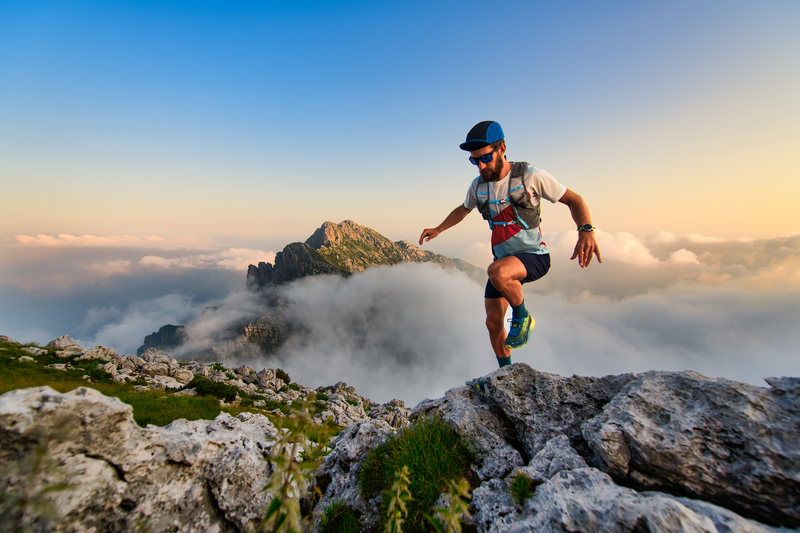
94% of researchers rate our articles as excellent or good
Learn more about the work of our research integrity team to safeguard the quality of each article we publish.
Find out more
PERSPECTIVE article
Front. Med. , 13 April 2021
Sec. Infectious Diseases: Pathogenesis and Therapy
Volume 8 - 2021 | https://doi.org/10.3389/fmed.2021.641260
Virulence arresting drugs (VAD) are an expanding class of antimicrobial treatment that act to “disarm” rather than kill bacteria. Despite an increasing number of VAD being registered for clinical use, uptake is hampered by the lack of methods that can identify patients who are most likely to benefit from these new agents. The application of pathogen genomics can facilitate the rational utilization of advanced therapeutics for infectious diseases. The development of genomic assessment of VAD targets is essential to support the early stages of VAD diffusion into infectious disease management. Genomic identification and characterization of VAD targets in clinical isolates can augment antimicrobial stewardship and pharmacovigilance. Personalized genomics guided use of VAD will provide crucial policy guidance to regulating agencies, assist hospitals to optimize the use of these expensive medicines and create market opportunities for biotech companies and diagnostic laboratories.
Many first-in-class therapies are now entering the global marketplace, evolving at a rapid pace and fueled by advances in genetics and informatics and expectations of precision medicine (1, 2). Some “N-of-1” personalized therapies can be rationally designed and tailored to a particular patient (3). Between 2015 and 2019, a quarter of US FDA approvals for first-in-class' antimicrobial agents have been so-called virulence arresting drugs (VAD). VAD are an expanding class of therapies that act to “disarm” rather than kill pathogens (4, 5), and because most virulence traits are non-essential for bacterial survival VAD are less susceptible to the development of antimicrobial resistance (6). They can restore or augment the effect of traditional antibiotics, but are more pathogen-specific and generally spare the body's healthy bacteria (4).
Treatments focusing on anti-virulence effects are not a new idea—the first Nobel Prize in Medicine was awarded in 1901 for the discovery of diphtheria antitoxin. However, in recent years, recombinant DNA technology has revolutionized the scope of anti-virulence strategies, with multiple new drugs showing efficacy and safety in clinical trials (5, 7) and the possibility of engineered antimicrobials offering the prospect of selective killing of target bacteria (8). In total, more than 100 VAD candidates had been reported; seven are already registered by the US Federal Drug Agency (FDA) and/or European Medicines Agency (EMA), while eight more are in Phase II/III clinical trials. In addition, 40 re-purposed drugs with established safety profiles and registered with the FDA/EMA for the treatment of non-infective conditions may hold VAD promise. Figure 1 captures the most advanced classes of VAD with examples of agents available for clinical use or in clinical trials. However, there are also other virulence mechanisms targeted by VAD candidates such as bacterial metabolism (9, 10) and virulence transcription regulation (11, 12). The appeal of VADs for precision medicine is enhanced by the potential for new discoveries using forward and reverse genetics, and recognition of genetic variability among the various strains within species, which demands tailored treatment.
Critical challenges hampering the clinical use of VAD must be acknowledged. First, an empirical or “trial-and-error” approach to VAD prescribing will be extremely wasteful given their high cost and may delay the use of more appropriate treatment. Second, conventional drug susceptibility testing approaches are not useful for VAD selection. We consider how these challenges may be addressed and outline new opportunities in the application of pathogen genomics in facilitating the rational use of advanced therapeutics for infectious diseases.
