- 1Key Laboratory of Kidney Diseases of Hebei Province, Department of Pathology, Hebei Medical University, Shijiazhuang, China
- 2Department of Burn, The Second Hospital of Hebei Medical University, Shijiazhuang, China
- 3Department of Nephrology, The Second Hospital of Hebei Medical University, Shijiazhuang, China
Years of research revealed that crosstalk extensively existed among kidney cells, cell factors and metabolites and played an important role in the development of diabetic kidney disease (DKD). In the last few years, single-cell RNA sequencing (scRNA-seq) technology provided new insight into cellular heterogeneity and genetic susceptibility regarding DKD at cell-specific level. The studies based on scRNA-seq enable a much deeper understanding of cell-specific processes such as interaction between cells. In this paper, we aim to review recent progress in single cell transcriptomic analyses of DKD, particularly highlighting on intra- or extra-glomerular cell crosstalk, cellular targets and potential therapeutic strategies for DKD.
Introduction
Diabetic Kidney Disease (DKD) is a microvascular complication associated with type I or type II diabetes. It has become a public issue and seriously threaten human health and lives. As the leading single cause of end-stage renal disease (ESRD) in many countries, such as the United States, DKD accounts for more than half of all patients enrolled in renal replacement therapy (RRT) programs (1). Although there has been a decline in the incidence of DKD over the past 30 years due to improved diabetes managements, the absolute risk of renal and cardiovascular morbidity and mortality remains overwhelmingly high (2–6). A deeper insight into the pathogenesis of DKD is required for innovative treatment strategies to prevent, arrest, and reverse DKD. Hyperglycemia is thought to be a major factor for diabetic complications and causes accumulation of toxic glucose derivatives (7, 8). However, hyperglycemia alone is not sufficient to the development of DKD since about only 30% of patients with type 1 diabetes mellitus (DM1) and 40% of patients with type 2 diabetes mellitus (DM2) develop this microvascular complication (1, 9). Family aggregation of DKD shown by independent familial studies in different populations suggests a genetic predisposition to DKD (10, 11). Moreover, patients with DKD are not always present with micro/macro-albuminuria. A large proportion of diabetic patients have declined renal function in absence of substantial proteinuria (12). The DKD heterogeneity suggested by the aforementioned evidences implies variant modulation of kidney function in diabetes and highlights the need for better biomarkers to predict the progressive kidney failure in the patients without heavy proteinuria.
Kidney is a highly complex organ consisting of about a million nephrons in humans which is composed of more than 40 different cell types (13, 14). The need for better understanding of the complex cell-to-cell interaction within or even beyond the heterogeneous kidney milieu comes naturally and rationally to reveal the complex mechanism underlying kidney organization, function and disease. The current clinical diagnoses for renal diseases as well as experimental researches on kidney depend largely on morphological cell identification and their known biomarkers. However, some important disease discriminative and prognostic features may not be effectively captured due to the highly operator-dependent microscopical observation and the limited biomarkers available. A single cell transcriptional profiling by a new set of technologies–single cell RNA-sequencing (scRNA-seq) has emerged in the last 10 years as a powerful approach helping to decipher complex information in cells and organs (15, 16). Here we aim to review cell-to-cell cross talk in DKD, particularly highlighting the latest insight gained by scRNA-seq researches.
Brife Introduction of Single Cell RNA Sequencing
scRNA-seq is a new set of technologies for genome wide RNA profiling of individual cells based on whole-genome-amplification (WGA) methods and next-generation sequencing (NGS) technologies (17–20). Before the invention of scRNA-seq, the genome-wide transcriptomic information primarily came from “bulk” RNA-seq, whose data represent an average of gene expression across individual cells and thus may mask some transcriptional information from less representative subpopulation. Compared with bulk RNA-seq, scRNA-seq provides more unbiased gene expression profiles at a single-cell resolution. The scRNA-seq methods have gained considerable progress over the last decade while the single cell DNA sequencing (scDNA-seq) has proven to be more challenging than RNA due to the fact that a single cell contains only two copies of each DNA molecule, but thousands of copies of most RNA molecules, which result in more technical error in scDNA-seq (15). All scRNA-seq techniques include several common steps: single cell isolation, cell lysis and RNA capture, reverse transcription and transcriptome amplification, cDNA library preparation, and sequencing and quantification. The most challenge for scRNA-seq is cell isolation and individualized RNA capture (individual transcriptome barcoding). Two barcoding strategies are suggested, either (1) the addition of a cell-specific barcode to each transcriptome following cell isolation (Microfluidics-based scRNA-seq), or alternatively (2) the addition of a unique index combination to each cell transcriptome without physical partitioning (e.g., Split-seq) (21–25) (Figure 1). Bio information from sequencing is intensively analyzed by computer and the final result is generation of a digital expression matrix including all detected gene expression in each individual cell. High throughput scRNA-seq data are processed to cluster cells and visualized by dimensionality reduction graph. Cell types are identified by examining known marker gene expression in each cluster and shown by the heatmap. Gene-gene correlation analysis helps to clarify the relationship between two marker genes within a cluster as well as the relationship of two marker genes from different clusters. Dynamic gene expression in single cell can be tracked along pseudotemporal trajectory corresponding to a biological process (e.g., development, differentiation, and disease progression). Key regulators for the dynamic gene expression can also be revealed by regulatory network analysis on transcription factors (26).
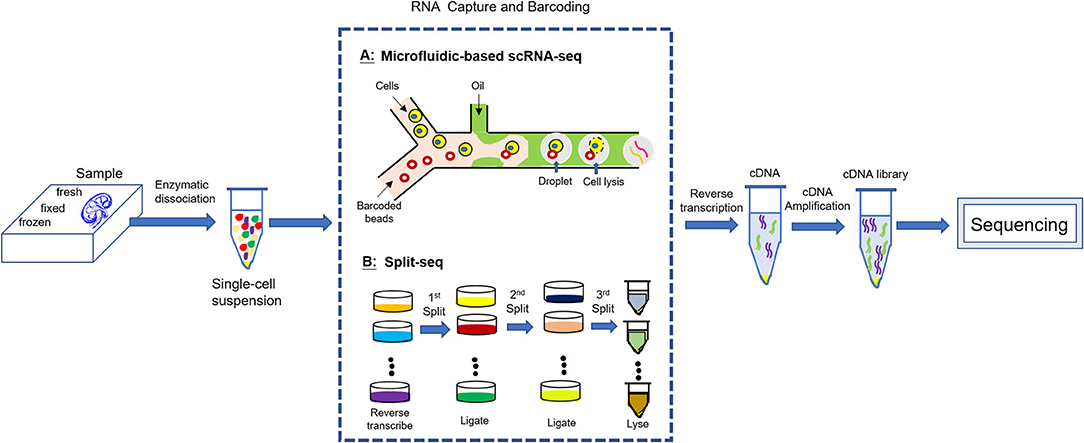
Figure 1. scRNA-seq experimental workflow. All scRNA-seq techniques include several common steps: enzymatic dissociation of the sample into a single cell suspension; individualized RNA capture (individual transcriptome barcoding); reverse transcription and transcriptome amplification; cDNA library preparation; sequencing.
