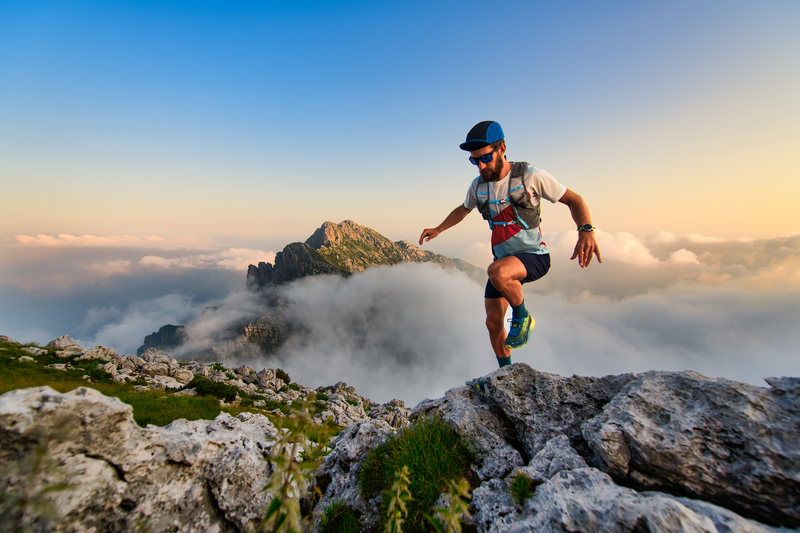
94% of researchers rate our articles as excellent or good
Learn more about the work of our research integrity team to safeguard the quality of each article we publish.
Find out more
MINI REVIEW article
Front. Med. , 11 August 2021
Sec. Rheumatology
Volume 8 - 2021 | https://doi.org/10.3389/fmed.2021.718922
This article is part of the Research Topic Early Origins of Psoriatic Arthritis View all 5 articles
Psoriatic arthritis (PsA) is a chronic inflammatory disease that develops in up to 30% of patients with psoriasis. In the vast majority of cases, cutaneous symptoms precede musculoskeletal complaints. Progression from psoriasis to PsA is characterized by subclinical synovio-entheseal inflammation and often non-specific musculoskeletal symptoms that are frequently unreported or overlooked. With the development of increasingly effective therapies and a broad drug armamentarium, prevention of arthritis development through careful clinical monitoring has become priority. Identifying high-risk psoriasis patients before PsA onset would ensure early diagnosis, increased treatment efficacy, and ultimately better outcomes; ideally, PsA development could even be averted. However, the current model of care for PsA offers only limited possibilities of early intervention. This is attributable to the large pool of patients to be monitored and the limited resources of the health care system in comparison. The use of digital technologies for health (eHealth) could help close this gap in care by enabling faster, more targeted and more streamlined access to rheumatological care for patients with psoriasis. eHealth solutions particularly include telemedicine, mobile technologies, and symptom checkers. Telemedicine enables rheumatological visits and consultations at a distance while mobile technologies can improve monitoring by allowing patients to self-report symptoms and disease-related parameters continuously. Symptom checkers have the potential to direct patients to medical attention at an earlier point of their disease and therefore minimizing diagnostic delay. Overall, these interventions could lead to earlier diagnoses of arthritis, improved monitoring, and better disease control while simultaneously increasing the capacity of referral centers.
Psoriasis (PsO) is a common chronic inflammatory skin disease affecting 1–3% of the general population (1). Approximately 1–3 in 10 patients with PsO will eventually progress to psoriatic arthritis (PsA), a chronic progressive seronegative spondyloarthropathy, characterized by arthritis, dactylitis, and enthesitis, with a debilitating course if untreated (2). Overall, an estimated 80% of PsA cases occur after a pre-existing diagnosis of PsO (3).
In the last 30 years, increasingly effective treatments for PsA have been developed and successfully implemented, with overwhelmingly positive effects on prognosis and long-term disability. This has shifted the focus of attention from treatment to treating, that is, to the early implementation and monitoring of available therapeutics.
Early diagnosis of PsA is fundamental, since a diagnostic delay as little as 6 months is associated with a poorer response to treatment, whereas early intervention with immune-modulating or anti-inflammatory drugs is linked with improved clinical and radiographic outcomes (4). However, factors responsible for the evolution from cutaneous to synovio-entheseal inflammation in these patients are still widely unknown. An earlier PsA diagnosis would require a better definition of disease phases and an in-depth characterization of the genetic, environmental, and immune-related events that precede PsA. In order to do so, large quantity of data must be generated through continuous access to rheumatological services and close clinical monitoring.
At present, the development of predictive models for PsA is impeded by a number of obstacles at multiple levels: at the macro level of diagnosis (i), at the meso level of access to specialist rheumatological cures (ii), and at the micro level of symptoms and disease monitoring (iii).
(i) Diagnostic delay currently represents one of the major challenges in rheumatology. The transition from PsO to PsA may either go unnoticed or be acknowledged with a major delay, leading to a poor window of treatment opportunity as well as to significant data loss (5–8). As musculoskeletal symptoms such as arthralgias, myalgias, and asthenia are quite common in the general population, it may be hard for general practitioners to correctly identify rheumatic symptoms that are indicative of an emerging PsA at its early stages (9). Also, patients may overlook their own musculoskeletal symptoms and wait for a spontaneous resolution or treat them with self-care methods (10).
(ii) Early access to rheumatological care is made difficult by a heavy workforce gap. Despite the global burden of chronic musculoskeletal disease being steadily on the rise, rheumatologist remain scarce and the global need of rheumatological cures cannot be met (11–13). The lack of sufficient numbers of rheumatologists has left some communities underserved and aggravated the problems of waiting times and diagnostic delay.
(iii) Even when a diagnosis of PsA has been suspected or ascertained, effectively monitoring the course of a rheumatic disease can prove to be a difficult task. While more frequent rheumatological visits are associated with greater improvements in pain and functionality (14), a sufficiently close monitoring of disease activity and patient-reported parameters is often not possible due to the relapsing-remitting nature of rheumatic diseases itself. Even in the occurrence of an exacerbation, an immediate rheumatological assessment is rarely a possibility and patients usually see their rheumatologist only after the disappearance of symptoms. Lastly, the advent of the coronavirus disease 2019 (COVID-19) pandemic further aggravated the problem, as many consultations had to be canceled or postponed (15–17).
Within this framework, the implementation of eHealth may step in to fill the gap. e-health interventions may offer a significant opportunity to both clinician and patients, as they promise to simultaneously improve disease monitoring and support self-management. e-health is already being extensively used beyond rheumatology and, to a lesser extent, in the management of rheumatoid arthritis. Thus, the question arises whether a similar change of paradigm in the current MoC for PsA could meet new necessities of patients and clinicians.
