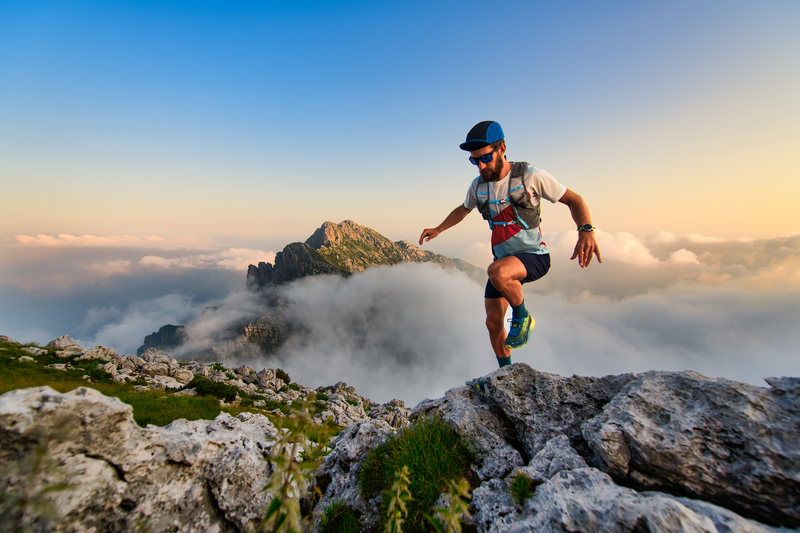
94% of researchers rate our articles as excellent or good
Learn more about the work of our research integrity team to safeguard the quality of each article we publish.
Find out more
REVIEW article
Front. Med. , 06 July 2021
Sec. Regulatory Science
Volume 8 - 2021 | https://doi.org/10.3389/fmed.2021.688438
This article is part of the Research Topic Women in Science - Regulatory Science 2021 View all 13 articles
Diabetes Mellitus is one of the World Health Organization's priority diseases under research by the first and second programmes of Innovative Medicines Initiative, with the acronyms IMI1 and IMI2, respectively. Up to October of 2019, 13 projects were funded by IMI for Diabetes & Metabolic disorders, namely SUMMIT, IMIDIA, DIRECT, StemBANCC, EMIF, EBiSC, INNODIA, RHAPSODY, BEAT-DKD, LITMUS, Hypo-RESOLVE, IM2PACT, and CARDIATEAM. In general, a total of €447 249 438 was spent by IMI in the area of Diabetes. In order to prompt a better integration of achievements between the different projects, we perform a literature review and used three data sources, namely the official project's websites, the contact with the project's coordinators and co-coordinator, and the CORDIS database. From the 662 citations identified, 185 were included. The data collected were integrated into the objectives proposed for the four IMI2 program research axes: (1) target and biomarker identification, (2) innovative clinical trials paradigms, (3) innovative medicines, and (4) patient-tailored adherence programmes. The IMI funded projects identified new biomarkers, medical and research tools, determinants of inter-individual variability, relevant pathways, clinical trial designs, clinical endpoints, therapeutic targets and concepts, pharmacologic agents, large-scale production strategies, and patient-centered predictive models for diabetes and its complications. Taking into account the scientific data produced, we provided a joint vision with strategies for integrating personalized medicine into healthcare practice. The major limitations of this article were the large gap of data in the libraries on the official project websites and even the Cordis database was not complete and up to date.
Innovative Medicines Initiative (IMI) is a unique pan-European public and private partnership that pioneered large-scale open collaborations between large pharmaceutical companies, small and medium-sized enterprises, public authorities (including regulators), organizations of patients, academia, and clinical centers to throw bottlenecks in research and development (R&D) of new effective and safer medicines (1).
To implement the Innovative Medicines Initiative, the European Commission and the European Federation of Pharmaceutical Industries and Associations (EFPIA) hold joint responsibility for creating and operating a new non-profit international organization (1).
IMI aims to accelerate the discovery and development of more effective vaccines, medicines, and treatments with fewer side-effects, especially in areas where there is an unmet medical or social need. IMI intends to implement patient centered projects, prompting the patient access to innovative pharmaceutical options (1, 2). This initiative provide socio-economic benefits and contribute to the health of European citizens, minimize duplication of work at different organizations, increase competitiveness, and help to establish Europe as the most attractive and competitive site for innovation (1, 2).
The first programme of IMI (IMI1) was created by Council Regulation (EC) n.° 73/2008, of 20th December 2007. The overall aim was to support pre-competitive pharmaceutical research and development, through the funding of innovative patient-centered projects for the research of European health priorities defined by the World Health Organization (WHO) (3). IMI1 programme was based on four strategic interdependent areas (Four-pillars) namely Safety, Efficacy, Knowledge Management, and Education and Training (1). The vision of this programme consisted on the creation of new scientific knowledge and capabilities/techniques to support the ability to identify a lack of efficacy or safety quickly in all stages of the medicine development process, even when a potential medicine has promising pre-clinical data (1). In addition, IMI1 programme intended to support the benefit-risk assessment conducted by the regulatory authorities (1, 2).
For this initiative, the budget committed was €2 billion (2, 4). During the execution period of IMI1 programme (2008 to 2013), eleven calls for proposals were released, which resulted in 59 funded-projects (4, 5).
The success of the IMI1 programme prompted the European Commission and the European Federation of Pharmaceutical Industries and Associations to take the commencing of initiating a second IMI programme (IMI2) under the Horizon 2020 vision of “improve the health and well-being of populations, reduce health inequalities, and ensure sustainable people-centered health systems” (5). Innovative Medicines Initiative 2 Joint Undertaking was established by Regulation (EU) n.° 557/2014, 6th of May (6). The major research axes recognized for IMI2 were: target & biomarker identification, innovative clinical trial paradigms, innovative medicines, and patient tailored adherence programmes (5). This programme ran from 2014 to 2020 and the budget committed was up to €3.276 billion, half funded by the European Comission and the other part from EFPIA.
Diabetes mellitus (DM) is one of the eleven priority diseases addressed by IMI1 and IMI2 programmes in the Strategic Reseach Agenda. This is a chronic metabolic disorder characterized by a defined phenotype (hyperglycemia accompanied by greater or lesser impairment in the metabolism of carbohydrates, lipids, and proteins), triggered by either lack of insulin secretion or decreased sensitivity of the tissues to insulin (7–9). Worldwide, a majority of diabetic patients (80–90%) have type 2 diabetes (T2D) and 5–10% type 1 diabetes (T1D) (8).
In 2014, WHO estimated that the prevalence of diabetes could reach more than 20% of the world's population within the next 20 years (8, 10, 11). Besides, the diabetes-associated mortality rate has been increasing, being the seventh leading cause of death in 2016 (12), and the disease and its acute and chronic complications represent a major economic burden on the global healthcare system and the wider global economy (5). For all the factors, previously presented, WHO considered this disease as the pandemic of the 21st century (8).
With the purpose of slowing the increasing prevalence, decreasing the mortality rate and diminishing the economic burden of diabetes and its related complications, IMI focused on projects aimed at understanding T1D and T2D, developing new precision medicines, identifying better patient-focused outcome measures for diagnosis, treatment selection and prognosis of T1D, T2D, and complications of diabetes, as well as promoting better lifestyle management and adherence to prescribed medicines (1, 5).
IMI1 programme funded six projects in the Diabetes & Metabolic disorders field, namely: Surrogate markers for micro- and macro-vascular hard endpoints for innovative diabetes tools (SUMMIT), Improving beta-cell function and identification of diagnostic biomarkers for treatment monitoring in diabetes (IMIDIA), Diabetes research on patient stratification (DIRECT), Stem cells for biological assays of novel medicines and predictive toxicology (StemBANCC), European Medical Information Framework (EMIF), and European Bank for induced pluripotent Stem Cells (EBiSC).
In IMI2 programme, until October of 2019, seven projects were supported in the area of Diabetes & metabolic disorders (13). These projects were: Translational approaches to disease modifying therapy of type 1 diabetes: an innovative approach toward understanding and arresting type 1 diabetes (INNODIA), Assessing risk and progression of prediabetes and type 2 diabetes to enable disease modification (RHAPSODY), Biomarker enterprise to attack DKD (BEAT-DKD), Liver Investigation: Testing Marker Utility in Steatohepatitis (LITMUS), Hypoglycaemia–redefining solutions for better lives (Hypo-RESOLVE), Investigating mechanisms and models predictive of accessibility of therapeutics into the brain (IM2PACT), and Cardiomyopathy in type 2 diabetes mellitus (CARDIATEAM).
Of the 13 projects, one was targeted to type 1 diabetes—INNODIA, three to type 2 diabetes—DIRECT, EMIF, and RHAPSODY, four to complications of diabetes—SUMMIT, BEAT-DKD, LITMUS, Hypo-RESOLVE, and CARDIATEAM, and the remaining four were scientific-oriented—StemBANCC, EBiSC, IMIDIA, and IMI2PACT. A more detailed description of the projects and its objectives is available in Supplementary Material section.
A total of €447.249.438 was mobilized for Diabetes (€253.865.866 from IMI1 and €193.383.572 from IMI2), however there has not been a systematization of scientific production by the IMI-funded projects.
The purpose of this literature review was to summarize the project results of IMI1 and IMI2 programmes into the major research axes of IMI2 programme and propose a joint vision model including the data collected into two inter-dependent paths, one scientific-oriented and the other medical-oriented.
The data sources used in this review were the IMI website, the official project websites, contact with the project coordinators and co-coordinators, and the CORDIS (The Community Research and Development Information Service) database. From IMI website it was collected the project's start and end date, the grant agreement number, the contributions, and the coordinators and co-coordinators' e-mail addresses. The aim of each project was retrieved from its official website. The sources of the publications were the project's official website, CORDIS database, and the contact via e-mail with the coordinators and co-coordinators (Table 1).
The contacts with the coordinators and co-coordinators were conducted in January of 2019 and for non-respondents, a recall in February of 2019. This step was performed for all projects.
The literature research on the project's websites and the CORDIS databases was conducted from February 2019 to October 2019. In October 2019, a new consultation was conducted on the IMI website, and the new funded projects (IMI2PACT and CARDIATEAM) were included.