Applications of Single Cell RNA Sequencing on Kidney Diseases
The knowledge regarding the transcriptional landscape of kidney in last 20 years was achieved largely from the “bulk” RNA-seq, which, though highly informative, is limited to describing an average transcriptome across a cell population in a bulk renal tissue or even in finely separated kidney compartments and thus masks or skews signals of interest (26–29). The comprehensive definition of cell types and states cooperating with examination of gene expression in specific cells by scRNA-seq makes it possible for determining specific disease-causal cells and genes. Park et al. performed scRNA-seq on kidneys from healthy male mice and unexpectedly identified a transitional cell type between intercalated cells (ICs) and principal cells (PCs) in collecting duct (30). They further demonstrated this IC-to-PC transition is mediated by Notch signaling and the shift toward the PC fate is the likely cause of metabolic acidosis in chronic kidney disease (CKD) (30). Recently Liao et al. delineated a transcriptomic map of human kidney cells basing on scRNA-seq analysis of normal human kidney (31). Another single-cell transcriptome profiling performed on human kidney allograft biopsy specimens (32) helped mapping previously defined rejection-associated genes to single cell types and revealed paracrine signaling pathway between infiltrating leukocytes and kidney parenchyma (33). A more recent scRNA-seq performed on purified glomeruli from four common kidney injury models (nephrotoxic serum nephritis, diabetes, doxorubicin toxicity and CD2AP deficiency) generated comprehensive snapshots of the altered genetic landscapes in multiple models (34). This research provided new insights into kidney injuries, such as that mesangial cell may shape the characters of the inflammation and wound healing programs in response to distinct types of injuries; persistent mesangial reaction may drive the chronic decline of kidney function in many disease; Hippo pathway is critical for podocyte repair in kidney injuries (34). In a scRNA-seq research on isolated glomerular cells from experimental diabetic mice, the unique trajectory analysis of scRNA-seq revealed dynamic changes of gene expression in endothelial and mesangial cells in diabetic mice (35). Subramanian et al. in a research regarding kidney organoids presented a comprehensive census of human organoids enabled by scRNA-seq in comparison to human adult and fetal kidneys (36). This census achieved some quantitative insight into organoid reproducibility and the data validated the faithfulness of kidney organoids from four different patient-derived induced pluripotent stem cell (iPSC) lines (AS, N1, N2, and ThF), which serve as surrogates of human kidney tissue for the study of a broad array of kidney diseases. The census data also addressed an issue of organoid quality, suggesting that the elimination of off-target cells may also benefit organoid maturity (36). In renal tumor research field, Young et al. studied Wilms' tumor, clear cell, and papillary renal cell carcinoma in relation to healthy fetal, pediatric, adolescent, and adult kidneys, as well as ureters (37). By analyzing tumor composition with scRNA-seq, they verified the hypothesis that Wilms' tumor cells are aberrant fetal cells and defined cancer-associated normal cells as well as delineated a complex VEGF signaling circuit (38). The power of scRNA-seq is not just to identify or catalog cells. It can help predict treatment outcomes and guide therapy. In a scRNA-seq research carried out by Park group, intratumoral heterogeneity was examined between a pair of primary renal cell carcinoma and its lung metastasis (37). The activation of drug target pathways demonstrated considerable variability between the primary and metastatic sites, as well as among individual cancer cells within each site. Guided by scRNA-seq analysis, a combinatorial regimen co-targeting two mutually exclusive pathways for the metastatic cancer cells gained better treatment efficacy over monotherapy (37).
More recently, Humphreys group (39) and Susztak group (40) both profiled kidney transcriptome and chromatin accessibility with sc/nRNA-seq and single nucleus assay for transposase-accessible chromatin using sequencing (snATAC-seq) respectively in their researches. These two multi-omics researches revealed the powerful potential of joint profiling with scRNA-seq in understanding kidney disease and development.
Challenges to Single Cell RNA Sequencing on Kidney Research
Despite the tremendous development of technology, scRNA-seq research is still facing many challenges. Cell isolation and individualized RNA capture remain to be the most challenges, since enzymatic dissociation protocols usually compromise cell viability and adult kidneys are relatively dense with matrix, thus the quality of single cell suspension does not accurately reflect the transcriptional state of each cell before dissociation (26). The possibility of selective cell loss during tissue dissociation and the transcriptional stress response induced by the proteolytic process as well as RNA degradation lead to bias. This may partly account for the failure in detecting about 25% of single kidney cells in sequencing in the work by Park et al. (30). The failure in detecting podocytes in transplant biopsy might be explained by the similar reason (33). The dissociation protocols need to be optimized responding to different kidney origins, since in some diseases, the injured podocytes are more susceptible to loss during enzymatic digestion whereas mesangial cells are less effectively isolated and captured due to the increased matrix. Cold dissociation was recommended by researchers as digestion on ice avoided stress and achieved more abundant cell types than warm dissociation at 37°C (41). The strategy adopted by Chen et al. and Karaiskos et al. in their experiments may partly correct some dissociative artifacts by dissecting specific portion of kidney tissues (e.g., proximal tubules or glomeruli) (42, 43). Human biopsy from patient is another challenge to scRNA-seq since scRNA-seq requires a relatively large number of cells for the automatic cell separation and capture system.
Recently, single nuclear sequencing (snRNASeq) rise popular as an alternative to scRNA-seq for its obvious advantages in gaining good quality nuclei from snap frozen sample while bypassing the proteolytic process at 37°C (44–46). But there were researches implied T, B, and NK lymphocytes were underrepresented in the single-nucleus libraries (40, 42, 44). When interpreting the results of scRNA-seq, protocol-specific biases must be taken into consideration as cryopreservation of dissociated cells results in a major loss of epithelial cell types while methanol fixation maintains the cellular composition but suffers from ambient RNA leakage (41).
In addition to the crucial step of cell dissociation, a successful scRNA-seq is also challenged through the computational workflow. Depending on the platform of choice, researchers individualize their own procedural steps and choose specific analytic tools for data processing from the step of raw counts normalization to feature selection, dimensionality reduction, and clustering. When inferring cell-cell communication from transcriptomics, most of the researchers built the lists of ligand-receptor pairs from multiple databases and literature curation. Armingol et al. collated publicly available lists into a single ligand-receptor pair repository to facilitate further use and comparison (47). However, integrating multiple sources of data is challenging and requires reconciliation of the different ways ligand–receptor pair confidence was assessed or how orthologs were determined (47). To identify the genes associated with cell communication, the gene expression matrix generated by scRNA-seq is filtered by ligand-receptor pairs and a communication score for each ligand-receptor pair is computed using their gene expression levels [function f(L, R), where L and R are the expression values of the ligand and the receptor, respectively] (47). An aggregated communication score is also computed to estimate the overall state of interaction between respective samples or cells and the final results are visualized as Circos plot, network, Heatmap, etc. (47). There may exist some false positives or negatives in the inferred cell communication due to the data-driven inference process, which can lead to different results depending on the strategy adopted (47). Although some powerful computational tools such as CellChat, CellPhoneDB, NicheNet help to minimize the false discoveries (48–50), the findings derived from scRNA-seq need to be validated by experimental tests including immunohistochemistry, western blot and other functional assessment.
Intraglomerular Crosstalk in DKD
Glomerulus is a highly organized complex with two major compartments, the glomerular capillary tuft and the so-called Bowman's capsule which surrounds the capillary tuft. Podocytes wrap around the glomerular capillary with foot processes, which are connected by slit diaphragms bridging the filtration slits. The intraglomerular mesangial cells reside between capillary loops in close contact with glomerular endothelium. Parietal epithelial cells (PECs) compose the outer layer of the capsule, directly connecting to proximal tubules. The formation and maintenance of the glomerular filtration barrier require the communication within glomerulus including a multidirectional crosstalk between podocytes, mesangial cells and endothelial cells as well as PECs (51–57). The normal glomerular structure is shown in Figure 2 (9).
In DKD, the glomeruli are exposed to various noxious stimuli such as high glucose, fatty acids, uric acid, growth factors, cytokines, and hormones, which dysregulate the communication in glomerulus.
Crosstalk Between Podocyte and Glomerular Endothelial Cell (GEC)
Studies have shown that the severity of DKD is correlated with endothelial dysfunction in T1DM and T2DM (58, 59). The growth and survival of GECs are regulated by paracrine vascular endothelial growth factor alpha signaling (VEGFA-VEGFR) (60, 61). Podocyte VEGFA deletion results in the development of heavy proteinuria, marked glomerulosclerosis and glomerular cell apoptosis (62). Conversely, increased podocyte-derived VEGFA was shown to be deleterious in non-diabetic mice, and the injury was further exacerbated with diabetes induction, resulting in advanced glomerulopathy with massive proteinuria (63). Podocyte-produced angiopoietins, functioning as endothelial cell-protective factors in diabetes, mediate podocyte-to-endothelial crosstalk and are critical for modulating the vascular response after the onset of diabetes (61, 64). Angiopoietin-1 (Angpt1) is expressed by podocytes and mesangial cells and its cognate tyrosine kinase receptor, Tie2/Tek is expressed by the glomerular endothelial cells. Angpt1 and Angpt2 both bind to Tie2 receptor and have classically been viewed as having opposing effects on microvascular development, with Angpt1 stabilizing the vasculature, and Angpt2 antagonizing these effects by binding to the Tie2 receptor in a competitive manner (52). Endothelin-1 (Edn-1)/endothelin receptor type A (EdnRA) axis has been demonstrated to be a causative regulator in promoting endothelial cell dysfunction in human biopsies and experimental model of FSGS, and is responsible for the loss of glomerular endothelial glycocalyx by increased degradation of glycosaminoglycans (65–67). In diabetes, EdnRA in GECs is activated by increased circulating Edn-1 or local Edn-1, resulting in mitochondrial stress and endothelial dysfunction (68, 69).
