- 1National Clinical Research Center for Obstetrical and Gynecological Diseases, Wuhan, China
- 2Key Laboratory of Cancer Invasion and Metastasis, Ministry of Education, Wuhan, China
- 3Department of Obstetrics and Gynecology, Tongji Hospital, Tongji Medical College, Huazhong University of Science and Technology, Wuhan, China
Background: Population-based data on the risk assessment of newly diagnosed cervical cancer patients' bone metastasis (CCBM) are lacking. This study aimed to develop various predictive models to assess the risk of bone metastasis via machine learning algorithms.
Materials and Methods: We retrospectively reviewed the CCBM patients from the Surveillance, Epidemiology, and End Results (SEER) database of the National Cancer Institute to risk factors of the presence of bone metastasis. Clinical usefulness was assessed by Akaike information criteria (AIC) and multiple machine learning algorithms based predictive models. Concordance index (C-index) and receiver operating characteristic (ROC) curve were used to define the predictive and discriminatory capacity of predictive models.
Results: A total of 16 candidate variables were included to develop predictive models for bone metastasis by machine learning. The areas under the ROC curve (AUCs) of the random forest model (RF), generalized linear model (GL), support vector machine (SVM), eXtreme Gradient Boosting (XGBoost), artificial neutral network (ANN), decision tree (DT), and naive bayesian model (NBM) ranged from 0.85 to 0.93. The RF model with 10 variables was developed as the optimal predictive model. The weight of variables indicated the top seven factors were organ-site metastasis (liver, brain, and lung), TNM stage and age.
Conclusions: Multiple machine learning based predictive models were developed to identify risk of bone metastasis in cervical cancer patients. By incorporating clinical characteristics and other candidate variables showed robust risk stratification for CCBM patients, and the RF predictive model performed best among these predictive models.
Introduction
Cervical cancer is one of the most common and deadly cancers in low-income and middle-income countries. Each year, more than half a million women are diagnosed with cervical cancer and the disease results in over 300,000 deaths worldwide (1). To date, multimodal therapy is promising for early-stage or locally advanced cervical cancer patients. However, there is no specific widely accepted access for cervical cancer patients with metastasis because of heterogeneous manifestations (2).
According to the International Federation of Gynecology and Obstetrics (FIGO) stage criteria, cervical cancer patients with FIGO stage I to IV, or according to the American Joint Committee Cancer (AJCC) criteria, any AJCC tumor[T] stage, lymph node[N] stage, and distant metastasis of peritoneal spread and involvement of supraclavicular-, para-aortic-, or mediastinal lymph node [M1], organ-site metastasis (lung, liver, brain, or bone) at initial diagnosis, or who have persistent/recurrent disease outside the pelvis, are classified as metastatic cases (3). For patients presenting with isolated or multiple metastasis who received more than one prior systemic therapy had dismal outcomes (4). Collectively, the survival outcomes of patients with metastatic cervical cancer are poor.
Bone is the third most common site of distant metastasis after the lung and liver (5). The incidence of bone metastasis from the carcinoma of the uterine cervix were reported from 0.8 to 23% (5–8). For most of CCBM patients, lesions of the bone were detected within 1 year after completion of the initial treatments by bone scan, FDG-PET, X-ray, or MRI (6). In short, there is a lag in diagnosis which make severe influence in prognosis. So early prediction of the occurrence of bone metastases and immediate treatment is important, to improve the quality of life in patients with cervical cancer. Besides, there is no standard accepted guideline for the treatment of CCBM patients involvement because of its low prevalence and the lack of large population-based study. Consequently, there is an urgent need to develop an accurate model for predicting the risk and survival outcome of CCBM patients that can be used to facilitate the management of clinical treatment.
In the present study, we established multiple predictive models which use data classification algorithm, including generalized linear model, random forest, support vector machine, extreme gradient boosting, artificial neutral network, decision tree, naive bayesian model, based on supervised machine learning algorithm to predict the risk factors for CCBM patients using the Surveillance, Epidemiology, and End Results (SEER) database. We then analyzed the predictive performance of this nomogram in a deviation cohort and then verified performance in an internal validation cohort.
Materials and Methods
Patients Enrollment From the SEER Database
Between January 1, 2010 and December 31, 2016, we retrospectively collated data from consecutive patients who had been diagnosed with cervical cancer from the SEER database. Data were acquired to generate the case listing via the SEER*Stat software version 8.3.6 (https://seer.cancer.gov/data/). Since the SEER data are anonymized, the need for institutional review board approval was waived. The SEER 18 registries were used for cases selection, which representing ~30% of the US population (9). According to the International Classification of Diseases for Oncology-3 (ICD-O-3)/WHO 2008, the entry name is “cervix uteri.” The exclusion criteria were as follows: (1) patients for whom the presence or absence of bone metastasis at diagnosis was unknown; (2) patients diagnosed at autopsy or death certificates; (3) patients younger than 18 years old; (4) patients diagnosed with carcinoma in situ, benign or borderline tumors. Besides, for individual patient IDs with multiple records, the primary registry was included. Hence, derived AJCC 6th and SEER combined stage (2016+) were used for tumor node metastasis (TNM) staging classification in our study. Figure 1 presented a flowchart of data screening from the SEER database and subsequent analysis followed.