Many pathogens excrete potent exotoxins as a major virulence factor (VF) that adversely affects host cells. Recent advances in bioengineering facilitate the design and synthesis of humanized monoclonal antibodies (hMAb) that can specifically target and neutralize these proteins (7, 8, 13). Several therapeutic agents have demonstrated benefit in clinical trials for the treatment of diseases caused by toxin-producing bacteria; for example, Bezlotoxumab (Zinplava®) and Actoxumab bind Clostridium difficile toxins B and A, respectively. Bezlotoxumab have been used to treat recurrent colitis caused by toxin B producing organisms (13). Urtoxazumab, a hMAb against Shiga-like toxin 2 aims to reduce the risk of hemolytic uremic syndrome in patients infected with Shiga-like toxin-producing Escherichia coli (7). Raxibacumab and Obiltoxaximab received accelerated FDA approval for the treatment and prevention of inhalational anthrax, which is a potential bioterrorism agent that may cause rapidly progressive fatal disease (14).
Disruption of the collective behavior of bacteria coordinated by small signaling molecules, known as “quorum sensing” (QS, also called density sensing), has been proposed as another anti-virulence strategy (Figure 2). These signaling molecules, which include self-inducing oligopeptides, can activate cellular processes that affect virulence, motility, biofilm formation and drug resistance mechanisms, when produced in sufficient numbers by high-density bacterial populations. Quorum sensing inhibition or quorum quenching (QQ) aims to reduce or destroy the QS signal (15). QS circuits represent hierarchical cross-regulated networks e.g., the las-QS circuit positively regulates the rhl- and pqs-QS circuits. In Pseudomonas aeruginosa the QS system consists of two N-acyl-homoserine-lactone (AHL) regulatory circuits (genes las and rhl) and a non-AHL-mediated QS signaling pathway using hierarchically connected alkyl-4-quinolones (16).
Figure 2. Examples of variation in virulence genes and proteins affecting VAD targets. The size of letters representing nucleotides (DNA) or amino acids (Protein) reflects their relative frequency in individual positions in the VAD target gene. Only 6% of Streptococcus pneumoniae comB gene (75-bp of 1,326-bp gene) and 2% of Clostridium difficile tcdB gene (90-bp of 7,101-bp gene) are shown; multiple sequence variations with amino acid relevance are present. Amino acids are colored according to their chemical properties: polar amino acids are green, basic blue, and hydrophobic are black. The height of the y-axis indicates the maximum entropy for the given sequence type. Prepared using Weblogo (www.weblogo.berkeley.edu).
Inhibition of QS can be achieved by interference with the QS signal biosynthesis, inactivation of the QS signals or blocking of QS signal detection by receiving receptors. Inactivation of QS signals after they have been secreted can be achieved either by QQ enzymes, monoclonal antibodies or synthetic polymers modifying or binding to autoinducer proteins. A number of small molecules have demonstrated the ability to interfere with QS networks (16, 17). QS signal detection inhibitors, such as Furanone C-30, are structural analogs that bind to the receptor. Antagonists of particular QS signals affect the signaling cascade and exert inhibitory activity on the whole QS network (17). Numerous QQ agents have been identified that inhibit key QS pathways, AHL synthesis or AHL receptors in P. aeruginosa and other bacteria. However, the majority of QQ drugs remain in pre-clinical stages with some repurposed drugs in clinical trials (Figure 1) (18).
The interruption of bacterial adhesion to host cells appeals as a strategy to slow cellular invasion and buy time for the immune system to mount an effective response (19, 20). Attachment proteins on the surface of bacteria are critical to bind to cell receptors and are primary targets for the development of therapeutic antibodies, monobodies (small target binding proteins), or small molecules that delay or prevent bacterial adhesion. Monoclonal antibodies against fimbrial adhesin FimH of uropathogenic Escherichia coli can displace the ligand from the binding pocket and thereby block bacterial adhesion necessary for surface-adherent biofilms, facilitating treatment of recurrent urinary tract infections (21). Small molecules with the ability to inhibit adhesion and effector proteins secreted by bacterial secretion systems (SS) have shown promise in animal models (20, 22). For instance, Licoflovanol exhibited strong inhibitory effects on the secretion of Salmonella pathogenicity island 1 (SPI-1)-associated effector proteins via regulating the transcription of the sicA/invF genes, without affecting the growth of the bacteria (23).
Potent antivirulence properties have been discovered in medicines that were approved for the treatment of conditions other than bacterial infections. These drugs have established safety and well-understood bioavailability profiles, presenting an appealing treatment option. For example, in Staphylococcus aureus, the production of virulence factors is controlled by multiple regulators including SarA, Agr, ArlRS, and the SaeRS two-component system (TCS). The TCS controls the production of over 20 virulence factors including toxins (alpha-hemolysin, gamma-haemolysin, and leukocidins), coagulases, adhesins and different enzymes (e.g., nucleases and proteases). The FDA-approved anti-cancer drugs streptozotocin and floxuridine reduces the virulence of S. aureus in experimental models by inhibiting TCS (11).
