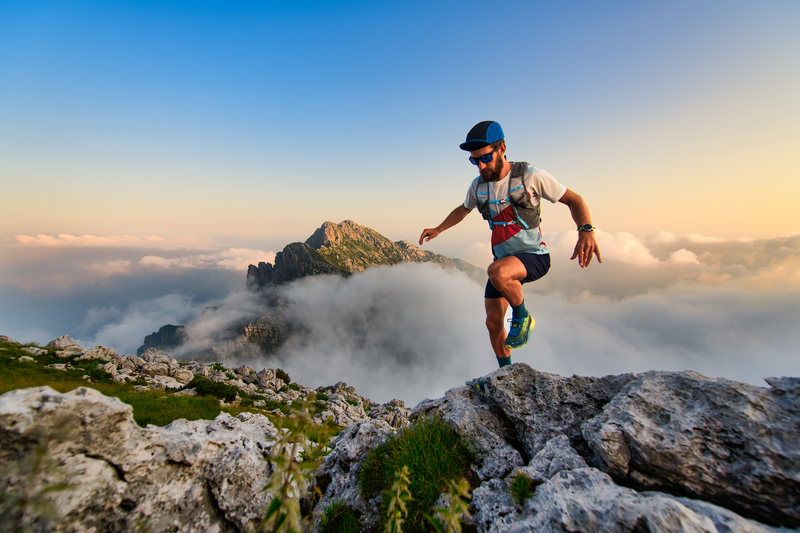
94% of researchers rate our articles as excellent or good
Learn more about the work of our research integrity team to safeguard the quality of each article we publish.
Find out more
ORIGINAL RESEARCH article
Front. Med. , 12 November 2021
Sec. Intensive Care Medicine and Anesthesiology
Volume 8 - 2021 | https://doi.org/10.3389/fmed.2021.752508
This article is part of the Research Topic Acute Respiratory Distress Syndrome and Mechanical Ventilation View all 15 articles
Background: Patient-ventilator asynchrony is common during pressure support ventilation (PSV) because of the constant cycling-off criteria and variation of respiratory system mechanical properties in individual patients. Automatic adjustment of inspiratory triggers and cycling-off criteria based on waveforms might be a useful tool to improve patient-ventilator asynchrony during PSV.
Method: Twenty-four patients were enrolled and were ventilated using PSV with different cycling-off criteria of 10% (PS10), 30% (PS30), 50% (PS50), and automatic adjustment PSV (PSAUTO). Patient-ventilator interactions were measured.
Results: The total asynchrony index (AI) and NeuroSync index were consistently lower in PSAUTO when compared with PS10, PS30, and PS50, (P < 0.05). The benefit of PSAUTO in reducing the total AI was mainly because of the reduction of the micro-AI but not the macro-AI. PSAUTO significantly improved the relative cycling-off error when compared with prefixed controlled PSV (P < 0.05). PSAUTO significantly reduced the trigger error and inspiratory effort for the trigger when compared with a prefixed trigger. However, total inspiratory effort, breathing patterns, and respiratory drive were not different among modes.
Conclusions: When compared with fixed cycling-off criteria, an automatic adjustment system improved patient-ventilator asynchrony without changes in breathing patterns during PSV. The automatic adjustment system could be a useful tool to titrate more personalized mechanical ventilation.
Pressure support ventilation (PSV) is the most widely used partial mode of assistance to minimize the effort of patients in breathing. During PSV, the assist is delivered by means of a pneumatic signal generated by patient effort and measured in the ventilatory circuit, i.e., flow or pressure (1). The ventilator usually cycles from inspiration to expiration when the inspiratory flow falls to a predetermined fraction of the peak inspiratory flow, which is the cycling-off criterion (2). Ideally, the ventilator trigger and cycling should coincide with the beginning and the end of the inspiratory effort of the patients (3). However, patient-ventilator asynchrony is common during PSV (4, 5), thereby contributing to the increased patient effort, increased duration of mechanical ventilation, and even increased mortality (6).
