- 1Department of Orthopedics & Spine Surgery, The First Affiliated Hospital of Anhui Medical University, Hefei, China
- 2Department of Respiratory and Critical Care Medicine, The First Affiliated Hospital of Anhui Medical University, Hefei, China
- 3School of Life Sciences, Anhui Medical University, Hefei, China
Background and Objective: Adolescent idiopathic scoliosis (AIS) is a complex three-dimensional deformity of the spine. Mesenchymal stem cells (MSCs) regulate bone mass homeostasis in AIS, which might be related to the pathogenesis of AIS. However, the mRNA–miRNA–lncRNA network linked to the regulation of the genetic pathogenesis of MSCs remains unknown.
Methods: We conducted an exhaustive literature search of PubMed, EMBASE, and the Gene Expression Omnibus database to find differentially expressed genes (DEGs), differentially expressed miRNAs (DE miRNAs), and differentially expressed lncRNAs (DE lncRNAs). Functional enrichment analysis was performed through Enrichr database. Protein–protein interaction (PPI) network was constructed using STRING database, and hub genes were identified by CytoHubba. Potential regulatory miRNAs and lncRNAs of mRNAs were predicted by miRTarBase and RNA22, respectively.
Results: We identified 551 upregulated and 476 downregulated genes, 42 upregulated and 12 downregulated miRNAs, and 345 upregulated and 313 downregulated lncRNAs as DEGs, DE miRNAs, and DE lncRNAs, respectively. Functional enrichment analysis revealed that they were significantly enriched in protein deglutamylation and regulation of endoplasmic reticulum unfolded protein response. According to node degree, one upregulated hub gene and eight downregulated hub genes were identified. After drawing the Venn diagrams and matching to Cytoscape, an mRNA–miRNA–lncRNA network linked to the pathogenesis of MSCs in AIS was constructed.
Conclusion: We established a novel triple regulatory network of mRNA–miRNA–lncRNA ceRNA, among which all RNAs may be utilized as the pathogenesis biomarker of MSCs in AIS.
Introduction
Adolescent idiopathic scoliosis (AIS) is characterized by a complex 3D deformity of the spine, but its etiology and pathogenesis remain unclear (1–3). Abnormal skeletal growth and low bone mass are factors that may be associated with disturbed bone metabolism existing in patients with AIS (4–6). Recent studies have suggested that mesenchymal stem cells (MSCs) play a crucial role in bone mass homeostasis in AIS; however, the regulation of the genetic pathogenesis of MSCs remains yet to be elucidated (7, 8).
MicroRNAs (miRNAs) are a group of endogenous small single-stranded non-coding RNAs with a length of ~21–25 nucleotides (9). miRNAs can negatively regulate gene expression by binding to the 3′-untranslated region of messenger RNA (mRNA), leading to direct mRNA degradation or protein translation inhibition. Thus, miRNAs are involved in the regulation of many biological processes, such as proliferation, apoptosis, cell cycle, differentiation, and DNA repair (10). Competing endogenous RNA (ceRNA) hypothesis indicates that cross-talk between non-coding RNA (ncRNA) and mRNA is achieved by competitively binding to shared miRNAs (11, 12). Long non-coding RNAs (lncRNAs) are a class of ncRNA, which can decrease miRNA abundance as miRNA sponges and increase the expression of downstream target mRNAs of miRNAs (13, 14). Increasing evidence indicates that miRNAs and lncRNAs have an effect on the osteoblastic differentiation of MSCs (15–17). However, current knowledge of mRNA–miRNA–lncRNA in MSCs of AIS is limited. The aim of the present study is to explore and construct a key mRNA–miRNA–lncRNA ceRNA triple subnetwork in AIS based on comprehensive bioinformatics analysis.
In this study, we acquired differentially expressed genes (DEGs), differentially expressed miRNAs (DE miRNAs), and differentially expressed lncRNAs (DE lncRNAs) by mining previously published datasets and studies. The DEGs were analyzed by gene ontology (GO) annotation and Kyoto Encyclopedia of Genes and Genomes (KEGG) pathway enrichment analysis. Then, a protein–protein interaction (PPI) network was established to identify hub genes, and potential regulatory miRNAs were predicted. The key DE miRNAs were identified by drawing Venn diagrams, and the functions of the key DE miRNAs were also determined. Subsequently, potential regulatory lncRNAs were predicted, and the key DE lncRNAs were identified using Venn diagrams. A novel mRNA–miRNA–lncRNA regulatory network associated with the pathogenesis of patients with AIS was successfully established.