Mepacrine, a 400-Da acridine derivative, has been used to prevent and treat protozoal infections. Recent recognition of mepacrine's inhibition of enterotoxin produced by Clostridium perfringens motivated its inclusion in the list of potential antivirulence drugs (24). Azathioprine, an immunosuppressive drug, also affect 5-aminoimidazole-4-carboxamide ribotide (AICAR) transformylase, an enzyme involved in purine biosynthesis in P. aeruginosa and other bacteria. Interestingly, this activity is abolished in an E. coli purH mutant strain, unable to produce AICAR transformylase (25), but its potential clinical use has not been explored. In silico molecular docking demonstrated that the antifungal medications clotrimazole and miconazole, as well as an antibacterial compound active against Gram-positive pathogens, clofoctol, can act as QS inhibitors by targeting the transcriptional regulator PqsR. The most active inhibitor, clofoctol, specifically inhibited the expression of pqs-controlled virulence traits in P. aeruginosa, such as pyocyanin production, swarming motility, biofilm formation, and expression of genes involved in siderophore production (26). The hypertension drug guanfacine can also act as a potent QS inhibitor for P. aeruginosa in biofilms (27), although the mechanism of action requires further elucidation. Antimicrobial effects can be achieved by lower doses of these repurposed drugs than those required for anti-cancer activity or immunosuppression, however, their safety and tolerability profiles as VAD candidates require further elucidation. For example, it might be inappropriate and unsafe to use hypertension or immunosuppression drugs as VAD, even in lower doses, especially in patients with low blood pressure or pre-existing immunosuppression.
The concept of a “druggable genome” was inspired by the identification of proteins as potential targets for novel agents or repurposed drugs using whole genome association studies (28). This concept opens the whole genome to interrogation aimed at predicting susceptibility to all available treatment options (29). The growing uptake of bench-top sequencing instruments by microbiology laboratories greatly improves access to pathogen genomics. The sequencing of clinically relevant bacteria has become appealing from both high-resolution diagnostics and laboratory automation points of view. Whole genome sequencing of bacteria from clinical cultures allows reliable drug susceptibility inference for a range of pathogens, especially those with well-defined resistance conferring mutations such as Mycobacterium tuberculosis. Several multi-center studies demonstrated high correlation between phenotypic and genotypic resistance in M. tuberculosis (30, 31). The growing size and improved curation of bacterial genome databases strengthen sequence similarity-based detection of VF and enable machine learning classifiers that utilize both genome composition and sequence homology (32, 33). Furthermore, the maturation of bioinformatics tools for bacterial genome assembly and annotation makes the analysis of core and variable genomes and the identification of important virulence markers more feasible (34).
The emergence of bacterial pathogens with multi-drug resistance strengthens the case for exploring alternative therapeutic approaches. Antibiotics are typically prescribed on an empirical basis because of their broad-spectrum antimicrobial activity and the limitations of culture guided clinical decision-making. The resultant misuse of antibiotics has contributed to the current antimicrobial resistance (AMR) crisis. Key examples of high priority AMR pathogens with potential VAD targets and candidate drugs that have demonstrated activity against these AMR pathogens are summarized in Table 1. High-resolution diagnostics are essential for rational VAD selection and current laboratory methods are insufficient to guide optimal therapy choice. The best patient group for using VAD as adjunct therapy to existing antibiotic treatment may be patients with chronic persistent infections caused by antibiotic resistant bacteria. Close alignment of VAD use with existing antibiotic stewardship practices and governance should minimize the risk of misuse threats from acquired resistance.
The precision of VAD selection within species of bacterial pathogens can be achieved by using comparative and subtractive genomics approaches. This in silico can identify species- and strain-specific genes or groups of genes that are responsible for a unique phenotype (i.e., virulence) and can be targeted by different classes of VAD. It is widely accepted that the virulence of bacterial pathogens can be predicted from their genomes, once the full diversity of VF has been documented (33). Genomic determinants of virulence can be classified into genes responsible for host damage, genes coding for proteins regulating the expression of these VF genes, and so called “life-style” genes enabling colonization, intracellular survival or immune system evasion. Thus, routine sequencing of bacteria responsible for infections of high consequence offers an opportunity to identify the presence or absence of VADs targets and mutations that may modify pathogen susceptibility to supplementary treatment.
While VADs possess no bactericidal activity there is emerging evidence that resistance mutations can occur, since VAD treatment may indirectly affect bacterial survival (44, 45). For example, in vitro resistance to QS inhibitor, furanone C-30, arose rapidly in clinical strains of P. aeruginosa due to silencing mutations in the mexR gene (44). This gene is a negative regulator of efflux pumps and is involved in the export of the C12-HSL autoinducer protein. Furthermore, as a number of significant VF are coded by genes located on plasmids and prophages, gene loss and gene acquisitions occur frequently. Indeed, plasmid flux occurs quickly, both independent of and much faster than the slow accumulation of single nucleotide polymorphisms (SNPs) (46). While not documented thus far, the risks of strain replacement following VAD administration requires consideration and laboratories should test for resistance after repeated VAD exposure.