In this review, we cover current evidence on digital approaches in the early diagnosis of PsA in the PsO-PsA transition. Moreover, we review current eHealth tools for arthritis and discuss their possible implementations in this field.
e-Health is defined by the World Health Organization (WHO) as a collective term comprising the use of digital, mobile and wireless technologies to achieve health-related information, resources, and services (18). Examples of e-health include electronic medical records, telemedicine, symptom checkers, and mobile health (mHealth). As modern issues in the field of PsA management span across all levels of healthcare systems, the possibility of a “one-size-fits-all” solution appears highly unlikely. e-health can provide a diversified set of digital tools that may offer both parties—the patient and the clinician—new opportunities of information and knowledge exchange, as well as new more effective methods of disease monitoring. A number of digital approaches have already been tested both inside and outside rheumatology, and may bear great potential for application in the different stages of PsA (Figure 1).
Figure 1. The arrow with the yellow-red gradient represent the progression phases from psoriasis to psoriatic arthritis (from left to right). In genetically predisposed patients with psoriasis, interactions between genetic end environmental factors may lead to disease progression toward arthritis. In the preclinical phase, no clinical, serological, or imaging alterations are detectable. It is proposed that this phase is characterized by the beginning of an aberrant innate immune response in key tissues (i.e., skin, entheses, intestinal mucosa). The following subclinical phase is characterized by the presence of serum biomarkers and imaging alterations of musculoskeletal change without clinically symptomatic arthritis. This is followed by a phase of prodromal PsA in which fatigue and non-specific musculoskeletal symptoms may occur. The last stage is that of a fully blown clinically symptomatic Psoriatic Arthritis. The curve represents the burden and frequency of arthritis-related symptoms during each phase. The light blue boxes indicate the possible eHealth interventions for the management of the Psoriasis/Psoriatic arthritis progression. PsO, Psoriasis; PsA, Psoriatic Arthritis; ePROMs, electronic patient-reported outcome measures.
Telemedicine is the practice of medicine at a distance using an electronic mean of communication (19). All interventions, diagnoses, and therapeutic recommendations are based on patient information and data that are either synchronously or asynchronously transmitted through telecommunication systems (i.e., by telephone, e-mail, video conferencing). The use of telemedicine in rheumatology (telerheumatology) can facilitate the dematerialization of several processes that are normally hampered by deficient infrastructures or personnel (e.g., renewal of medical prescriptions, follow-up visits for long-term stable disease) (20, 21). This could effectively improve the accessibility to rheumatological care and help bridge the workforce gap by increasing the capacity of referral centers (22). The effectiveness of telerheumatology could be equal to or greater than face-to-face outpatient clinic visits in terms of diagnostic accuracy and remission induction, especially in patients with low disease activity or remission (23–27).
From a patient's point of view, a very positive attitude toward telemedicine in general and its use in rheumatology has been reported in multiple studies (23, 28–30). Most notably, in a German survey study on rheumatological patients 64.4% of participants declared they would rather use telemedicine instead of regular in-person visits during follow-up (31). Surveys have also shown that general practitioners, and to an even greater extent rheumatologists, would welcome the addition of telemedicine to routine care (31, 32). This, however, is in sharp contrast with the actual numbers of telemedicine use in rheumatology (31). A survey from the American Medical Association showed that short of 10% of U.S. rheumatologists used telemedicine, a relatively low number and significantly less than other specialists such as radiologists, which attested at around 43% (33). Other surveys also found similar results, suggesting the existence of significant difficulties in implementing telerheumatolgy at a global level (31, 34). Organizational matters, rather than clinical ones, were described as the main barriers to the introduction of telemedicine by physicians. These include limited knowledge of the topic and the need for specific training, excessive administrative expenses for the purchase of equipment, and lacking reimbursement opportunities (31).
However, with the sudden begin of the COVID-19 pandemic, an unprecedented resort to telemedicine took place worldwide. Drastic changes in the organization of rheumatological care had to be made in little time due to infection control policies aimed at reducing in-person visits (35, 36). This would have meant canceling or postponing large numbers of appointments, leaving patients unable to receive adequate rheumatological treatment. A common solution to this critical scenario came from the application of basic telemedicine, that is, by converting in-person visits to telephone or video consultations whenever possible (15, 37, 38). In the case of patients suffering from PsO, PsA, and other kinds of inflammatory arthritis, this approach has been well-documented in the literature and has involved large numbers of participants (39–41). As a result of the pandemic, it seems that patient and physician disposition toward telemedicine has improved, as well as the willingness to promote such instruments by health institutions (29, 32). This unusual opportunity is likely to accelerate the use of telemedicine and foster the development of new healthcare standards (42).
Telerheumatology also comprises several instruments that can be exploited by both physicians and patients for diagnosing, monitoring, and self-monitoring. The use of wearable sensors has already been experimented in rheumatoid arthritis to passively monitor hand mobility, step count, and energy expenditure and could easily find implementation in PsA as well (43, 44). The body-worn sensors generate data that can accurately reflect disease activity, flareups, and the degree of functional disability after a therapeutic intervention (45). Results can then be visualized to therefore provide individual support and recommendations and to improve therapy management and outcomes (46). Imaging is also an important factor for the detection of disease activity. Ultrasound is a cost effective and highly sensitive technique (47). Remote tele-mentored ultrasound (tele-ultrasound) is a tool that allows the sonographic evaluation of patients from a distance in a synchronous or asynchronous manner (48). It utilizes a single centralized physician with training in ultrasound interpretations alongside a bedside operator that performs image acquisition under the guidance of the former. This technique has already demonstrated its feasibility and accuracy in critical care applications (49), and even on the international space station (50). So far in rheumatology no reports are available, but the potential of this technique could be a good match in the early diagnosis of PsA. It is already known that a relevant portion of patients with PsO will exhibit subclinical joint and entheseal inflammation/disease before developing a clinically patent form of arthritis (51–53). Many of these patients, however, have no access to sonographic examination at their general practitioner's or in the dermatology clinic. Implementing tele-ultrasound of key articular and entheseal structures under the guidance of a rheumatologist may prove as an accurate and cost-effective solution. This may greatly contribute to the early diagnosis of PsA while simultaneously bypassing the problem of low accessibility to rheumatologists.
