation, and reticulation pattern, which were reported by Hozumi et al. as the most distinctive HRCT patterns for MDA5+ DM-ILD (26). Skewness measured the asymmetry of CT value distribution, which was also discovered to indicate the disease progression of IPF in previous studies (27). Of note, in the population of MDA5+ DM-ILD, the organizing pneumonia pattern was found to be dominant in HRCT, with lower zone consolidation correlating with rapidly progressive ILD (28). Moreover, another study with pathological evidences discovered that a perilobular opacity transforming to consolidation corresponded to diffuse alveolar damage, which was presented as the main finding in MDA5+ DM associated with rapidly progressive ILD (29). Thus, these manifestations might result in the changes of CT values and texture, making these radiomic features important for diagnosis, risk stratification, and prognosis. In addition, the Rad-score performed even better than visual score in a more aggressive subgroup with worse prognosis, supported by our subgroup analysis.
Consistent with our previous study of prognosis on this disease, the clinically relevant parameters were age and FVC% levels in the final Rad-score plus model, and coincidentally, they were also the main components in GAP staging system (18, 30). Moreover, HRCT imaging in combination with pulmonary function test was acknowledged as the gold standard for a pivotal and non-invasive assessment of ILD (31). Thus, it was rational to combine FVC% with Rad-score to further enhance its predictive performance.
The limitations of our study first lie in the retrospective design of data collection and relatively limited sample size of external validation. Notably, the treatment regimens were relatively dissimilar between two centers, with significantly higher intensity of glucocorticoid and immunosuppressant exposure in the external validation cohort. However, the overall mortality and other baseline parameters were comparable between them. The verification of our findings would be better in a larger-scale prospective multicenter validation cohort, by which the biases of different machine conditions, patient selection, and treatment protocols could be minimized and controlled. However, even in tertiary centers, the rarity of this disease is well recognized. Second, since the computer-based radiomic features are known as agnostic features (12) and beyond visual conception, it is difficult to interpret the Rad-score pathophysiologically. Possibly, further work in radiogenomics may help to establish an in-depth link between radiomic changes and pathogenesis. Lastly, the feature extraction was based on the whole lung rather than the visible extent of ILD. Although this could include all information on the lung, it may also dilute the texture features of the affected regions. Further research focused on the affected regions is required.
Conclusions
A quantitative computer-derived radiomic signature was a superior and reliable prognostic predictor for the 6-month mortality in MDA5+ DM-ILD. This instrument may grant a step forward to elegant clinical trial design and precision management of this challenging disease.
Data Availability Statement
The datasets presented in this study can be found in online repositories. The names of the repository/repositories and accession number(s) can be found in the article/Supplementary Material.
Ethics Statement
Written informed consent was obtained from the individual(s) for the publication of any potentially identifiable images or data included in this article.
Author Contributions
WX, WW, QH, YZho, and SY contributed to the project design. WX, WW, ZC, and DZ collected the clinical data of derivation cohort. BG, QL, and QH collected the clinical data of validation cohort. JZ and KW were responsible for laboratory data. YZhe contributed to the assessment of pulmonary function tests. ZC and YZhe conducted visual HRCT evaluation. WX, YZhe, and XT operated the Radiomics software. WX, WW, and XT contributed to analysis of all data. WX, WW, and SY wrote and revised the manuscript. All authors have read and agreed to the final version of the manuscript.
Funding
This study was supported by Clinical Research Plan of SHDC (No. SHDC2020CR1015B).
Conflict of Interest
XT is an employee of Siemens Healthineers.
The remaining authors declare that the research was conducted in the absence of any commercial or financial relationships that could be construed as a potential conflict of interest.
Publisher's Note
All claims expressed in this article are solely those of the authors and do not necessarily represent those of their affiliated organizations, or those of the publisher, the editors and the reviewers. Any product that may be evaluated in this article, or claim that may be made by its manufacturer, is not guaranteed or endorsed by the publisher.