A similar stressed endothelial-to-podocyte crosstalk via mitochondrial oxidative stress in endothelial cells downstream from Edn-1/EdnRA could also underlie segmental lesions in DKD and highlighted a potential mechanism for the proven renoprotective activities of EdnRA inhibitors (70, 71). Activated protein C (APC) formation, which is regulated by endothelial thrombomodulin, is required for podocyte viability (72). Loss of thrombomodulin-dependent APC formation interrupts crosstalk between the vascular compartment and podocytes, causing glomerular apoptosis and diabetic nephropathy (72). Krüppel-like factor 2 (KLF2) is a shear stress-inducible transcription factor and has endoprotective effects on podocytes. Endothelial cell knockout of KLF2 resulted in reduction of the endothelial glycocalyx and podocyte injury in diabetes (73). Recently, a study showed that endothelial derived exosomes, which are enriched with TGF-β1 mRNA, could mediate epithelial-to-mesenchymal transition (EMT) and induce dysfunction of podocytes in a paracrine manner with activation of canonical Wnt/β-catenin signaling (74).
Crosstalk Between Podocyte and Parietal Epithelial Cell (PECs)
Enlightened by delicate balance between visceral and parietal epithelial cells across Bowman's space, the crosstalk between podocytes and PECs are thought to be as equally important as the tight interaction between endothelial cells and podocytes across the glomerular basement membrane (GBM) (75). Indeed, multiple studies have consistently corroborated that the depleted podocytes can be regenerated via differentiation of the adjacent PECs, which serve as the local progenitors of podocytes to reconstitute the podocyte population upon glomerular injury and podocyte loss (53, 76–83). Injured podocytes secrete heparin-binding epidermal growth factor-like growth factor (HB-EGF), which in turn stimulates and promotes the proliferation of PECs while disturbs their compensatory differentiation toward podocytes (77, 83). Another growth factor, insulin-like growth factor-1(IGF-1) has been proved more critical for promoting the differentiation of PECs into podocytes (83). A 3D multiscale modeling study suggested that promoting PECs differentiation are as equivalently important as amelioration of glomerulus stress for podocyte regeneration (83).
Crosstalk Between Glomerular Mesangial Cell (GMC) and Other Glomerular Cell Types
Mesangial cells are considered to be specialized pericytes due to their spatial intimacy with endothelial cells, thus functioning in stabilizing vasculature, synthesizing components of the basement membrane, and participating in controlling capillary vascular tone (84). The tight link between the fates of mesangial cell and endothelial cell has been well established by demonstrating the importance of platelet-derived growth factor B (PDGF-B) and its receptor PDGFR-β in the interaction of endothelial and mesangial cells (84–87). Recent evidences have revealed a significant role of exosomes as the messenger cargos for intercellular communications within glomerulus in DKD (88). Wu et al. demonstrated exosomes released by high glucose-treated GECs could promote α-smooth muscle actin (α-SMA) expression, proliferation and extracellular matrix protein overproduction in GMCs through the TGF-β1/Smad3 signaling pathway (89). In respect of the crosstalk between podocyte and mesangial cell, several signaling pathways have been suggested to be involved including VEGF, Edn-1, CCR7, and its ligand SLC/CCL21, PDGF, connective tissue growth factor (CTGF), hepatocyte growth factor (HGF) and TGF-β (84, 90). Among these signalings, VEGFA and nitric oxide (NO) are considered to play a pivotal role in driving the development of typical DKD lesions, causing as important effects on GMCs as those on endothelial cells. (91). The diabetic podocyte produces excessive VEGF in the setting of low endothelial NO and stimulates growth and proliferation of mesangial and endothelial cells, leading to increased extracellular matrix accumulation, hyperfiltration, and proteinuria (92). A recent research has shown that an intraglomerular crosstalk between mesangial cells and podocytes can inhibit physiological endoplasmic reticulum stress-associated degradation (ERAD) and suppress the phosphorylation of nephrin in podocytes, which thereby lead to podocyte injury under diabetic conditions (92).
The intraglomerular crosstalks are summarized in Table 1.
Findings Based on scRNA-Seq
By comparing the differential gene expression detected by scRNA-seq between specific cell types with the existing ligand-receptor database (http://fantom.gsc.riken.jp/5/) or the potential paracrine secreted ligand-to-membrane receptor pair list obtained by using Human Protein Atlas (https://www.proteinatlas.org/humanproteome/ secretome) and BIOGRID v3.5.165 (https://thebiogrid.org), the researchers can identify cell-cell crosstalk between glomerular cell types. To date, crosstalk data from scRNA-seq research regarding DKD are quite limited. Fu et al. performed scRNA-seq analysis on isolated glomerular cells from induced diabetic eNOS−/− mice (35). They analyzed a total of 644 cells (326 control and 318 diabetic) with a median of 3,457 genes per cell (3,417 control and 3,509 diabetic). With less cells capture but much greater sequencing depth per cell (five-fold) in a plate-based platform, as the researchers mentioned, compared with the microdroplet-based platform, several ligand-receptor pairs in the glomerular cell were identified, some of which are well established (e.g., podocyte VEGFA-endothelial Flt1 and Kdr) while the others are less well characterized in the glomerular homeostasis (e.g., mesangial Epha3-endothelial Efna1) (35). The ligand-receptor pair analysis in scRNA-seq is unprecedentedly informative to suggest almost all the potential direct cell-to-cell crosstalks. Taking Fu et al.'s research for example, this analysis not only identified the established crosstalks such as VEGFA pair, which can be subjected to cross validation with the existing literatures, but also guide future exploration for those less established interaction, such as podocyte BMP7-mesangial BMPR2 pair and mesangial Angpt4-endothelial Tie1 pair, which are implied by insufficient literature, yet to be validated (35). Unlike the above-mentioned crosstalk of podocyte VEGFA-endothelial Flt1 and Kdr having handful supportive evidences, the literatures in respect of the cellular crosstalk involving bone morphogenetic protein-7 (BMP-7) in DKD are limited. BMP-7 in podocytes was reported possibly having protective effects against renal damage produced by hyperglycemia via the interaction with receptor BMPR2 (93, 94). But its cellular crosstalk in DKD via the interaction with its receptor needs more solid evidences. So does the pair of mesangial Angpt4- endothelial Tie1. Moreover, by comparing the diabetic ligand-receptor pairs with control, the changed crosstalk can be revealed, for example, the pairs of mesangial Angpt4-endothelia receptors were not detected in control, but showed in diabetes, even more prominent than other Angpt pairs (35).
In another scRNA-seq on cryopreserved human diabetic kidney samples, 23,980 single-nucleus transcriptomes were generated from three control and three early diabetic nephropathy samples (91). The researchers examined differentially expressed ligand–receptor pairs in glomerular cell types and found human diabetic mesangial cells had increased expression of CCN1 and SLIT3 (95). CCN1 responding extracellular protein, ITGAV, ITGB3, ITGB5 were expressed by podocytes, which interact with CCN1 and subsequently regulate tissue repair. Another CCN1 responding protein, ITGB3 was expressed by endothelial cells. ROBO2 was expressed by podocytes and endothelial cells, which interacted with SLIT3 to modulate cell migration. Diabetic endothelial cells also expressed increased LTBP1, which regulated targeting of latent TGF-β complexes (95). A scRNA-seq on mouse GMCs revealed GMCs having a high enrichment of genes involved in endothelial activity, supporting the long-existing notion that mesangial cells are specialized pericytes (96). Interestingly, the researchers also found that some mesangial cells express podocyte marker genes (e.g., Wt1) as well as endothelial cell marker genes (Tie2, Flk1, Flt1/ Vegfr1) (96).