Evidence in support of an unrestricted use of telerheumatology, however, is currently limited. From a critical point of view, the loss of physical contact and the difficulties that emerge in establishing an emotional relationship between patient and physician have been regarded as the drawbacks of telemedicine (54, 55). In addition, problems of social nature may also emerge, as elderly and socioeconomically disadvantaged patients may lack the necessary skills or equipment to use telemedicine (56). In general, a lack of sufficient eHealth literacy has been found in both patients and physicians and needs to be addressed by offering specific education (34, 57). Further studies are needed to determine the best applications and conditions for the use of telemedicine, and especially a more rigorous assessment with specifically designed randomized clinical trials (58). Also, many of the available tools yet lack validation for PsA. It is likely that in the future patient- and physician-adapted telemedicine options will emerge, with the possibility of triaging patients for either digital or in-person consultations, as more appropriate for the specific situation (59, 60).
Modern treatment options for PsO and PsA include both pharmaceutical and non-pharmaceutical interventions. They require long-term adherence to medication regimen, phototherapy and physical therapy sessions, as well as a regular follow-up schedule. This increase in the complexity of the management of psoriasis has left many patients unwilling or unable to closely adhere to treatment plans (61). Tangible help in self-management and personalisation may come from mobile technology. The WHO defines mHealth as “the use of mobile and wireless technologies to support the achievement of health objectives” (62). Smartphone and mobile devices ownership is growing rapidly around the world. In Europe and the United States, over three quarters of the adult population are active smartphone users and use internet-based applications daily (63). The use of mobile devices for health purposes has thus become an increasingly accessible commodity for many, and numerous health-related smartphone applications are now available (64, 65). These include symptom checkers (66, 67) and referral tools (68) that could be helpful for the screening of early arthritic manifestations in PsO, as well as applications for the passive and active monitoring of disease activity [e.g., wearable sensors applications for the passive monitoring of key disease features (45, 69, 70), self-reporting tools (71)]. Medication adherence could also be improved via notification reminders on personal smartphones (72–74).
mHealth holds great potential for getting patients with rheumatic diseases involved in the self-management of their conditions, as well as for improving communications with their health care providers (75). Rheumatic patients already showed a propensity toward the use of mobile technologies to track their symptoms and disease activity in various surveys, and declared themselves optimistic about the utility of mHealth (32, 57). Sharing disease-related data for research purposes was also regarded favorably (32). Furthermore, rheumatologists themselves have increased their use of mobile health applications in recent years (33, 76). As with telehealth, COVID-19 acted as a catalyser for the acceptance of mHealth for both patients and physicians (32).
From a rheumatologist's perspective, mHealth can allow real-time symptom and disease activity monitoring and rapidly generate large quantities of patient-reported measures for clinical and research purposes (57, 77–79). Electronic patient-reported outcome measures (ePROMs) have many advantages compared to paper-based alternatives. Firstly, they seem to be preferred to traditional paper forms (80–82) and lead to comparable results (83). Furthermore, data collection through ePROMs is easier and faster, and completely bypasses the step of data digitalization (81). This could make the inclusion of PROMs into clinical practice more feasible for many rheumatologists. Indeed, a main reason for not implementing ePROMs is the unawareness of suitable software solutions (84). Also, sharing PROM results with patients can improve their knowledge of the disease and improve adherence by consolidating trust (85).
In the case of PsO, Schreier and colleagues developed a mobile phone-based teledermatology app for the real-time reporting of active psoriatic lesions through photographs (86). Similar applications, if integrated with further functionalities such as symptom checkers and monitoring tools, may facilitate the identification of patients at higher risk of developing arthritis and improve treatment outcomes for those who were already diagnosed.
Generalized enthusiasm toward mHealth, however, is not always matched by sufficient levels of competence with mobile technology. Studies found poor levels of literacy in this field, especially in older patients (57). Furthermore, analysis of the available applications for common smartphone operating systems found a lack of high-quality apps in terms of scientific accuracy and compliance to evidence-based guidelines (87–89). To address and reduce these limitations, the European alliance of associations for rheumatology (EULAR) has recently published recommendations for the development of mobile rheumatology applications (90). Nonetheless, specific studies demonstrating the effects of mobile applications in the field of rheumatology are direly needed.
Symptom checkers are artificial-intelligence (AI)-based tools designed to help patients determining the possible causes of their symptoms and eventually direct them to medical attention. In recent years, the use of internet- and mobile- based support for self-diagnosing has greatly increased, and an increasing number of patients perceive symptom checkers as useful for diagnosis (67, 91). In rheumatology, symptom checkers could help reducing diagnostic delay by ensuring quicker referral of patients who are at higher risk of having or developing a rheumatic condition. Bechterew-check is an online patient-facing self-referral tool for patients with chronic low back pain and has been developed for the early recognition of axial spondyloarthritis (92). This tool proved effective in the detection of previously undiagnosed cases of spondyloartrhitis by bringing high-risk patients to medical attention on the basis of clinical and demographic parameters. While holding great potential for early diagnosis, rheumatologists are not yet keen to recommend symptom checkers, as reliability remains one of the major drawbacks and there is still little evidence in their support (93). The full potential of symptom checkers, therefore, may only be reached in combination with other objective disease measures (94).
The Joint Pain Assessment Scoring Tool (JPAST) is an European Union-funded project on digital health in rheumatology that aims at combining eHealth with the analysis of validated biomarkers (95). The JPAST acts as a digital prognostic program by merging patient symptom checker inputs with serological and genetic data. This approach could greatly improve the early identification of patients at high risk of developing rheumatic diseases as well-facilitating a timely intervention.
Since risk stratification is the main conundrum of the PsO-PsA transition, combined eHealth tools such as JPAST hold great potential in this field. The generation and analysis of high-quality data is likely to allow to pre-emptively identify PsA patients in their pre-clinical phases, and to adjust therapeutic interventions and monitoring accordingly. Patient-centered eHealth platforms for spondyloarthritis such as SpA-NET in the Netherlands (96) and SpAMS in China (97) have already been developed and successfully implemented in clinical practice. This highlights the possibilities for eHealth in PsA management. In the future, the full potential of combined tools in rheumatological practice should be explored in more specific studies.
As research questions in rheumatology become more complex, finding the right answers requires growing quantities of patients and data that cannot always be provided by traditional clinical research. Electronic health has the potential to bridge this gap by using digital tools to increase data output while simultaneously reducing administrative efforts (57). With the possibility of rapidly generating large high-quality datasets, it is likely that innovative data collection systems such as digital crowdsourcing will be key elements for answering future research questions. Digital crowdsourcing is a technique of data collection that gathers the collective outputs of large numbers of participants (i.e., patients, clinicians) through technological means to rapidly provide answers to specific questions (98). Continuously generated data may be further elaborated and analyzed by AI and used as a basis for machine learning systems, that in turn can be trained to detect disease activity and flareups (99), predict therapy responses (100), and patient phenotyping (101, 102).