During PSV, prefixed pneumatic controllers can become progressively less effective, especially when patients have abnormal respiratory mechanics or ventilator over-assist (7). Delayed or missed triggers are sensed as an uncomfortable isometric load leading to increased effort intensity and pronounced dyspnea (8). Moreover, with prefixed cycling-off criteria, such as the default value of 30% peak flow in some ventilators, premature cycling is more frequent in patients with restrictive breathing patterns characterized by low respiratory system compliance and may result in double triggering. Delayed cycling occurs more frequently in patients with an obstructive pattern characterized by high resistance (6, 9). Different approaches for optimal ventilator triggering and cycling have been developed to minimize these problems, such as flow-triggering sensitivity and adjustable flow cycling during PSV.
It has been demonstrated that a noninvasive method based on flow and airway-pressure tracings was effective for detecting asynchrony (10–12). Therefore, an automatic adjustment system (IntelliCycleTM2.0) capable of automatically adjusting, breath by breath, the triggering and cycling-off criteria based on pressure-time and flow-time waveforms during PSV have been developed (see Supplementary Material).
The objective of our study was to show a reduction in patient-ventilator asynchrony with the use of an automatic adjustment system as compared with prefixed trigger and cycling-off criteria in patients with PSV.
This unblinded crossover study was conducted in a 60-bed general intensive care unit of a teaching hospital affiliated with Southeast University in China. The protocol was approved by the Institutional Ethics Committee of Zhongda Hospital (number 2016ZDSYLL067-P01). Written informed consent was obtained from the legal primary decision-maker, which was the spouse of the patient or the parent or child if no spouse. The trial was registered at clinicaltrials.gov (NCT04091269).
Postoperative (abdominal surgery or orthopedic surgery) or acute respiratory failure patients were eligible when meeting all the following criteria: receiving invasive mechanical ventilation and being able to sustain PSV more than 1 h with inspiratory support ≤ 15 cm H2O. Patients were excluded if (1) age <18 or >85 years; (2) tracheostomy at time of the study; (3) sedation level on the Richmond Agitation–Sedation Scale ≤ −2 or ≥ 2; (4) contraindication for nasogastric tube insertion, e.g., history of esophageal varices, gastroesophageal surgery in the previous 12 months, or gastroesophageal bleeding in the previous 7 days, international standard ratio > 1.5, activated partial thromboplastin time > 44 s, history of leukemia (13); and (5) hemodynamic instability (heart rate > 140 beats/min, vasopressors required with ≥ 5 μg/kg/min dopamine/dobutamine, or ≥ 0.2 μg/kg/min norepinephrine).
After obtaining consent, enrolled patients were switched to a Servo-i ventilator (Maquet, Solna, Stockholm, Sweden). A 16-F nasogastric feeding tube (NeuroVent Research Inc., Toronto, ON, Canada) with electrodes measuring the electrical activity of the diaphragm (EAdi) and balloons measuring esophageal (Pes) pressures was inserted through the nose and secured after confirming positioning according to the recommendations of the manufacturer. Static respiratory system compliance (CRS), resistance (RRS), and intrinsic positive end-expiratory pressure (PEEPi) were measured during volume control ventilation (without spontaneous drive) (see Supplementary Material).
Then sedation was decreased to maintain light sedation with the Richmond Agitation–Sedation Scale ranging from 0 to −2. As spontaneous breathing and EAdi recovered, patients were switched to an SV800 ventilator with IntelliCycleTM2.0 which can automatically adjust triggering and cycling-off criteria breath-by-breath, (Mindray, Shenzhen, China) and were ventilated by PSV with the pressure support level adjusted to a target tidal volume (VT) of 6 ml/kg (of predict body weight, PBW). During the entire recording period, PEEPe and a fraction of inspired oxygen (FiO2) were maintained as set by the clinician in charge of the patient.