When interpreting the data of ligand-receptor pair analysis, bias coming from misrepresented cell population must be taken into consideration. Podocytes, especially the injured podocytes are susceptible to loss during the dissociation process, which leads to limited podocytes detected, therefore results in the podocyte population underrepresented in most of the scRNA-seq research. It is also difficult to clearly identify mesangial cells due to the similarity between mesangial cells and stromal mesenchymal cells. Chung et al. found that several genes which were used in their study to identify mesangial cells are not specific while in some cases are specific to smooth muscle cells (SMCs) (Myh11, Rergl, Pln, and Olfr558) (34). They pointed that the study by Fu et al. was limited by the small number of cells, as the reason they referred were that the authors were unable to distinguish mesangial cells from SMCs/JG cells and neither obtain sufficient numbers of podocytes from diabetic mice for analysis (34).
Intraglomerular crosstalk based on ligand-receptor pair analysis of scRNA-seq are shown in Figure 3.
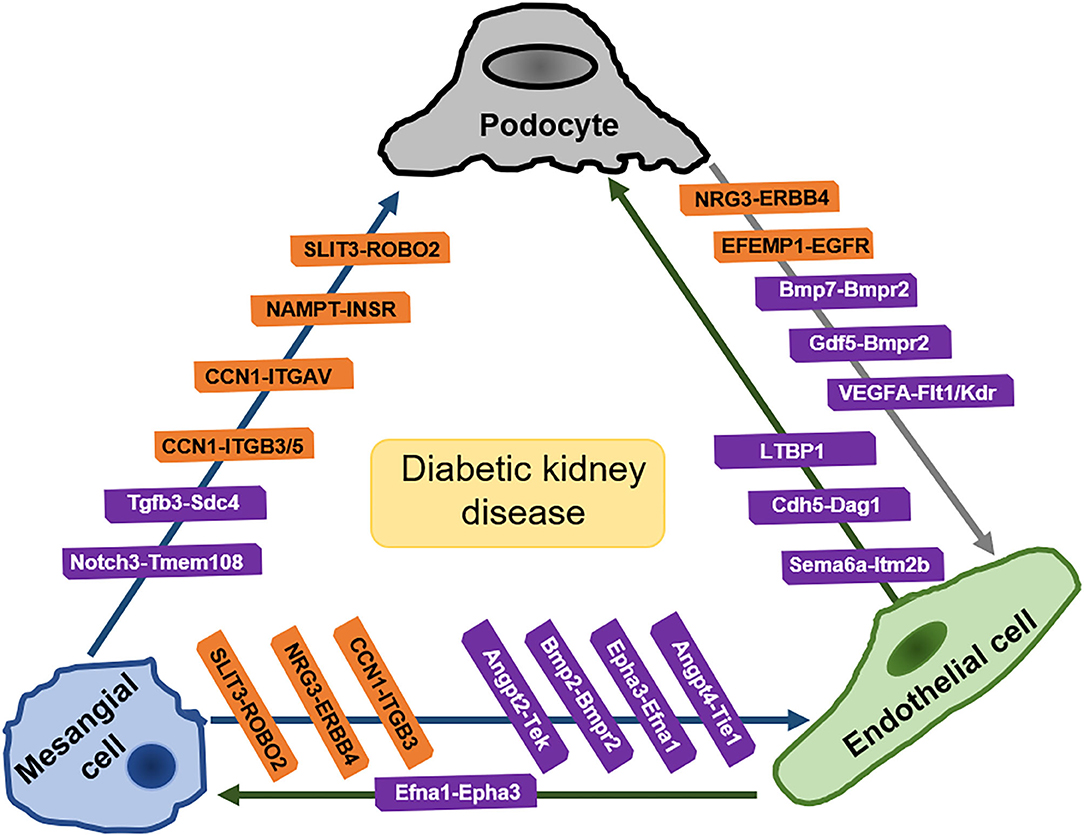
Figure 3. Intraglomerular crosstalk based on ligand-receptor pair analysis of scRNA-seq. Pairs in orange frames come from the analysis of kidney of human with early diabetic nephropathy. Pairs in purple frames come from the analysis of kidney of diabetic mice. SLIT3, slit guidance ligand 3; ROBO2, roundabout guidance receptor 2; NAMPT, Nicotinamide phosphoribosyltransferase; INSR, insulin receptors; CCN1, cellular communication network factor1; ITGB3/5, integrin beta3/5; ITGAV, integrin subunit alpha-V; Tgfb3, Transforming growth factor 3; Sdc4, syndecan 4; Notch3, notch reporter 3; Tmem108, transmembrane protein 108; NRG3, neuregulin-3; ERBB4, Erb-B2 receptor tyrosine kinase 4; Bmp2, bone morphogenetic protein 2; Bmp7, bone morphogenetic protein 7; Bmpr2, bone morphogenetic protein receptor 2; Epha3, erythropoietin-producing hepatocellular carcinoma A3; VEGFA, vascular endothelial growth factor A; Flt1(VEGFR1, vascular endothelial growth factor receptor 1; Kdr (VEGFR2), vascular endothelial growth factor receptor 2; Angpt, angiopoietin; Tie (Tek): angiopoietin 1 receptor; Efnb2, ephrin B2; Efna1, ephrin A1; LTBP1, latent transforming growth factor (TGF)-beta binding protein-1; Cdh5, cadherin 5; Dag1, dystroglycan 1; Sema6a, semaphorin 6a; Itm2b, Integral membrane protein 2B; Cdf5, cycling dof factors 5; EFEMP1, Epidermal Growth Factor-containing Fibulin-like Extracellular Matrix Protein 1; EGFR, Epidermal Growth Factor Receptor. [Pairs in orange are summarized from Mitu et al. (93) and pairs in purple are summarized from Fu et al. (35)].
Extraglomerular Crosstalk in DKD
Tubular-glomerular interplay, which includes two well-known components, glomerular-tubular balance and tubuloglomerular feedback (TGF), has been demonstrated to play important roles in physiological renal function as well as in DKD (97). Proteins leak from glomeruli and arrive at tubular regions, then leading to further tubular injury, which is caused by the accumulation of proteinuria-inducing reactive oxygen species and various cytokines (98). Sirtuin 1 (SIRT1), a nuclear deacetylating enzyme, which mediates deacetylation of transcription factors and histone, is found being downregulated in proximal tubules preceding podocyte injury in DKD (99). Claudin-1 is a membrane protein involved in the formation of tight junctions and is normally expressed in parietal epithelial cells, which creates tight junctions that might prevent leakage from Bowman's capsule (100). In DKD, the downregulated proximal tubular SIRT1 decreases SIRT1 level in podocytes, thereby leading to the ectopic expression of claudin-1 in podocytes and causing albuminuria (101). Glomerular hyperfiltration is proposed to be resulted from tubular growth and upregulates sodium-glucose cotransporter 2 (SGLT2), which enhances proximal tubular reabsorption, leading a reduction of sodium chloride (NaCl) delivery to the macula densa, therefore increasing GFR via TGF response (SGLT2-NaCl pathway) (99–101).
Recently Hasegawa group also reported SGLT2 was elevated during early stages of DKD, which could upregulate intracellular glucose levels in proximal tubules and subsequently decrease SIRT1 expression whereas SGLT2 inhibitors preserved SIRT1 expression (102). SGLT2 inhibitors, suggested Hasegawa, might maintain the proximal tubule-podocyte communication. Other tubular-glomerular communications include a group of exosomes enriched with microRNA (miR) mediating podocyte or proximal tubular cell damage (103).
The communications between macrophages and kidney cells rely much on extracellular vesicles (EV). It was reported that miR-21-5p in macrophage-derived EVs regulated pyroptosis-mediated podocyte injury by A20 in DKD (104). It was also suggested that exosomal miR-19b-3p mediated the communication between injured tubular cells and macrophages, leading to M1 macrophage activation (105). Exosomes from high glucose-treated macrophages were implied to activate GMCs via TGF-β1/Smad3 pathway (106).