Effects of mutations or sequence variations in VF coding regions require more nuanced methods of assessment, both computational and phenotypic. Within-host pathogen heterogeneity is problematic, since gene sequence diversity among bacterial meta-populations can hide target protein conformational changes in minority populations; as demonstrated for common pathogens Salmonella typhimurium and Streptococcus pneumoniae (47, 48). Sequencing of different S. typhimurium sequence types (ST19, ST34, and ST36) revealed a pool of 152 virulence genes and 79 virulence signatures (49), and examination of VAD targets suggest highly variable efficacy in different sequence types. Within species variability of VAD targets includes the absence of coding genes and/or variation in their sequences. For example, within species identity of lasI/lasR genes in Pseudomonas aeruginosa and comD gene in Streptococcus pneumoniae targeted by QS inhibitors can be, in some clinical strains, as low as 94.5 and 57.8%, respectively (Figure 2).
Sequencing of cancers has become a mainstay in oncology with a proliferation of advanced therapeutics targeting tumor-specific gene mutations. Personalized cancer care is enabled by the development of data analytics to assess the treatment implications associated with specific mutants and linking patients to optimal therapeutic approaches (29, 50–52). We are now in a position to extend the precision medicine paradigm to infectious diseases. Figure 3 outlines the proposed pathway for using genomic profiling for intelligent VAD selection and precision infectious diseases (ID) medicine. We suggest four levels of significance for VF-associated genome structures: level 1—recognized contributor to the clinical severity or prognosis of an infectious disease and a VAD target; level 2—recognized contributor to the severity or prognosis of an infectious disease but no VAD available; level 3—genome variant of a recognized VF potentially modified by VAD; level 4—uncertain if VF contribute to the severity or prognosis of infectious disease.
The narrow spectrum of targeted VAD, the tolerability profiles of repurposed VADs, as well as their high cost, indicate that contemporary antimicrobial utilization models are inadequate. We need to move toward a highly-tailored approach to ensure clinical- and cost-effective use of these novel therapeutics. The standard approach using minimum inhibitory concentrations (MIC) as predictors of clinical response to antibiotics cannot be extrapolated to VAD use and MIC-based PK/PD targets are not relevant. Novel approaches are needed to match specific virulence properties of an individual pathogen to the optimal mix of treatment modalities available. The lack of methods to enable the most effective (including cost-effective) use of such tailored treatment limits clinical uptake. We argue that inferences about VAD susceptibility requires microbial genomics. Table 2 illustrates a hierarchy of genomic variants that can be relevant for such inferences, using automated high-quality genome assembly and annotation to accurately reconstruct the accessory genome (46). However, the presence/absence or expression variability of VAD targets may be insufficient for accurate in silico determination of susceptibility (44), and a synthesis of genomic, transcriptomic and bacterial phenome data may be required to optimize treatment recommendations. High-resolution genome sequence analysis can also illuminate within-host heterogeneity of bacterial populations. Such an approach would form an important nucleus of knowledge upon which we can assemble a much larger strategy for value-based use of advanced therapeutics. This approach will require bioinformaticians to work closely with pathologists and clinicians to identify the most therapeutic options.
Microbial genomics can stratify a pathogen's likely VAD response by comparing the sequenced genome to a reference genome with known susceptibility or resistance to different classes of VAD. It is important to ensure availability of these reference genomes to diagnostic laboratories for timely in silico selection of the most appropriate VAD. While several pipelines for genomics surveillance and drug resistance determination have been implemented in practice (30, 52, 53), genomic inferences focused on VAD susceptibility are underdeveloped (38). It does not come as a surprise that, given the high complexity and cost of advanced therapeutics, the establishment of appropriate national registries has been a condition of their approval by the FDA/EMA in other domains. Effective post-registration monitoring and the establishment of a VAD register is important to record the indications and outcomes of VAD administration, as well as relevant pathogen details. This register will integrate available data, including relevant host data to develop predictive analytics to guide VAD use.
“Similarity assessments” may identify patients who experienced a desirable health outcome following VAD treatment and displayed similar clinical characteristics (54). The establishment and curation of databases linking genotypes and phenotypes is essential to improve the consistency of variant interpretation and relevant data sharing may be considered the ethical and legal duty of microbiology laboratories (55, 56). Ideally pathogen genomic data should be integrated into laboratory information systems and electronic health records (EHR). EHR systems have not yet anticipated the complexity and variety of microbial genomic information that needs to be captured, interpreted and acted upon. As with human genomics, improved integration of pathogen genomics data with EHR systems should assist clinical decision making and secondary use of data for translational research. The absence of well-established nomenclatures presents another challenge for integration and standardization as terminology standards developed for human genomics have not been applied with vigor in the infectious disease domain (57, 58).