Materials and Methods
Search Literature and Microarray Assay
We conducted an exhaustive literature search of PubMed, EMBASE, and the Gene Expression Omnibus database (http://www.ncbi.nlm.nih.gov/geo/) for studies that compared the gene or miRNA or lncRNA expression in bone marrow MSCs from patients with AIS and those from healthy individuals. Studies were selected and excluded based on the following criteria: (1) The study population consisted of patients with AIS who underwent a comprehensive clinical and radiological examination to rule out other causes of scoliosis and to determine the diagnosis of AIS. (2) In the non-AIS patients' group, each of the subjects had a straight spine and normal forward bending test on physical examination, determined not to have any associated medical conditions or spinal deformity. (3) Bone marrow aspirates were obtained from both groups. (4) Studies containing more than five AIS samples and five normal samples were included, whereas studies based on animal models were excluded. The titles and abstracts of these studies were screened, and information on the studies of interest was further evaluated. The search terms included “adolescent idiopathic scoliosis,” “AIS,” “mesenchymal stem cells,” and “MSCs.” We did not limit the languages or publication date.
Differential Expression Analysis
DEGs and DE miRNAs were obtained through literature search of previous studies (8, 18). Gene reannotation was performed, and “Limma” R package was used for differential expression analysis of lncRNAs. |FoldChange| > 1 and p < 0.05 were set as the cut-off criteria.
Functional Analysis
The GO and KEGG pathways of DEGs were analyzed based on the Enrichr database (http://amp.pharm.mssm.edu/Enrichr/) by performing functional annotation (biological process, BP, molecular function, MF, and cellular component, CC) and pathway enrichment analysis. p < 0.05 was considered statistically significant (19, 20). Then, the top 10 enriched GO terms and KEGG pathways were downloaded from the webpage. The functions of key DE miRNAs were analyzed through TAM 2.0 tools (21, 22) by using the hypergeometric test to evaluate the overexpression of each miRNA set (257 sets) in the “miRNA list.”
PPI Network
The potential PPI relationship of DEGs was mapped to the Search Tool for the Retrieval of Interacting Genes (STRING) database (http://string-db.org/), which is designed to analyze PPI information (23). PPI node pairs with a medium combined score ≥0.4 were selected for further analysis. The PPI network was then visualized using the Cytoscape software (version 3.7.2, www.cytoscape.org/). Nodes with a high degree of connectivity are essential in maintaining the stability of the entire network (24). CytoHubba is a plugin in Cytoscape that calculates the degree of each protein node. In our study, the top 30 hub genes were visualized in Cytoscape, and the top 10 hub genes were chosen for subsequent analysis.
Prediction and Validation of miRNA and Construction of the mRNA–miRNA Network
The miRTarbase database, which collects experimentally validated microRNA-target interactions by a reporter assay, Western blot analysis, qPCR, microarray, and next-generation sequencing experiments, was used to predict the potential miRNAs binding to hub genes (25). Then, the common DE miRNAs between predicted and previous DE miRNAs were identified as the key DE miRNAs by drawing Venn diagrams. The network of key genes and miRNA was constructed through the Cytoscape software.
Prediction and Validation of lncRNA and Construction of the mRNA–miRNA–lncRNA Network
The RNA22 tool was used to predict the potential lncRNAs binding to previously identified commonly DE miRNAs (26). Then, the commonly DE lncRNAs between predicted and previously DE lncRNAs were identified as the key DE lncRNAs by drawing Venn diagrams. The ceRNA network was constructed through the Cytoscape software.
Results
Study Search and Identification of DEGs, DE miRNAs, and DE lncRNAs
A summary of the study selection process is shown in Figure 1A. A total of 102 relevant studies were inspected via electronic search. A total of 27 duplicate studies were excluded. After the titles and abstracts were assessed, 51 studies that did not meet the eligibility criteria were eliminated. After the full text of the remaining 24 studies was verified, only three studies were included (8, 17, 18). The gene dataset was performed on the Affymetrix Gene Chip Human Transcript 2.0 arrays containing ten human AIS samples and five non-AIS samples and as did the miRNA dataset. The lncRNA dataset GSE110359 was also analyzed on the Affymetrix Gene Chip Human Transcript 2.0 arrays containing 12 human AIS samples and five non-AIS samples. Gene, miRNA, lncRNA datasets of the AIS group and the control group were from three different cohorts.