During prefixed pneumatically controlled PSV, the inspiratory trigger was set at 1.5 L/min for flow triggering, and the rate of rise in pressure was set to 0.05 s in all patients. The cycling-off criteria were set to 10% (PS10), 30% (PS30), and 50% (PS50). During automatic adjustment PSV, the inspiratory trigger was set as flow-trigger 1.5 L/min, the rate of rise in pressure was set to 0.05 s, and the cycling-off criterion was set to “AUTO” (PSAUTO). Both the trigger and cycling-off criteria were adjusted by the automatic adjustment system according to an established algorithm based on the pressure-time and flow-time waveforms (Supplementary Figures 1, 2). First, patients were ventilated with four independent modes (PS10, PS30, PS50, and PSAUTO) applied in randomized order (Supplementary Table 1). Each independent condition was maintained for 20 min without washout periods (Supplementary Figure 3).
Flow, airway pressure (Paw), esophageal pressure (Pes), and EAdi were acquired during the 20-min time window in each condition at 100 Hz from the ventilator via an RS 232 interface connected to a computer. Data were stored for later offline analysis (NeuroVent Research Inc., Toronto, ON, Canada). To quantify patient-ventilator interaction, all variables were calculated manually breath by breath from a stable 3-min period in each condition using customized software (NeuroVent Research Inc., Toronto, ON, Canada) by two independent researchers who were blinded to the patient number and assigned order of crossover treatments, and mean values were calculated. In the event of a mismatch, a third researcher was consulted.
Six types of asynchrony were analyzed as previously described by Thille et al. and Lamouret et al. (6, 14). Macro asynchronies include ineffective triggering, which is defined by the existence of a diaphragmatic signal without a respiratory cycle; auto-triggering is defined by the existence of a ventilator cycle without a diaphragmatic signal; and double triggering is defined by the presence of two successive inspiratory cycles without an intermediate expiration or with an interrupted expiration. Micro-asynchronies are defined by a time difference exceeding 200 ms between the onset of the EAdi and the early initial rise in Paw; between the 70% of peak EAdi and early decrease in airway pressure (the opening of the expiratory valve)-late cycling; and between the decrease in airway pressure and 70% of peak EAdi-premature cycling. For each subtype of asynchrony, a percentage of asynchronies was calculated as follows: the number of asynchrony events divided by the total neural respiratory rate (which corresponds to the total EAdi signals) × 100%. Macro-asynchrony index (AI), micro-AI, and total AI were calculated as the number of macro asynchrony events, micro-asynchrony, or total asynchrony events divided by the neural respiratory rate × 100%.
Triggering and cycling-off errors, which were classified as either too late (positive values) or too early (negative values) (13), breathing pattern, inspiratory effort, and inspiratory effort for triggering were measured (see Supplementary Material). To estimate the overall extent of asynchrony and dys-synchrony, the NeuroSync index was calculated by averaging the percentage errors in triggering and cycling-off for all breaths (13). The primary endpoint was the difference in the total AI between PSAUTO and PSV with prefixed triggering and cycling-off criteria (PS10, PS30, and PS50).
All statistical analyses were carried out using SPSS 20 (Chicago, IL, USA). The values are stated as mean ± SD unless specified otherwise. Data from two post-hoc subgroups, a restrictive subgroup defined as having CRS < 40 ml/cm H2O with RRS < 12 cm H2O/LS, and an obstructive subgroup, defined as having RRS > 12 cm H2O/LS with CRS > 40 ml/cm H2O, were analyzed. The normal distribution of continuous variables was assessed by using the Shapiro–Wilk test. Log-transformation was used for skewed data. Variables were compared between modes using repeated-measures ANOVA followed by Bonferroni's post-hoc test. Categorical data were compared by the chi-square test followed by Bonferroni's post-hoc test. P-values <0.05 were considered significant.
The study included 24 patients, such as eight patients in the restrictive subgroup, eight patients in the obstructive subgroup, and eight other patients without obvious acute respiratory failure (CRS > 40 ml/cm H2O with RRS < 12 cm H2O/LS). Patient characteristics and lung mechanism are summarized in Table 1.