Study Covariables
We collected demographical and clinical variables as follows: age at initial diagnosis, race [White, Black, and other (American Indian/Alaska Native, Asian Native, and Asian/Pacific Islander)], the year of diagnosis, primary site, the SEER historic, lymph biopsy, regional lymph nodes examined, surgery, tumor size, marital status, tumor grade [well-differentiated (grade1), moderately differentiated (grade2), poorly differentiated (grade 3), pathology, and undifferentiated (grade 4)], survival status, survival time [median (IQR)], distant lymph metastasis and the presence of other distant site metastasis (brain, liver and lung), TNM staging (Tumor, Node, and metastasis), insurance status.
Construction of Machine Learning Based Predictive Models
According to the rules of clinical predictive model establishment, all CCBM patients were randomly divided into training set and test set by 7:3, keeping the distribution of bone metastasis data in both groups consistent. Seven supervised learning model were developed to predict the risk of bone metastasis, including random forest model (RF), generalized linear model (GL), support vector machine (SVM), eXtreme Gradient Boosting (XGBoost), artificial neutral network (ANN), decision tree (DT), and naive bayesian model (NBM).
Strategy for Eigenfactor Selection and Model Validation
In order to avoid over fitting the model and the loss of information as much as possible, the EasyEnsemble, BalanceCascade and 10-fold cross-validation were used to select eigenfactor. For each repeated time, subsets were randomly arranged in the training and test group. The rank of each candidate variable from the training set was included in the seven machine learning based predictive model, and validated in the test set.
Statistical Analysis
Continuous variables are expressed as mean (standard deviation) and compared using the two-tailed t-test or the Mann-Whitney test. Categorical variables were compared using the χ2 test or Fisher's exact test. To explore potential predictive factors, we also calculated the odds ratio (OR) and the corresponding 95% confidence interval (CI) from the generalized linear (GLM) model. The risk factors for cervical cancer patients with bone metastasis were predicted primarily by univariable logistic regression. The useful univariable logistic regression (P < 0.05) were considered as candidates for the further multivariable logistic analysis. A nomogram was formulated based on results arising from the Akaike information criteria (AIC) analysis. The nomogram was based on the proportional conversion of each regression coefficient in the multivariate logistic regression to a 0 to 100-point scale. The effect of the variable with the highest β coefficient (the absolute value) was assigned 100 points (10). Points were added for all independent variables in order to create a total which was then converted to predicted probabilities. Next, we used bootstrapping plots to calculate the concordance index (C-index) and area under the receiver operating characteristic curve (AUC) so that we could evaluate our ability to calibrate the curve. Typically, C-index and AUC values that exceeded 0.6 were suggestive of a reasonable estimation. We also used net reclassification index (NRI) and integrated discrimination improvement (IDI) to evaluate the clinical benefits and utility of the nomogram, as described previously (11, 12). The cut-off point for risk stratifications was selected using X-tile. All analyses were conducted using SAS, version 9.1 (SAS Institute Inc.) and the R statistical package (v.3.6.2; R Foundation for Statistical Computing, Vienna, Austria; https://www.r-project.org). A P-value < 0.05 was considered to be statistically significant.
Results
Patient Characteristics
A total of 22,792 of CCBM patients' clinical characteristics and pathological baseline data were summarized in Table 1. The old patients (age ≥50) presented with a significantly increased incidence of bone metastasis compared with patients with young age (P < 0.001). Moreover, patients with high grade, pathology (adenocarcinoma vs. squamous cell carcinoma), lymph vascular invasion (diagnosed 2010+ for the schemas for penis and testis only), TNM stage, lymph biopsy (regional lymph nodes removed or not), surgery, regional lymph nodes examination, distant site metastasis (liver, brain and lung), and tumor size also contributed to higher bone metastasis incidence. We constructed generalized linear model, random forest model and another five supervised machine learning algorithm in classification outcomes predication. Besides, to develop machine learning based predictive models, a total of 16 features were selected: age at initial diagnosis (as continuous variable), race, primary site (Cervix uteri, Endocervix, Exocervix equivalent FIGO I, Overlapping lesion of cervix uteri equivalent FIGO II), the SEER historic, surgery, tumor size, distant lymph metastasis, tumor grade, pathology and the presence of other distant site metastasis (brain, liver and lung), TNM staging (Tumor, Node, and metastasis), insurance status. The whole patients were randomly split into a training set (N = 15,954, 70%) and validation set (N = 6,838, 30%).