For SUMMIT's project, a total of 98 citations were screened, 52 from the SUMMIT's website and 46 from the CORDIS database. A total of 67 references were excluded: (i) duplicates−29, (ii) book chapters−2, (iii) not access to the full text−7, (iv) the publication's objective was not related to diabetes mellitus or its complications−9, and (v) the publication's achievements did not allow to induce a scientific advance in Diabetes field (e.g., state of the art, outdated information, the article's data don't address an objective of the IMI2 programme)−20. For this project, a total of 31 articles were included.
For IMIDIA's project, a total of 29 citations were screened, 13 from the IMIDIA's website and 12 from CORDIS database. A total of 11 references were excluded: (i) duplicates−5, (ii) book chapters−1, (iii) the publication's objective was not related with diabetes mellitus or its complications−3, and (iv) the publication's achievements did not allow to induce a scientific advance in Diabetes field (e.g., state of the art, outdated information, the article's data don't address an objective of the IMI2 programme)−2. For this project, a total of 18 articles were included.
For DIRECT's project, a total of 25 citations were screened on the list of publications sent by the project coordinator. Since this list is not available online (either on the project's website or on CORDIS database), we present it in the Supplementary Material section. A total of nine references were excluded: (i) duplicates−1, (ii) not access to the full text−2, and (iii) the publication's achievements did not allow to induce a scientific advance in Diabetes field (e.g., state of the art, outdated information, the article's data don't address an objective of the IMI2 programme)−6. For this project, a total of 16 articles were included.
For StemBANCC's project, a total of 122 citations were screened, 91 from the StemBANCC's website and 31 from CORDIS database. A total of 103 references were excluded: (i) duplicates−30, (ii) book chapters−1, (iii) the publication's objective was not related to diabetes mellitus or its complications−71, and (iv) the publication's achievements did not allow to induce a scientific advance in Diabetes field (e.g., state of the art, outdated information, the article's data don't address an objective of the IMI2 programme)−1. For this project, a total of 19 articles were included.
For EMIF's project, a total of 165 citations were screened, all from the EMIF's website. A total of 136 references were excluded: (i) duplicates−1, (ii) article's exclusion criterion was the presence of diabetes−1, (iii) the publication's objective was not related with diabetes mellitus or its complications−120, and (iv) the publication's achievements did not allow to induce a scientific advance in Diabetes field (e.g., state of the art, outdated information, the article's data don't address an objective of the IMI2 programme)−14. For this project, a total of 29 articles were included.
For EBiSC's project, a total of 15 citations were screened, six from the EBiSC's website and 9 from CORDIS database. A total of 14 references were excluded: (i) the publication's objective was not related to diabetes mellitus or its complications (e.g., state of the art, outdated information, the article's data don't address an objective of the IMI2 programme)−14. For this project, a total of one article was included.
For INNODIA's project, a total of 79 citations were screened, 47 from the INNODIA's website and 32 from CORDIS database. A total of 34 references were excluded: (i) duplicates−32, (ii) not access to the full text−1, and (iii) the publication's achievements did not allow to induce a scientific advance in Diabetes field (e.g., state of the art, outdated information, the article's data don't address an objective of the IMI2 programme)−11. For this project, a total of 35 articles were included.
For RHAPSODY's project, a total of 39 citations were screened, 20 from the RHAPSODY's website and 19 from CORDIS database. A total of 28 references were excluded: (i) duplicates−21, (ii) book chapters−1, and (iii) the publication's achievements did not allow to induce a scientific advance in Diabetes field (e.g., state of the art, outdated information, the article's data don't address an objective of the IMI2 programme)−6. For this project, a total of 11 articles were included.
For BEAT-DKD's project, a total of 90 citations were screened, 52 from the BEAT-DKD's website and 38 from CORDIS database. A total of 65 references were excluded: (i) duplicates−40, (ii) the publication's objective was not related to diabetes mellitus or its complications−10, and (iii) the publication's achievements did not allow to induce a scientific advance in Diabetes field (e.g., state of the art, outdated information, the article's data don't address an objective of the IMI2 programme)−15.
No results were identified in LITMUS, Hypo-RESOLVE, IMI2PACT, and CARDIATEAM projects.
The search and screening processes are summarized in Figure 1.
The results gathered in the literature review were integrated into the axes presented by the IMI2 programme, namely target & biomarker identification, innovative clinical trials paradigms, innovative medicines, and patient-tailored adherence programmes.
The data collected was organized according to the objectives established for each axis by the Strategic Research Agenda (SRA).
In Target & Biomarker identification axis, from the 10 objectives outlined in SRA, those achieved were (1) identify and validate biological markers, tools and assays, (2) determinants of inter-individual variability, (3) understand the molecular mechanisms underlying the disease, (4) develop a platform of pre-clinical assays, and (5) develop systems models.
For the “innovative clinical trial paradigms” axis, the data applied two of the twelve objectives defined in SRA, especially (1) utilize innovative endpoints, trial designs, simulation and analytical approaches to devise new clinical trial paradigms and (2) develop innovative clinical endpoints.
In the innovative medicines axis, from the eleven objectives in SRA, those with results were: (1) identify new or alternative therapeutic concepts (targets) for treatment and prevention of disease, (2) develop novel therapeutic agents and disease prevention strategies, and (3) as implement new approaches for the development and production of biopharmaceuticals and tissue engineering.
Lastly, from the seven objectives in SRA, the data collected for maximizing patient-tailored adherence programmes address only one goal, namely develop patient-centered predictive models.
In short, the outline of the projects with results in the research axes of the IMI2 programme, namely target & biomarker identification, innovative clinical trials paradigms, innovative medicines, and patient-tailored adherence programmes, is displayed in Figure 2.
No outputs were identified in LITMUS, Hypo-RESOLVE, IMI2PACT, and CARDIATEAM projects, as these were starting close to or during the literature search process.
Due to the quantity and diversity of data collected, we summarized the results obtained by each IMI funded-project in figures that are presented in Supplementary Material section.
A wide range of biomarkers have been identified for the onset of type 1 diabetes (14–24) by INNODIA; for risk prediction (14, 15, 17–21) and identification of patients at high-risk of type 2 diabetes (25) by SUMMIT, IMIDIA, DIRECT, and EMIF; for pancreatic β-cells function and protection by RHAPSODY (26, 27) and INNODIA (14, 16, 22–24); for hyperglycemia by RHAPSODY (28); for protection, prediction, initiation, progression, patient stratification, and medicine efficacy of diabetic kidney disease (DKD) (29, 30) by SUMMIT and BEAT-DKD (31–39); for development of cardiovascular disease (CVD) by SUMMIT (30, 40, 41); and for the development of diabetic retinopathy (DR) by SUMMIT (30, 42, 43).
Additionally, several novel tools were identified for diabetes, T1D, T2D, diabetic complications, and genetic research. The tools for diabetes intended to diagnose and monitoring disease progression [IMIDIA (44–49), DIRECT (50), and RHAPSODY (51)]. The tools designed for T1D were focused on monitor β-cell function, screen individuals at high risk, and select the more benefic intervention [INNODIA (15, 52, 53)]. For T2D, a new test was proposed to follow-up patients' insulin treatment need [EMIF (54, 55)]. Regarding diabetic complications, new tools were developed to enable the detection of patients at high risk of developing CVD and DR [SUMMIT (30, 56, 57)]. At last, in genetic research area, new tools were validated for the identification of single nucleotide polymorphism [SUMMIT (58–61) and StemBANCC (62, 63)].
Concerning the novel determinants of inter-individual variability, SUMMIT (43, 60, 64–72), IMIDIA (46, 49, 73–76), DIRECT (50, 77–82), EMIF (83–89), INNODIA (22, 23, 90–93), and RHAPSODY (27, 28, 94, 95) proposed a significant number of genetic markers for predisposition, initiation, identification, and progression of diabetes and its complications. Additionally, SUMMIT and DIRECT verified the influence of genetic factors in patients' medicine-response (96–101), and BEAT-DKD identified a non-genetic inter-individual therapeutic variability factor, i.e., NT-proBNP levels (37, 102, 103). DIRECT also confirmed the influence of gut composition (99, 100), age of diagnosis (50), year of diagnosis (104), and BMI factors (105) on the onset of diabetes, and EMIF showed the association with other factors such as ethnicity and metabolic health on T2D risk and development of complications (106). Moreover, two models of patient stratification were proposed, one by INNODIA for glycemic control in patients with T1D (107) and another by RHAPSODY and BEAT-DKD related with the identification of the patient's risk level for certain diabetic complications (108).
Novel relevant pathways were proposed to understand β-cell development and function [by IMIDIA (109–113), StemBANCC (114–119), and RHAPSODY (27)], type 1 diabetes (by INNODIA (16, 20, 23, 120–125), type 2 diabetes [by DIRECT (50), EMIF (85, 126–130), and Rhapsody (131)], CVD [SUMMIT (30, 132) and INNODIA (133)], DKD [BEAT-DKD (32, 36, 134–138)], DR [SUMMIT (30, 42, 43)], endometrial cancer risk [EMIF (139)], dementia [EMIF (140)], non-alcoholic fatty liver disease [EMIF (54, 55, 141–143)], anorexia or bulimia [INNODIA (125)], and attention-deficit hyperactivity disorder [INNODIA (125)].
In terms of pre-clinical studies, StemBANCC (114, 144, 145), EBiSC (146, 147), and IMIDIA (45, 49) proposed innovative iPSCs lines derived from diabetes and created their own databases; StemBANCC, INNODIA and RHAPSODY developed three catalogs, namely β-cell' Bi-DOCS (148), HLA-I peptidome of β-cells (21) and cis-eQTLs for T2D (149); IMIDIA (44–49), StemBANCC (144, 150), and RHAPSODY (151) established several protocols to improve the reliability of laboratory studies; and SUMMIT (30, 152, 153) and IMIDIA (46) developed new specific animal models.
Regarding systems models, two new in silico models were generated by SUMMIT (30), one for clinical complications in T1D and the other for aspirin action. In addition, BEAT-DKD proposed three models associated with DKD, namely the Drosophila nephrocyte to reveal mechanisms of podocyte function and glomerular diseases (154), the systems biology to better prediction of patient's medicine- response (155), and an in-silico analysis to identify compounds reversing a set of renal age-associated genes associated with the disease's progression (32).
For clinical trials, two novel design models were proposed, one by DIRECT and a second by BEAT—DKD: the Genotype-based Recall (GBR) (156) and “umbrella” or “platform” trials (157, 158), respectively. Regarding the innovative clinical endpoints, DIRECT validated a prediction model for T2D–DIRECT-DETECT, which may be used in the selection process in clinical trials (159, 160).