The accurate diagnosis of pathogens based on next generation sequencing have substantial potential to improve value-based precision ID medicine. The maturation of genome-scale analysis offers a new prism through which to explore individually targeted therapy. Such an approach will identify the most beneficial treatment for each pathogen based on its genomic profile. Emerging markets for advanced therapeutics are pushing the boundaries of health systems and service delivery models. Better methods to identify patients who are most likely to benefit from expensive novel antimicrobial agents such as VAD, should facilitate regulatory approval and improve clinical uptake. Such a new paradigm is essential for future therapy design, which may also include engineered microbes designed to treat immunological or metabolic disorders, or to inhibit specific pathogens, often with site-specific delivery. The development of in silico assessment models is timely as it coincides with the crucial early stages of VAD roll out, where optimal systems are required to optimize its clinical value and cost effectiveness. It will augment antimicrobial stewardship and pharmacovigilance for advanced therapeutics, provide crucial policy guidance to regulating agencies, assist hospitals to optimize the use of these expensive medicines and create market opportunities for biotech companies and microbiology laboratories.
The original contributions presented in the study are included in the article/supplementary material, further inquiries can be directed to the corresponding author/s.
VS and BM designed the manuscript. All co-authors contributed to writing the manuscript and production of figures.
This study was supported by the Prevention Research Support Program funded by the New South Wales Ministry of Health and the NHMRC Center for Research Excellence in Emerging Infectious Diseases (GNT1102962) and NHMRC Centre for Research Excellence in Tuberculosis (GNT1153493). The funders of this study had no role in the study design, data collection, data analysis and interpretation, or writing of the report. The corresponding author has final responsibility for the decision to submit for publication.
The authors declare that the research was conducted in the absence of any commercial or financial relationships that could be construed as a potential conflict of interest.
1. Leguia M, Vila-Sanjurjo A, Chain PSG, Maljkovic-Berry I, Jarman RG, Pollett S. Precision medicine and precision public health in the era of pathogen next generation sequencing. J Infect Dis. (2020) 21 (Suppl. 3):S289–S91. doi: 10.1093/infdis/jiz424
2. Faulkner E, Spinner DS, Ringo M, Carroll M. Are global health systems ready for transformative therapies? Value Health. (2019) 22:627–41. doi: 10.1016/j.jval.2019.04.1911
3. Kim J, Hu C, El Achkar CM, Black LE, Douville J, Larson A, et al. Patient-customized oligonucleotide therapy for a rare genetic disease. New Engl J Med. (2019) 381:1644–52. doi: 10.1056/NEJMoa1813279
4. Dickey SW, Cheung GYC, Otto M. Different drugs for bad bugs: antivirulence strategies in the age of antibiotic resistance. Nat Rev Drug Discov. (2017) 16:457–71. doi: 10.1038/nrd.2017.23
5. Allen RC, Popat R, Diggle SP, Brown SP. Targeting virulence: can we make evolution-proof drugs? Nat Rev Microbiol. (2014) 12:300–8. doi: 10.1038/nrmicro3232
6. Rasko DA, Sperandio V. Anti-virulence strategies to combat bacteria-mediated disease. Nature Rev Drug Discov. (2010) 9:117–30. doi: 10.1038/nrd3013
7. López EL, Contrini MM, Glatstein E, González Ayala S, Santoro R, Allende D, et al. Safety and pharmacokinetics of urtoxazumab, a humanized monoclonal antibody against Shiga-like toxin 2 in healthy adults and pediatric patients infected with Shiga-like toxin-producing Escherichia coli. Antimicrob Agents Chemother. (2010) 54:239–43. doi: 10.1128/AAC.00343-09
8. López-Igual R, Bernal-Bayard J, Rodríguez-Patón A, Ghigo JM, Mazel D. Engineered toxin-intein antimicrobials can selectively target and kill antibiotic-resistant bacteria in mixed populations. Nat Biotech. (2019) 37:755–60. doi: 10.1038/s41587-019-0105-3
9. Nguyen T, Kim T, Ta HM, Yeo WS, Choi J, Mizar P, et al. Targeting mannitol metabolism as an alternative antimicrobial strategy based on the structure-function study of mannitol-1-phosphate dehydrogenase in Staphylococcus aureus. mBio. (2019) 10:e02660–18. doi: 10.1128/mBio.02660-18