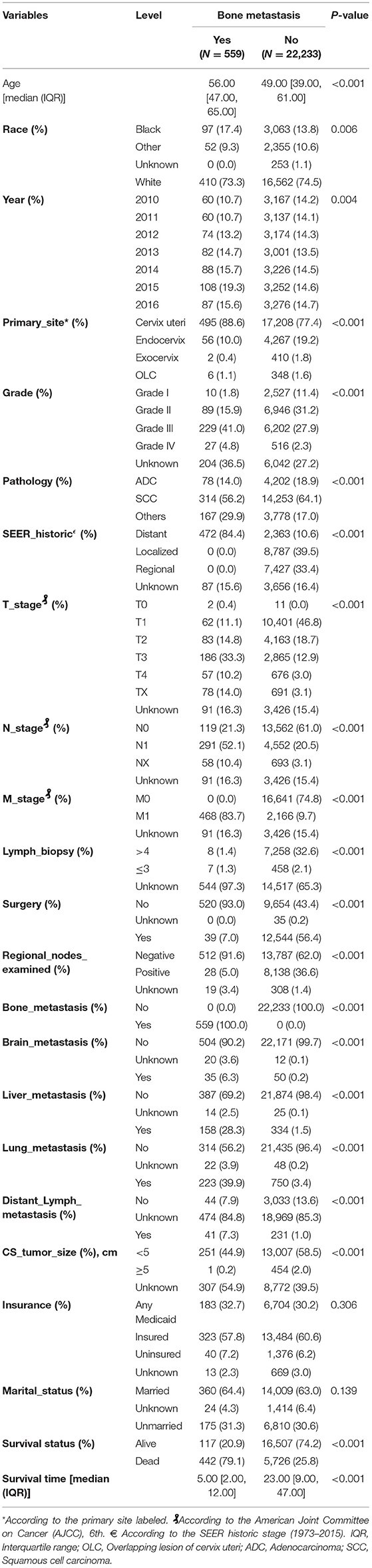
Table 1. Baseline demographic and clinical characteristics of included patients diagnosed with and without bone metastasis.
Risk Assessment of Bone Metastasis With GL Model
Traditionally, linear regression has been the technique of choice for predicting medical risk (13). The GL model is reasonably well-known, with the exception of logistic, log-linear, and some survival models. The risk factors associated with bone metastasis were screened using univariate and multivariate logistic regression, as presented in Table 2. Based on the AIC results, the lymph biopsy, brain metastasis, liver metastasis, lung metastasis, and distant lymph metastasis were positively correlated with the development of bone metastasis. The nomogram was constructed using these five significant risk factors listed above (Figure 2A). The Brier score showed the robust accuracy of probabilistic predictions (Figure 2B). The C-indexes of the nomogram for predicting risk of bone metastasis were 0.85 (95% CI: 0.83–0.86), which also showed good predictive value of the nomogram in the validation cohort (Figure 2C).
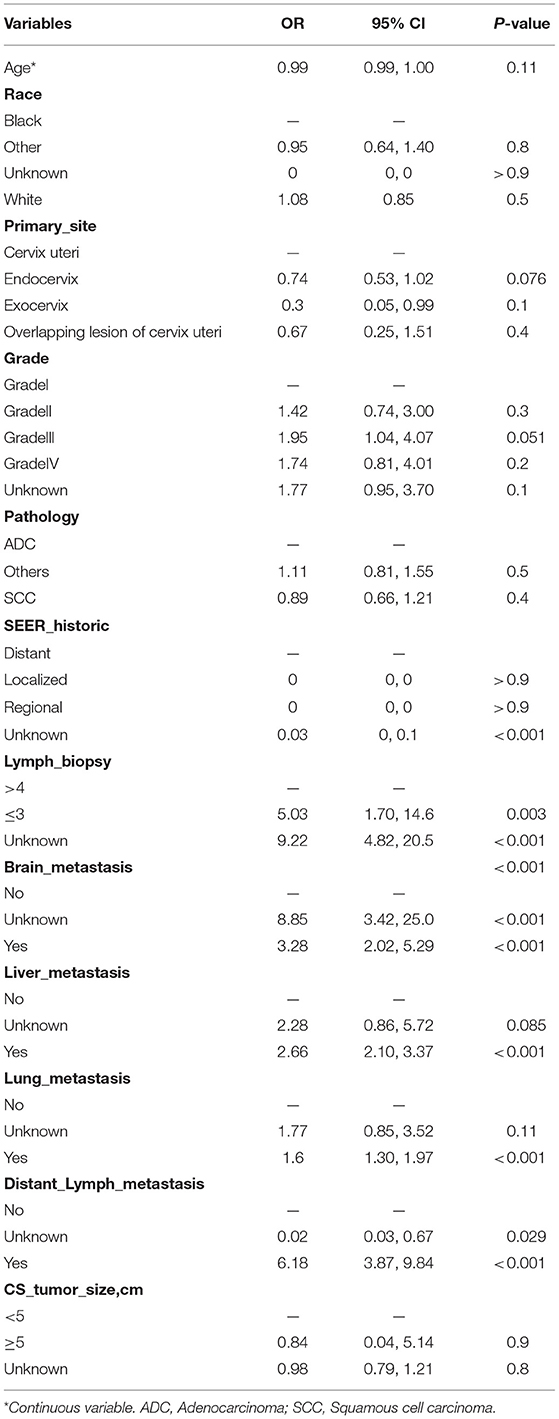
Table 2. Multivariate logistic regression analysis for risk factors associated with bone metastasis in cervical patients.