Total AI was consistently lower in PSAUTO when compared with PS10, PS30, and PS50, (P < 0.05). The benefit of PSAUTO in reducing total AI was mainly in the reduction of micro-AI but not macro-AI (Figure 1). The percentages of all kinds of asynchronies are reported in Table 2. Total AI and micro-AI were lower in PSAUTO when compared with PS10 and PS30 in the obstructive subgroup and were lower in PSAUTO when compared with PS50 in the restrictive subgroup (Supplementary Table 2).
Figure 1. Total AI (A), macro-AI (B), and micro-AI (C) in different modes. AI, asynchrony index; PS10, pressure support ventilation with cycling-off criteria set to 10%; PS30, pressure support ventilation with cycling-off criteria set to 30%; PS50, pressure support ventilation with cycling-off criteria set to 50%; PSAUTO, pressure support ventilation with automatic. Gray lines showed median (interquartile range). Compared with PS10, aP < 0.05; Compared with PS30, bP < 0.05; compared with PS50, cP < 0.05.
Table 2. Asynchronies, NeuroSync index, inspiratory effort, and relative timing errors of cycling-off and trigger in different modes.
The NeuroSync index (average of the percentage errors of triggering and cycling-off) was consistently lower in PSAUTO when compared with PS10, PS30, and PS50, indicating improved patient-ventilator interaction (Table 2). Figure 2 shows a plot of the percentage errors of triggering (X-axis) and cycling-off (Y-axis) for every breath. We have inserted a small centered box suggesting “perfect” asynchrony to be ≤10% of neural timing and a larger box suggesting “acceptable” asynchrony to be ≤33% of neural timing (15). There were more “Perfect” asynchrony breaths and “Acceptable” asynchrony breaths in PSAUTO than in the fixed cycling-off criteria mode (PS10, PS30, and PS50, all P < 0.05; Figure 2).
Figure 2. Breath density graph for relative trigger (X-axis) and cycling-off (Y-axis) errors, for all breaths in all patients, during each ventilator mode. PS10, pressure support ventilation with cycling-off criteria set to 10%; PS30, pressure support ventilation with cycling-off criteria set to 30%; PS50, pressure support ventilation with cycling-off criteria set to 50%; PSAUTO, pressure support ventilation with automatic adjustment system; asynchrony error, breathes inside the box of percentage error of neural timings.
Automatic adjustment PSV significantly improved the relative cycling-off error when compared with PS10, PS30, and PS50 in the whole population (Figure 3). The relative cycling-off error in PSAUTO was comparable with that in PS50 in the obstructive subgroup and was comparable with that in PS10 in the restrictive subgroup. PSAUTO significantly shortened the absolute and relative triggering errors when compared with a prefixed trigger (PS10, PS30, or PS50; Figure 3). The Absolute and relative triggering errors were significantly lower when compared with PS10, PS30, and PS50 in the obstructive subgroup but not in the restrictive subgroup (Supplementary Figure 4).
Figure 3. Cycling-off error and trigger error in different modes. (A) cycling-off error, (B) relative cycling-off error, (C) trigger error, and (D) relative trigger error, Y-axis for cycling-off error: positive values indicate late cycling-off, and negative values indicate early cycling off. Magenta line showed median (interquartile range). ms, millisecond; PS10, pressure support ventilation with cycling-off criteria set to 10%; PS30, pressure support ventilation with cycling-off criteria set to 30%; PS50, pressure support ventilation with cycling-off criteria set to 50%; PSAUTO, pressure support ventilation with automatic. Compared with PS10, aP < 0.05; Compared with PS30, bP < 0.05; Compared with PS50, cP < 0.05.
Inspiratory effort for triggering determined by PTPes−trig was significantly lower in PSAUTO when compared in PS10 and PS30; however, total inspiratory effort determined by PTPes was not different among modes (Table 3). In the obstructive subgroup, PTPes−trig was significantly lower in PSAUTO than in PS10, PS30, and PS50 (P < 0.05; Supplementary Table 2). Peak airway pressure was higher in PS10 than in other modes. There was no difference in the respiratory drive between modes (Table 3). Breathing patterns and respiratory drive in obstructive and restrictive subgroups are shown in Supplementary Table 3.