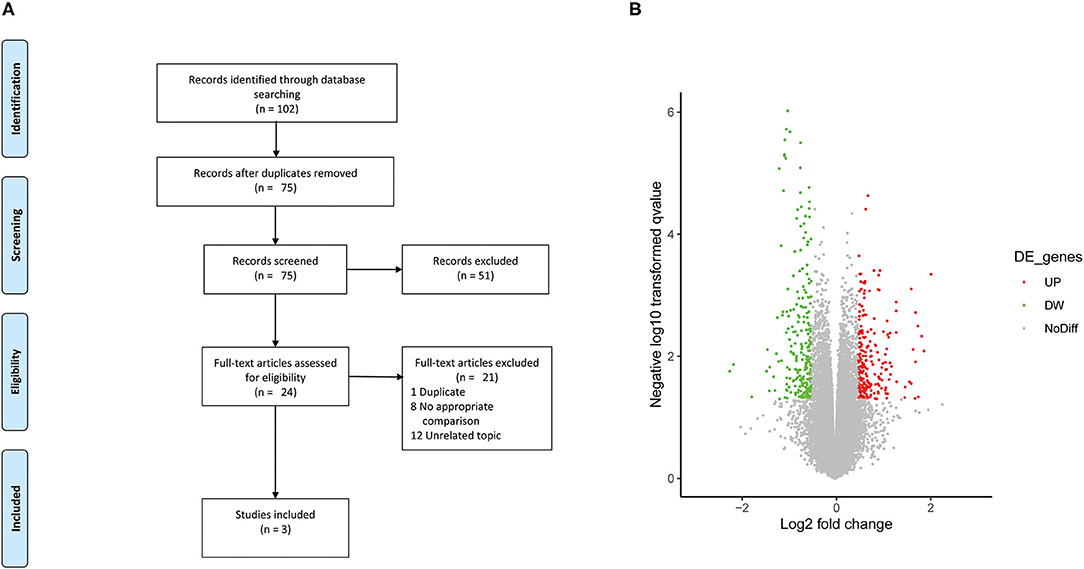
Figure 1. (A) Flow diagram of study selection. (B) Volcano plot of the DE lncRNAs. The black dots represent lncRNAs that are not differentially expressed, and the red dots and green dots represent the upregulated and downregulated lncRNAs, respectively.
A total of 1,027 genes (551 upregulated and 476 downregulated genes) and 54 miRNAs (42 upregulated and 12 downregulated miRNAs) were extracted from the included studies (8, 18) and identified as DEGs and DE miRNAs. Based on differential expression analysis, 658 lncRNAs (345 upregulated and 313 downregulated lncRNAs) were identified as DE lncRNAs, and the volcano plots of these DE lncRNAs is shown in Figure 1B.
GO and KEGG Analyses of DEGs
As shown in Figures 2A1–A3, the upregulated genes between AIS and normal samples are significantly enriched in protein deglutamylation, negative regulation of mitotic cell cycle, and positive regulation of nuclear-transcribed mRNA poly (A) tail shortening in the BP category, cullin-RING ubiquitin ligase complex, SWI/SNF complex, and mitotic spindle in the CC category and in transcription coactivator activity, syntaxin binding, and metallocarboxypeptidase activity in the MF category. KEGG enrichment analysis for these DEGs revealed that the thyroid hormone signaling pathway, p53 signaling pathway, and pancreatic cancer are significantly enriched pathways (Figure 3A).