Findings Based on scRNA-Seq
A scRNA-seq performed on whole kidney cells from healthy mice revealed specific cell types responding to specific kidney related disorders (30). CKD related genes are strongly enriched in proximal tubules. The researchers identified a transitional cell type between principle cell (PC) and intercalated cell (IC) in collecting duct. Notch regulates the cellular identity of neighboring cells by the expression of either Notch ligands or Notch receptors. Genes encoding Notch ligands were highly expressed in ICs while Notch2 receptor and its transcriptional target Hes1 were shown in PCs with high expression level, suggesting that PCs are the Notch signal-receiving cells in the collecting duct. A higher ratio of PCs to ICs in human diabetic kidney biopsy with increased Notch signaling and HES1 expression suggested a shift toward PCs, which is likely the cause of metabolic acidosis in mouse models and patients with CKD (30). A human kidney scRNA-seq research identified NAMPT expressed in mesangial cells, which regulates insulin secretion in pancreatic β-cells, while uncovered a decreased expression of insulin receptors in diabetic podocytes (95). Interestingly, a single-cell transcriptome profiling performed on BTBR ob/ob mice, which do not develop hypertension, showed those animals had no major changes in endothelial cell gene expression while surprisingly gave the vascular disease stereotype of diabetes (34). The researchers suggested that rather hypertension not diabetes induce transcriptional changes in endothelial cells given the prevalence of hypertension in patients with diabetes (34). They thought hypertension might be more important in injuring endothelial cells. However, there remains confusion since findings to date about kidney crosstalk much developed from researches set under the diabetic milieu. The extraglomerular crosstalks are summarized in Table 2.
Discussion
scRNA-seq is a powerful tool providing unprecedented insight into cell transcriptome, including deciphering cell-to-cell communication in diseases such as DKD. With the aid of scRNA-seq, some new and complicated cellular interactive in DKD have been revealed as well as some new cell subpopulations have been identified in kidney, which imply some key regulators and therapeutic targets for DKD. Though the technology has achieved great advances, the researchers have to face several challenges during seRNA-seq. The cell isolation protocol needs to be optimized to be more efficient when balancing between cell dissociative efficacy and viability since kidney has relatively dense matrix and some of kidney cells under abnormal conditions are susceptible to loss. The big discrepancy on kidney cell numbers and gene expressions gained in different kidney seRNA-seq researches by now is attributed much to their different dissociation protocols. The types of kidney cell identified in scRNA-seq researches primarily depend on the available cell markers, which may not be specific enough or even yet be revealed. Moreover, the huge volume of complex data generated by seRNA-seq needs appropriate analytical and statistical methods and the interpretation of raw data is determined by the choices of computational tools and databases. Of the most importance, the findings drawn from scRNA-seq need to be validated by subsequent experimental tests.
More researches of scRNA-seq coupled with multiomic approaches are expected in future to gain closer access to profound pathogenesis of DKD and contribute to develop new therapeutic strategies.
Author Contributions
All literatures were reviewed by FY and CD. Materials were collected by YR, GL, YY, and ZY. The final manuscript was drafted by CD and FY, reviewed by FY and approved by all authors.
Funding
This study was supported by grants from the National Natural Science Foundation of China (No. 82000773), S&T Program of HeBei (18277748D), Major projection of Hebei Provincial Department of Education (ZD2018007), and Natural Science Foundation of Hebei Province (No. H2020206340).
Conflict of Interest
The authors declare that the research was conducted in the absence of any commercial or financial relationships that could be construed as a potential conflict of interest.
Publisher's Note
All claims expressed in this article are solely those of the authors and do not necessarily represent those of their affiliated organizations, or those of the publisher, the editors and the reviewers. Any product that may be evaluated in this article, or claim that may be made by its manufacturer, is not guaranteed or endorsed by the publisher.
References
1. Thomas MC, Brownlee M, Susztak K, Sharma K, Jandeleit-Dahm KA, Zoungas S, et al. Diabetic kidney disease. Nat Rev Dis Primers. (2015) 1:15018. doi: 10.1038/nrdp.2015.70
2. Bojestig M, Arnqvist HJ, Hermansson G, Karlberg BE, Ludvigsson J. Declining incidence of nephropathy in insulin-dependent diabetes mellitus. N Engl J Med. (1994) 330:15–8. doi: 10.1056/NEJM199401063300103
3. Hovind P, Tarnow L, Rossing K, Rossing P, Eising S, Larsen N, et al. Decreasing incidence of severe diabetic microangiopathy in type 1 diabetes. Diabetes Care. (2003) 26:1258–64. doi: 10.2337/diacare.26.4.1258
4. Burrows NR Li Y, Geiss LS. Incidence of treatment for end-stage renal disease among individuals with diabetes in the U. S continues to decline. Diabetes Care. (2010) 33:73–7. doi: 10.2337/dc09-0343
5. Zoccali C, Kramer A, Jager K. The databases: renal replacement therapy since 1989-the European Renal Association and European Dialysis and Transplant Association (ERA-EDTA). Clin J Am Soc Nephrol. (2009) 1:S18–22. doi: 10.2215/CJN.05210709
6. Ogurtsova K, da Rocha Fernandes JD, Huang Y, Linnenkamp U, Guariguata L, Cho NH, et al. IDF diabetes atlas: global estimates for the prevalence of diabetes for 2015 and 2040. Diabetes Res Clin Pract. (2017) 128:40–50. doi: 10.1016/j.diabres.2017.03.024
7. Westwood ME, Argirov OK, Abordo EA, Thornalley PJ. Methylglyoxal-modified arginine residues–a signal for receptor-mediated endocytosis and degradation of proteins by monocytic THP-1 cells. Biochim Biophys Acta. (1997) 1356:84–94. doi: 10.1016/S0167-4889(96)00154-1
8. Brownlee M. Biochemistry and molecular cell biology of diabetic complications. Nature. (2001) 414:813–20. doi: 10.1038/414813a
9. Alicic RZ, Rooney MT, Tuttle KR. Diabetic Kidney Disease: Challenges, Progress, and Possibilities. Clin J Am Soc Nephrol. (2017) 12:2032–45. doi: 10.2215/CJN.11491116
10. Borch-Johnsen K, Nørgaard K, Hommel E, Mathiesen ER, Jensen JS, Deckert T, et al. Is diabetic nephropathy an inherited complication? Kidney Int. (1992) 41:719–22. doi: 10.1038/ki.1992.112
11. Seaquist ER, Goetz FC, Rich S, Barbosa J. Familial clustering of diabetic kidney disease. Evidence for genetic susceptibility to diabetic nephropathy. N Engl J Med. (1989) 320:1161–5. doi: 10.1056/NEJM198905043201801
12. Retnakaran R, Cull CA, Thorne KI, Adler AI, Holman RR, Group US. Risk factors for renal dysfunction in type 2 diabetes: U.K. prospective diabetes study 74. Diabetes. (2006) 55:1832–9. doi: 10.2337/db05-1620