The traditional authoritative MoCs in which physician were the sole arbitrators in all matters of patients' therapies revealed several limitations in managing the PsO-PsA transition. This is due to both the high complexity of the issue and to the absence of effective means of collecting relevant disease-related data with traditional methods. Modern MoCs for PsO and PsA emphasize the importance of patient engagement and self-management (103). Within this context, eHealth approaches such as telemedicine, mHealth, and combined tools offer important opportunities to complement rheumatology care in order to establish timely and more accurate diagnoses, and to intercept high-risk PsO patients before their clinical transition toward arthritis (Figure 1). Previous experiences indicate that screening for at-risk patients is feasible through the monitoring of clinical and biometric parameters (e.g., pain, body weight, physical activity) (2), early imaging (51, 53, 104), and through screening questionnaires (105). At the moment, however, there are too little data to establish a definite strategy for the integration of eHealth in this aspect of PsA management. Until no validated tools for PsA are developed and tested in clinical trials, the potential of eHealth in this field is therefore bound to remain partly speculative. Digital solutions have already been implemented with promising results in rheumatoid arthritis (106, 107), and have the potential to be transferred and adapted to PsA. If successful, these measures could address diagnostic delay and low treatment adherence, improving disease monitoring and eventually leading to better disease outcomes.
FF and DS: literature research and manuscript drafting. FF, JK, AK, and DS: conceptualization. KT has expertise in the field of digital rheumatology and critically revised the manuscript. He also contributed to the revision phase. All authors contributed to the article and approved the submitted version.
This study was supported by the Deutsche Forschungsgemeinschaft (DFG-FOR2886 PANDORA and the CRC1181 Checkpoints for Resolution of Inflammation). Additional funding was received by the Bundesministerium für Bildung und Forschung (BMBF; project MASCARA), the ERC Synergy grant 4D Nanoscope, the IMI funded projects RTCure and HIPPOCRATES, the Emerging Fields Initiative MIRACLE of the Friedrich-Alexander-Universität Erlangen-Nürnberg, and the Else Kröner-Memorial Scholarship (DS, no. 2019_EKMS.27).
The authors declare that the research was conducted in the absence of any commercial or financial relationships that could be construed as a potential conflict of interest.
All claims expressed in this article are solely those of the authors and do not necessarily represent those of their affiliated organizations, or those of the publisher, the editors and the reviewers. Any product that may be evaluated in this article, or claim that may be made by its manufacturer, is not guaranteed or endorsed by the publisher.
1. Michalek IM, Loring B, John SM. A systematic review of worldwide epidemiology of psoriasis. J Eur Acad Dermatol Venereol. (2017) 31:205–12. doi: 10.1111/jdv.13854
2. Scher JU, Ogdie A, Merola JF, Ritchlin C. Preventing psoriatic arthritis: focusing on patients with psoriasis at increased risk of transition. Nat Rev Rheumatol. (2019) 15:153–66. doi: 10.1038/s41584-019-0175-0
3. Olivieri I, Padula A, D'Angelo S, Cutro MS. Psoriatic arthritis sine psoriasis. J Rheumatol. (2009) 36:28–9. doi: 10.3899/jrheum.090218
4. Haroon M, Gallagher P, FitzGerald O. Diagnostic delay of more than 6 months contributes to poor radiographic and functional outcome in psoriatic arthritis. Ann Rheum Dis. (2015) 74:1045–50. doi: 10.1136/annrheumdis-2013-204858
5. Sørensen J, Hetland ML. Diagnostic delay in patients with rheumatoid arthritis, psoriatic arthritis and ankylosing spondylitis: results from the Danish nationwide DANBIO registry. Ann Rheum Dis. (2015) 74:e12. doi: 10.1136/annrheumdis-2013-204867
6. Raza K, Stack R, Kumar K, Filer A, Detert J, Bastian H, et al. Delays in assessment of patients with rheumatoid arthritis: variations across Europe. Ann Rheum Dis. (2011) 70:1822–5. doi: 10.1136/ard.2011.151902
7. Stack RJ, Nightingale P, Jinks C, Shaw K, Herron-Marx S, Horne R, et al. Delays between the onset of symptoms and first rheumatology consultation in patients with rheumatoid arthritis in the UK: an observational study. BMJ Open. (2019) 9:e024361. doi: 10.1136/bmjopen-2018-024361
8. Simons G, Belcher J, Morton C, Kumar K, Falahee M, Mallen CD, et al. Symptom recognition and perceived urgency of help-seeking for rheumatoid arthritis and other diseases in the general public: a mixed method approach. Arthritis Care Res. (2017) 69:633–41. doi: 10.1002/acr.22979
9. Van Der Linden MPM, Le Cessie S, Raza K, Van Der Woude D, Knevel R, Huizinga TWJ, et al. Long-term impact of delay in assessment of patients with early arthritis. Arthritis Rheum. (2010) 62:3537–46. doi: 10.1002/art.27692
10. Kumar K, Daley E, Carruthers DM, Situnayake D, Gordon C, Grindulis K, et al. Delay in presentation to primary care physicians is the main reason why patients with rheumatoid arthritis are seen late by rheumatologists. Rheumatology. (2007) 46:1438–40. doi: 10.1093/rheumatology/kem130
11. Zink A, Schneider M. Work force requirements in rheumatology: key points from the 2016 update of the memorandum of the German Society for Rheumatology on Health Care Quality. Aktuelle Rheumatol. (2018) 43:390–4. doi: 10.1055/a-0573-8722
12. Al Maini M, Adelowo F, Al Saleh J, Al Weshahi Y, Burmester GR, Cutolo M, et al. The global challenges and opportunities in the practice of rheumatology: white paper by the world forum on rheumatic and musculoskeletal diseases. Clin Rheumatol. (2015) 34:819–29. doi: 10.1007/s10067-014-2841-6
13. Krusche M, Sewerin P, Kleyer A, Mucke J, Vossen D, Morf H. Specialist training quo vadis? Z Rheumatol. (2019) 78:692–7. doi: 10.1007/s00393-019-00690-5
14. Ward M. Rheumatology visit frequency and changes in functional disability and pain in patients with rheumatoid arthritis. J Rheumatol. (1997) 24:35–42.
15. Dejaco C, Alunno A, Bijlsma JWJ, Boonen A, Combe B, Finckh A, et al. Influence of COVID-19 pandemic on decisions for the management of people with inflammatory rheumatic and musculoskeletal diseases: a survey among EULAR countries. Ann Rheum Dis. (2021) 80:518–26. doi: 10.1136/annrheumdis-2020-218697
16. Perniola S, Alivernini S, Varriano V, Paglionico A, Tanti G, Rubortone P, et al. Telemedicine will not keep us apart in COVID-19 pandemic. Ann Rheum Dis. (2021) 80:e48. doi: 10.1136/annrheumdis-2020-218022
17. López-Medina C, Escudero A, Collantes-Estevez E. COVID-19 pandemic: an opportunity to assess the utility of telemedicine in patients with rheumatic diseases. Ann Rheum Dis. (2021) 80:e50. doi: 10.1136/annrheumdis-2020-218008
18. WHO. Resolution WHA71.7, 71st World Health Assembly. Geneva (2018). Available online at: https://apps.who.int/gb/ebwha/pdf_files/WHA71/A71_R7-en.pdf?ua=1 (accessed May 17, 2021).