New potential therapeutic targets were suggested for the treatment of accelerated atherosclerosis in diabetic patients (161) (SUMMIT), for treating glomerular disease in T2D patients (36, 135, 162–164) (SUMMIT), for T2D patients with obesity (165) (EMIF), for counteracting hyperglycemia in individuals with T2D (166) (IMIDIA), for prevent or reverse β-cell loss [IMIDIA (167), StemBANCC (114, 116, 168, 169) and INNODIA (14, 90, 120, 121, 170–172)], and for diabetes with a focus on the use of new concepts such as epitranscriptome-based therapy by RHAPSODY (173), StemBANCC (114–116, 168, 169, 174), EMIF (86), and RHAPSODY (173). In terms of novel pharmacologic agents for T2D, SUMMIT developed the clinical trials of Aleglitazar (175), RAAS inhibitors (176), and supported the use of low-dose aspirin for the secondary prevention of cerebro-cardiovascular events (177).
Furthermore, DIRECT demonstrated the cardio-metabolic benefit of metformin (178), BEAT-DKD supported the clinical efficacy of SGLT2 inhibitors and GLP1R agonists in diabetic patients with DKD (179, 180), and StemBANCC established four different stem cell-based replacement treatments (181–183). For large-scale production, StemBANCC demonstrated that the continuous peristaltic pump-based circulation technology, in a hydraulically driven bioreactor, can be a potential 3D tool and a key in this process (184).
Lastly, it was established two patient-centered screening tools for T2D, more precisely the “palette” model, based on molecular taxonomy, and the DIRECT-DETECT prediction model, composed by glycaemic deterioration biomarkers (159, 160, 185).
Based on the objectives of the IMI funded-projects and the results previously mentioned, we propose an integrated model addressing diabetes in its multiple dimensions, which includes two inter-dependent paths that should be executed in parallel, the first one being more scientific-oriented and the second one medical-oriented, as illustrated in Figure 3.
Figure 3. Integrated vision model for diabetes, based on the objectives and results of the IMI funded-projects. Color meaning: gray, general population; green, individuals at low risk of diabetes; yellow, individuals at medium risk of diabetes; orange, individuals at medium-high risk of diabetes; red, individuals at high risk of diabetes; dark orange, individuals at high risk of DKD; dark red, individuals at high risk of CVD; brown, individuals at high risk of DR; light red, individuals at high risk of NAFLD; and light orange, individuals at high risk of hypoglycaemia. BEAT-DKD, Biomarker Enterprise to Attack DKD Project; CARDIATEAM, Cardiomyopathy in Type 2 Diabetes Mellitus Project; CVD, Cardiovascular diseases; DIRECT, Diabetes Research on Patient Stratification Project; DKD, Diabetic Kidney Disease; DR, Diabetic Retinopathy; EBiSC, European Bank for induced pluripotent Stem Cells Project; EMIF, European Medical Information Framework Project; Hypo-RESOLVE, Hypoglycaemia, Redefining Solutions for Better Lives Project; IMIDIA, Improving Beta-cell Function and Identification of Diagnostic Biomarkers for Treatment Monitoring in Diabetes Project; INNODIA, Translational Approaches to Disease Modifying Therapy of Type 1 Diabetes: An Innovative Approach Toward Understanding and Arresting Type 1 Diabetes Project; LITMUS, Liver Investigation: Testing Marker Utility in Steatohepatitis Project; NAFLD, Non-Alcoholic Fatty Liver disease; RHAPSODY, Assessing Risk and Progression of Prediabetes and Type 2 Diabetes to Enable Disease Modification Project; StemBANCC, Stem Cells for Biological Assays of Novel Medicines and Predictive Toxicology Project; SUMMIT, Surrogate Markers for Micro- and Macro-Vascular Hard Endpoints for Innovative Diabetes Tools Project.
The scientific dimension would include the acquisition of more biological samples and genetic data and with the help of SUMMIT, IMIDIA, EBiSC, StemBANCC, EMIF, and IMI2PACT promote the research on β-cells as well as validate new biomarkers, genetic markers, patient stratification, discover more molecular mechanisms/pathways, and develop new treatments for T1D, T2D and diabetic complications. Besides, this approach aims at conducting clinical trials with more safety and efficacy endpoints through the application of those identified by SUMMIT, DIRECT, INNODIA, and BEAT-DKD to allow a marketing authorization of innovative medicines/therapeutics in a shorter time, less expensive and more focused on personalized medicine.
The medical dimension would include the use of predisposition markers developed by IMIDIA, DIRECT and INNODIA to identify people at higher risk of developing diabetes, with a particular interest in T2D, promoting the possibility of early intervention mainly in lifestyle habits, diet and physical exercise, and thus delaying the disease. Following the natural cycle of the disease, the objective would be to diagnose the recent-onset patients, through imaging technologies, tools and patient-centered models for clinicians developed by DIRECT, IMIDIA, INNODIA and RHAPSODY. Subsequently, the characterization of the subtype of patient and the treatment selection would be performed through the application of the DIRECT, INNODIA or RHAPSODY/BEAT-DKD stratification models and considering the inter-individual factors that impact the patient's response to the therapeutic agents identified by SUMMIT and DIRECT. The monitoring of disease progression would be possible in case of implementation of biomarkers, genetic markers and tools created by SUMMIT, IMIDIA, DIRECT, INNODIA, EMIF and RHAPSODY, with adaptations of pharmacological treatment dose or medicines changes in case of inadequate response. Additionally, with the use of biomarkers, genetic factors and tools developed by SUMMIT and BEAT-DKD, it would be possible to identify patients who during the progression of the disease, are more likely to develop diabetic complications, enabling to act in advance. With the application of imaging technologies developed by SUMMIT and EMIF and the information provided by BEAT-DKD, LITMUS, Hypo-RESOLVE, and CARDIATEAM, it would also be possible to predict the identification of patients with diabetic complications, especially diabetic nephropathy, diabetic retinopathy, cardiovascular disease, hypoglycemia, and non-Alcoholic Fatty Liver disease. Through the use of genetic factors and biomarkers developed by SUMMIT and BEAT-DKD, it would be desirable to select the best pharmacological treatment option according to the patient's characteristics and then monitor the follow-up to retard/stabilize its progression.
Summarizing, our integrated vision model supports a clinical model directed primarily and mainly at prevention, through the individual genetic and biological knowledge; as a first-line intervention, acting in the delay of diabetes onset; and in cases of diagnosis, to promote treatment according to the subtype of patients and monitor the progression of the disease. Only in this way, it will be possible to decrease the incidence and mortality rate of diabetes, provide an increase in the patient's quality of life, ensure sustainable people-centered health systems, and minimize direct and indirect diabetes-related costs in health systems.
Overall, it was found that the target & biomarker identification and innovative medicines axis have more published data. This was because these were major bottlenecks addressed by IMI 1 programme and included goals that corresponded to the key unmet needs in Diabetes during the programme's execution period 2008–2013.
Our literature review is subject to some limitations. When collecting the projects' data, we found a large gap in their publication's library, mainly SUMMIT, IMIDIA, and DIRECT projects. On SUMMIT website, there were only publications between 2010 and 2014, however, on the Cordis database, we found publications up to 2018. Similarly, although the IMIDIA website only included publications from 2011 and 2012, the Cordis database had articles until 2014. Regarding the DIRECT project, its website had only assembled the publications of the participating companies, all published before obtaining funding. Other limitations include the unsuccessful response from the project's coordinators and co-coordinators, and the CORDIS database was also not updated and complete.
In order to reduce the incidence, the mortality rate, and the economic burden on healthcare systems, as well as to improve disease management, until October of 2019, IMI1 and IMI2 programmes funded 13 projects encompassing several bottlenecks identified for R&D and clinical practice in Diabetes area.
Taking into account the scientific production available by these projects, we prepared a joint vision model including two paths: one scientific-oriented and the other medical-oriented. The scientific dimension integrates the current knowledge regarding this disease, research tools, as well as clinical trial designs and endpoints to allow marketing authorization of new effective and safer medicines in a shorter time and less expensive. The medical dimension includes the application of predisposition markers (biological and genetic), diagnostic tools, stratification models, treatment selection, and monitoring the progression of the disease to prevent/delay the development of diabetic complications.
As IMI programmes fostered the enhancing of knowledge and the improvement of the medical practice (with better tools, medicines, and prediction models), being a big step for the implementation of personalized medicine, it is clear that this initiative has an important role in the scientific advances that have occurred in recent years.
In terms of future perspectives, the biggest bottleneck will be the implementation of the proposed joint vision model, or a similar one, into the clinical practice, although all IMI-funded projects highlight this trend as the only one able to provide an effective response in the treatment of chronic diseases, in particular diabetes, and there are already proves of shifts in the paradigm. Nevertheless, the involvement of key stakeholders, including patients, will always be essential to the success of this process.
MB was responsible for the conduct of the study, data extraction and analysis and drafted the manuscript. BS-L had the original idea for the manuscript and supervised study conduct. BS-L and CT participated in the collection of additional literature, contributed to the writing of the manuscript and revised it critically for important intellectual content. All authors read and approved the final manuscript.
The authors declare that the research was conducted in the absence of any commercial or financial relationships that could be construed as a potential conflict of interest.
The Supplementary Material for this article can be found online at: https://www.frontiersin.org/articles/10.3389/fmed.2021.688438/full#supplementary-material
1. Innovative Medicines Initiative. The Innovative Medicines Initiative (IMI) Research Agenda - Creating Biomedical R&D Leadership for Europe to Benefit Patients and Society. (2008). Available online at: http://www.imi.europa.eu/sites/default/files/uploads/documents/SRArevised2011.pdf%5Cnhttp://www.imi.europa.eu/sites/default/files/uploads/documents/imi-gb-006v2-15022008-research-agenda_en.pdf (accessed January 22, 2019).
2. Centre for Innovation in Regulatory Science (CIRS). Socio-Economic Impact Report on IMI1 projects. London: Clarivate's Centre (2021).
3. COUNCIL REGULATION (EC) No 73/2008 of 20 December 2007. Official Journal of the European Union (2008).
4. Innovative Medicines Initiative. History – the IMI Story so Far. (2014). Available online at: https://www.imi.europa.eu/about-imi/history-imi-story-so-far (accessed January 21, 2019).