Supplementary Material
The Supplementary Material for this article can be found online at: https://www.frontiersin.org/articles/10.3389/fmed.2021.768052/full#supplementary-material
References
1. Allenbach Y, Uzunhan Y, Toquet S, Leroux G, Gallay L, Marquet A, et al. Different phenotypes in dermatomyositis associated with anti-MDA5 antibody: study of 121 cases. Neurology. (2020) 95:e70–8. doi: 10.1212/WNL.0000000000009727
2. Gono T, Sato S, Kawaguchi Y, Kuwana M, Hanaoka M, Katsumata Y, et al. Anti-MDA5 antibody, ferritin and IL-18 are useful for the evaluation of response to treatment in interstitial lung disease with anti-MDA5 antibody-positive dermatomyositis. Rheumatology. (2012) 51:1563–70. doi: 10.1093/rheumatology/kes102
3. Ye S, Chen XX, Lu XY, Wu MF, Deng Y, Huang WQ, et al. Adult clinically amyopathic dermatomyositis with rapid progressive interstitial lung disease: a retrospective cohort study. Clin Rheumatol. (2007) 26:1647–54. doi: 10.1007/s10067-007-0562-9
4. Tsuji H, Nakashima R, Hosono Y, Imura Y, Yagita M, Yoshifuji H, et al. Multicenter Prospective study of the efficacy and safety of combined immunosuppressive therapy with high-dose glucocorticoid, tacrolimus, and cyclophosphamide in interstitial lung diseases accompanied by anti-melanoma differentiation-associated gene 5-positive dermatomyositis. Arthritis Rheumatol. (2020) 72:488–98. doi: 10.1002/art.41105
5. Wu W, Guo L, Fu Y, Wang K, Zhang D, Xu W, et al. interstitial lung disease in anti-MDA5 positive dermatomyositis. Clin Rev Allergy Immunol. (2021) 60:293–304. doi: 10.1007/s12016-020-08822-5
6. Zou J, Guo Q, Chi J, Wu H, Bao C, HRCT. score and serum ferritin level are factors associated to the 1-year mortality of acute interstitial lung disease in clinically amyopathic dermatomyositis patients. Clin Rheumatol. (2015) 34:707–14. doi: 10.1007/s10067-015-2866-5
7. Lian X, Zou J, Guo Q, Chen S, Lu L, Wang R, et al. Mortality risk prediction in amyopathic dermatomyositis associated with interstitial lung disease: the flair model. Chest. (2020) 158:1535–45. doi: 10.1016/j.chest.2020.04.057
8. Ichikado K, Suga M, Muranaka H, Gushima Y, Miyakawa H, Tsubamoto M, et al. Prediction of prognosis for acute respiratory distress syndrome with thin-section CT: validation in 44 cases. Radiology. (2006) 238:321–9. doi: 10.1148/radiol.2373041515
9. Yamaguchi K, Yamaguchi A, Itai M, Kashiwagi C, Takehara K, Aoki S, et al. Clinical features of patients with anti-melanoma differentiation-associated gene-5 antibody-positive dermatomyositis complicated by spontaneous pneumomediastinum. Clin Rheumatol. (2019) 38:3443–50. doi: 10.1007/s10067-019-04729-5
10. Fujisawa T, Hozumi H, Kono M, Enomoto N, Hashimoto D, Nakamura Y, et al. Prognostic factors for myositis-associated interstitial lung disease. PLoS ONE. (2014) 9:e98824. doi: 10.1371/journal.pone.0098824
11. Xu W, Wu W, Zhang D, Chen Z, Tao X, Zhao J, et al. A novel CT scoring method predicts the prognosis of interstitial lung disease associated with anti-MDA5 positive dermatomyositis. Sci Rep. (2021) 11:17070. doi: 10.1038/s41598-021-96292-w
12. RJ G, PE K, Radiology HHJ. Radiomics: images are more than pictures, they are data. Radiology. (2016) 278:563–77. doi: 10.1148/radiol.2015151169
13. Qiu J, Peng S, Yin J, Wang J, Jiang J, Li Z, et al. Radiomics: the bridge between medical imaging and personalized medicine. Nat Rev Clin Oncol. (2017) 14:749–62. doi: 10.1038/nrclinonc.2017.141
14. Bohan A, Peter JB. Polymyositis and dermatomyositis (first of two parts). N Engl J Med. (1975) 292:344–7. doi: 10.1056/NEJM197502132920706
15. Sontheimer RD. Would a new name hasten the acceptance of amyopathic dermatomyositis (dermatomyositis sine myositis) as a distinctive subset within the idiopathic inflammatory dermatomyopathies spectrum of clinical illness? J Am Acad Dermatol. (2002) 46:626–36. doi: 10.1067/mjd.2002.120621
16. Mammen AL, Allenbach Y, Stenzel W, Benveniste O, Group EtWS. 239th ENMC international workshop: classification of dermatomyositis, Amsterdam, the Netherlands, 14-16 December 2018. Neuromuscul Disord. (2020) 30:70–92. doi: 10.1016/j.nmd.2019.10.005
17. Tibshirani R. The lasso method for variable selection in the Cox model. Stat Med. (1997) 16:385–95. doi: 10.1002/(SICI)1097-0258(19970228)16:4<385::AID-SIM380>3.0.CO;2-3
18. Ley B, Ryerson CJ, Vittinghoff E, Ryu JH, Tomassetti S, Lee JS, et al. A multidimensional index and staging system for idiopathic pulmonary fibrosis. Ann Intern Med. (2012) 156:684–91. doi: 10.7326/0003-4819-156-10-201205150-00004