19. WHO. Telemedicine in Member States. Report on the second global survey on eHealth Global Observatory for eHealth. (2010). Available online at: https://www.who.int/goe/publications/goe_telemedicine_2010.pdf (accessed May 17, 2021).
20. Rezaian MM, Brent LH, Roshani S, Ziaee M, Sobhani F, Dorbeigi A, et al. Rheumatology care using telemedicine. Telemed e-Health. (2020) 26:335–40. doi: 10.1089/tmj.2018.0256
21. Ward IM, Schmidt TW, Lappan C, Battafarano DF. How critical is tele-medicine to the rheumatology workforce? Arthritis Care Res. (2016) 68:1387–9. doi: 10.1002/acr.22853
22. Johnston K, Kennedy C, Murdoch I, Taylor P, Cook C. The cost-effectiveness of technology transfer using telemedicine. Health Policy Plan. (2004) 19:302–9. doi: 10.1093/heapol/czh035
23. Leggett P, Graham L, Steele K, Gilliland A, Stevenson M, O'Reilly D, et al. Telerheumatology–diagnostic accuracy and acceptability to patient, specialist, and general practitioner. Br J Gen Pract. (2001) 51:746–8.
24. Kuusalo L, Sokka-Isler T, Kautiainen H, Ekman P, Kauppi MJ, Pirilä L, et al. Automated text message–enhanced monitoring versus routine monitoring in early rheumatoid arthritis: a randomized trial. Arthritis Care Res. (2020) 72:319–25. doi: 10.1002/acr.23846
25. Sarzi-Puttini P, Marotto D, Caporali R, Montecucco CM, Favalli EG, Franceschini F, et al. Prevalence of COVID infections in a population of rheumatic patients from Lombardy and Marche treated with biological drugs or small molecules: a multicentre retrospective study. J Autoimmun. (2021) 116:102545. doi: 10.1016/j.jaut.2020.102545
26. de Thurah A, Stengaard-Pedersen K, Axelsen M, Fredberg U, Schougaard LMV, Hjollund NHI, et al. Tele-health followup strategy for tight control of disease activity in rheumatoid arthritis: results of a randomized controlled trial. Arthritis Care Res. (2018) 70:353–60. doi: 10.1002/acr.23280
27. Piga M, Cangemi I, Mathieu A, Cauli A. Telemedicine for patients with rheumatic diseases: systematic review and proposal for research agenda. Semin Arthritis Rheum. (2017) 47:121–8. doi: 10.1016/j.semarthrit.2017.03.014
28. Müehlensiepen F, Kurkowski S, Krusche M, Mucke J, Prill R, Heinze M, et al. Digital health transition in rheumatology: a qualitative study. Int J Environ Res Public Health. (2021) 18:1–11. doi: 10.3390/ijerph18052636
29. Matsumoto RA, Barton JL. Telerheumatology: before, during, and after a global pandemic. Curr Opin Rheumatol. (2021) 33:262–9. doi: 10.1097/BOR.0000000000000790
30. Shenoy P, Ahmed S, Paul A, Skaria TG, Joby J, Alias B. Switching to teleconsultation for rheumatology in the wake of the COVID-19 pandemic: feasibility and patient response in India. Clin Rheumatol. (2020) 39:2757–762. doi: 10.1007/s10067-020-05200-6
31. Muehlensiepen F, Knitza J, Marquardt W, Engler J, Hueber A, Welcker M. Acceptance of telerheumatology by rheumatologists and general practitioners in Germany: Nationwide cross-sectional survey study. J Med Internet Res. (2021) 23:e23742. doi: 10.2196/23742
32. Kernder A, Morf H, Klemm P, Vossen D, Haase I, Mucke J, et al. Digital rheumatology in the era of COVID-19: results of a national patient and physician survey. RMD Open. (2021) 7:1548. doi: 10.1136/rmdopen-2020-001548
33. Kane CK, Gillis K. The use of telemedicine by physicians: still the exception rather than the rule. Health Aff. (2018) 37:1923–30. doi: 10.1377/hlthaff.2018.05077
34. Van Der Vaart R, Drossaert CHC, De Heus M, Taal E, Van De Laar MAFJ. Measuring actual ehealth literacy among patients with rheumatic diseases: a qualitative analysis of problems encountered using health 1.0 and health 2.0 applications. J Med Internet Res. (2013) 15:e2428. doi: 10.2196/jmir.2428
35. Mehta B, Jannat-Khah D, Fontana MA, Moezinia CJ, Mancuso CA, Bass AR, et al. Impact of COVID-19 on vulnerable patients with rheumatic disease: results of a worldwide survey. RMD Open. (2020) 6:e001378. doi: 10.1136/rmdopen-2020-001378
36. Freudenberg S, Vossen D. Impact of COVID-19 on rheumatological care: a national survey in April 2020. Z Rheumatol. (2020) 79:584–9. doi: 10.1007/s00393-020-00833-z
37. Kavadichanda C, Shah S, Daber A, Bairwa D, Mathew A, Dunga S, et al. Tele-rheumatology for overcoming socioeconomic barriers to healthcare in resource constrained settings: lessons from COVID-19 pandemic. Rheumatology (Oxford). (2021) 60:3369–379. doi: 10.1093/rheumatology/keaa791
38. Gkrouzman E, Wu DD, Jethwa H, Abraham S. Telemedicine in rheumatology at the advent of the COVID-19 Pandemic. HSS J. (2020) 16:108–11. doi: 10.1007/s11420-020-09810-3
39. Piaserico S, Gisondi P, Cazzaniga S, Naldi L. Lack of evidence for an increased risk of severe COVID-19 in psoriasis patients on biologics: a cohort study from Northeast Italy. Am J Clin Dermatol. (2020) 21:749–51. doi: 10.1007/s40257-020-00552-w
40. Damiani G, Pacifico A, Bragazzi NL, Malagoli P. Biologics increase the risk of SARS-CoV-2 infection and hospitalization, but not ICU admission and death: real-life data from a large cohort during red-zone declaration. Dermatol Ther. (2020) 33:e13475. doi: 10.1111/dth.13475
41. Carugno A, Gambini DM, Raponi F, Vezzoli P, Locatelli AGC, Di Mercurio M, et al. COVID-19 and biologics for psoriasis: a high-epidemic area experience—Bergamo, Lombardy, Italy. J Am Acad Dermatol. (2020) 83:292–4. doi: 10.1016/j.jaad.2020.04.165
42. Hollander JE, Carr BG. Virtually perfect? Telemedicine for Covid-19. N Engl J Med. (2020) 382:1679–81. doi: 10.1056/NEJMp2003539
43. Fortune E, Tierney M, Scanaill CN, Bourke A, Kennedy N, Nelson J. Activity level classification algorithm using SHIMMER™ wearable sensors for individuals with rheumatoid arthritis. Annu Int Conf IEEE Eng Med Biol Soc. (2011) 2011:3059–62. doi: 10.1109/IEMBS.2011.6090836
44. Connolly J, Curran K, Condell J, Gardiner P. Wearable rehab technology for automatic measurement of patients with arthritis. In: 2011 5th International Conference on Pervasive Computing Technologies for Healthcare (PervasiveHealth) and Workshops. (2011). p. 508−9.