5. IMI Publications. The Right Prevention and Treatment for the Right Patient at the Right Time - Strategic Reseach Data for Innovative Medicines Initiative 2. Brussels: IMI Publications (2014).
7. Kasper DL, Fauci AS, Hauser SL, Longo DL, Jameson JL. Harrison's Principles of Intermal Medicine. 19th ed. New York, NY: McGraw-Hill Education (2015).
9. Baynest HW. Classification, pathophysiology, diagnosis and management of diabetes mellitus. J Diabetes Metab. (2015) 6:1–9. doi: 10.4172/2155-6156.1000541
11. Zheng Y, Ley SH, Hu FB. Global aetiology and epidemiology of type 2 diabetes mellitus and its complications. Nat Rev Endocrinol. (2018) 14:88–98. doi: 10.1038/nrendo.2017.151
12. World Health Organization. The top 10 Causes of Death. Geneva: World Health Organization. (2018).
13. Project Factsheets | Diabetes & metabolic disorders | IMI Innovative Medicines Initiative. Available online at: https://www.imi.europa.eu/projects-results/project-factsheets?keywords=&status=All&call=All&programmes=All&disease_areas=DA4&products=All&tools=All (accessed August 19, 2019).
14. Meyerovich K, Violato NM, Fukaya M, Dirix V, Pachera N, Marselli L, et al. MCL-1 is a key anti-apoptotic protein in human and rodent pancreatic β-cells. Diabetes. (2018) 66:1–46. doi: 10.2337/db16-1252
15. Demine S, Balhuizen A, Debaille V, Joosten L, Fereau M, Chilla SNM, et al. Imaging of human insulin secreting cells with GD-dotA-P88, a paramagnetic contrast agent targeting the beta cell biomarker FxyD2γA. Molecules. (2018) 23:1–16. doi: 10.3390/molecules23092100
16. Paula FMM, Leite NC, Borck PC, Freitas-Dias R, Cnop M, Chacon-Mikahil MPT, et al. Exercise training protects human and rodent β cells against endoplasmic reticulum stress and apoptosis. FASEB J. (2018) 32:1524–36. doi: 10.1096/fj.201700710R
17. Mathieu C, Lahesmaa R, Bonifacio E, Achenbach P, Tree T. Immunological biomarkers for the development and progression of type 1 diabetes. Diabetologia. (2018) 61:2252–8. doi: 10.1007/s00125-018-4726-8
18. Colli ML, Hill JLE, Marroquí L, Chaffey J, Dos Santos RS, Leete P, et al. PDL1 is expressed in the islets of people with type 1 diabetes and is up-regulated by interferons-α and-γ via IRF1 induction. EBioMedicine. (2018) 36:367–75. doi: 10.1016/j.ebiom.2018.09.040
19. Buitinga M, Callebaut A, Sodré FMC, Crèvecoeur I, Blahnik-Fagan G, Yang ML, et al. Inflammation-induced citrullinated glucose-regulated protein 78 elicits immune responses in human type 1 diabetes. Diabetes. (2018) 67:2337–48. doi: 10.2337/db18-0295
20. Müller D, Telieps T, Eugster A, Weinzierl C, Jolink M, Ziegler AG, et al. Novel minor HLA DR associated antigens in type 1 diabetes. Clin Immunol. (2018) 194:87–91. doi: 10.1016/j.clim.2018.07.001
21. Gonzalez-Duque S, Azoury ME, Colli ML, Afonso G, Turatsinze JV, Nigi L, et al. Conventional and neo-antigenic peptides presented by β cells are targeted by circulating naïve CD8+ T Cells in type 1 diabetic and healthy donors. Cell Metab. (2018) 28:946–60. doi: 10.1016/j.cmet.2018.07.007
22. Harbige J, Eichmann M, Peakman M. New insights into non-conventional epitopes as T cell targets: the missing link for breaking immune tolerance in autoimmune disease? J Autoimmun. (2017) 84:12–20. doi: 10.1016/j.jaut.2017.08.001
23. Dotta F, Ventriglia G, Snowhite IV, Pugliese A. MicroRNAs: markers of β-cell stress and autoimmunity. Curr Opin Endocrinol Diabetes Obes. (2018) 25:237–45. doi: 10.1097/MED.0000000000000420
24. Santos RS, Marroqui L, Grieco FA, Marselli L, Suleiman M, Henz SR, et al. Protective role of complement C3 against cytokine-mediated β-cell apoptosis. Endocrinology. (2017) 158:2503–21. doi: 10.1210/en.2017-00104
25. Haid M, Muschet C, Wahl S, Römisch-Margl W, Prehn C, Möller G, et al. Long-term stability of human plasma metabolites during storage at−80°c. J Proteome Res. (2018) 17:203–11. doi: 10.1021/acs.jproteome.7b00518
26. Richards P, Rachdi L, Oshima M, Marchetti P, Bugliani M, Armanet M, et al. MondoA is an essential glucose responsive transcription factor in human pancreatic beta cells. Diabetes. (2018) 67:461–72. doi: 10.2337/db17-0595
27. Diedisheim M, Oshima M, Albagli O, Huldt CW, Ahlstedt I, Clausen M, et al. Modeling human pancreatic beta cell dedifferentiation. Mol Metab. (2018) 10:74–86. doi: 10.1016/j.molmet.2018.02.002
28. Gerst F, Jaghutriz BA, Staiger H, Schulte AM, Lorza-Gil E, Kaiser G, et al. The expression of aldolase B in islets is negatively associated with insulin secretion in humans. J Clin Endocrinol Metab. (2018) 103:4373–83. doi: 10.1210/jc.2018-00791
29. Lamerz J, Friedlein A, Soder N, Cutler P, Döbeli H. Determination of free desmosine in human plasma and its application in two experimental medicine studies. Anal Biochem. (2013) 436:127–36. doi: 10.1016/j.ab.2013.01.012
30. SUMMIT. IMI1 Final Project Report Public Summary - SUrrogate Markers for Vascular Micro- and Macrovascular Hard Endpoints for Innovative Diabetes Tools. SUMMIT (2016).
31. Lindenmeyer MT, Kretzler M. Renal biopsy-driven molecular target identification in glomerular disease. Pflugers Arch Eur J Physiol. (2017) 469:1021–8. doi: 10.1007/s00424-017-2006-y
32. Koppelstaetter C, Leierer J, Rudnicki M, Kerschbaum J, Kronbichler A, Melk A, et al. Computational drug screening identifies compounds targeting renal age-associated molecular profiles. Comput Struct Biotechnol J. (2019) 17:843–53. doi: 10.1016/j.csbj.2019.06.019
33. Wolf M, de Boer A, Sharma K, Boor P, Leiner T, Sunder-Plassmann G, et al. Magnetic resonance imaging T1- and T2-mapping to assess renal structure and function: a systematic review and statement paper. Nephrol Dial Transplant. (2018) 33:ii41–50. doi: 10.1093/ndt/gfy198
34. Barreiro K, Holthofer H. Urinary extracellular vesicles. A promising shortcut to novel biomarker discoveries. Cell Tissue Res. (2017) 369:217–27. doi: 10.1007/s00441-017-2621-0
35. Hamidi H, Kretzler M. Systems biology approaches to identify disease mechanisms and facilitate targeted therapy in the management of glomerular disease. Physiol Behav. (2017) 176:139–48. doi: 10.1097/MNH.0000000000000446
36. Henique C, Bollée G, Loyer X, Grahammer F, Dhaun N, Camus M, et al. Genetic and pharmacological inhibition of microRNA-92a maintains podocyte cell cycle quiescence and limits crescentic glomerulonephritis. Nat Commun. (2017) 8:1829. doi: 10.1038/s41467-017-01885-7
37. Idzerda NMA, Persson F, Pena MJ, Brenner BM, Brunel P, Chaturvedi N, et al. N-terminal pro-brain natriuretic peptide (NT-proBNP) predicts the cardio-renal response to aliskiren in patients with type 2 diabetes at high renal and cardiovascular risk. Diabetes Obes Metab. (2018) 20:2899–904. doi: 10.1111/dom.13465
38. Perco P, Mayer G. Endogenous factors and mechanisms of renoprotection and renal repair. Eur J Clin Invest. (2018) 48:1–13. doi: 10.1111/eci.12914
39. Perco P, Ju W, Kerschbaum J, Leierer J, Menon R, Zhu C, et al. Identification of dicarbonyl and L-xylulose reductase as a therapeutic target in human chronic kidney disease. JCI Insight. (2019) 4:e128120. doi: 10.1172/jci.insight.128120
40. Silveira A, McLeod O, Strawbridge RJ, Gertow K, Sennblad B, Baldassarre D, et al. Plasma IL-5 concentration and subclinical carotid atherosclerosis. Atherosclerosis. (2015) 239:125–30. doi: 10.1016/j.atherosclerosis.2014.12.046
41. Deleskog A, Piksasova O, Silveira A, Gertow K, Baldassarre D, Veglia F, et al. Serum 25-hydroxyvitamin D concentration in subclinical carotid atherosclerosis. Arterioscler Thromb Vasc Biol. (2013) 33:2633–8. doi: 10.1161/ATVBAHA.113.301593
42. Kinnunen K, Heinonen SE, Kalesnykas G, Laidinen S, Uusitalo-Järvinen H, Uusitalo H, et al. LDLR-/-ApoB100/100 mice with insulin-like growth factor II overexpression reveal a novel form of retinopathy with photoreceptor atrophy and altered morphology of the retina. Mol Vis. (2013) 19:1723–33.