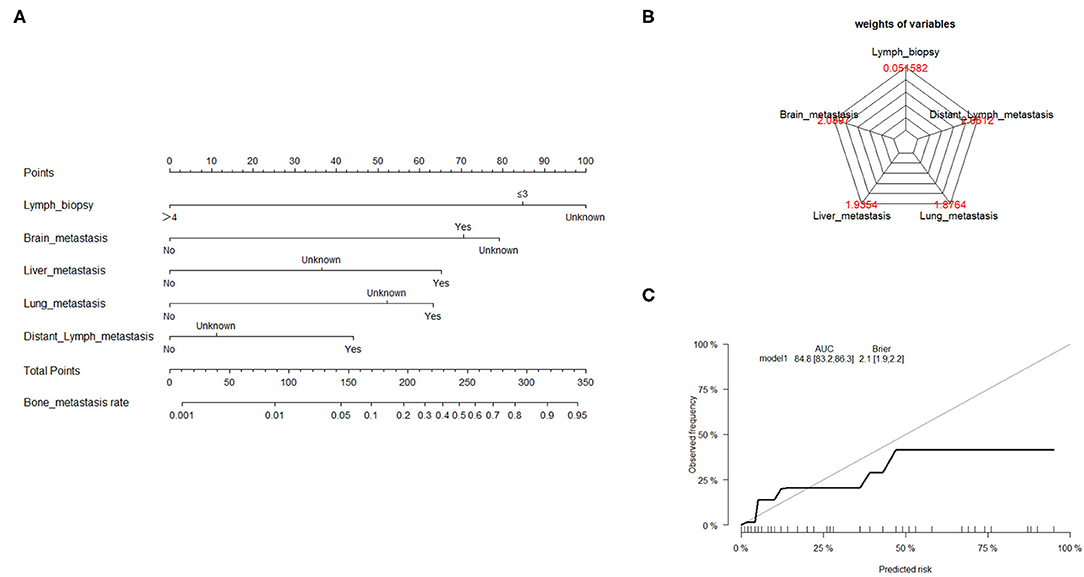
Figure 2. Nomogram to estimate the risk of bone metastasis. (A) A nomogram for predicting the risk of bone metastasis showing the proportion (%) of parameters included in the score scale. To use the bone metastasis nomogram score, it is important to identify the point of each variable on the corresponding axis; the total number of points can then be summated from all variables. (B) Radar plot showing the relative weight of candidate parameters arising from stepwise regression analysis. (C) Calibration curves depicting the robust performance of the nomogram in terms of consensus between the predicted risk and actual risk assessment.
Prediction of Bone Metastasis With RF Model
The Random Forest technique has great advantages over other algorithms and performs well on many current data sets. It is a regression tree technique which uses bootstrap aggregation and randomization of predictors to achieve a high degree of predictive accuracy (14). Although random forest model cannot generate a score sheet, it can handle data of very high dimensions (many features) and give out which features are more important after training. In the forest, the class predictions produced by each tree were assembled and the model prediction was finally determined according to the majority vote (15). As indicated in Table 3, sixteen variables were ordered according to the Mean Decrease Gini index. The random forest could better distinguish cervical cancer patients with bone metastasis or not when the number of decision tree was 500 (Figure 3B). The AUC was 0.93 (95% CI: 0.91–0.96), which showed robust consistency between the probability and observation in the RF model (Figure 3C).