45. Gossec L, Guyard F, Leroy D, Lafargue T, Seiler M, Jacquemin C, et al. Detection of flares by decrease in physical activity, collected using wearable activity trackers in rheumatoid arthritis or axial spondyloarthritis: an application of machine learning analyses in rheumatology. Arthritis Care Res. (2019) 71:1336–43. doi: 10.1002/acr.23768
46. Henderson J, Condell J, Connolly J, Kelly D, Curran K. Review of wearable sensor-based health monitoring glove devices for rheumatoid arthritis. Sensors. (2021) 21:1–32. doi: 10.3390/s21051576
47. Amorese-O'Connell L, Gutierrez M, Reginato AM. General applications of ultrasound in rheumatology practice. Fed Pract. (2015) 32:8S–20S.
48. Britton N, Miller MA, Safadi S, Siegel A, Levine AR, McCurdy MT. Tele-ultrasound in resource-limited settings: a systematic review. Front Public Heal. (2019) 7:244. doi: 10.3389/fpubh.2019.00244
49. Robertson TE, Levine AR, Verceles AC, Buchner JA, Lantry JH, Papali A, et al. Remote tele-mentored ultrasound for non-physician learners using FaceTime: a feasibility study in a low-income country. J Crit Care. (2017) 40:145–8. doi: 10.1016/j.jcrc.2017.03.028
50. Fincke EM, Padalka G, Lee D, Van Holsbeeck M, Sargsyan AE, Hamilton DR, et al. Evaluation of shoulder integrity in space: first report of musculoskeletal US on the International Space Station. Radiology. (2005) 234:319–22. doi: 10.1148/radiol.2342041680
51. Simon D, Tascilar K, Kleyer A, Bayat S, Kampylafka E, Sokolova M, et al. Structural entheseal lesions in patients with psoriasis are associated with an increased risk of progression to psoriatic arthritis. Arthritis Rheumatol. (2020). doi: 10.1002/art.41239. [Epub ahead of print].
52. Simon D, Faustini F, Kleyer A, Haschka J, Englbrecht M, Kraus S, et al. Analysis of periarticular bone changes in patients with cutaneous psoriasis without associated psoriatic arthritis. Ann Rheum Dis. (2016) 75:660–6. doi: 10.1136/annrheumdis-2014-206347
53. Faustini F, Simon D, Oliveira I, Kleyer A, Haschka J, Englbrecht M, et al. Subclinical joint inflammation in patients with psoriasis without concomitant psoriatic arthritis: a cross-sectional and longitudinal analysis. Ann Rheum Dis. (2016) 75:2068–74. doi: 10.1136/annrheumdis-2015-208821
54. Onor ML, Misan S. The clinical interview and the doctor-patient relationship in telemedicine. Telemed J e-Health. (2005) 11:102–5. doi: 10.1089/tmj.2005.11.102
55. Menage J. Why telemedicine diminishes the doctor-patient relationship. BMJ. (2020) 371:m4348. doi: 10.1136/bmj.m4348
56. Hjelm NM. Benefits and drawbacks of telemedicine. J Telemed Telecare. (2005) 11:60–70. doi: 10.1258/1357633053499886
57. Knitza J, Simon D, Lambrecht A, Raab C, Tascilar K, Hagen M, et al. Mobile health usage, preferences, barriers, and ehealth literacy in rheumatology: patient survey study. JMIR mHealth uHealth. (2020) 8:e19661. doi: 10.2196/19661
58. McDougall JA, Ferucci ED, Glover J, Fraenkel L. Telerheumatology: a systematic review. Arthritis Care Res. (2017) 69:1546–57. doi: 10.1002/acr.23153
59. Kulcsar Z, Albert D, Ercolano E, Mecchella JN. Telerheumatology: a technology appropriate for virtually all. Semin Arthritis Rheum. (2016) 46:380–5. doi: 10.1016/j.semarthrit.2016.05.013
60. Taylor-Gjevre R, Nair B, Bath B, Okpalauwaekwe U, Sharma M, Penz E, et al. Addressing rural and remote access disparities for patients with inflammatory arthritis through video-conferencing and innovative inter-professional care models. Musculoskeletal Care. (2018) 16:90–5. doi: 10.1002/msc.1215
61. Murage MJ, Tongbram V, Feldman SR, Malatestinic WN, Larmore CJ, Muram TM, et al. Medication adherence and persistence in patients with rheumatoid arthritis, psoriasis, and psoriatic arthritis: a systematic literature review. Patient Prefer Adherence. (2018) 12:1483–503. doi: 10.2147/PPA.S167508
62. WHO. mHealth New horizons for Health Through Mobile Technologies. Report on the Third Global Survey on eHealth Global Observatory. (2011). Available online at: https://www.who.int/goe/publications/goe_mhealth_web.pdf (accessed May 17, 2021).
63. Newzoo. 2020 Free Global Mobile Market Report. (2020). Available online at: https://resources.newzoo.com/hubfs/Reports/2020_Free_Global_Mobile_Market_Report (accessed May 26, 2021).