This study showed that when compared with PSV with prefixed pneumatic controllers, an automatic adjustment system decreased total AI and improved patient-ventilator interaction mainly through a decrease of micro-asynchronies. The automatic system was associated with the lower cycling-off error, triggering error, and triggering effort in PSV patients.
Both AI and the NeuroSync index are indicators that reflect the overall patient-ventilator interaction from different perspectives. PSAUTO constantly reduced total AI and the NeuroSync index when compared with PSV with prefixed pneumatic controllers, indicating improved patient-ventilator interaction. Given that macro-asynchronies were rare, the benefit of PSAUTO in reducing the total AI was mainly due to the reduction of micro-asynchronies. These findings agree with previous work comparing PSV and neurally adjusted ventilatory assist, which showed the difference in AI is found only in micro-asynchronies (14).
The present study showed a higher total AI (median of 23–57% during PS10, PS30, PS50, and PSAUTO) when compared with those in previous studies (range from 0 to 27%) (6, 16, 17). Despite the differences among study patients and ventilators used, the major reason for the apparent differences between studies might relate to the calculation method for the AI. First, inspiratory trigger delay was included in the calculation of the AI in the present study, which provided about one-third to one-half of the total AI during PSV with prefixed pneumatic controllers. However, the previous study did not calculate inspiratory trigger delay in the AI (6). Second, we defined asynchrony as an error of 200 ms between the origin of the EAdi and ventilator insufflation, which was more sensitive than the threshold used in previous studies (6, 16–18). Therefore, the AI in the present study is more sensitive and comprehensive and therefore not comparable to those in other studies.
Cycling-off asynchrony is dependent on factors, such as the inspiratory effort, neural inspiratory time, assist levels, the time constant of the respiratory system, and cycling-off criteria of the patients (3). Consequently, the optimum flow cycling-off criteria vary among patients and can range from very low levels (5%) in patients with a restrictive condition (such as acute respiratory distress syndrome) (4, 19) to more than 50% in patients with an obstructive condition (such as chronic obstructive pulmonary disease) (5, 20, 21). A previous study showed in a mixed sample of patients that the use of a variable, real-time-adjusted termination criterion improved some indices of patient-ventilator asynchrony when compared with a fixed termination criterion (5% of peak inspiratory flow) (22). However, a termination criterion of 5% of peak inspiratory flow was not commonly used clinically during PSV. Our results showed a significant improvement in relative cycling-off error during PSAUTO when compared with PSV with prefixed cycling-off criteria of 10%, 30%, and 50%. It was not unexpected that during PSV with prefixed pneumatic controllers, PS50 and PS10 were the “best” cycling-off settings with the lowest relative cycling-off errors in the obstructive and restrictive subgroups. In each subgroup, relative cycling-off error in PSAUTO was comparable with the “best” cycling-off setting during PSV with a prefixed cycling-off.
The present study showed the median delay for triggering during PS10, PS30,PS50, and PSAUTO ranged from 187 to 130 ms. These values fall within the 80–540 ms range of values previously reported for PSV (1, 18, 23). Beloncle et al. reported absolute values for trigger delay <200 ms in almost all patients, which was lower than that reported in the present study (18). The different ventilators and flow-trigger used in different studies might be one reason, and different types of the enrolled patients might be another reason for the difference in trigger delay. During PSAUTO, the algorithm will trigger the ventilator to initiate the inspiratory phase when it detects a sudden increase of flow waveform, which reflects the inspiratory effort, leading to a reduced triggering delay. Furthermore, triggering delay was likely reduced as a consequence of reduced cycling-off delay during PSAUTO, which led to a longer expiration time and lower PEEPi, especially in patients with obstructive conditions (5). Unfortunately, we did not measure PEEPi during each mode.