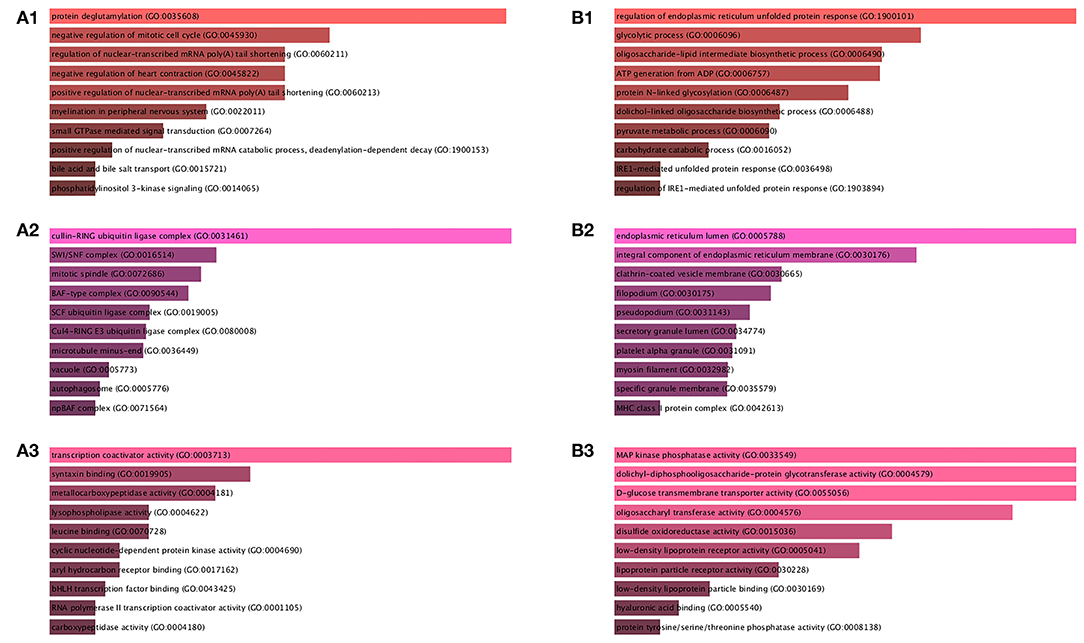
Figure 2. GO functional annotation for the significant DEGs. (A1) The top 10 enriched BP of the upregulated significant DEGs. (A2) The top 10 enriched CC of the upregulated significant DEGs. (A3) The top 10 enriched MF of the upregulated significant DEGs. (B1) The top 10 enriched BP of the downregulated significant DEGs. (B2) The top 10 enriched CC of the downregulated significant DEGs. (B3) The top 10 enriched MF of the downregulated significant DEGs. Ranking by combined score. Red color indicates p < 0.05 in BP; Pink color indicates p < 0.05 in CC; Jacinth color indicates p < 0.05 in MF.

Figure 3. KEGG pathway enrichment analysis for the significant DEGs. (A) Upregulated significant DEGs. (B) Downregulated significant DEGs. Ranking by combined score. Blue color indicates p < 0.05; Gray color indicates p ≥ 0.05.
As shown in Figures 2B1–B3, downregulated genes between AIS and normal samples were significantly enriched in regulation of endoplasmic reticulum unfolded protein response, glycolytic process, and oligosaccharide-lipid intermediate biosynthetic process in the BP category; endoplasmic reticulum lumen, integral component of endoplasmic reticulum membrane, and clathrin-coated vesicle membrane in the CC category; and MAP kinase phosphatase activity, dolichyl-diphosphooligosaccharide-protein glycotransferase activity, and D-glucose transmembrane transporter activity in the MF category. KEGG enrichment analysis for these DEGs revealed that N-glycan biosynthesis, antigen processing and presentation, and complement and coagulation cascades were significantly enriched pathways (Figure 3B).
PPI Network Construction and Hub Gene Identification
Protein interactions among the DEGs were predicted with STRING tools. A total of 403 nodes and 537 edges were involved in the PPI network (PPI enrichment p < 7.47e−08) of upregulated DEGs, as shown in Figure 4A. The top 30 upregulated genes are shown in Figure 4B and Table 1. A total of 437 nodes and 739 edges were involved in the PPI network (PPI enrichment p < 1.0e−16) of downregulated DEGs, as presented in Figure 4C, and the top 30 downregulated genes are shown in Figure 4D and Table 1. The top 10 upregulated hub genes included CREBBP, WDTC1, KAT2B, PRKACG, TP53BP1, UBXN7, SMARCC2, NCOA1, SMAD3, and NCOA2. The top ten downregulated hub genes included HSPA5, CYCS, PDIA3, KDR, PGK1, PDIA6, SERPINE1, PPIB, CKAP4, and TGOLN2. Those 20 hub genes were chosen for the following analyses.