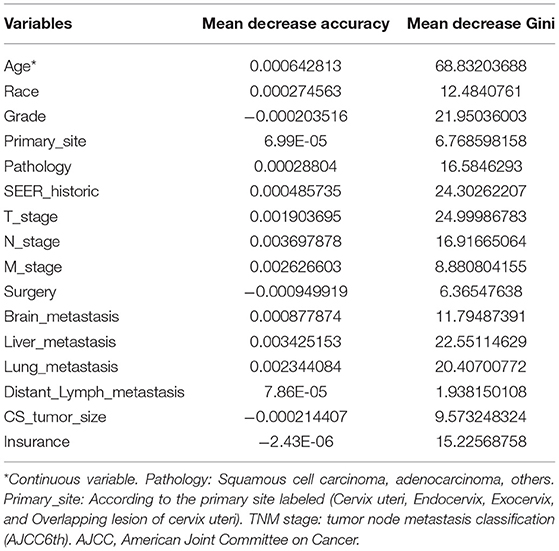
Table 3. The candidate variables screening associated with bone metastasis based on random forest model.
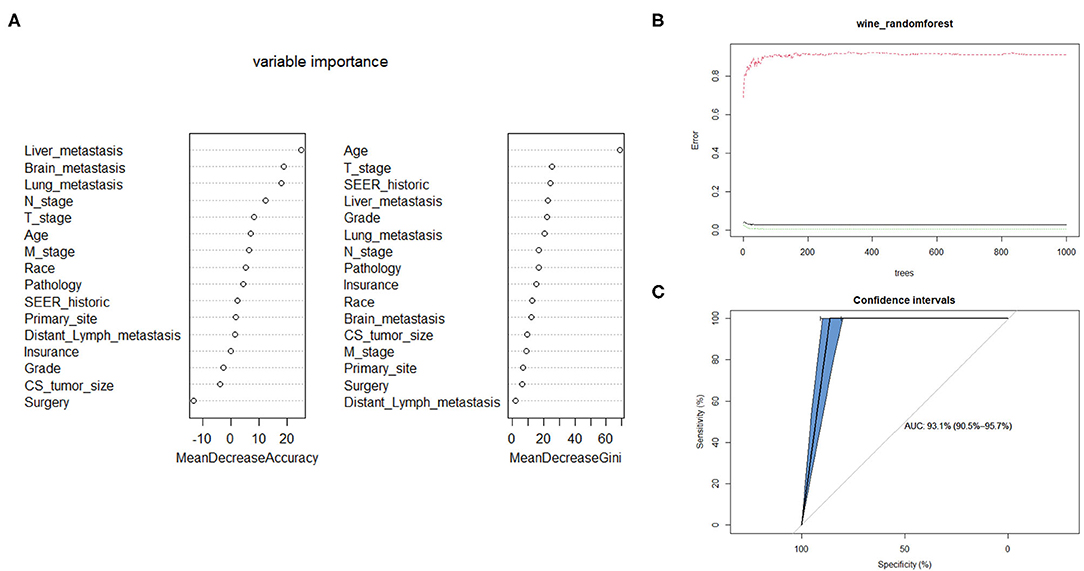
Figure 3. Random forest model. (A) The candidate factors associated with micrometastasis of lymph nodes were ordered according to the mean decreased Gini index. (B) Relationship of dynamic changes between the prediction error and the number of decision trees. (C) Performance of the prediction model with increasing numbers of features in the ROC curve.
Another Five Supervised Learning Models Developed for CCBM
On the basis of monofactor analysis of baseline characteristics of included patients, we further use another five supervised learning models to conduct CCBM risk assessment to see if we can improve prediction performance. A total of 16 candidate variables were used to develop predictive model for bone metastasis based on supervised learning algorithms. The predictive performance of all models were shown in Table 4. By feature selection, the variables for each algorithm were ranked by their predictive importance, the optimal permutation and combination of variables were included in model construction. The RF model with 10 variables, as shown in Figure 3A, had the highest net benefits almost across the entire range of threshold probabilities. Five models (SVM, XGBoost, ANN, DT, NBM) performed significantly better than the GL model at most of threshold points. Among these five model, we can see naive bayesian model is the best which has highest mean AUC.
Discussion
Hematogenous metastasis and lymphatic metastasis remain a major cause of cervical cancer related death in women (3). However, the bone manifestation is rare in cervical cancer patients. The rates of bone metastasis in cervical cancer patients with early-stage and advanced stage were reported from 4.0 to 22.9% (16–19). As for bone metastasis, vertebral column is the most frequent site, particularly the lumbar spine (20). Among the 22,792 patients with solitary metastasis or multiple metastasis analyzed for incidence, we found in this study that the incidence of cervical cancer with bone metastasis was 2.5%, consistent with previous studies (16, 21, 22). Early diagnosis and proper treatment of CCBM patients can prevent or relieve symptoms such as severe pain, pathological fracture, and even disability.