Of note, a single flow-trigger level in the present study made it hard to draw conclusions regarding the effect of the PSAUTO mode on inspiratory triggering asynchronies when compared with lower flow-triggering (e.g., 1.0 L/min). From this perspective, a fixed flow-trigger of 1.5 L/min might be not sensitive enough. Considering the similar or shorter triggering delay and no obvious auto-triggering during PSAUTO, automatic adjustment of triggering based on waveforms might be a useful tool for making the triggering setting easier.
Because PSAUTO significantly reduced triggering delay, it was not unexpected that it was associated with lower inspiratory effort for triggering. The present study showed a comparable breathing pattern and respiratory drive between PSAUTO and PSV with prefixed pneumatic controllers. Of interest, neural expiratory time remained unchanged at the various cycling-off settings in the present study. These findings agree with previous work in which expiratory time did not change with the increase in cycling-off criteria in chronic obstructive pulmonary disease patients (5, 20). However, the findings contradict those in previous studies which show an increased expiratory time in the presence of delayed cycling in acute lung injury (24, 25). Therefore, PSAUTO improved the cycling-off criteria, which was demonstrated to affect the inspiratory time only at high-pressure support (20). Peak EAdi around 12 μV confirmed the absence of over-assistance during PSV in the present study.
There are some limitations that should be noted. First, our study was conducted in a small group of patients. Second, respiratory mechanics were evaluated in patients under sedation who were not actively breathing, therefore, the results will be different from those measured during PSV. Third, patients were maintained at each mode setting for only 20 min, and steady-state conditions might not have been achieved. However, the duration was in line with that of several studies on the effects of cycling criteria modifications (4, 19).
An automatic adjustment system based on waveform was associated with less patient-ventilator asynchrony when compared with PSV with prefixed pneumatic controllers. Our results indicated that this system might be a useful tool to titrate more personalized mechanical ventilation, especially in patients with a high risk of patient-ventilator asynchrony.
The raw data supporting the conclusions of this article will be made available by the authors, without undue reservation.
The studies involving human participants were reviewed and approved by Institutional Ethics Committee of Zhongda Hospital. The patients/participants provided their written informed consent to participate in this study.
LL, YYa, DC, and HQ have given substantial contributions to the conception or the design of the manuscript. LL, YYu, XX, and QS to acquisition, analysis, and interpretation of the data. All authors contributed equally to the manuscript and read and approved the final version of the manuscript.
This study was supported by the Clinical Science and Technology Specific Projects of Jiangsu Province [BE2020786 and BE2019749], the National Natural Science Foundation of China [Grant Numbers 81870066 and 81670074], the Natural Science Foundation of Jiangsu Province (BK20171271), Jiangsu Provincial Medical Youth Talent (QNRC 2016807), and Third Level Talents of the 333 High Level Talents Training Project in the fifth phase in Jiangsu (LGY2016051). This study received funding from Mindray (China). The funder was not involved in the study design, collection, analysis, interpretation of data, the writing of this article, or the decision to submit it for publication.
LL and HQ received a grant from Mindray (China).
The remaining authors declare that the research was conducted in the absence of any commercial or financial relationships that could be construed as a potential conflict of interest.
All claims expressed in this article are solely those of the authors and do not necessarily represent those of their affiliated organizations, or those of the publisher, the editors and the reviewers. Any product that may be evaluated in this article, or claim that may be made by its manufacturer, is not guaranteed or endorsed by the publisher.
The authors thank Mr. Jinglei Liu, Xiaoyong Zhou, and Jun Chen (Shenzhen Mindray Bio-Medical Electronics Co., Ltd.) for helping to making the figures.