64. Doarn CR, Merrell RC. There's an app for that. Telemed e-Health. (2013) 19:811–2. doi: 10.1089/tmj.2013.9983
65. Boulos MNK, Wheeler S, Tavares C, Jones R. How smartphones are changing the face of mobile and participatory healthcare: an overview, with example from eCAALYX. Biomed Eng Online. (2011) 10:1–14. doi: 10.1186/1475-925X-10-24
66. Kataria S, Ravindran V. Digital health: a new dimension in rheumatology patient care. Rheumatol Int. (2018) 38:1949–57. doi: 10.1007/s00296-018-4037-x
67. Knitza J, Mohn J, Bergmann C, Kampylafka E, Hagen M, Bohr D, Morf H, et al. Accuracy, patient-perceived usability, and acceptance of two symptom checkers (Ada and Rheport) in rheumatology: interim results from a randomized controlled crossover trial. Arthritis Res Ther. (2021) 23:112. doi: 10.1186/s13075-021-02498-8
68. Urruticoechea-Arana A, León-Vázquez F, Giner-Ruiz V, Andréu-Sánchez JL, Olivé-Marqués A, Freire-González M, et al. Development of an application for mobile phones (App) based on the collaboration between the Spanish Society of Rheumatology and Spanish Society of Family Medicine for the referral of systemic autoimmune diseases from primary care to rheumatology. Reumatol Clin. (2020) 16:373–7. doi: 10.1016/j.reuma.2019.09.001
69. Azevedo R, Bernardes M, Fonseca J, Lima A. Smartphone application for rheumatoid arthritis self-management: cross-sectional study revealed the usefulness, willingness to use and patients' needs. Rheumatol Int. (2015) 35:1675–85. doi: 10.1007/s00296-015-3270-9
70. Yamada M, Aoyama T, Mori S, Nishiguchi S, Okamoto K, Ito T, et al. Objective assessment of abnormal gait in patients with rheumatoid arthritis using a smartphone. Rheumatol Int. (2012) 32:3869–74. doi: 10.1007/s00296-011-2283-2
71. Shinohara A, Ito T, Ura T, Nishiguchi S, Ito H, Yamada M, et al. Development of lifelog sharing system for rheumatoid arthritis patients using smartphone. Proc Annu Int Conf IEEE Eng Med Biol Soc EMBS. (2013) 2013:7266–9. doi: 10.1109/EMBC.2013.6611235
72. Yang J, Weng L, Chen Z, Cai H, Lin X, Hu Z, et al. Development and testing of a mobile app for pain management among cancer patients discharged from hospital treatment: randomized controlled trial. JMIR Mhealth Uhealth. (2019) 7:e12542. doi: 10.2196/12542
73. Kang H, Park HA. A mobile app for hypertension management based on clinical practice guidelines: development and deployment. JMIR Mhealth Uhealth. (2016) 4:e12. doi: 10.2196/mhealth.4966
74. Mary A, Boursier A, Desailly Henry I, Grados F, Séjourné A, Salomon S, et al. Mobile phone text messages and effect on treatment adherence in patients taking methotrexate for rheumatoid arthritis: a randomized pilot study. Arthritis Care Res. (2019) 71:1344–52. doi: 10.1002/acr.23750
75. Gyorffy Z, Radó N, Mesko B. Digitally engaged physicians about the digital health transition. PLoS ONE. (2020) 15:e0238658. doi: 10.1371/journal.pone.0238658
76. Knitza J, Vossen D, Geffken I, Krusche M, Meyer M, Sewerin P, et al. Use of medical apps and online platforms among German rheumatologists: results of the 2016 and 2018 DGRh conference surveys and research conducted by rheumadocs. Z Rheumatol. (2019) 78:839–46. doi: 10.1007/s00393-018-0578-3
77. Richter JG, Chehab G, Kiltz U, Callhoff J, Voormann A, Lorenz HM, et al. Digital health in rheumatology - status 2018/19. Dtsch Medizinische Wochenschrift. (2019) 144:464–9. doi: 10.1055/a-0740-8773
78. Sendra S, Parra L, Lloret J, Tomás J. Smart system for children's chronic illness monitoring. Inf Fusion. (2018) 40:76–86. doi: 10.1016/j.inffus.2017.06.002
79. Waite-Jones JM, Majeed-Ariss R, Smith J, Stones SR, Van Rooyen V, Swallow V. Young people's, parents', and professionals' views on required components of mobile apps to support self-management of juvenile arthritis: qualitative study. JMIR Mhealth Uhealth. (2018) 6:e25. doi: 10.2196/mhealth.9179
80. Salaffi F, Gasparini S, Ciapetti A, Gutierrez M, Grassi W. Usability of an innovative and interactive electronic system for collection of patient-reported data in axial spondyloarthritis: comparison with the traditional paper-administered format. Rheumatol. (2013) 52:2062–70. doi: 10.1093/rheumatology/ket276
81. Wilson AS, Kitas GD, Carruthers DM, Reay C, Skan J, Harris S, et al. Computerized information-gathering in specialist rheumatology clinics: an initial evaluation of an electronic version of the Short Form 36. Rheumatology. (2002) 41:268–73. doi: 10.1093/rheumatology/41.3.268
82. Richter JG, Becker A, Koch T, Nixdorf M, Widers R, Monser R, et al. Self-assessments of patients via Tablet PC in routine patient care: comparison with standardised paper questionnaires. Ann Rheum Dis. (2008) 67:1739–41. doi: 10.1136/ard.2008.090209
83. Campbell N, Ali F, Finlay AY, Salek SS. Equivalence of electronic and paper-based patient-reported outcome measures. Qual Life Res. (2015) 24:1949–61. doi: 10.1007/s11136-015-0937-3
84. Krusche M, Klemm P, Grahammer M, Mucke J, Vossen D, Kleyer A, et al. Acceptance, usage, and barriers of electronic patient-reported outcomes among german rheumatologists: survey study. JMIR Mhealth Uhealth. (2020) 8:e18117. doi: 10.2196/18117
85. El Miedany Y, El Gaafary M, Palmer D. Assessment of the utility of visual feedback in the treatment of early rheumatoid arthritis patients: a pilot study. Rheumatol Int. (2012) 32:3061–8. doi: 10.1007/s00296-011-2098-1
86. Schreier G, Hayn D, Kastner P, Koller S, Salmhofer W, Hofmann-Wellenhof R. A mobile-phone based teledermatology system to support self-management of patients suffering from psoriasis. Annu Int Conf IEEE Eng Med Biol Soc. (2008) 2008:5338–41. doi: 10.1109/IEMBS.2008.4650420
87. Abroms LC, Padmanabhan N, Thaweethai L, Phillips T. iPhone apps for smoking cessation: a content analysis. Am J Prev Med. (2011) 40:279–85. doi: 10.1016/j.amepre.2010.10.032
88. Knitza J, Tascilar K, Messner EM, Meyer M, Vossen D, Pulla A, et al. German mobile apps in rheumatology: review and analysis using the mobile application rating scale (MARS). JMIR mHealth uHealth. (2019) 7:e14991. doi: 10.2196/14991
89. Grainger R, Townsley H, White B, Langlotz T, Taylor WJ. Apps for people with rheumatoid arthritis to monitor their disease activity: a review of apps for best practice and quality. JMIR mHealth uHealth. (2017) 5:e6956. doi: 10.2196/mhealth.6956
90. Najm A, Nikiphorou E, Kostine M, Richez C, Pauling JD, Finckh A, et al. EULAR points to consider for the development, evaluation and implementation of mobile health applications aiding self-management in people living with rheumatic and musculoskeletal diseases. RMD Open. (2019) 5:e001014. doi: 10.1136/rmdopen-2019-001014
91. Meyer AND Giardina TD Spitzmueller C Shahid U Scott TMT Singh H. Patient perspectives on the usefulness of an artificial intelligence-assisted symptom checker: cross-sectional survey study. J Med Internet Res. (2020) 22:e14679. doi: 10.2196/14679
92. Proft F, Spiller L, Redeker I, Protopopov M, Rodriguez VR, Muche B, et al. Comparison of an online self-referral tool with a physician-based referral strategy for early recognition of patients with a high probability of axial spa. Semin Arthritis Rheum. (2020) 50:1015–21. doi: 10.1016/j.semarthrit.2020.07.018 93.