13. Bertram JF, Douglas-Denton RN, Diouf B, Hughson MD, Hoy WE. Human nephron number: implications for health and disease. Pedia Nephrol. (2011) 26:1529–33. doi: 10.1007/s00467-011-1843-8
14. Lindstrom NO, McMahon JA, Guo J, Tran T, Guo Q, Rutiedge E, et al. Conserved and divergent features of human and mouse kidney organogenesis. J Am Soc Nephrol. (2018) 29:785–805. doi: 10.1681/ASN.2017080887
15. Wang Y, Navin NE. Advances and applications of single-cell sequencing technologies. Mol Cell. (2015) 58:598–609. doi: 10.1016/j.molcel.2015.05.005
16. Gawad C, Koh W, Quake SR. Single-cell genome sequencing: current state of the science. Nat Rev Genet. (2016) 17:175–88. doi: 10.1038/nrg.2015.16
17. Dean FB, Hosono S, Fang L, Wu X, Faruqi AF, Bray-Ward P, et al. Comprehensive human genome amplification using multiple displacement amplification. Proc Natl Acad Sci U S A. (2002) 99:5261–6. doi: 10.1073/pnas.082089499
18. Mardis, ER A. decade's perspective on DNA sequencing technology. Nature. (2011) 470:198–203. doi: 10.1038/nature09796
19. Navin N, Kendall J, Troge J, Andrews P, Rodgers L, McIndoo J, et al. Tumour evolution inferred by single-cell sequencing. Nature. (2011) 472:90–4. doi: 10.1038/nature09807
20. Tang F, Barbacioru C, Wang Y, Nordman E, Lee C, Xu N, et al. mRNA-Seq whole-transcriptome analysis of a single cell. Nat Methods. (2009) 6:377–82. doi: 10.1038/nmeth.1315
21. Streets AM, Zhang X, Cao C, Pang Y, Wu X, Xiong L, et al. Microfluidic single-cell whole transcriptome sequencing. Proc Natl Acad Sci U S A. (2014) 111:7048–53. doi: 10.1073/pnas.1402030111
22. Shalek AK, Satija R, Shuga J, Trombetta JJ, Gennert D, Lu D, et al. Single-cell RNA-seq reveals dynamic paracrine control of cellular variation. Nature. (2014) 510:363–9. doi: 10.1038/nature13437
23. Macosko EZ, Basu A, Satija R, Nemesh J, Shekhar K, Goldman M, et al. Highly parallel genome-wide expression profiling of individual cells using nanoliter droplets. Cell. (2015) 161:1202–14. doi: 10.1016/j.cell.2015.05.002
24. Klein AM, Mazutis L, Akartuna I, Tallapragada N, Veres A, Li V, et al. Droplet barcoding for single cell transcriptomics applied to embryonic stem cells. Cell. (2015) 161:1187–201. doi: 10.1016/j.cell.2015.04.044
25. Rosenberg AB, Roco CM, Muscat RA, Kuchina A, Sample P, Yao Z, et al. Single-cell profiling of the developing mouse brain and spinal cord with split-pool barcoding. Science. (2018) 360:176–82. doi: 10.1126/science.aam8999
26. Wu H, Humphreys BD. The Promise of Single Cell RNA-Sequencing for Kidney Disease Investigation. Kidney Int. (2017) 96:1334–42. doi: 10.1016/j.kint.2017.06.033
27. Zhou Q, Xiong Y, Huang XR, Tang P, Yu X, Lan HY, et al. Identification of genes associated with Smad3-dependent Renal Injury by RNA-seq-based transcriptome analysis. Sci Rep. (2015) 5:17901. doi: 10.1038/srep17901
28. Nakagawa S, Nishihara K, Miyata H, Shinke H, Tomita E, Kajiwara M, et al. Molecular markers of tubulointerstitial fibrosis and tubular cell damage in patients with chronic kidney disease. PLoS ONE. (2015) 10:e0136994. doi: 10.1371/journal.pone.0136994
29. McMahon AP, Aronow BJ, Davidson DR, Davies JA, Gaido KW, Grimmond S, et al. GUDMAP: the genitourinary developmental molecular anatomy project. J Am Soc Nephrol. (2008) 19:667–71. doi: 10.1681/ASN.2007101078
30. Park J, Shrestha R, Qiu C, Kondo A, Huang S, Werth M, et al. Single-cell transcriptomics of the mouse kidney reveals potential cellular targets of kidney disease. Science. (2018) 360:758–63. doi: 10.1126/science.aar2131
31. Liao J, Yu Z, Chen Y, Bao M, Zou C, Zhang H, et al. Single-cell RNA sequencing of human kidney. Sci Data. (2020) 7:4. doi: 10.1038/s41597-019-0351-8
32. Benjamin Humphreys (Re)Building a Kidney Consortium. (2018) Available online at: https://doi.org/10.25548/14-4KG6
33. Wu H, Malone AF, Donnelly EL, Kirita Y, Uchimura K, Ramakrishnan SM, et al. Single-Cell Transcriptomics of a Human Kidney Allograft Biopsy Specimen Defines a Diverse Inflammatory Response. J Am Soc Nephrol. (2018) 29:2069–80. doi: 10.1681/ASN.2018020125
34. Chung JJ, Goldstein L, Chen YJ, Lee J, Webster JD, Roose-Girma M, et al. Single-Cell Transcriptome Profiling of the Kidney Glomerulus Identifies Key Cell Types and Reactions to Injury. J Am Soc Nephrol. (2020) 31:2341–54. doi: 10.1681/ASN.2020020220
35. Fu J, Akat KM, Sun Z, Zhang W, Schlondorff D, Liu Z, et al. Single-Cell RNA Profiling of Glomerular Cells Shows Dynamic Changes in Experimental Diabetic Kidney Disease. J Am Soc Nephrol. (2019) 30:533–45. doi: 10.1681/ASN.2018090896
36. Subramanian A, Sidhom EH, Emani M. Vernon1K, SahakianN, Zhou Y, et al. Single cell census of human kidney organoids shows reproducibility and diminished off-target cells after transplantation. Nat Commun. (2019) 10:5462. doi: 10.1038/s41467-019-13382-0
37. Kim KT, Lee HW, Lee HO, Song HJ, Jeong DE, Shin S, et al. Application of single-cell RNA sequencing in optimizing a combinatorial therapeutic strategy in metastatic renal cell carcinoma. Genome Bio. (2016) 17:80. doi: 10.1186/s13059-016-0945-9
38. Young MD, Mitchell T, Vieira Braga FA, Tran MGB, Stewart BJ, Ferdinand JR, et al. Single-cell transcriptomes from human kidneys reveal the cellular identity of renal tumors. Science. (2018) 361:594–9. doi: 10.1126/science.aat1699
39. Muto Y, Wilson PC, Ledru N, Wu H, Dimke H, Waikar SS, et al. Single cell transcriptional and chromatin accessibility profiling redefine cellular heterogeneity in the adult human kidney. Nat Commun. (2021) 12:2190. doi: 10.1038/s41467-021-22368-w
40. Miao Z, Balzer MS, Ma Z, Liu H, Wu J, Shrestha R, et al. Single cell regulatory landscape of the mouse kidney highlights cellular differentiation programs and disease targets. Nat Commun. (2021) 12:2277. doi: 10.1038/s41467-021-22266-1
41. Denisenko E, Guo BB, Jones M, Hou R, de Kock L, Lassmann T, et al. Systematic assessment of tissue dissociation and storage biases in single-cell and single-nucleus RNA-seq workflows. Genome Biol. (2020) 21:130. doi: 10.1186/s13059-020-02048-6
42. Chen L, Lee JW, Chou CL, Nair AV. Battis- tone MA, Păunescu TG, et al. Transcriptomes of major renal collecting duct cell types in mouse identified by single-cell RNA-seq. Proc Natl Acad Sci U S A. (2017) 114:E9989–98. doi: 10.1073/pnas.1710964114
43. Karaiskos N, Rahmatollahi M, Boltengagen A, Liu H, Hoehne M, Rinschen M, et al. A single-cell transcriptome atlas of the mouse glomerulus. J Am Soc Nephrol. (2018) 29:2060–8. doi: 10.1681/ASN.2018030238
44. Wu H, Kirita Y, Donnelly EL, Humphreys BD. Advantages of single-nucleus over single-cell RNA sequencing of adult kidney: rare cell types and novel cell states revealed in fibrosis. J Am Soc Nephrol. (2019) 30:23–32. doi: 10.1681/ASN.2018090912
45. Park J, Liu C, Kim J, Susztak K. Understanding the kidney one cell at a time. Kidney Int. (2019) 96:862–70. doi: 10.1016/j.kint.2019.03.035