43. Sandholm N, McKnight AJ, Salem RM, Brennan EP, Forsblom C, Harjutsalo V, et al. Chromosome 2q31.1 associates with ESRD in women with type 1 diabetes. J Am Soc Nephrol. (2013) 24:1537–43. doi: 10.1681/ASN.2012111122
44. Bötticher G, Sturm D, Ehehalt F, Knoch KP, Kersting S, Grützmann R, et al. Isolation of human islets from partially pancreatectomized patients. J Vis Exp. (2011) 53:2962. doi: 10.3791/2962
45. Ravassard P, Hazhouz Y, Pechberty S, Bricout-neveu E, Armanet M, Czernichow P, et al. A genetically engineered human pancreatic β cell line exhibiting glucose-inducible insulin secretion. J Clin Invest. (2011). 121:3589–97. doi: 10.1172/JCI58447
46. Rutter GA, Herrera PL, Cruciani-Guglielmacci C, Mourougavelou V, Magnan C, da Silva Xavier G, et al. Pancreatic alpha cell-selective deletion of Tcf7l2 impairs glucagon secretion and counter-regulatory responses to hypoglycaemia in mice. Diabetologia. (2017) 60:1043–50. doi: 10.1007/s00125-017-4242-2
47. Lamprianou S, Immonen R, Nabuurs C, Gjinovci A, Vinet L, Montet XCR, et al. High-resolution magnetic resonance imaging quantitatively detects individual pancreatic islets. Diabetes. (2011) 60:2853–60. doi: 10.2337/db11-0726
48. Kaminski MT, Lenzen S, Baltrusch S. Real-time analysis of intracellular glucose and calcium in pancreatic beta cells by fluorescence microscopy. Biochim Biophys Acta Mol Cell Res. (2012) 1823:1697–707. doi: 10.1016/j.bbamcr.2012.06.022
49. Rutter GA, Weitz J, Kleeberg M, Siddiq A, Meinel J, Burdet F, et al. Systems biology of the IMIDIA biobank from organ donors and pancreatectomised patients defines a novel transcriptomic signature of islets from individuals with type 2 diabetes. Diabetologia. (2017) 61:641–57. doi: 10.1007/s00125-017-4500-3
50. Donnelly LA, Zhou K, Doney ASF, Jennison C, Franks PW, Pearson ER. Rates of glycaemic deterioration in a real-world population with type 2 diabetes. Diabetologia. (2018) 61:607–15. doi: 10.1007/s00125-017-4519-5
51. Babbar R, Heni M, Peter A, de Angelis MH, Häring HU, Fritsche A, et al. Prediction of glucose tolerance without an oral glucose tolerance test. Front Endocrinol (Lausanne). (2018) 9:82. doi: 10.3389/fendo.2018.00082
52. Willemsen RH, Burling K, Barker P, Ackland F, Dias RP, Edge J, et al. Frequent monitoring of C-peptide levels in newly diagnosed type 1 subjects using dried blood spots collected at home. J Clin Endocrinol Metab. (2018) 103:3350–8. doi: 10.1210/jc.2018-00500
53. Pociot F. Capturing residual beta cell function in type 1 diabetes. Diabetologia. (2019) 62:28–32. doi: 10.1007/s00125-018-4768-y
54. Hyysalo J, Männistö VT, Zhou Y, Arola J, Kärjä V, Leivonen M, et al. A population-based study on the prevalence of NASH using scores validated against liver histology. J Hepatol. (2014) 60:839–46. doi: 10.1016/j.jhep.2013.12.009
55. Orešič M, Hyötyläinen T, Kotronen A, Gopalacharyulu P, Nygren H, Arola J, et al. Prediction of non-alcoholic fatty-liver disease and liver fat content by serum molecular lipids. Diabetologia. (2013) 56:2266–74. doi: 10.1007/s00125-013-2981-2
57. Hellberg S, Silvola JMU, Kiugel M, Liljenbäck H, Metsälä O, Viljanen T, et al. Type 2 diabetes enhances arterial uptake of choline in atherosclerotic mice: an imaging study with positron emission tomography tracer 18F-fluoromethylcholine. Cardiovasc Diabetol. (2016) 15:1–13. doi: 10.1186/s12933-016-0340-6
58. Malovini A, Barbarini N, Bellazzi R, De Michelis F. Hierarchical Naive Bayes for genetic association studies. BMC Bioinformatics. (2012) 13(Suppl. 1):S6. doi: 10.1186/1471-2105-13-S14-S6
59. Sambo F, Trifoglio E, Di Camillo B, Toffolo GM, Cobelli C. Bag of Naïve Bayes: biomarker selection and classification from genome-wide SNP data. BMC Bioinformatics. (2012) 13(Suppl. 1):S2. doi: 10.1186/1471-2105-13-S14-S2
60. Sambo F, Malovini A, Sandholm N, Stavarachi M, Forsblom C, Mäkinen VP, et al. Novel genetic susceptibility loci for diabetic end-stage renal disease identified through robust naive Bayes classification. Diabetologia. (2014) 57:1611–22. doi: 10.1007/s00125-014-3256-2
61. Di Camillo B, Sambo F, Toffolo G, Cobelli C. ABACUS: an entropy-based cumulative bivariate statistic robust to rare variants and different direction of genotype effect. Bioinformatics. (2014) 30:384–91. doi: 10.1093/bioinformatics/btt697
62. Sandor C, Beer NL, Webber C. Diverse type 2 diabetes genetic risk factors functionally converge in a phenotype-focused gene network. PLoS Comput Biol. (2017) 13:1–23. doi: 10.1371/journal.pcbi.1005816
63. Beer NL, Gloyn AL. Genome-edited human stem cell-derived beta cells: a powerful tool for drilling down on type 2 diabetes GWAS biology. F1000Research. (2016) 5:1711. doi: 10.12688/f1000research.8682.1
64. Xie W, Wood AR, Lyssenko V, Weedon MN, Knowles JW, Alkayyali S, et al. Genetic variants associated with glycine metabolism and their role in insulin sensitivity and type 2 diabetes. Diabetes. (2013) 62:2141–50. doi: 10.2337/db12-0876
65. Ahluwalia TS, Lindholm E, Groop LC. Common variants in CNDP1 and CNDP2, and risk of nephropathy in type 2 diabetes. Diabetologia. (2011) 54:2295–302. doi: 10.1007/s00125-011-2178-5
66. Sandholm N, Salem RM, McKnight AJ, Brennan EP, Forsblom C, Isakova T, et al. New susceptibility loci associated with kidney disease in type 1 diabetes. PLoS Genet. (2012) 8:e1002921. doi: 10.1371/journal.pgen.1002921
67. Fagerholm E, Ahlqvist E, Forsblom C, Sandholm N, Syreeni A, Parkkonen M, et al. SNP in the genome-wide association study hotspot on chromosome 9p21 confers susceptibility to diabetic nephropathy in type 1 diabetes. Diabetologia. (2012) 55:2386–93. doi: 10.1007/s00125-012-2587-0
68. He B, Österholm AM, Ojala JRM, Andersson AC, Tryggvason K. A remote cis-acting variant at 3q links glomerular NCK1 to diabetic nephropathy. PLoS ONE. (2013) 8:e56414. doi: 10.1371/journal.pone.0056414
69. Sandholm N, Forsblom C, Mäkinen VP, McKnight AJ, Österholm AM, He B, et al. Genome-wide association study of urinary albumin excretion rate in patients with type 1 diabetes. Diabetologia. (2014) 57:1143–53. doi: 10.1007/s00125-014-3202-3
70. Strawbridge RJ, Hilding A, Silveira A, Österholm C, Sennblad B, McLeod O, et al. Soluble CD93 is involved in metabolic dysregulation but does not influence carotid intima-media thickness. Diabetes. (2016) 65:2888–99. doi: 10.2337/db15-1333
71. Zeng B, Chen GL, Garcia-Vaz E, Bhandari S, Daskoulidou N, Berglund LM, et al. Orai channels are critical for receptor-mediated endocytosis of albumin. Nat Commun. (2017) 8:1920. doi: 10.1038/s41467-017-02094-y
72. Williams WW, Salem RM, McKnight AJ, Sandholm N, Forsblom C, Taylor A, et al. Association testing of previously reported variants in a large case-control meta-analysis of diabetic nephropathy. Diabetes. (2012) 61:2187–94. doi: 10.2337/db11-0751
73. Rutter J, Semplici F, Meur G, Fontés G, Diraison F, Fogarty S, et al. Human mutation within Per-Arnt-Sim (PAS) domain-containing Protein Kinase (PASK) causes basal insulin hypersecretion. J Biol Chem. (2011) 286:44005–14. doi: 10.1074/jbc.M111.254995
74. Di Paola R, Caporarello N, Marucci A, Dimatteo C, Iadicicco C, Del Guerra S, et al. ENPP1 affects insulin action and secretion: evidences from in vitro studies. PLoS ONE. (2011) 6:e19462. doi: 10.1371/journal.pone.0019462
75. Locke JM, Xavier GDS, Rutter GA, Harries LW. An alternative polyadenylation signal in TCF7L2 generates isoforms that inhibit T cell factor / lymphoid-enhancer factor (TCF/LEF) -dependent target genes. Diabetologia. (2011). 2:3078–82. doi: 10.1007/s00125-011-2290-6
76. Bugliani M, Liechti R, Cheon H, Suleiman M, Marselli L, Kirkpatrick C, et al. Microarray analysis of isolated human islet transcriptome in type 2 diabetes and the role of the ubiquitin-proteasome system in pancreatic beta cell dysfunction. Mol Cell Endocrinol. (2013) 367:1–10. doi: 10.1016/j.mce.2012.12.001
77. Pasquali L, Gaulton KJ, Rodríguez-seguí SA, Gómez-marín C, Bunt Van D M, Ponsa-cobas J, et al. Pancreatic islet enhancer clusters enriched in type 2 diabetes risk – associated variants. Nat Genet. (2014). 46:136–43. doi: 10.1038/ng.2870
78. Poveda A, Koivula RW, Ahmad S, Barroso I, Hallmans G, Johansson I, et al. Innate biology versus lifestyle behaviour in the aetiology of obesity and type 2 diabetes: the GLACIER Study. Diabetologia. (2016) 59:462–71. doi: 10.1007/s00125-015-3818-y