The Supplementary Material for this article can be found online at: https://www.frontiersin.org/articles/10.3389/fmed.2021.752508/full#supplementary-material
1. Spahija J, Marchie M, Albert M, Bellemare P, Delisle S, Beck J, et al. Patient-ventilator interaction during pressure support ventilation and neurally adjusted ventilatory assist. Crit Care Med. (2010) 38:518–26. doi: 10.1097/CCM.0b013e3181cb0d7b
2. MacIntyre NR. Clinically available new strategies for mechanical ventilatory support. Chest. (1993) 104:560–5. doi: 10.1378/chest.104.2.560
3. Yamada Y, Du HL. Analysis of the mechanisms of expiratory asynchrony in pressure support ventilation: a mathematical approach. J Appl Physiol. (2000) 88:2143–50. doi: 10.1152/jappl.2000.88.6.2143
4. Tokioka H, Tanaka T, Ishizu T, Fukushima T, Iwaki T, Nakamura Y, et al. The effect of breath termination criterion on breathing patterns and the work of breathing during pressure support ventilation. Anesth Analg. (2001) 92:161–5. doi: 10.1097/00000539-200101000-00031
5. Tassaux D, Gainnier M, Battisti A, Jolliet P. Impact of expiratory trigger setting on delayed cycling and inspiratory muscle workload. Am J Respir Crit Care Med. (2005) 172:1283–9. doi: 10.1164/rccm.200407-880OC
6. Thille AW, Rodriguez P, Cabello B, Lellouche F, Brochard L. Patient-ventilator asynchrony during assisted mechanical ventilation. Intensive Care Med. (2006) 32:1515–22. doi: 10.1007/s00134-006-0301-8
7. Jubran A, Van de Graaff WB, Tobin MJ. Variability of patient-ventilator interaction with pressure support ventilation in patients with chronic obstructive pulmonary disease. Am J Respir Crit Care Med. (1995) 152:129–36. doi: 10.1164/ajrccm.152.1.7599811
8. Gilstrap D, MacIntyre N. Patient-ventilator interactions. Implications for clinical management. Am J Respir Crit Care Med. (2013) 188:1058–68. doi: 10.1164/rccm.201212-2214CI
9. Garofalo E, Bruni A, Pelaia C, Liparota L, Lombardo N, Longhini F, et al. Recognizing, quantifying and managing patient-ventilator asynchrony in invasive and noninvasive ventilation. Expert Rev Respir Med. (2018) 12:557–67. doi: 10.1080/17476348.2018.1480941
10. Thille AW, Brochard L. Promoting patient-ventilator synchrony. Clin Pulm Med. (2007) 14:350–9. doi: 10.1097/CPM.0b013e31815b1234
11. Giannouli E, Webster K, Roberts D, Younes M. Response of ventilator-dependent patients to different levels of pressure support and proportional assist. Am J Respir Crit Care Med. (1999) 159:1716–25. doi: 10.1164/ajrccm.159.6.9704025
12. Mellott KG, Grap MJ, Munro CL, Sessler CN, Wetzel PA. Patient-ventilator dysasynchrony: clinical significance and implications for practice. Crit Care Nurse. (2009) 29:41–55. doi: 10.4037/ccn2009612
13. Liu L, Xia F, Yang Y, Longhini F, Navalesi P, Beck J. Neural versus pneumatic control of pressure support in patients with chronic obstructive pulmonary diseases at different levels of positive end expiratory pressure: a physiological study. Crit Care. (2015) 19:244. doi: 10.1186/s13054-015-0971-0
14. Lamouret O, Crognier L, Vardon BF, Conil JM, Dilasser C, Raimondi T, et al. Neurally adjusted ventilatory assist (NAVA) versus pressure support ventilation: patient-ventilator interaction during invasive ventilation delivered by tracheostomy. Crit. Care. (2019) 23:22. doi: 10.1186/s13054-018-2288-2
15. Sinderby C, Liu S, Colombo D, Camarotta G, Slutsky AS, Navalesi P, et al. An automated and standardized neural index to quantify patient-ventilator interaction. Crit Care. (2013) 17:R239. doi: 10.1186/cc13063
16. Demoule A, Clavel M, Rolland-Debord C, Perbet S, Terzi N, Kouatchet A, et al. Neurally adjusted ventilatory assist as an alternative to pressure support ventilation in adults: a French multicenter randomized trial. Intensive Care Med. (2016) 42:1723–32. doi: 10.1007/s00134-016-4447-8