46. Lake BB, Chen S, Hoshi M, Plongthongkum N, Salamon D, Knoten A, et al. A single-nucleus RNA-sequencing pipeline to decipher the molecular anatomy and pathophysiology of human kidneys. Nat Commu. (2019) 10:2832. doi: 10.1038/s41467-019-10861-2
47. Armingol E, Officer A, Harismendy O, Lewis NE. Deciphering cell-cell interactions and communication from gene expression. Nat Rev Genet. (2021) 22:71–88. doi: 10.1038/s41576-020-00292-x
48. Jin S, Guerrero-Juarez CF, Zhang L, Chang I, Romos R, Kuan CH, et al. Inference and analysis of cell-cell communication using CellChat. Nature Communications. (2021) 12:1088. doi: 10.1038/s41467-021-21246-9
49. Vento-Tormo R, Efremova M, Botting RA, Turco MY, Vento-Tormo M, Meyer KB, et al. Single-cell reconstruction of the early maternal-fetal interface in humans. Nature. (2018) 563:347–53. doi: 10.1038/s41586-018-0698-6
50. Browaeys R, Saelens W. & Saeys, Y. Niche. Net: modeling intercellular communication by linking ligands to target genes. Nat Methods. (2020) 17:159–62. doi: 10.1038/s41592-019-0667-5
51. Schlondorff D. Putting the glomerulus back together: per aspera ad astra (“a rough road leads to the stars”). Kidney Int. (2014) 85:991–8. doi: 10.1038/ki.2014.51
52. Dimke H, Maezawa Y, Quaggin SE. Crosstalk in glomerular injury and repair. Curr Opin Nephrol Hypertens. (2015) 24:231–8. doi: 10.1097/MNH.0000000000000117
53. Siddiqi FS, Advani A. Endothelial-podocyte crosstalk: the missing link between endothelial dysfunction and albuminuria in diabetes. Diabetes. (2013) 62:3647–55. doi: 10.2337/db13-0795
54. Daehn I. Shift in focus to explore the role of the endothelium in kidney disease. HSOA J Nephrol Ren Ther. (2016) 2:004. doi: 10.24966/NRT-7313/100004
55. Ebefors K, Nystrom J. New insights into crosstalk in the kidney. Curr Opin Nephrol Hypertens. (2017) 26:143–7. doi: 10.1097/MNH.0000000000000310
56. Ohse T, Vaughan MR, Kopp JB, Krofft RD, Marshall CB, Chang AM, et al. De novo expression of podocyte proteins in parietal epithelial cells during experimental glomerular disease. Am J Physiol Renal Physiol. (2010) 298:F702–11. doi: 10.1152/ajprenal.00428.2009
57. Luque Y, Lenoir O, Bonnin P, Hardy L, Chipont A, Placier S, et al. Endothelial Epas1 deficiency is sufficient to promote parietal epithelial cell activation and FSGS in experimental hypertension. J Am Soc Nephrol. (2017) 28:3563–78. doi: 10.1681/ASN.2016090960
58. Persson F, Rossing P, Hovind P, Stehouwer CD, Schalkwijk C, Tarnow L, et al. Irbesartan treatment reduces biomarkers of inflammatory activity in patients with type 2 diabetes and microalbuminuria: an IRMA 2 substudy. Diabetes. (2006) 55:3550–5. doi: 10.2337/db06-0827
59. Toyoda M, Najafian B, Kim Y, Caramori ML, Mauer M. Podocyte detachment and reduced glomerular capillary endothelial fenestration in human type 1 diabetic nephropathy. Diabetes. (2007) 56:2155–60. doi: 10.2337/db07-0019
60. Sison K, Eremina V, Baelde H, Min W, Hirashima M, Fantus IG, et al. Glomerular structure and function require paracrine, not autocrine, VEGF- VEGFR-2 signaling. J Am Soc Nephrol. (2010) 21:1691–701. doi: 10.1681/ASN.2010030295
61. Jeansson M, Gawlik A, Anderson G, Li C, Kerjaschki D, Henkelman M, et al. Angiopoietin-1 is essential in mouse vasculature during development and in response to injury. J Clin Invest. (2011) 121:2278–89. doi: 10.1172/JCI46322
62. Sivaskandarajah GA, Jeansson M, Maezawa Y, Eremina V, Baelde HJ, Quaggin SE. Vegfa protects the glomerular microvasculature in diabetes. Diabetes. (2012) 61:2958–66. doi: 10.2337/DB11-1655
63. Veron D, Reidy KJ, Bertuccio C, Teichman J, Villegas G, Jimenez J, et al. Overexpression of VEGF-A in podocytes of adult mice causes glomerular disease. Kidney Int. (2010) 77:989–99. doi: 10.1038/ki.2010.64
64. Satchell SC, Harper SJ, Tooke J, Kerjaschki N, Saleem M, Mathieson PW. Human podocytes express angiopoietin 1, a potential regulator of glomerular vascular endothelial growth factor. J Am Soc Nephrol. (2002) 13:544–50. doi: 10.1681/ASN.V132544
65. Daehn I, Casalena G, Zhang T, Shi S, Fenninger F, Barasch N, et al. Endothelial mitochondrial oxidative stress determines podocyte depletion in segmental glomerulosclerosis. J Clin Investig. (2014) 124:1608–21. doi: 10.1172/JCI71195
66. Ebefors K, Wiener RJ Yu L, Azeloglu EU Yi Z, Jia F, et al. Endothelin receptor-A mediates degradation of the glomerular endothelial surface layer via pathologic crosstalk between activated podocytes and glomerular endothelial cells. Kidney Int. (2019) 96:957–70. doi: 10.1016/j.kint.2019.05.007
67. Boels MGS, Avramut MC, Koudijs A, Dane MJ, Lee DH, Van Der Vlag J, et al. Atrasentan reduces albuminuria by restoring the glomerular endothelial glycocalyx barrier in diabetic nephropathy. Diabetes. (2016) 65:2429–39. doi: 10.2337/db15-1413
68. Qi H, Casalena G, Shi S, Yu L. Ebefors, K, Sun Y, et al. Glomerular endothelial mitochondrial dysfunction is essential and characteristic of diabetic kidney disease susceptibility. Diabetes. (2017) 66:763–78. doi: 10.2337/db16-0695
69. Lassén M Daehn IS. Molecular mechanisms in early diabetic kidney disease: glomerular endothelial cell dysfunction. Int J Mol Sci. (2020) 21:9456. doi: 10.3390/ijms21249456
70. Sayyed SG, Hägele H, Kulkarni OP, Endlich K, Segerer S, Eulberg D, et al. Podocytes produce homeostatic chemokine stromal cell-derived factor-1/CXCL12. which contributes to glomerulosclerosis, podocyte loss and albuminuria in a mouse model of type 2 diabetes. Diabetologia. (2009) 52:2445–54. doi: 10.1007/s00125-009-1493-6
71. Daehn IS. Glomerular endothelial Cell Stress and Cross-Talk with Podocytes in early Diabetic Kidney Disease. Front Med (Lausanne). (2018) 23:76. doi: 10.3389/fmed.2018.00113
72. Isermann B, Vinnikov IA, Madhusudhan T, Herzog S, Kashif M, Blautzik J, et al. Activated protein C protects against diabetic nephropathy by inhibiting endothelial and podocyte apoptosis. Nat Med. (2007) 13:1349–58. doi: 10.1038/nm1667
73. Zhong F, Chen H, Wei C, Zhang W, Li Z, Jain MK, et al. Reduced Krüppel-like factor 2 expression may aggravate the endothelial injury of diabetic nephropathy. Kidney Int. (2015) 87:382–95. doi: 10.1038/ki.2014.286
74. Wu X, Gao Y, Xu L, Dang W, Yan H, Zou D, et al. Exosomes from high glucose-treated glomerular endothelial cells trigger the epithelial-mesenchymal transition and dysfunction of podocytes. Sci Rep. (2017) 7:9371. doi: 10.1038/s41598-017-09907-6
75. Yuen DA, Stead BE, Zhang Y, White KE, Kabir MG, Thai K et al. eNOS Deficiency Predisposes Podocytes to Injury in Diabetes. J Am Soc Nephrol. (2012) 23:1810–23. doi: 10.1681/ASN.2011121170
76. Grahammer F, Benzing T, Huber TB. New insights into mechanisms of glomerular injury and repair from the 10th International Podocyte Conference 2014. Kidney Int. (2015) 87:885–93. doi: 10.1038/ki.2015.5
77. Bollee G, Flamant M, Schordan S, Fligny C, Rumpel E, Milon M, et al. Epidermal growth factor receptor promotes glomerular injury and renal failure in rapidly progressive crescentic glomerulonephritis. Nat Med. (2011) 17:1242–50. doi: 10.1038/nm.2491