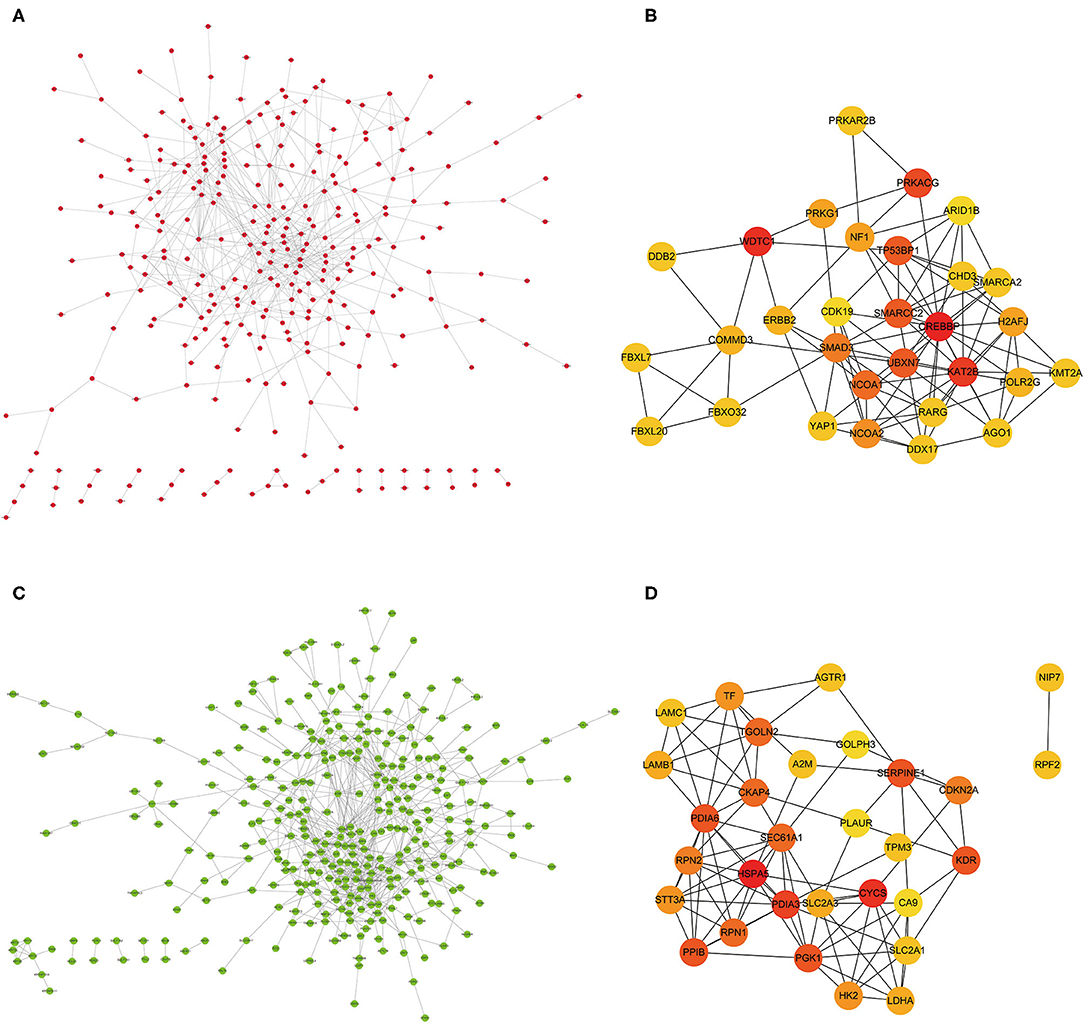
Figure 4. (A) PPI network of the significant upregulated DEGs. (B) The top 30 hub genes of the significant upregulated DEGs. (C) The PPI network of the significant downregulated DEGs. (D) The top 30 hub genes of the significant downregulated DEGs.
Prediction and Validation of Potential Key miRNAs of Key Genes
The potential miRNAs binding to the 20 key genes were predicted through the miRTarBase, and a total of 241 miRNAs were identified for upregulated DEGs. After Venn diagrams were constructed with DE miRNAs, one downregulated miRNA that could potentially regulate one key gene (WDTC1) expression was identified as presented in Figure 5A. Potential miRNAs binding to the nine key upregulated genes (CREBBP, KAT2B, PRKACG, TP53BP1, UBXN7, SMARCC2, NCOA1, SMAD3, and NCOA2) were not observed. For downregulated hub genes, a total of 439 miRNAs were identified. After the construction of Venn diagrams with DE miRNAs, we identified 18 upregulated miRNAs that could potentially regulate eight key gene (HSPA5, CYCS, KDR, PGK1, PDIA6, PPIB, CKAP4, and TGOLN2) expression as presented in Figure 5B. Potential miRNAs binding to two key downregulated genes (PDIA3 and SERPINE1) were not observed.