17. Yonis H, Crognier L, Conil JM, Serres I, Rouget A, Virtos M, et al. Patient-ventilator asynchrony in neurally adjusted ventilatory assist (NAVA) and pressure support ventilation (PSV): a prospective observational study. BMC Anesthesiol. (2015) 15:117. doi: 10.1186/s12871-015-0091-z
18. Beloncle F, Piquilloud L, Rittayamai N, Sinderby C, Rozé H, et al. A diaphragmatic electrical activity-based optimization strategy during pressure support ventilation improves synchronization but does not impact work of breathing. Crit Care. (2017) 21:21. doi: 10.1186/s13054-017-1599-z
19. Chiumello D, Pelosi P, Taccone P, Slutsky A, Gattinoni L. Effect of different inspiratory rise time and cycling off criteria during pressure support ventilation in patients recovering from acute lung injury. Crit Care Med. (2003) 31:2604–10. doi: 10.1097/01.CCM.0000089939.11032.36
20. Chiumello D, Polli F, Tallarini F, Chierichetti M, Motta G, Azzari S, et al. Effect of different cycling-off criteria and positive end-expiratory pressure during pressure support ventilation in patients with chronic obstructive pulmonary disease. Crit. Care Med. (2007) 35:2547–52. doi: 10.1097/01.CCM.0000287594.80110.34
21. Tassaux D, Michotte JB, Gainnier M, Gratadour P, Fonseca S, Jolliet P. Expiratory trigger setting in pressure support ventilation: from mathematical model to bedside. Crit Care Med. (2004) 32:1844–50. doi: 10.1097/01.CCM.0000138561.11634.6F
22. Hoff FC, Tucci MR, Amato MBP, Santos LJ, Victorino JA. Cycling-off modes during pressure support ventilation: effects on breathing pattern, patient effort, and comfort. J Crit Care. (2014) 29:380–5. doi: 10.1016/j.jcrc.2014.01.015
23. Meric H, Calabrese P, Pradon D, Lejaille M, Lofaso F, Terzi N. Physiological comparison of breathing patterns with neurally adjusted ventilatory assist (NAVA) and pressure-support ventilation to improve NAVA settings. Respir Physiol Neurobiol. (2014) 195:11–8. doi: 10.1016/j.resp.2014.01.021
24. Younes M, Kun J, Webster K, Roberts D. Response of ventilator-dependent patients to delayed opening of exhalation valve. Am J Respir Crit Care Med. (2002) 166:21–30. doi: 10.1164/rccm.2107143
Keywords: automatic adjustment system, pressure support ventilation, patient-ventilator asynchrony, cycling-off, trigger
Citation: Liu L, Yu Y, Xu X, Sun Q, Qiu H, Chiumello D and Yang Y (2021) Automatic Adjustment of the Inspiratory Trigger and Cycling-Off Criteria Improved Patient-Ventilator Asynchrony During Pressure Support Ventilation. Front. Med. 8:752508. doi: 10.3389/fmed.2021.752508
Received: 03 August 2021; Accepted: 11 October 2021;
Published: 12 November 2021.
Edited by:
Longxiang Su, Peking Union Medical College Hospital (CAMS), ChinaReviewed by:
Dan Stieper Karbing, Aalborg University, DenmarkCopyright © 2021 Liu, Yu, Xu, Sun, Qiu, Chiumello and Yang. This is an open-access article distributed under the terms of the Creative Commons Attribution License (CC BY). The use, distribution or reproduction in other forums is permitted, provided the original author(s) and the copyright owner(s) are credited and that the original publication in this journal is cited, in accordance with accepted academic practice. No use, distribution or reproduction is permitted which does not comply with these terms.
*Correspondence: Yi Yang, eWl5aXlhbmcyMDA0QDE2My5jb20=
†These authors have contributed equally to this work
Disclaimer: All claims expressed in this article are solely those of the authors and do not necessarily represent those of their affiliated organizations, or those of the publisher, the editors and the reviewers. Any product that may be evaluated in this article or claim that may be made by its manufacturer is not guaranteed or endorsed by the publisher.
Research integrity at Frontiers
Learn more about the work of our research integrity team to safeguard the quality of each article we publish.