10. Yep A, McQuade T, Kirchhoff P, Larsen M, Mobley HL. Inhibitors of TonB function identified by a high-throughput screen for inhibitors of iron acquisition in uropathogenic Escherichia coli CFT073. mBio. (2014) 5:e01089–13. doi: 10.1128/mBio.01089-13
11. Yeo WS, Arya R, Kim KK, Jeong H, Cho KH, Bae T. The FDA-approved anti-cancer drugs, streptozotocin and floxuridine, reduce the virulence of Staphylococcus aureus. Sci Rep. (2018) 8:2521. doi: 10.1038/s41598-018-20617-5
12. Imdad S, Chaurasia AK, Kim KK. Identification and validation of an antivirulence agent targeting HlyU-regulated virulence in Vibrio vulnificus. Front Cell Infect Microbiol. (2018) 8:152. doi: 10.3389/fcimb.2018.00152
13. Wilcox MH, Gerding DN, Poxton IR, Kelly C, Nathan R, Birch T, et al. Bezlotoxumab for prevention of recurrent Clostridium difficile infection. N Engl J Med. (2017) 376:305–17. doi: 10.1056/NEJMoa1602615
14. Migone TS, Subramanian GM, Zhong J, Healey LM, Corey A, Devalaraja M, et al. Raxibacumab for the treatment of inhalational anthrax. New Engl J Med. (2009) 361:135–44. doi: 10.1056/NEJMoa0810603
15. Paluch E, Rewak-Soroczynska J, Jedrusik I, Mazurkiewicz E, Jermakow K. Prevention of biofilm formation by quorum quenching. Appl Microbiol Biotechnol. (2020) 104:1871–81. doi: 10.1007/s00253-020-10349-w
16. Garca-Reyes S, Soberón-Chávez G, Cocoti-Yanez M. The third quorum-sensing system of Pseudomonas aeruginosa: Pseudomonas quinolone signal and the enigmatic PqsE protein. J Med Microbiol. (2020) 69:25–34. doi: 10.1099/jmm.0.001116
17. Martinez OF, Cardoso MH, Ribeiro S, Franco OL. Recent advances in anti-virulence therapeutic strategies with a focus on dismantling bacterial membrane microdomains, toxin neutralization, quorum-sensing interference and biofilm inhibition. Front Cell Infect Microbiol. (2019) 9:74. doi: 10.3389/fcimb.2019.00074
18. Chbib C. Impact of the structure-activity relationship of AHL analogues on quorum sensing in Gram-negative bacteria. Biorg Med Chem. (2020) 28:115282. doi: 10.1016/j.bmc.2019.115282
19. François B, Luyt CE, Stover CK, Brubaker JO, Chastre J, Jafri HS. New strategies targeting virulence factors of Staphylococcus aureus and Pseudomonas aeruginosa. Semin Respir Crit Care Med. (2017) 38:346–358. doi: 10.1055/s-0037-1602715
20. Fasciano AC, Shaban L, Mecsas J. Promises and challenges of the Type Three Secretion System—Injectisome as an anti-virulence target. EcoSal Plus. (2019) 8:10. doi: 10.1128/ecosalplus.ESP-0032-2018
21. Kisiela DI, Avagyan H, Friend D, Jalan A, Gupta S, Interlandi G, et al. Inhibition and reversal of microbial attachment by an antibody with parasteric activity against the FimH adhesion of uropathogenic E. coli. PLoS Pathog. (2015) 11:e1004857. doi: 10.1371/journal.ppat.1004857
22. Mellini M, Di Muzio E, D'Angelo F, Baldelli V, Ferrillo S, Visca P, et al. In silico selection and experimental validation of FDA-approved drugs as anti-quorum sensing agents. Front Microbiol. (2019) 10:2355. doi: 10.3389/fmicb.2019.02355
23. Guo Z, Li X, Li J, Yang X, Zhou Y, Lu C, et al. Licoflavonol is an inhibitor of the type three secretion system of Salmonella enterica serovar Typhimurium. Biochem Biophys Res Commun. (2016) 477:998–4. doi: 10.1016/j.bbrc.2016.07.018
24. Freedman JC, Hendericks MR, McClane BA. The potential therapeutic agent mepacrine protects Caco-2 cells against Clostridium perfringens enterotoxin action. mSphere. (2017) 2:e00352–17. doi: 10.1128/mSphere.00352-17
25. Antoniani D, Rossi E, Rinaldo S, Bocci P, Lolicato M, Paiardini A, et al. The immunosuppressive drug azathioprine inhibits biosynthesis of the bacterial signal molecule cyclic-di-GMP by interfering with intracellular nucleotide pool availability. Appl Microbiol Biotechnol. (2013) 97:7325–36. doi: 10.1007/s00253-013-4875-0
26. D'Angelo F, Baldelli V, Halliday N, Pantalone P, Polticelli F, Fiscarelli E, et al. Identification of FDA-approved drugs as antivirulence agents targeting the pqs quorum-sensing system in Pseudomonas aeruginosa. Antimicrob Agents Chemother. (2018) 62:e01296–18. doi: 10.1128/AAC.01296-18