19. Ryerson C, Vittinghoff E, Ley B, Lee J, Mooney J, Jones K, et al. Predicting survival across chronic interstitial lung disease: the ILD-GAP model. Chest. (2014) 145:723–8. doi: 10.1378/chest.13-1474
20. Berthold DR, Pond GR, Roessner M, De Wit R, Eisenberger M, Tannock IF. X-tile: a new bio-informatics tool for biomarker assessment and outcome-based cut-point optimization Clinical cancer research: an official journal of the American Association for Cancer Research. Clic Cancer Res. (2004) 10:7252–9. doi: 10.1158/1078-0432.CCR-04-0713
21. Kattan MW. Judging new markers by their ability to improve predictive accuracy. J Natl Cancer Inst. (2003) 95:634–5. doi: 10.1093/jnci/95.9.634
22. Demler OV, Paynter NP, Cook NR. Tests of calibration and goodness-of-fit in the survival setting. Stat Med. (2015) 34:1659–80. doi: 10.1002/sim.6428
23. KF K, MD B. K Z, Oncology JHJJocoojotASoC. Assessing the clinical impact of risk prediction models with decision curves: guidance for correct interpretation and appropriate use. J Clin Oncol. (2016) 34:2534–40. doi: 10.1200/JCO.2015.65.5654
24. Chen Z, Wang X, Ye S. Tofacitinib in amyopathic dermatomyositis-associated interstitial lung disease. N Engl J Med. (2019) 381:291–3. doi: 10.1056/NEJMc1900045
25. Wang K, Zhao J, Chen Z, Li T, Tan X, Zheng Y, et al. CD4+CXCR4+ T cells as a novel prognostic biomarker in patients with idiopathic inflammatory myopathy-associated interstitial lung disease. Rheumatology. (2019) 58:511–21. doi: 10.1093/rheumatology/key341
26. Hozumi H, Fujisawa T, Nakashima R, Johkoh T, Sumikawa H, Murakami A, et al. Comprehensive assessment of myositis-specific autoantibodies in polymyositis/dermatomyositis-associated interstitial lung disease. Respir Med. (2016) 121:91–9. doi: 10.1016/j.rmed.2016.10.019
27. Best A, Meng J, Lynch A, Bozic C, Miller D, Grunwald G, et al. Idiopathic pulmonary fibrosis: physiologic tests, quantitative CT indexes, and CT visual scores as predictors of mortality. Radiology. (2008) 246:935–40. doi: 10.1148/radiol.2463062200
28. Zuo Y, Ye L, Liu M, Li S, Liu W, Chen F, et al. Clinical significance of radiological patterns of HRCT and their association with macrophage activation in dermatomyositis. Rheumatology. (2020) 59:2829–37. doi: 10.1093/rheumatology/keaa034
29. Chino H, Sekine A, Baba T, Kitamura H, Iwasawa T, Okudela K, et al. interstitial lung disease with anti-melanoma differentiation-associated protein 5 antibody: rapidly progressive perilobular opacity. Intern Med. (2019) 58:2605–13. doi: 10.2169/internalmedicine.2328-18
30. Wu W, Xu W, Sun W, Zhang D, Zhao J, Luo Q, et al. Forced vital capacity predicts the survival of interstitial lung disease in anti-MDA5 positive dermatomyositis: a multi-centre cohort study. Rheumatology. (2021) 21:305. doi: 10.1093/rheumatology/keab305
Keywords: anti-melanoma differentiation-associated gene 5, dermatomyositis, interstitial lung disease, prognosis, radiomics
Citation: Xu W, Wu W, Zheng Y, Chen Z, Tao X, Zhang D, Zhao J, Wang K, Guo B, Luo Q, Han Q, Zhou Y and Ye S (2021) A Computed Tomography Radiomics-Based Prediction Model on Interstitial Lung Disease in Anti-MDA5-Positive Dermatomyositis. Front. Med. 8:768052. doi: 10.3389/fmed.2021.768052
Received: 31 August 2021; Accepted: 22 October 2021;
Published: 29 November 2021.
Edited by:
Chris Wincup, University College London, United KingdomReviewed by:
Albert Selva-O'Callaghan, Vall D'Hebron University Hospital, SpainJin Woo Song, Asan Medical Center, South Korea
Latika Gupta, Sanjay Gandhi Postgraduate Institute of Medical Sciences, India
Copyright © 2021 Xu, Wu, Zheng, Chen, Tao, Zhang, Zhao, Wang, Guo, Luo, Han, Zhou and Ye. This is an open-access article distributed under the terms of the Creative Commons Attribution License (CC BY). The use, distribution or reproduction in other forums is permitted, provided the original author(s) and the copyright owner(s) are credited and that the original publication in this journal is cited, in accordance with accepted academic practice. No use, distribution or reproduction is permitted which does not comply with these terms.
*Correspondence: Shuang Ye, ye_shuang2000@163.com; Yan Zhou, clare1475@hotmail.com; Qian Han, hanqian1020@yahoo.com
†These authors have contributed equally to this work and share first authorship