Currently, there are no referential screening guidelines for the warning of CCBM patients, the identification of predictive model for the development of bone metastasis could contribute to cervical cancer patients with high risk for developing bone metastasis, and if possible, a predictive model is guidable for appropriate preventive treatment at an early stage. In this study, we found that cervical cancer patients with older age (≥50 years), poorly differentiation, advanced stage, non-squamous histology type, combined with other organ metastasis and without operation at initial treatment were more inclined to suffering bone metastasis. Indeed, it's not hesitated that cervical cancer patients with elder age, advanced disease, non-squamous type, and lymphatic metastasis are associated with high risk of bone metastasis, as well as these risk factors have been elucidated to contribute to poor prognosis.
There are also other prognostic factors which could be used for the prognostic model. Nartthanarung A et al. reported that patients younger than 45 years with bone metastasis at the time of the cervical cancer diagnosis have a poorer prognosis than elderly patients (16). Previous studies also demonstrated that elder cervical cancer patients had adverse prognosis regardless of FIGO stage and histologic subtypes (23, 24). Based on these findings, we developed a predictive score system that can be fabricated to evaluate the probability of the cervical cancer patients with bone metastasis development in the future. These nomograms had better calibration and discriminatory ability, and could be used for clinically meaningful prognostic and predictive assessment of bone metastasis.
Until now, due to the lack of large population-based study with first-diagnosed metastatic cervical cancer, the way of treatment for CCBM patients is still controversial. Hamanishi et al. reported that timely hemipelvectomy for lateral recurrent cervical cancer had reduced tumor pain and prolonged survival (25). Pasricha et al. reported that surgical excision improved the patient's quality of life and palliating pain (26). Park et al. reported that CCBM patients who do not receive therapy for bone metastasis survive for <6 months (22). Hence, for resectable bone metastasis is still far from satisfactory. However, for cervical carcinoma metastatic to the bone, existed evidence demonstrated that concurrent chemotherapy and bisphosphonate administration might be promising (3). Ratanatharathorn et al. reported that radiotherapy provided moderate palliation for treatable patients (27). However, Yu et al. reported that local radiotherapy was merely useful for pain relief, the prognosis was not prolonged (28). Kanayama et al. reported that radiotherapy followed by cisplatin-based chemotherapy for cervical cancer patients with calcaneal metastasis with ideal general condition (29). Collectively, there is no standard treatment option for CCBM patients. With regard to chemotherapy, palliative transcatheter arterial chemoembolization/embolization, compared to intravenous administration, seems to be a suitable treatment method for symptomatic bone metastasis (30). For symptomatic and uncomplicated bone metastasis, a single dose of 8 Gy treatment prescribed to the appropriate target volume is recommended (31). However, a total dose of 30 Gy in 10 fractions is also considered as a standard method with lower rates of pathological fracture and spinal cord compression (32, 33).
In addition, this study inevitably has some limitations. Firstly, due to the insufficient information medical records by SEER database, external validation is warranted in the future. Secondly, the therapeutic experience in our study and many of references are small sized retrospective studies, future large sized and prospective studies are required to provide more instructive information. Thirdly, it was not recommended to perform the survival analysis stratified by radiotherapy and chemotherapy as the records were lacking from the SEER database. Further investigations should be performed to elucidate these results.
Conclusion
This population-based study depended on the internal validation to evaluate the role of predictive model as to bone metastasis of cervical cancer. In this study, we established seven predictive models for the risk estimation of bone metastasis in CCBM patients. Random forest model performed highest predictive capability among seven predictive models. We also developed a predictive score system based on generalized linear model that can be fabricated to evaluate the probability of the cervical cancer patients with bone metastasis development in the future.
Although we explored seven different machine algorithms to build bone metastasis risk models for cervical cancer patients, there is no significant difference in their predictive performance. In actual clinical practice, we can select multiple models for prediction based on the relevant characteristic information provided by the patient. When the prediction results are consistent, the credibility of the results can be upgraded.
Data Availability Statement
The original contributions presented in the study are included in the article/supplementary material, further inquiries can be directed to the corresponding author/s.
Author Contributions
SW and YL designed this study. SZ, JD, and MW drafted the manuscript. BW and JZ prepared all the figures and tables. All authors contributed to the article and approved the submitted version.
Funding
This work was financially supported by the National Key R&D Program of China (No. 2016YFC1302902) and the Clinical Research Pilot Project of Tongji Hospital, Huazhong University of Science and Technology (No. 2019CR205).
Conflict of Interest
The authors declare that the research was conducted in the absence of any commercial or financial relationships that could be construed as a potential conflict of interest.
Publisher's Note
All claims expressed in this article are solely those of the authors and do not necessarily represent those of their affiliated organizations, or those of the publisher, the editors and the reviewers. Any product that may be evaluated in this article, or claim that may be made by its manufacturer, is not guaranteed or endorsed by the publisher.