27. Okada BK, Li A, Seyedsayamdost MR. Identification of the hypertension drug Guanfacine as an anti-virulence agent in Pseudomonas aeruginosa. Chembiochem. (2019) 20:2005–11. doi: 10.1002/cbic.201900129
28. Wang J, Yazdani S, Han A, Schapira M. Structure-based view of the druggable genome. Drug Discovery Today. (2020) 25:561–7. doi: 10.1016/j.drudis.2020.02.006
29. Piñeiro-Yáñez E, Reboiro-Jato M, Gómez-López G, Perales-Patón J, Troulé K, Rodríguez JM et al. PanDrugs: A novel method to prioritize anticancer drug treatments according to individual genomic data. Genome Med. (2018) 10:41. doi: 10.1186/s13073-018-0546-1
30. CRyPTIC Consortium the 100,000 Genomes Project, Allix-Beguec C, Arandjelovic I, Beckert P, Bonnet M, Bradley P, et al. Prediction of susceptibility to first-line tuberculosis drugs by DNA sequencing. New Engl J Med. (2018) 379:1403–15. doi: 10.1056/NEJMoa1800474
31. Farhat MR, Sultana R, Iartchouk O, Bozeman S, Galagan J, Sisk P, et al. Genetic determinants of drug resistance in Mycobacterium tuberculosis and their diagnostic value. Am J Respir Crit Care Med. (2016) 194:621–30. doi: 10.1164/rccm.201510-2091OC
32. Li J, Tai C, Deng Z, Zhong W, He Y, Ou H-Y. VRprofile: gene-cluster-detection-based profiling of virulence and antibiotic resistance traits encoded within genome sequences of pathogenic bacteria. Brief Bioinform. (2018) 19:566–74. doi: 10.1093/bib/bbw141
33. Rentzsch R, Deneke C, Nitsche A, Renard BY. Predicting bacterial virulence factors—evaluation of machine learning and negative data strategies. Brief Bioinform. (2020) 21:1596–608. doi: 10.1093/bib/bbz076
34. Tonkin-Hill G, MacAlasdair N, Ruis C, Weimann A, Horesh G, Lees JA, et al. Producing polished prokaryotic pangenomes with the Panaroo pipeline. Genom Biol. (2020) 21:180. doi: 10.1186/s13059-020-02090-4
35. Yu X-Q, Robbie GJ, Wu Y, Esser MT, Jensen K, Schwartz HI, et al. Safety, tolerability, and pharmacokinetics of MEDI4893, an investigational, extended-half0life, anti-Staphylococcus aureus Alpha-Toxin human monoclonal antibody, in healthy adults. Antimicrob Agents Chemother. (2016) 61:e01020–6. doi: 10.1128/AAC.01020-16
36. Morrison C. Antibacterial antibodies gain traction. Nat Rev Drug Discovery. (2015) 14:737–38. doi: 10.1038/nrd4770
37. Wang Q, Chang C-S, Pennini M, Pelletier M, Rajan S, Zha J et al. Target-agnostic identification of functional monoclonal antibodies against Klebsiella pneumoniae multimeric MrkA fimbrial subunit. J Infect Dis. (2016) 213:1800–8. doi: 10.1093/infdis/jiw021
38. Chabane YN, Mlouka MB, Alexandre S, Nicol M, Marti S, Pestel-Caron M, et al. Virstatin inhibits biofilm formation and motility of Acinetobacter baumanii. BMC Microbiol. (2014) 14:62. doi: 10.1186/1471-2180-14-62
39. Ueda A, Attila C, Whiteley M, Wood TK. Uracil influences quorum sensing and biofilm formation in Pseudomonas aeruginosa and fluorouracil is an antagonist. Microb Biotechnol. (2009) 2:62–74. doi: 10.1111/j.1751-7915.2008.00060.x
40. Pethe K, Alonso S, Biet F, Delogu G, Brennan MJ, Locht C, et al. The heparin-binding haemagglutinin of M. tuberculosis is required for extrapulmonary dissemination. Nature. (2001) 412:190–4. doi: 10.1038/35084083
41. Perez LJ, Karagounis TK, Hurley A, Bassler BL, Semmelhack MF. Highly potent, chemically stable quorum sensing agonists for Vibrio cholerae. Chem Sci. (2014) 5:151–5. doi: 10.1039/C3SC52572D
42. Laterre P-F, Colin G, Dequin P-F, Dugernier T, Boulain T, Azeredo da Silveira S, et al. CAL02, a novel antitoxin liposomal agent, in severe pneumococcal pneumonia: A first-in-human, double-blind, placebo-controlled, randomised trial. Lancet Infect Dis. (2019) 19:620–30. doi: 10.1016/S1473-3099(18)30805-3
43. Bitzan M, Poole R, Mehran M, Sicard E, Brockus C, Thuning-Roberson C, et al. Safety and pharmacokinetics of chimeric anti-Shiga toxin 1 and anti-Shiga toxin 2 monoclonal antibodies in healthy volunteers. Antimicrob Agents Chemother. (2009) 53:3081–7. doi: 10.1128/AAC.01661-08
44. Maeda T, Garcia-Contreras R, Pu M, Sheng L, Garcia LR, Tomás M, et al. Quorum quenching quandary: resistance to antivirulence compounds. ISME J. (2012) 6:493–501. doi: 10.1038/ismej.2011.122
45. Imperi F, Fiscarelli EV, Visaggio D, Leoni L, Visca P. Activity and impact on resistance development of two antivirulence fluoropyrimidine drugs in Pseudomonas aeruginosa. Front Cell Infect Microbiol. (2019). 9:49. doi: 10.3389/fcimb.2019.00049