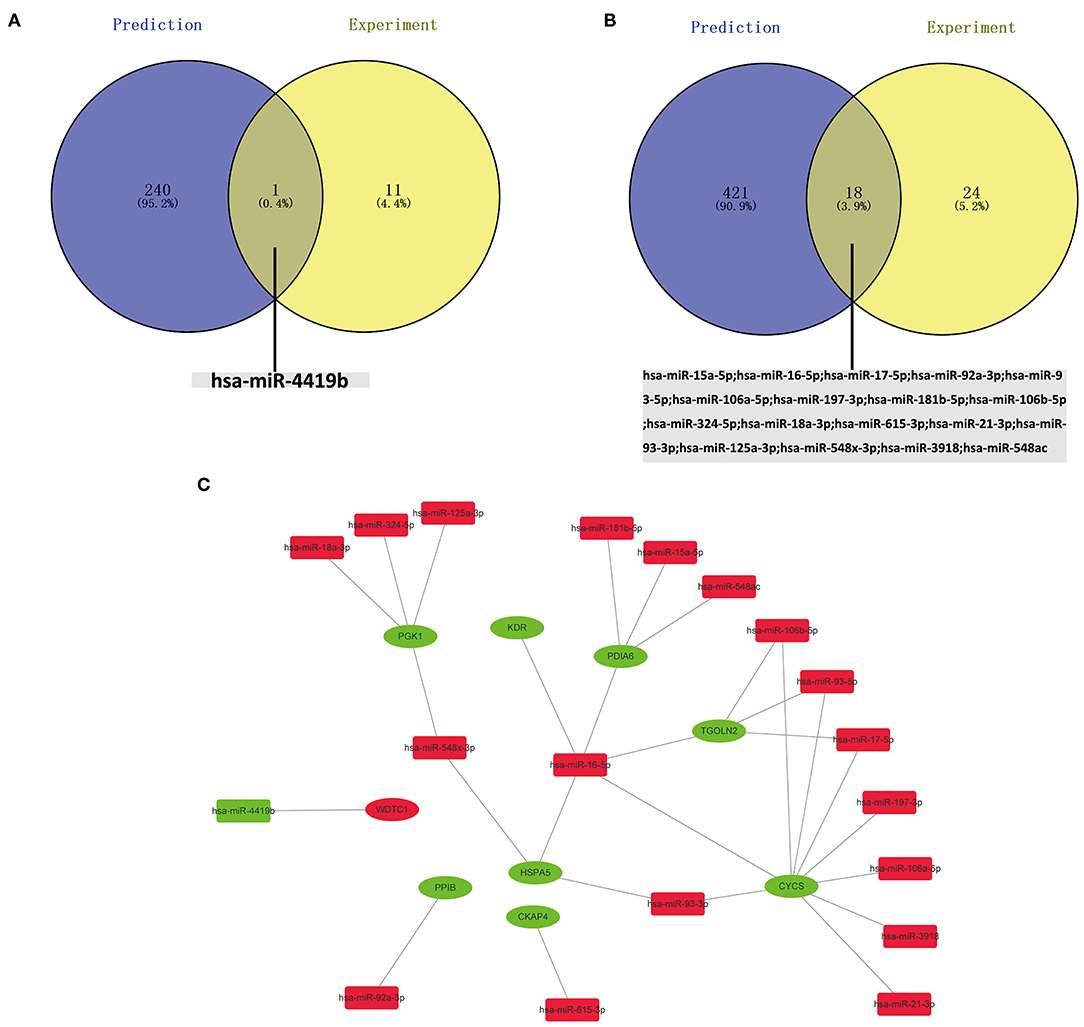
Figure 5. (A) Identification of key downregulated miRNAs by combining prediction and experiment analyses. (B) Identification of key unregulated miRNAs by combining prediction and experiment analyses. (C) Construction of the miRNA–gene network.
Construction of the Key mRNA–miRNA Network in AIS
Based on the classical inverse relationship between miRNA and target gene, the 18 potential upregulated miRNAs binding to eight downregulated genes (HSPA5, CYCS, KDR, PGK1, PDIA6, PPIB, CKAP4, and TGOLN2) were identified, and only one potential downregulated miRNA binding to an upregulated gene (WDTC1) was identified. The network (miR-93-3p/miR-16-5p/miR-548x-3p - HSPA5, miR-16-5p/miR-17-5p/miR-93-5p/miR-106a-5p/miR-197-3p/miR-106b-5p/miR-21-3p/miR-93-3p/miR-3918 - CYCS, miR-16-5p - KDR, miR-324-5p/miR-18a-3p/miR-125a-3p/miR-548x-3p - PGK1, miR-15a-5p/miR-16-5p/miR-181b-5p/miR-548ac - PDIA6, miR-92a-3p - PPIB, miR-615-3p - CKAP4, miR-16-5p/miR-17-5p/miR-93-5p/miR-106b-5p - TGOLN2, miR-4419b - WDTC1) of key mRNA and miRNA is shown in Figure 5C.