References
1. Cohen PA, Jhingran A, Oaknin A, Denny L. Cervical cancer. Lancet. (2019) 393:169–82. doi: 10.1016/S0140-6736(18)32470-X
2. van Meir H, Kenter GG, Burggraaf J, Kroep JR, Welters MJ, Melief CJ, et al. The need for improvement of the treatment of advanced and metastatic cervical cancer, the rationale for combined chemo-immunotherapy. Anticancer Agents Med Chem. (2014) 14:190–203. doi: 10.2174/18715206113136660372
3. Li H, Wu X, Cheng X. Advances in diagnosis and treatment of metastatic cervical cancer. J Gynecol Oncol. (2016) 27:e43. doi: 10.3802/jgo.2016.27.e43
4. Hou MM, Liu X, Wheler J, Naing A, Hong D, Bodurka D, et al. Outcomes of patients with metastatic cervical cancer in a phase I clinical trials program. Anticancer Res. (2014) 34:2349–55. doi: 10.1038/nrc3731
5. Thanapprapasr D, Nartthanarung A, Likittanasombut P, Na Ayudhya NI, Charakorn C, Udomsubpayakul U, et al. Bone metastasis in cervical cancer patients over a 10-year period. Int J Gynecol Cancer. (2010) 20:373–8. doi: 10.1111/IGC.0b013e3181d4a0a1
6. Agarwal U, Dahiya P, Chauhan A, Sangwan K, Purwar P. Scalp metastasis in carcinoma of the uterine cervix–a rare entity. Gynecol Oncol. (2002) 87:310–2. doi: 10.1006/gyno.2002.6829
7. Babar S, Rockall A, Goode A, Shepherd J, Reznek R. Magnetic resonance imaging appearances of recurrent cervical carcinoma. Int J Gynecol Cancer. (2007) 17:637–45. doi: 10.1111/j.1525-1438.2007.00849.x
8. Disibio G, French SW. Metastatic patterns of cancers: results from a large autopsy study. Arch Pathol Lab Med. (2008) 132:931–9. doi: 10.5858/2008-132-931-MPOCRF
9. Martin AM, Cagney DN, Catalano PJ, Warren LE, Bellon JR, Punglia RS, et al. Brain metastases in newly diagnosed breast cancer: a population-based study. JAMA Oncol. (2017) 3:1069–77. doi: 10.1001/jamaoncol.2017.0001
10. Steyerberg EW, Vergouwe Y. Towards better clinical prediction models: seven steps for development and an ABCD for validation. Eur Heart J. (2014) 35:1925–31. doi: 10.1093/eurheartj/ehu207
11. Vickers AJ, Cronin AM, Elkin EB, Gonen M. Extensions to decision curve analysis, a novel method for evaluating diagnostic tests, prediction models and molecular markers. BMC Med Inform Decis Mak. (2008) 8:53. doi: 10.1186/1472-6947-8-53
12. Vickers AJ, Elkin EB. Decision curve analysis: a novel method for evaluating prediction models. Med Decis Making. (2006) 26:565–74. doi: 10.1177/0272989X06295361
13. Blough DK, Madden CW, Hornbrook MC. Modeling risk using generalized linear models. J Health Eco. (1999) 18:153–71. doi: 10.1016/S0167-6296(98)00032-0
15. Pavey TG, Gilson ND, Gomersall SR, Clark B, Trost SG. Field evaluation of a random forest activity classifier for wrist-worn accelerometer data. J Sci Med Sport. (2017) 20:75–80. doi: 10.1016/j.jsams.2016.06.003
16. Nartthanarung A, Thanapprapasr K, Udomsubpayakul U, Thanapprapasr D. Age and survival of cervical cancer patients with bone metastasis. Asian Pac J Cancer Prev. (2014) 15:8401–4. doi: 10.7314/APJCP.2014.15.19.8401
17. Nanthamongkolkul K, Hanprasertpong J. Predictive factors of pelvic lymph node metastasis in early-stage cervical cancer. Oncol Res Treat. (2018) 41:194–8. doi: 10.1159/000485840
18. Boyraz G, Basaran D, Salman MC, Ozgul N, Yuce K. Clinical and pathological characteristics related to parametrial involvement in clinical early-stage cervical cancer. Ginekol Pol. (2016) 87:417–21. doi: 10.5603/GP.2016.0018
19. Shen G, Zhou H, Jia Z, Deng H. Diagnostic performance of diffusion-weighted MRI for detection of pelvic metastatic lymph nodes in patients with cervical cancer: a systematic review and meta-analysis. Br J Radiol. (2015) 88:20150063. doi: 10.1259/bjr.20150063
20. Fulcher AS, O'Sullivan SG, Segreti EM, Kavanagh BD. Recurrent cervical carcinoma: typical and atypical manifestations. Radiographics. (1999) 19:quiz S264–5. doi: 10.1148/radiographics.19.suppl_1.g99oc19s103