78. Appel D, Kershaw DB, Smeets B, Yuan G, Fuss A, Frye B, et al. Recruitment of podocytes from glomerular parietal epithelial cells. J Am Soc Nephrol. (2009) 20:333–43. doi: 10.1681/ASN.2008070795
79. Ohse T, Pippin JW, Chang AM, Krofft RD, Miner JH, Vaughan MR, et al. The enigmatic parietal epithelial cell is finally getting noticed: a review. Kidney Int. (2009) 76:1225–38. doi: 10.1038/ki.2009.386
80. Ronconi E, Sagrinati C, Angelotti ML, Lazzeri E, Mazzinghi B, Ballerini L, et al. Regeneration of Glomerular Podocytes by Human Renal Progenitors. J Am Soc Nephrol. (2009) 20:322–32. doi: 10.1681/ASN.2008070709
81. Lasagni L, Romagnani P. Glomerular epithelial stem cells: the good, the bad, and the ugly. J Am Soc Nephrol. (2010) 21:1612–9. doi: 10.1681/ASN.2010010048
82. Sagrinati C, Netti GS, Mazzinghi B, Lazzeri E, Liotta F, Frosali F, et al. Isolation and characterization of multipotent progenitor cells from the Bowman's capsule of adult human kidneys. J Am Soc Nephrol. (2006) 17:2443–56. doi: 10.1681/ASN.2006010089
83. Tan H, Yi H, Zhao W, Ma JX, Zhang Y, Zhou X. Intraglomerular crosstalk elaborately regulates podocyte injury and repair in diabetic patients: insights from a 3D multiscale modeling study. Oncotarget. (2016) 7:73130–46. doi: 10.18632/oncotarget.12233
84. Schlöndorff D, Banas B. The mesangial cell revisited: No cell is an island. J Am Soc Nephrol. (2009) 20:1179–87. doi: 10.1681/ASN.2008050549
85. Floege J, Eitner F, Alpers CE A. new look at platelet-derived growth factor in renal disease. J Am Soc Nephrol. (2008) 19:12–23. doi: 10.1681/ASN.2007050532
86. Leveen P, Pekny M, Gebre-Medhin S, Swolin B, Larsson E, Betsholtz C. Mice deficient for PDGF B show renal, cardiovascular, and hematological abnormalities. Genes Dev. (1994) 8:1875–87. doi: 10.1101/gad.8.16.1875
87. Soriano P. Abnormal kidney development and hematological disorders in PDGF beta-receptor mutant mice. Genes Dev. (1994) 8:1888–96. doi: 10.1101/gad.8.16.1888
88. Thongboonkerd V. Roles for exosome in various kidney diseases and disorders. Front Pharmacol. (2020) 31:1655. doi: 10.3389/fphar.2019.01655
89. Wu XM, Gao Y, Cui F, Zhang N. Exosomes from high glucose-treated glomerular endothelial cells activate mesangial cells to promote renal fibrosis. Biol Open. (2016) 5:484–91. doi: 10.1242/bio.015990
90. Tufro A, Veron D VEGF. and Podocytes in diabetic nephropathy. Semin Nephrol. (2012) 32:385–93. doi: 10.1016/j.semnephrol.2012.06.010
91. Khan S, Lakhe-Reddy S, McCarty JH, Sorenson CM, Sheibani N, Reichardt LF, et al. Mesangial cell integrin αvβ8 provides glomerular endothelial cell cytoprotection by sequestering TGF-β and regulating PECAM-1. Am J Pathol. (2011) 178:609–20. doi: 10.1016/j.ajpath.2010.10.031
92. Fujimoto D, Kuwabara T, Hata Y, Umemoto S, Kank T, Nishiguchi Y, et al. Suppressed ER-associated degradation by intraglomerular cross talk between mesangial cells and podocytes causes podocyte injury in diabetic kidney disease. FASEB J. (2020) 34:15577–90. doi: 10.1096/fj.202000078RR
93. Mitu GM, Wang S, Hirschberg R. BMP7 is a podocyte survival factor and rescues podocytes from diabetic injury. Am J Physiol Renal Physiol. (2007) 293:F1641–8. doi: 10.1152/ajprenal.00179.2007
94. Yeh CH, Chang CK, Cheng MF, Lin HJ, Cheng JT. Decrease of bone morphogenetic protein-7 (BMP-7) and its type II receptor (BMP-RII) in kidney of type 1-like diabetic rats. Horm Metab Res. (2009) 41:605–11. doi: 10.1055/s-0029-1220736
95. Wilson PC, Wu H, Kirta Y, Uchimura K, Ledru N, Rennke HG et al. The single-cell transcriptomic landscape of early human diabetic nephropathy. Proc Natl Acad Sci U S A. (2019) 116:19619–25. doi: 10.1073/pnas.1908706116
96. Lu Y, Ye Y, Yang Q, Shi S. Single-cell RNA-sequence analysis of mouse glomerular mesangial cells uncovers mesangial cell essential genes. Kidney Int. (2017) 92:504–13. doi: 10.1016/j.kint.2017.01.016
97. Vallon V, Richter K, Blantz RC, Thomson S, Osswald H. Glomerular hyperfiltration in experimental diabetes mellitus: Potential role of tubular reabsorption. J Am Soc Nephrol. (1999) 10:2569–76. doi: 10.1681/ASN.V10122569
98. Vallon V, Thomson SC. Renal function in diabetic disease models: The tubular system in the pathophysiology of the diabetic kidney. Annu Rev Physiol. (2012) 74:351–75. doi: 10.1146/annurev-physiol-020911-153333
99. Umino H, Hasegawa K, Minakuchi H, Muraoka H, Kawaguchi T, Kanda T, et al. High basolateral glucose increases sodium-glucose cotransporter 2 and reduces sirtuin-1 in renal tubules through glucose transporter-2 detection. Sci Rep. (2018) 8:6791. doi: 10.1038/s41598-018-25054-y
100. Gong Y, Hou J. Claudins in barrier and transport function-the kidney. Pflugers Arch. (2017) 469:105–13. doi: 10.1007/s00424-016-1906-6
101. Thomson SC, Deng A, Bao D, Satriano J, Blantz RC, Vallon V. Ornithine decarboxylase, kidney size, and the tubular hypothesis of glomerular hyperfiltration in experimental diabetes. J Clin Invest. (2001) 107:217–24. doi: 10.1172/JCI10963
102. Hasegawa K, Wakino S, Simic P, Sakamaki Y, Minakuchi H, Fujimura K. Renal tubular Sirt1 attenuates diabetic albuminuria by epigenetically suppressing Claudin-1 overexpression in podocytes. Nat Med. (2013) 19:1496–504. doi: 10.1038/nm.3363
103. Su H, Qiao J, Hu J, Li Y, Lin J, Yu Q, et al. Podocyte-derived extracellular vesicles mediate renal proximal tubule cells dedifferentiation via microRNA-221 in diabetic nephropathy. Mol Cell Endocrinol. (2020) 518:111034. doi: 10.1016/j.mce.2020.111034
104. Ding X, Jing N, Shen A, Guo F, Song Y, Pan M, et al. MiR-21-5p in macrophage-derived extracellular vesicles affects podocyte pyroptosis in diabetic nephropathy by regulating A20. J Endocrinol Invest. (2020) 15. doi: 10.1007/s40618-020-01401-7
105. Lv LL, Feng Y, Wu M, Wang B, Li ZL, Zhong X, et al. Exosomal miRNA-19b-3p of tubular epithelial cells promotes M1 macrophage activation in kidney injury. Cell Death Differ. (2020) 27:210–26. doi: 10.1038/s41418-019-0349-y
Keywords: single-cell RNA sequencing, crosstalk, diabetic kidney disease, glomerulus, tubular epithelial cell
Citation: Du C, Ren Y, Li G, Yang Y, Yan Z and Yao F (2021) Single Cell Transcriptome Helps Better Understanding Crosstalk in Diabetic Kidney Disease. Front. Med. 8:657614. doi: 10.3389/fmed.2021.657614
Received: 23 January 2021; Accepted: 26 July 2021;
Published: 17 August 2021.
Edited by:
Quan Hong, Chinese PLA General Hospital, ChinaReviewed by:
Seung Seok Han, Seoul National University, South KoreaAnjun Ma, The Ohio State University, United States
Copyright © 2021 Du, Ren, Li, Yang, Yan and Yao. This is an open-access article distributed under the terms of the Creative Commons Attribution License (CC BY). The use, distribution or reproduction in other forums is permitted, provided the original author(s) and the copyright owner(s) are credited and that the original publication in this journal is cited, in accordance with accepted academic practice. No use, distribution or reproduction is permitted which does not comply with these terms.
*Correspondence: Fang Yao, yaofang2006@hotmail.com