Functional Analysis of Key miRNAs
Functional enrichment analysis for these miRNAs revealed that aging, immune system, and immune response are significantly enriched pathways (Figure 6).
Prediction and Validation of Potential Key lncRNAs Binding to Key miRNAs
Given that no direct database can accommodate queries on the pairing relationship between lncRNAs and miRNAs in large quantities, RNA22 was used to predict the potential lncRNAs binding to the 19 key miRNAs, and a total of 6,293 lncRNAs were identified for downregulated miRNAs. After Venn diagrams with previous DE lncRNAs were constructed, 15 lncRNAs that could potentially regulate one key downregulated miRNA (miR-4419b) expression was identified as presented in Figure 7A. A total of 12,065 lncRNAs were identified for upregulated miRNAs. After Venn diagrams with previous DE lncRNAs were constructed, 41 lncRNAs that could potentially regulate the expression of 17 key miRNAs (miR-15a-5p, miR-16-5p, miR-17-5p, miR-92a-3p, miR-93-5p, miR-106a-5p, miR-197-3p, miR-181b-5p, miR-106b-5p, miR-324-5p, miR-18a-3p, miR-615-3p, miR-21-3p, miR-93-3p, miR-125a-3p, miR-3918, and miR-548x-3p) were identified as shown in Figure 7B. Potential lncRNAs binding to one key upregulated miRNA (miR-548ac) were not observed.
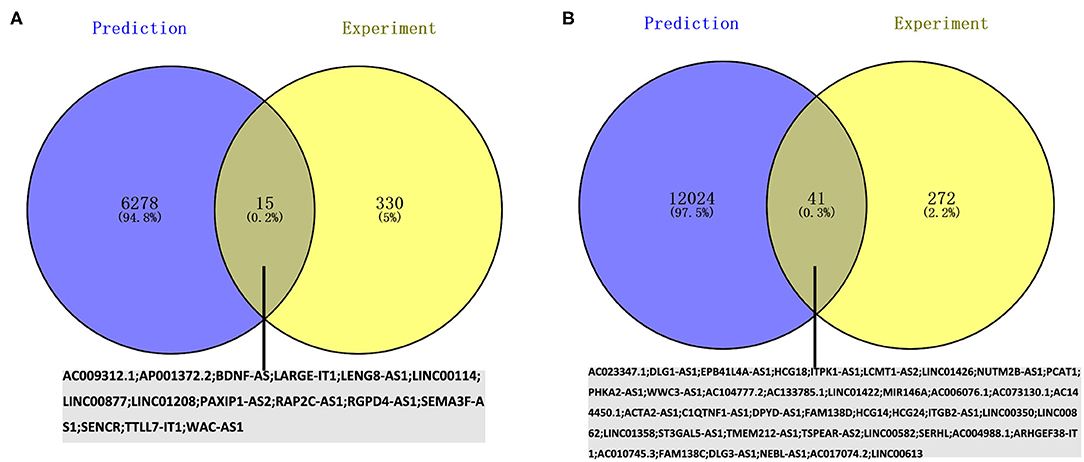
Figure 7. Screening the key lncRNAs in AIS. (A) Identification of key upregulated miRNAs by combining prediction and experiment analyses. (B) Identification of key downregulated miRNAs by combining prediction and experiment analyses.
Construction of Key mRNA–miRNA–lncRNA Triple Subnetwork in AIS
Based on the aforementioned analysis, an mRNA–miRNA–lncRNA network was constructed using Cytoscape. The triple subnetwork of upregulated and downregulated hub genes is shown in Figures 8, 9. The detailed information of subnetworks is listed in Supplementary Tables 1, 2. Each of the 18 miRNAs (miR-15a-5p, miR-16-5p, miR-17-5p, miR-92a-3p, miR-93-5p, miR-106a-5p, miR-197-3p, miR-181b-5p, miR-106b-5p, miR-324-5p, miR-18a-3p, miR-615-3p, miR-21-3p, miR-93-3p, miR-125a-3p, miR-3918, miR-548x-3p, and miR-4419b) was regulated by lncRNAs, which then regulated the target genes of miRNAs. The differential expression level of lncRNA RAP2C-AS1 was the highest in the network regulating miR-4419b, and other certain lncRNAs (HCG18 and TSPEAR-AS2) and miRNAs (miR-16-5p, miR-93-3p, and miR-93-5p) may play important roles in the ceRNA network as they regulate the majority of the downregulated hub genes. Therefore, the RAP2C-AS1 - miR-4419b - WDTC1, TSPEAR-AS2 - miR-16-5p - CYCS/KDR/PDIA6/TGOLN2/HSPA5, TSPEAR-AS2/HCG18 - miR-93-3p - CYCS/HSPA5, TSPEAR-AS2/HCG18 - miR-93-5p - CYCS/ TGOLN2, TSPEAR-AS2/HCG18 - miR-615-3p - CKAP4, and TSPEAR-AS2/HCG18 - miR-125a-3p - PGK1 axes may play critical roles in the network (Figure 10).