79. Ahmad S, Rukh G, Varga TV, Ali A, Kurbasic A, Shungin D, et al. Gene × physical activity interactions in obesity: combined analysis of 111,421 individuals of European Ancestry. PLoS Genet. (2013) 9:1–9. doi: 10.1371/journal.pgen.1003607
80. Wood AR, Jonsson A, Jackson AU, Wang N, Van Leewen N, Palmer ND, et al. A genome-wide association study of IVGTT-based measures of first-phase insulin secretion refines the underlying physiology of type 2 diabetes variants. Diabetes. (2017) 66:2296–309. doi: 10.2337/db16-1452
81. Franks PW, McCarthy MI. Exposing the exposures responsible for type 2 diabetes and obesity. Science. (2016) 354:69–73. doi: 10.1126/science.aaf5094
82. Gudmundsdottir V, Pedersen HK, Allebrandt KV, Brorsson C, van Leeuwen N, Banasik K, et al. Integrative network analysis highlights biological processes underlying GLP-1 stimulated insulin secretion: a DIRECT study. PLoS ONE. (2018) 13:1–19. doi: 10.1371/journal.pone.0189886
83. Payne F, Lim K, Girousse A, Brown RJ, Kory N, Robbins A, et al. Mutations disrupting the Kennedy phosphatidylcholine pathway in humans with congenital lipodystrophy and fatty liver disease. Proc Natl Acad Sci. (2014) 111:8901–6. doi: 10.1073/pnas.1408523111
84. Payne F, Colnaghi R, Rocha N, Seth A, Harris J, Carpenter G, et al. Hypomorphism in human NSMCE2 linked to primordial dwarfism and insulin resistance. J Clin Invest. (2014) 124:4028–38. doi: 10.1172/JCI73264
85. Cederberg H, Stančáková A, Kuusisto J, Laakso M, Smith U. Family history of type 2 diabetes increases the risk of both obesity and its complications: is type 2 diabetes a disease of inappropriate lipid storage? J Intern Med. (2015) 277:540–51. doi: 10.1111/joim.12289
86. Lotta LA, Scott RA, Sharp SJ, Burgess S, Luan J, Tillin T, et al. Genetic predisposition to an impaired metabolism of the branched-chain amino acids and risk of type 2 diabetes: a mendelian randomisation analysis. PLoS Med. (2016) 13:1–22. doi: 10.1371/journal.pmed.1002179
87. Hogling DE, Petrus P, Gao H, Bäckdahl J, Dahlman I, Laurencikiene J, et al. Adipose and circulating CCL18 levels associate with metabolic risk factors in women. J Clin Endocrinol Metab. (2016) 101:4021–9. doi: 10.1210/jc.2016-2390
88. Huang-Doran I, Tomlinson P, Payne F, Gast A, Sleigh A, Bottomley W, et al. Insulin resistance uncoupled from dyslipidemia due to C-terminal PIK3R1 mutations. JCI Insight. (2016) 1:1–15. doi: 10.1172/jci.insight.88766
89. Yew Tan C, Virtue S, Murfitt S, Robert LD, Phua YH, Dale M, et al. Adipose tissue fatty acid chain length and mono-unsaturation increases with obesity and insulin resistance. Sci Rep. (2015) 5:1–10. doi: 10.1038/srep18366
90. Balboa D, Saarimäki-Vire J, Borshagovski D, Survila M, Lindholm P, Galli E, et al. Insulin mutations impair beta-cell development in a patient-derived iPSC model of neonatal diabetes. Elife. (2018) 7:1–35. doi: 10.7554/eLife.38519
91. Grieco FA, Sebastiani G, Juan-Mateu J, Villate O, Marroqui L, Ladrière L, et al. MicroRNAs miR-23a-3p, miR-23b-3p, and miR-149-5p regulate the expression of proapoptotic bh3-only proteins DP5 and PUMA in human pancreatic β-cells. Diabetes. (2017) 66:100–12. doi: 10.2337/db16-0592
92. Reinauer C, Rosenbauer J, Bächle C, Herder C, Roden M, Ellard S, et al. The clinical course of patients with preschool manifestation of type 1 diabetes is independent of the HLA DR-DQ genotype. Genes (Basel). (2017) 8:2–17. doi: 10.3390/genes8050146
93. Dos Santos RS, Marroqui L, Velayos T, Olazagoitia-Garmendia A, Jauregi-Miguel A, Castellanos-Rubio A, et al. DEXI, a candidate gene for type 1 diabetes, modulates rat and human pancreatic beta cell inflammation via regulation of the type I IFN/STAT signalling pathway. Diabetologia. (2019) 62:459–72. doi: 10.1007/s00125-018-4782-0
94. Cosentino C, Toivonen S, Villamil ED, Atta M, Ravanat JL, Demine S, et al. Pancreatic -cell tRNA hypomethylation and fragmentation link TRMT10A deficiency with diabetes. Nucleic Acids Res. (2018) 46:10302–18. doi: 10.1093/nar/gky839
95. Slieker RC, van der Heijden AAWA, van Leeuwen N, Mei H, Nijpels G, Beulens JW, et al. HbA1c is associated with altered expression in blood of cell cycle- and immune response-related genes. Diabetologia. (2018) 61:138–46. doi: 10.1007/s00125-017-4467-0
96. Zhou K, Donnelly L, Yang J, Li M, Deshmukh H, Zuydam Van N, et al. Heritability of variation in glycaemic response to metformin: a genome-wide complex trait analysis. Lancet Diabetes Endocrinol. (2014) 2:481–7. doi: 10.1016/S2213-8587(14)70050-6
97. Rocca B, Dragani A, Pagliaccia F. Identifying determinants of variability to tailor aspirin therapy. Expert Rev Cardiovasc Ther. (2013) 11:365–79. doi: 10.1586/erc.12.144
98. Rocca B, Santilli F, Pitocco D, Mucci L, Petrucci G, Vitacolonna E, et al. The recovery of platelet cyclooxygenase activity explains interindividual variability in responsiveness to low-dose aspirin in patients with and without diabetes. J Thromb Haemost. (2012) 10:1220–30. doi: 10.1111/j.1538-7836.2012.04723.x
99. Franks PW, Pearson E, Florez JC. Gene-environment and gene-treatment interactions in type 2 diabetes: Progress, pitfalls, and prospects. Diabetes Care. (2013) 36:1413–21. doi: 10.2337/dc12-2211
100. Pearson ER. Personalized medicine in diabetes: the role of “omics” and biomarkers. Diabet Med. (2016) 33:712–7. doi: 10.1111/dme.13075
101. Rouskas K, Cauchi S, Raverdy V, Yengo L, Froguel P, Pattou F. Weight loss independent association of TCF7 L2 gene polymorphism with fasting blood glucose after Roux-en-Y gastric bypass in type 2 diabetic patients. Surg Obes Relat Dis. (2014) 10:679–83. doi: 10.1016/j.soard.2013.12.016
102. Apperloo EM, Pena MJ, Zeeuw D, Denig P, Heerspink HJL. Individual variability in response to renin angiotensin aldosterone system inhibition predicts cardiovascular outcome in patients with type 2 diabetes: a primary care cohort study. Diabetes Obes Metab. (2018) 20:1377–83. doi: 10.1111/dom.13226
103. Idzerda NMA, Pena MJ, Heerspink HJL. Personalized medicine in diabetic kidney disease: a novel approach to improve trial design and patient outcomes. Curr Opin Nephrol Hypertens. (2018) 27:426–32. doi: 10.1097/MNH.0000000000000447
104. Franks PW, Atabaki-Pasdar N. Causal inference in obesity research. J Intern Med. (2017) 281:222–32. doi: 10.1111/joim.12577
105. Lotta LA, Abbasi A, Sharp SJ, Sahlqvist AS, Waterworth D, Brosnan JM, et al. Definitions of metabolic health and risk of future type 2 diabetes in body mass index categories : a systematic review and network. Diabetes Care. (2016). 38:2177–87. doi: 10.2337/dc15-1218
106. Sattar N, Gill JMR. Type 2 diabetes in migrant south Asians: mechanisms, mitigation, and management. Lancet Diabetes Endocrinol. (2015) 3:1004–16. doi: 10.1016/S2213-8587(15)00326-5
107. Schwandt A, Hermann JM, Rosenbauer J, Boettcher C, Dunstheimer D, Grulich-Henn J, et al. Longitudinal trajectories of metaboliccontrol from childhood to young adulthood in type 1 diabetes from a large German/ austrian registry: a group-based modeling approach. Diabetes Care. (2017) 40:309–16. doi: 10.2337/dc16-1625
108. Ahlqvist E, Storm P, Käräjämäki A, Martinell M, Dorkhan M, Carlsson A, et al. Novel subgroups of adult-onset diabetes and their association with outcomes: a data-driven cluster analysis of six variables. Lancet Diabetes Endocrinol. (2018) 6:361–9. doi: 10.1016/S2213-8587(18)30051-2
109. Magnan C, Thorens B, Campana M, Lenoir V, Prip-Buus C, Hainault I, et al. Protective role of the ELOVL2/docosahexaenoic acid axis in glucolipotoxicity-induced apoptosis in rodent beta cells and human islets. Diabetologia. (2018) 61:1780–93. doi: 10.1007/s00125-018-4629-8
110. Lortz S, Gurgul-Convey E, Naujok O, Lenzen S. Overexpression of the antioxidant enzyme catalase does not interfere with the glucose responsiveness of insulin-secreting INS-1E cells and rat islets. Diabetologia. (2013) 56:774–82. doi: 10.1007/s00125-012-2823-7
111. Klee P, Allagnat F, Pontes H, Cederroth M, Charollais A, Caille D, et al. Connexins protect mouse pancreatic β cells against apoptosis. J Clin Investig. (2011) 121:4870–9. doi: 10.1172/JCI40509
112. Mehmeti I, Gurgul-convey E, Lenzen S, Lortz S. Induction of the intrinsic apoptosis pathway in insulin-secreting cells is dependent on oxidative damage of mitochondria but independent of caspase-12 activation. BBA - Mol Cell Res. (2011) 1813:1827–35. doi: 10.1016/j.bbamcr.2011.06.022