46. Achtman M, Zhou Z. Distinct genealogies for plasmids and chromosome. PLoS Genet. (2014) 10:e1004874. doi: 10.1371/journal.pgen.1004874
47. Fu S, Hiley L, Octavia S, Tanaka MM, Sintchenko V, Lan R. Comparative genomics of Australian and international isolates of Salmonella Typhimurium: Correlation of core genome evolution with CRISPR and prophage profiles. Sci Rep. (2017) 7:9733. doi: 10.1038/s41598-017-06079-1
48. Rockett RJ, Oftadeh S, Bachmann NL, Timms V, Kong F, Gilbert GL, et al. Genome-wide analysis of Streptococcus pneumoniae serogroup 19 in the decade after the introduction of pneumococcal vaccines in Australia. Sci Rep. (2018) 8:16969. doi: 10.1038/s41598-018-35270-1
49. Rockett RJ, Arnott A, Wang Q, Howard P, Sintchenko V. Genomic surveillance enables suitability assessment of Salmonella gene targets used for culture-independent diagnostic testing. J Clin Microbiol. (2020) 58:e00038–20. doi: 10.1128/JCM.00038-20
50. Zeng J, Johnson A, Shufean MA, Kahle M, Yang D, Woodman SE, et al. Operationalization of next-generation sequencing and decision support for precision oncology. JCO Clin Cancer Inform. (2019) 3:1–2. doi: 10.1200/CCI.19.00089
51. Yu Y, Wang Y, Xia Z, Zhang X, Jin K, Yang J, et al. PreMedKB: an integrated precision medicine knowledgebase for interpreting relationships between diseases, genes, variants and drugs. Nucl Acids Res. (2019) 47:D1090–101. doi: 10.1093/nar/gky1042
52. Liu B, Zheng D, Jin Q, Chen L, Yang J. VFDB 2019: A comparative pathogenomic platform with an interactive web interface. Nucl Acids Res. (2019) 47:D687–92. doi: 10.1093/nar/gky1080
53. Ezewudo M, Borens A, Chiner-Oms A, Miotto P, Chindelevitch L, Starks AM, et al. Integrating standardized whole genome sequence analysis with a global Mycobacterium tuberculosis antibiotic resistance knowledgebase. Sci Rep. (2018) 8:15382. doi: 10.1038/s41598-018-33731-1
54. Chen Y, Guzauskas GF, Gu C, Wang BCM, Furnback WE, Xie G, et al. Precision health economics and outcomes research to support precision medicine: Big data meets patient heterogeneity on the road to value. J Pers Med. (2016) 6:20. doi: 10.3390/jpm6040020
55. Thorogood A, Cook-Deegan R, Knoppers BM. Public variant databases: Liability? Genet Med. (2017) 19:838–841. doi: 10.1038/gim.2016.189
56. Johnson SB, Parker M. The ethics of sequencing infectious disease pathogens for clinical and public health. Nat Rev Genet. (2019) 20:313–5. doi: 10.1038/s41576-019-0109-3
57. Gwinn M, MacCannell D, Armstrong GL. Next-generation sequencing of infectious pathogens. JAMA. (2019) 321:893–4. doi: 10.1001/jama.2018.21669
Keywords: virulence, genome sequence analysis, antibacterial treatment, surveillance, antimicrobial stewardship
Citation: Sintchenko V, Timms V, Sim E, Rockett R, Bachmann N, O'Sullivan M and Marais B (2021) Microbial Genomics as a Catalyst for Targeted Antivirulence Therapeutics. Front. Med. 8:641260. doi: 10.3389/fmed.2021.641260
Received: 13 December 2020; Accepted: 17 March 2021;
Published: 13 April 2021.
Edited by:
Leonardo Neves de Andrade, University of São Paulo, BrazilReviewed by:
Oana Sandulescu, Carol Davila University of Medicine and Pharmacy, RomaniaCopyright © 2021 Sintchenko, Timms, Sim, Rockett, Bachmann, O'Sullivan and Marais. This is an open-access article distributed under the terms of the Creative Commons Attribution License (CC BY). The use, distribution or reproduction in other forums is permitted, provided the original author(s) and the copyright owner(s) are credited and that the original publication in this journal is cited, in accordance with accepted academic practice. No use, distribution or reproduction is permitted which does not comply with these terms.
*Correspondence: Vitali Sintchenko, dml0YWxpLnNpbnRjaGVua29Ac3lkbmV5LmVkdS5hdQ==
Disclaimer: All claims expressed in this article are solely those of the authors and do not necessarily represent those of their affiliated organizations, or those of the publisher, the editors and the reviewers. Any product that may be evaluated in this article or claim that may be made by its manufacturer is not guaranteed or endorsed by the publisher.
Research integrity at Frontiers
Learn more about the work of our research integrity team to safeguard the quality of each article we publish.