21. Kumar R, Gupta R, Khullar S, Padhy AK, Julka PK, Malhotra A. Bone scanning for bone metastasis in carcinoma cervix. J Assoc Physicians India. (2000) 48:808–10. doi: 10.5124/pi.2000.0212
22. Zhao Y, Wang JL, Wei LH, Bao DM. [Clinical analysis of eight cases of bone metastasis of uterine carcinomas]. Zhonghua Fu Chan Ke Za Zhi. (2006) 41:822–5. doi: 10.3760/j.issn:0529-567X.2006.12.008
23. Sawaya GF, Sung HY, Kearney KA, Miller M, Kinney W, Hiatt RA, et al. Advancing age and cervical cancer screening and prognosis. J Am Geriatr Soc. (2001) 49:1499–504. doi: 10.1046/j.1532-5415.2001.4911243.x
24. Quinn BA, Deng X, Colton A, Bandyopadhyay D, Carter JS, Fields EC. Increasing age predicts poor cervical cancer prognosis with subsequent effect on treatment and overall survival. Brachytherapy. (2019) 18:29–37. doi: 10.1016/j.brachy.2018.08.016
25. Hamanishi J, Higuchi T, Mandai M, Fukuhara K, An M, Nakayama T, et al. Intractable recurrent cervical cancer with pelvic bone involvement successfully treated with external hemipelvectomy. J Obstet Gynaecol Res. (2008) 34:112–6. doi: 10.1111/j.1447-0756.2007.00712.x
26. Pasricha R, Tiwari A, Aggarwal T, Lal P. Carcinoma of uterine cervix with isolated metastasis to fibula and its unusual behavior: report of a case and review of literature. J Cancer Res Ther. (2006) 2:79–81. doi: 10.4103/0973-1482.25857
27. Ratanatharathorn V, Powers WE, Steverson N, Han I, Ahmad K, Grimm J. Bone metastasis from cervical cancer. Cancer. (1994) 73:2372–9. doi: 10.1002/1097-0142(19940501)73:9<2372::AID-CNCR2820730921>3.0.CO;2-E
28. Yu GZ, Yao ZH, Sun JH. [Bone metastases from cancer of the cervix]. Zhonghua Zhong Liu Za Zhi. (1994) 16:231–3.
29. Kanayama T, Mabuchi S, Fujita M, Kimura T. Calcaneal metastasis in uterine cervical cancer: a case report and a review of the literature. Eur J Gynaecol Oncol. (2012) 33:524–5. doi: 10.1159/000342876
30. Koike Y, Takizawa K, Ogawa Y, Muto A, Yoshimatsu M, Yagihashi K, et al. Transcatheter arterial chemoembolization (TACE) or embolization (TAE) for symptomatic bone metastases as a palliative treatment. Cardiovasc Intervent Radiol. (2011) 34:793–801. doi: 10.1007/s00270-010-0031-8
31. Wu JS, Wong RK, Lloyd NS, Johnston M, Bezjak A, Whelan T. Radiotherapy fractionation for the palliation of uncomplicated painful bone metastases - an evidence-based practice guideline. BMC Cancer. (2004) 4:71. doi: 10.1186/1471-2407-4-71
32. Chow E, Zeng L, Salvo N, Dennis K, Tsao M, Lutz S. Update on the systematic review of palliative radiotherapy trials for bone metastases. Clin Oncol. (2012) 24:112–24. doi: 10.1016/j.clon.2011.11.004
Keywords: cervical cancer, bone metastasis, predictive model, machine learning algorithm, SEER
Citation: Han Y, Wang B, Zhang J, Zhou S, Dai J, Wu M, Li Y and Wang S (2021) Risk Assessment of Bone Metastasis for Cervical Cancer Patients by Multiple Models: A Large Population Based Real-World Study. Front. Med. 8:725298. doi: 10.3389/fmed.2021.725298
Received: 15 June 2021; Accepted: 31 August 2021;
Published: 05 October 2021.
Edited by:
Alfredo Ercoli, University of Messina, ItalyReviewed by:
Nazan Yurtcu, Sivas Cumhuriyet University Faculty of Medicine, TurkeySilvana Parisi, Università degli Studi di Messina, Italy
Copyright © 2021 Han, Wang, Zhang, Zhou, Dai, Wu, Li and Wang. This is an open-access article distributed under the terms of the Creative Commons Attribution License (CC BY). The use, distribution or reproduction in other forums is permitted, provided the original author(s) and the copyright owner(s) are credited and that the original publication in this journal is cited, in accordance with accepted academic practice. No use, distribution or reproduction is permitted which does not comply with these terms.
*Correspondence: Yan Li, liyan@tjh.tjmu.edu.cn; Shixuan Wang, shixuanwang@tjh.tjmu.edu.cn
†These authors have contributed equally to this work and share first authorship