113. Gurgul-convey E, Mehmeti I, Lortz S. Cytokine toxicity in insulin-producing cells is mediated by nitro-oxidative stress-induced hydroxyl radical formation in mitochondria. J Mol Med (Berl). (2011) 89:785–98. doi: 10.1007/s00109-011-0747-1
114. Efrat S. Mechanisms of adult human β-cell in vitro dedifferentiation and redifferentiation. Diabetes Obes Metab. (2016) 18:97–101. doi: 10.1111/dom.12724
115. Soggia A, Ramond C, Akiyama H, Scharfmann R, Duvillie B. von Hippel–Lindau gene disruption in mouse pancreatic progenitors and its consequences on endocrine differentiation in vivo: importance of HIF1-α and VEGF-A upregulation. Diabetologia. (2014) 57:2348–56. doi: 10.1007/s00125-014-3365-y
116. Honoré C, Rescan C, Hald J, McGrath PS, Petersen MBK, Hansson M, et al. Revisiting the immunocytochemical detection of Neurogenin 3 expression in mouse and man. Diabetes Obes Metab. (2016) 18:10–22. doi: 10.1111/dom.12718
117. Ramond C, Glaser N, Berthault C, Ameri J, Kirkegaard JS, Hansson M, et al. Reconstructing human pancreatic differentiation by mapping specific cell populations during development. Elife. (2017) 6:1–22. doi: 10.7554/eLife.27564
118. Hoarau E, Chandra V, Rustin P, Scharfmann R, Duvillie B. Pro-oxidant/antioxidant balance controls pancreatic β-cell differentiation through the ERK1/2 pathway. Cell Death Dis. (2014) 5:e1487–10. doi: 10.1038/cddis.2014.441
119. Rachdi L, Kariyawasam D, Guez F, Aïello V, Arbonés ML, Janel N, et al. Dyrk1a haploinsufficiency induces diabetes in mice through decreased pancreatic beta cell mass. Diabetologia. (2014) 57:960–9. doi: 10.1007/s00125-014-3174-3
120. Vig S, Buitinga M, Rondas D, Crèvecoeur I, van Zandvoort M, Waelkens E, et al. Cytokine-induced translocation of GRP78 to the plasma membrane triggers a pro-apoptotic feedback loop in pancreatic beta cells. Cell Death Dis. (2019) 10:309. doi: 10.1038/s41419-019-1518-0
121. Marroqui L, Santos RS, Op de beeck A, Coomans de Brachène A, Marselli L, Marchetti P, et al. Interferon-α mediates human beta cell HLA class I overexpression, endoplasmic reticulum stress and apoptosis, three hallmarks of early human type 1 diabetes. Diabetologia. (2017) 60:656–67. doi: 10.1007/s00125-016-4201-3
122. Bugliani M, Syed F, Paula FMM, Omar BA, Suleiman M, Mossuto S, et al. DPP-4 is expressed in human pancreatic beta cells and its direct inhibition improves beta cell function and survival in type 2 diabetes. Mol Cell Endocrinol. (2018) 473:186–93. doi: 10.1016/j.mce.2018.01.019
123. Yeo L, Lorenc A, Eichmann M, Pujol-Autonell I, Skowera A, Peakman M, et al. Autoreactive T effector memory differentiation mirrors β cell function in type 1 diabetes. J Clin Invest. (2018) 128:3460–74. doi: 10.1172/JCI120555
124. Tesovnik T, Kovac J, Hovnik T, Dovc K, Bratina N, Battelino T, et al. Association of glycemic control and cell stress with telomere attrition in type 1 diabetes. JAMA Pediatr. (2018) 172:879–81. doi: 10.1001/jamapediatrics.2018.1175
125. Prinz N, Schwandt A, Becker M, Denzer C, Flury M, Fritsch M, et al. Trajectories of body mass index from childhood to young adulthood among patients with type 1 diabetes—a longitudinal group-based modeling approach based on the DPV registry. J Pediatr. (2018) 201:78–85.e4. doi: 10.1016/j.jpeds.2018.05.014
126. Zhou Y, Llauradó G, Orešič M, Hyötyläinen T, Orho-Melander M, Yki-Järvinen H. Circulating triacylglycerol signatures and insulin sensitivity in NAFLD associated with the E167K variant in TM6SF2. J Hepatol. (2015) 62:657–63. doi: 10.1016/j.jhep.2014.10.010
127. Eriksson-Hogling D, Andersson DP, Bäckdahl J, Hoffstedt J, Rössner S, Thorell A, et al. Adipose tissue morphology predicts improved insulin sensitivity following moderate or pronounced weight loss. Int J Obes. (2015) 39:893–8. doi: 10.1038/ijo.2015.18
128. Dahlman I, Sinha I, Gao H, Brodin D, Thorell A, Rydén M, et al. The fat cell epigenetic signature in post-obese women is characterized by global hypomethylation and differential DNA methylation of adipogenesis genes. Int J Obes. (2015) 39:910–9. doi: 10.1038/ijo.2015.31
129. Sattar N, Gill JMR. Type 2 diabetes as a disease of ectopic fat? BMC Med. (2014) 12:1–6. doi: 10.1186/s12916-014-0123-4
130. Eliasson B, Rawshani A, Axelsen M, Hammarstedt A, Smith U. Cephalic phase of insulin secretion in response to a meal is unrelated to family history of type 2 diabetes. PLoS ONE. (2017) 12:1–7. doi: 10.1371/journal.pone.0173654
131. Toledo PL, Torkko JM, Müller A, Wegbrod C, Sönmez A, Solimena M, et al. ICA512 RESP18 homology domain is a protein-condensing factor and insulin fibrillation inhibitor. J Biol Chem. (2019) 294:8564–76. doi: 10.1074/jbc.RA119.007607
132. Silvola JMU, Saraste A, Laitinen I, Savisto N, Laine VJO, Heinonen SE, et al. Effects of age, diet, and type 2 diabetes on the development and FDG uptake of atherosclerotic plaques. JACC Cardiovasc Imaging. (2011) 4:1294–301. doi: 10.1016/j.jcmg.2011.07.009
133. Bohn B, Mönkemöller K, Hilgard D, Dost A, Schwab KO, Lilienthal E, et al. Oral contraception in adolescents with type 1 diabetes and its association with cardiovascular risk factors. A multicenter DPV study on 24,011 patients from Germany, Austria or Luxembourg. Pediatr Diabetes. (2018) 19:937–44. doi: 10.1111/pedi.12656
134. Wanner N, Vornweg J, Combes A, Wilson S, Plappert J, Rafflenbeul G, et al. DNA methyltransferase 1 controls nephron progenitor cell renewal and differentiation. J Am Soc Nephrol. (2019) 30:63–78. doi: 10.1681/ASN.2018070736
135. Rinschen MM, Hoppe A-K, Kohli P, Hagmann H, Bertsch S, Höhne M, et al. YAP-mediated mechanotransduction determines the podocyte's response to damage. Sci Signal. (2017) 10:1–16. doi: 10.1126/scisignal.aaf8165
136. Hurcombe JA, Hartley P, Lay AC, Ni L, Bedford JJ, Leader JP, et al. Podocyte GSK3 is an evolutionarily conserved critical regulator of kidney function. Nat Commun. (2019) 10:403. doi: 10.1038/s41467-018-08235-1
137. Schell C, Rogg M, Suhm M, Helmstädter M, Sellung D, Yasuda-Yamahara M, et al. The FERM protein EPB41L5 regulates actomyosin contractility and focal adhesion formation to maintain the kidney filtration barrier. Proc Natl Acad Sci. (2017) 114:E4621–30. doi: 10.1073/pnas.1617004114
138. Lay AC, Hurcombe JA, Betin VMS, Barrington F, Rollason R, Ni L, et al. Prolonged exposure of mouse and human podocytes to insulin induces insulin resistance through lysosomal and proteasomal degradation of the insulin receptor. Diabetologia. (2017) 60:2299–311. doi: 10.1007/s00125-017-4394-0
139. Nead KT, Sharp SJ, Thompson DJ, Painter JN, Savage DB, Semple RK, et al. Evidence of a causal association between insulinemia and endometrial cancer: a mendelian randomization analysis. J Natl Cancer Inst. (2015) 107:1–7. doi: 10.1093/jnci/djv178
140. Lovestone S, Smith U. Advanced glycation end products, dementia, and diabetes. Proc Natl Acad Sci. (2014) 111:4743–4. doi: 10.1073/pnas.1402277111
141. Katrina Loomis A, Kabadi S, Preiss D, Hyde C, Bonato V, Louis MS, et al. Body mass index and risk of nonalcoholic fatty liver disease: two electronic health record prospective studies. J Clin Endocrinol Metab. (2016) 101:945–52. doi: 10.1210/jc.2015-3444
142. Lallukka S, Yki-Järvinen H. Non-alcoholic fatty liver disease and risk of type 2 diabetes. Best Pract Res Clin Endocrinol Metab. (2016) 30:385–95. doi: 10.1016/j.beem.2016.06.006
143. Franzini M, Musetti V, Guarino D, Caponi L, Paolicchi A, Emdin M, et al. γ-glutamyltransferase fractions in obese subjects with type 2 diabetes: relation to insulin sensitivity and effects of bariatric surgery. Obes Surg. (2018) 28:1363–71. doi: 10.1007/s11695-017-3017-7
144. StemBANCC. IMI1 Final Project Report Public Summary - Stem cells for Biological Assays of Novel drugs and prediCtive toxiCology (StemBANCC). StemBANCC (2018).
145. Avior Y, Sagi I, Benvenisty N. Pluripotent stem cells in disease modelling and drug discovery. Nat Rev Mol Cell Biol. (2016) 17:170–82. doi: 10.1038/nrm.2015.27
146. EBiSC. The European Bank for induced Pluripotent Stem Cells Operated through The European Collection of Authenticated Cell Cultures. EBiSC (2017).
147. Potter J. Executive Summary of the Final Project Report - European Bank for Induced Pluripotent Stem Cells (EBISC). EBiSC (2018).