93. Powley L, Mcilroy G, Simons G, Raza K. Are online symptoms checkers useful for patients with inflammatory arthritis? BMC Musculoskelet Disord. (2016). 17:362. doi: 10.1186/s12891-016-1189-2
94. Morf H, Krusche M, Knitza J. Patient self-sampling: a cornerstone of future rheumatology care? Rheumatol Int. (2021) 41:1187–188. doi: 10.1007/s00296-021-04853-z
95. Knitza J, Knevel R, Raza K, Bruce T, Eimer E, Gehring I, et al. Toward earlier diagnosis using combined eHealth tools in rheumatology: the joint pain assessment scoring tool (JPAST) project. JMIR Mhealth Uhealth. (2020) 8:e17507. doi: 10.2196/17507
96. Webers C, Beckers E, Boonen A, van Eijk-Hustings Y, Vonkeman H, van de Laar M, et al. Development, usability and acceptability of an integrated eHealth system for spondyloarthritis in the Netherlands (SpA-Net). RMD Open. (2019) 5:e000860. doi: 10.1136/rmdopen-2018-000860.
97. Ji X, Hu L, Wang Y, Luo Y, Zhu J, Zhang J, et al. “Mobile Health” for the management of spondyloarthritis and its application in China. Curr Rheumatol Rep. (2019) 21:1–6. doi: 10.1007/s11926-019-0860-7
98. Krusche M, Burmester GR, Knitza J. Digital crowdsourcing: unleashing its power in rheumatology. Ann Rheum Dis. (2020) 79:1139–40. doi: 10.1136/annrheumdis-2020-217697
99. Norgeot B, Glicksberg BS, Trupin L, Lituiev D, Gianfrancesco M, Oskotsky B, et al. Assessment of a deep learning model based on electronic health record data to forecast clinical outcomes in patients with rheumatoid arthritis. JAMA Netw Open. (2019) 2:e190606. doi: 10.1001/jamanetworkopen.2019.0606
100. Guan Y, Zhang H, Quang D, Wang Z, Parker SCJ, Pappas DA, et al. Machine learning to predict anti–tumor necrosis factor drug responses of rheumatoid arthritis patients by integrating clinical and genetic markers. Arthritis Rheumatol. (2019) 71:1987–96. doi: 10.1002/art.41056
101. Orange DE, Agius P, DiCarlo EF, Robine N, Geiger H, Szymonifka J, et al. Identification of three rheumatoid arthritis disease subtypes by machine learning integration of synovial histologic features and RNA sequencing data. Arthritis Rheumatol. (2018) 70:690–701. doi: 10.1002/art.40428
102. Eng SWM, Olazagasti JM, Goldenberg A, Crowson CS, Oddis C V, Niewold TB, et al. A clinically and biologically based subclassification of the idiopathic inflammatory myopathies using machine learning. ACR Open Rheumatol. (2020) 2:158–66. doi: 10.1002/acr2.11115
103. Speerin R, Slater H, Li L, Moore K, Chan M, Dreinhöfer K, et al. Moving from evidence to practice: models of care for the prevention and management of musculoskeletal conditions. Best Pract Res Clin Rheumatol. (2014) 28:479–515. doi: 10.1016/j.berh.2014.07.001
104. Zabotti A, McGonagle DG, Giovannini I, Errichetti E, Zuliani F, Zanetti A, et al. Transition phase towards psoriatic arthritis: clinical and ultrasonographic characterisation of psoriatic arthralgia. RMD Open. (2019) 5:e001067. doi: 10.1136/rmdopen-2019-001067
105. Husni ME, Meyer KH, Cohen DS, Mody E, Qureshi AA. The PASE questionnaire: pilot-testing a psoriatic arthritis screening and evaluation tool. J Am Acad Dermatol. (2007) 57:581–7. doi: 10.1016/j.jaad.2007.04.001
106. Li LC, Adam PM, Backman CL, Lineker S, Jones CA, Lacaille D, et al. Proof-of-concept study of a web-based methotrexate decision aid for patients with rheumatoid arthritis. Arthritis Care Res. (2014) 66:1472–81. doi: 10.1002/acr.22319
Keywords: psoriatic arthritis, telemedicine, eHealth, mHealth, digital rheumatology, psoriasis
Citation: Fagni F, Knitza J, Krusche M, Kleyer A, Tascilar K and Simon D (2021) Digital Approaches for a Reliable Early Diagnosis of Psoriatic Arthritis. Front. Med. 8:718922. doi: 10.3389/fmed.2021.718922
Received: 01 June 2021; Accepted: 07 July 2021;
Published: 11 August 2021.
Edited by:
Carl Kieran Orr, Saint Vincent's University Hospital, IrelandReviewed by:
Alen Zabotti, Università degli Studi di Udine, ItalyCopyright © 2021 Fagni, Knitza, Krusche, Kleyer, Tascilar and Simon. This is an open-access article distributed under the terms of the Creative Commons Attribution License (CC BY). The use, distribution or reproduction in other forums is permitted, provided the original author(s) and the copyright owner(s) are credited and that the original publication in this journal is cited, in accordance with accepted academic practice. No use, distribution or reproduction is permitted which does not comply with these terms.
*Correspondence: David Simon, ZGF2aWQuc2ltb25AdWstZXJsYW5nZW4uZGU=
Disclaimer: All claims expressed in this article are solely those of the authors and do not necessarily represent those of their affiliated organizations, or those of the publisher, the editors and the reviewers. Any product that may be evaluated in this article or claim that may be made by its manufacturer is not guaranteed or endorsed by the publisher.
Research integrity at Frontiers
Learn more about the work of our research integrity team to safeguard the quality of each article we publish.