148. Thurner M, Shenhav L, Wesolowska-Andersen A, Bennett AJ, Barrett A, Gloyn AL, et al. Genes associated with pancreas development and function maintain open chromatin in iPSCs generated from human pancreatic beta cells. Stem Cell Rep. (2017) 9:1395–405. doi: 10.1016/j.stemcr.2017.09.020
149. Khamis A, Canouil M, Siddiq A, Crouch H, Falchi M, Bulow von M, et al. Laser capture microdissection of human pancreatic islets reveals novel eQTLs associated with type 2 diabetes. Mol Metab. (2019) 24:98–107. doi: 10.1016/j.molmet.2019.03.004
150. Bunt M, Lako M, Barrett A, Gloyn AL, Hansson M, McCarthy MI, et al. Insights into islet development and biology through characterization of a human iPSC-derived endocrine pancreas model. Islets. (2016) 8:83–95. doi: 10.1080/19382014.2016.1182276
151. Martinez-Sanchez A, Nguyen-Tu MS, Leclerc I, Rutter GA. Manipulation and measurement of AMPK activity in pancreatic islets. Methods Mol Biol. (2018) 1732:413–31. doi: 10.1007/978-1-4939-7598-3_26
152. Heinonen SE, Genové G, Bengtsson E, Hübschle T, Åkesson L, Hiss K, et al. Animal models of diabetic macrovascular complications: Key players in the development of new therapeutic approaches. J Diabetes Res. (2015) 2015:404085. doi: 10.1155/2015/404085
153. Engelbertsen D, To F, Dunér P, Kotova O, Söderberg I, Alm R, et al. Increased inflammation in atherosclerotic lesions of diabetic akita-LDLr -/- mice compared to nondiabetic LDLr -/- mice. Exp Diabetes Res. (2012) 2012:176162. doi: 10.1155/2012/176162
154. Helmstädter M, Huber TB, Hermle T. Using the drosophila nephrocyte to model podocyte function and disease. Front Pediatr. (2017) 5:262. doi: 10.3389/fped.2017.00262
155. Mulder S, Hamidi H, Kretzler M, Ju W. An integrative systems biology approach for precision medicine in diabetic kidney disease. Diabetes Obes Metab. (2018) 20:6–13. doi: 10.1111/dom.13416
156. Atabaki-Pasdar N, Ohlsson M, Shungin D, Kurbasic A, Ingelsson E, Pearson ER, et al. Statistical power considerations in genotype-based recall randomized controlled trials. Sci Rep. (2016) 6:37307. doi: 10.1038/srep37307
157. Zeeuw D, Heerspink HJL, Jardine M, Perkovic V. Renal trials in diabetes need a platform : time for a global approach? LANCET Diabetes Endocrinol. (2017) 8587:2–4. doi: 10.1016/S2213-8587(17)30263-2
158. Heerspink HJL, List J, Perkovic V. New clinical trial designs for establishing drug efficacy and safety in a precision medicine era. Diabetes Obes Metab. (2018) 20:14–8. doi: 10.1111/dom.13417
159. Koivula RW, Heggie A, Barnett A, Cederberg H, Hansen TH, Koopman AD, et al. Discovery of biomarkers for glycaemic deterioration before and after the onset of type 2 diabetes: rationale and design of the epidemiological studies within the IMI DIRECT Consortium. Diabetologia. (2014) 57:1132–42. doi: 10.1007/s00125-014-3216-x
160. Rauh SP, Heymans MW, Koopman ADM, Nijpels G, Stehouwer CD, Thorand B, et al. Predicting glycated hemoglobin levels in the non-diabetic general population: Development and validation of the DIRECT-DETECT prediction model-a DIRECT study. PLoS ONE. (2017) 12:1–13. doi: 10.1371/journal.pone.0171816
161. Zetterqvist AV, Berglund LM, Blanco F, Garcia-vaz E, Wigren M, Dune P, et al. Inhibition of Nuclear Factor of Activated T-Cells (NFAT) suppresses accelerated atherosclerosis in diabetic mice. PLoS ONE. (2013) 8:e65020. doi: 10.1371/journal.pone.0065020
162. Perco P, Pena M, Heerspink HJL, Mayer G. Multimarker panels in diabetic kidney disease: the way to improved clinical trial design and clinical practice? Kidney Int Rep. (2019) 4:212–21. doi: 10.1016/j.ekir.2018.12.001
163. Zschiedrich S, Bork T, Liang W, Wanner N, Eulenbruch K, Munder S, et al. Targeting mTOR signaling can prevent the progression of FSGS. J Am Soc Nephrol. (2017) 28:2144–57. doi: 10.1681/ASN.2016050519
164. Garner KL, Betin VMS, Pinto V, Graham M, Abgueguen E, Barnes M, et al. Enhanced insulin receptor, but not PI3K, signalling protects podocytes from ER stress. Sci Rep. (2018) 8:1–13. doi: 10.1038/s41598-018-22233-9
165. Montastier E, Déjean S, Le Gall C, Saris WHM, Langin D, Viguerie N. Adipose tissue CIDEA is associated, independently of weight variation, to change in insulin resistance during a longitudinal weight control dietary program in obese individuals. PLoS ONE. (2014) 9:e98707. doi: 10.1371/journal.pone.0098707
166. Baltrusch S, Schmitt H, Brix A, Langer S, Lenzen S. Additive activation of glucokinase by the bifunctional enzyme 6-phosphofructo-2-kinase/fructose-2,6-bisphosphatase and the chemical activator LY2121260. Biochem Pharmacol. (2012) 83:1300–6. doi: 10.1016/j.bcp.2012.01.020
167. Thorel F, Damond N, Chera S, Wiederkehr A, Thorens B, Meda P, et al. Normal glucagon signaling and β-cell function after near-total α-cell ablation in adult mice. Diabetes. (2011) 60:2872–82. doi: 10.2337/db11-0876
168. Friedman-Mazursky O, Elkon R, Efrat S. Redifferentiation of expanded human islet β cells by inhibition of ARX. Sci Rep. (2016) 6:1–11. doi: 10.1038/srep20698
169. Rachdi L, Kariawasam D, Aiëllo V, Herault Y, Janel N, Delabar JM, et al. Dyrk1A induces pancreatic β cell mass expansion and improves glucose tolerance. Cell Cycle. (2014) 13:2222–30. doi: 10.4161/cc.29250
170. Oshima M, Knoch K-P, Diedisheim M, Petzold A, Cattan P, Bugliani M, et al. Virus-like infection induces human β cell dedifferentiation. JCI Insight. (2018) 3:1–17. doi: 10.1172/jci.insight.97732
171. Hakonen E, Chandra V, Fogarty CL, Yu NYL, Ustinov J, Katayama S, et al. MANF protects human pancreatic beta cells against stress-induced cell death. Diabetologia. (2018) 61:2202–14. doi: 10.1007/s00125-018-4687-y
172. Buitinga M, Jansen T, van der Kroon I, Woliner-van der Weg W, Boss M, Janssen M, et al. Succinylated gelatin improves the theranostic potential of radiolabeled exendin-4 in insulinoma patients. J Nucl Med. (2019) 60:812–6. doi: 10.2967/jnumed.118.219980
173. Cosentino C, Cnop M, Igoillo-Esteve M. The tRNA epitranscriptome and diabetes: emergence of tRNA hypomodifications as a cause of pancreatic β-cell failure. Endocrinology. (2019) 160:1262–74. doi: 10.1210/en.2019-00098
174. Nathan G, Kredo-Russo S, Geiger T, Lenz A, Kaspi H, Hornstein E, et al. MiR-375 promotes redifferentiation of adult human β cells expanded in vitro. PLoS ONE. (2015) 10:1–18. doi: 10.1371/journal.pone.0122108
175. Bénardeau A, Verry P, Atzpodien EA, Funk JM, Meyer M, Mizrahi J, et al. Effects of the dual PPAR-α/γ agonist aleglitazar on glycaemic control and organ protection in the Zucker diabetic fatty rat. Diabetes Obes Metab. (2013) 15:164–74. doi: 10.1111/dom.12006
176. Gonçalves I, Edsfeldt A, Colhoun HM, Shore AC, Palombo C, Natali A, et al. Association between renin and atherosclerotic burden in subjects with and without type 2 diabetes. BMC Cardiovasc Disord. (2016) 16:1–10. doi: 10.1186/s12872-016-0346-8
177. Patrono C. Low-dose aspirin in primary prevention: cardioprotection, chemoprevention, both, or neither? Eur Heart J. (2013) 34:3403–11. doi: 10.1093/eurheartj/eht058
178. Preiss D, Dawed A, Welsh P, Heggie A, Jones AG, Dekker J, et al. Sustained influence of metformin therapy on circulating glucagon-like peptide-1 levels in individuals with and without type 2 diabetes. Diabetes Obes Metab. (2017) 19:356–63. doi: 10.1111/dom.12826
179. Breyer MD, Kretzler M. Novel avenues for drug discovery in diabetic kidney disease. Expert Opin Drug Discov. (2018) 13:65–74. doi: 10.1080/17460441.2018.1398731
180. Heerspink HJL, Perco P, Mulder S, Leierer J, Hansen MK, Heinzel A, et al. Canagliflozin reduces inflammation and fibrosis biomarkers: a potential mechanism of action for beneficial effects of SGLT2 inhibitors in diabetic kidney disease. Diabetologia. (2019) 62:1154–66. doi: 10.1007/s00125-019-4859-4
181. Davies JA, Chang C-H, Lawrence ML, Mills CG, Mullins JJ. Engineered kidneys: principles, progress, and prospects. Adv Regen Biol. (2014) 1:24990. doi: 10.3402/arb.v1.24990
182. Davies JA, Chang CH. Engineering kidneys from simple cell suspensions: an exercise in self-organization. Pediatr Nephrol. (2014) 29:519–24. doi: 10.1007/s00467-013-2579-4
183. Davies J. Engineered renal tissue as a potential platform for pharmacokinetic and nephrotoxicity testing. Drug Discov Today. (2014) 19:725–9. doi: 10.1016/j.drudis.2013.10.023
184. Massai D, Bolesani E, Diaz DR, Kropp C, Kempf H, Halloin C, et al. Sensitivity of human pluripotent stem cells to insulin precipitation induced by peristaltic pump-based medium circulation: Considerations on process development. Sci Rep. (2017) 7:1–15. doi: 10.1038/s41598-017-04158-x
Keywords: innovative medicines initiative, diabetes, complications of diabetes, personalized medicine, type 2 diabetes, type 1 diabetes
Citation: Brito MdF, Torre C and Silva-Lima B (2021) Scientific Advances in Diabetes: The Impact of the Innovative Medicines Initiative. Front. Med. 8:688438. doi: 10.3389/fmed.2021.688438
Received: 30 March 2021; Accepted: 02 June 2021;
Published: 06 July 2021.
Edited by:
Hilde Stevens, Université libre de Bruxelles, BelgiumReviewed by:
Frits Lekkerkerker, Consultant, Amsterdam, NetherlandsCopyright © 2021 Brito, Torre and Silva-Lima. This is an open-access article distributed under the terms of the Creative Commons Attribution License (CC BY). The use, distribution or reproduction in other forums is permitted, provided the original author(s) and the copyright owner(s) are credited and that the original publication in this journal is cited, in accordance with accepted academic practice. No use, distribution or reproduction is permitted which does not comply with these terms.
*Correspondence: Maria de Fátima Brito, bWFyaWEuZmF0aW1hLmJyaXRvQGNhbXB1cy51bC5wdA==
Disclaimer: All claims expressed in this article are solely those of the authors and do not necessarily represent those of their affiliated organizations, or those of the publisher, the editors and the reviewers. Any product that may be evaluated in this article or claim that may be made by its manufacturer is not guaranteed or endorsed by the publisher.
Research integrity at Frontiers
Learn more about the work of our research integrity team to safeguard the quality of each article we publish.