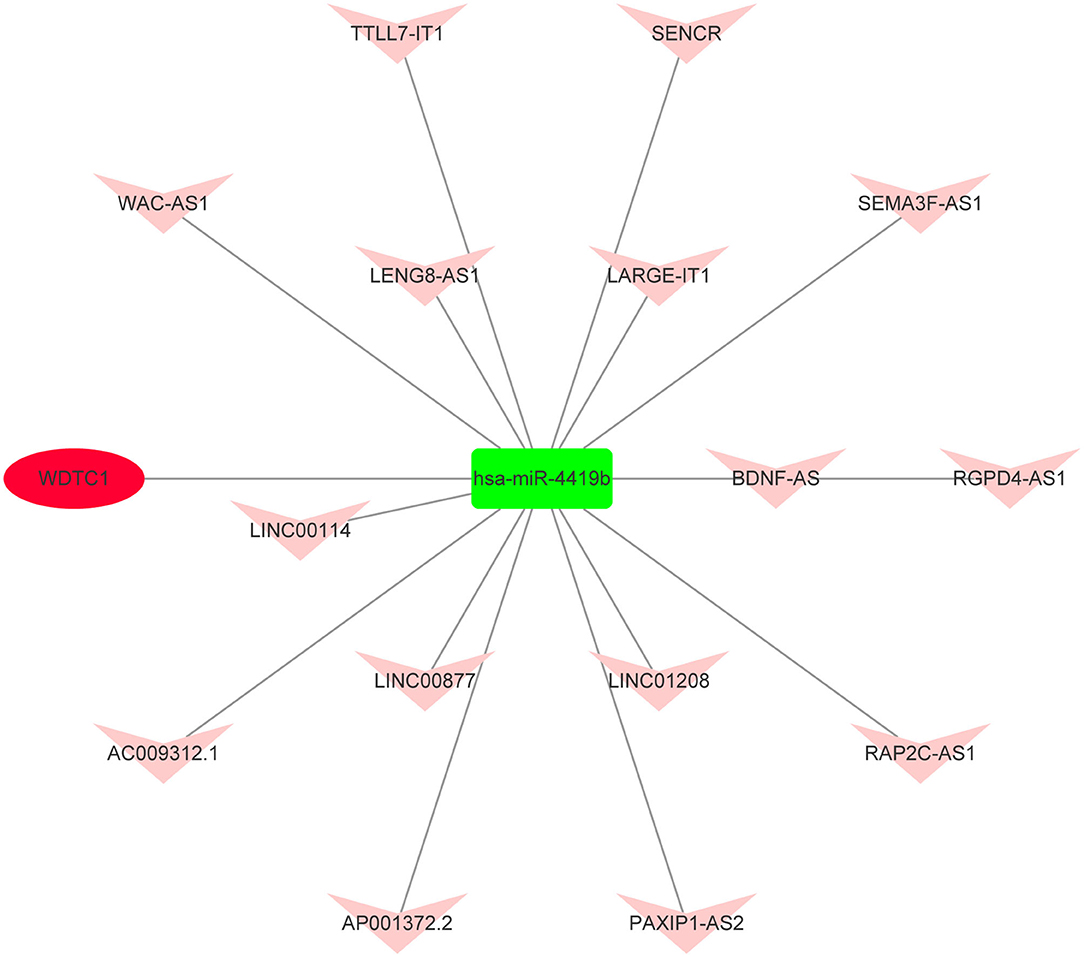
Figure 8. Novel upregulated key mRNA's competing endogenous RNA (ceRNA) triple regulatory network. The green rectangle in the network represents downregulated miRNAs. The red ellipse in the network represents upregulated hub genes. The red V in the network represents upregulated lncRNAs.
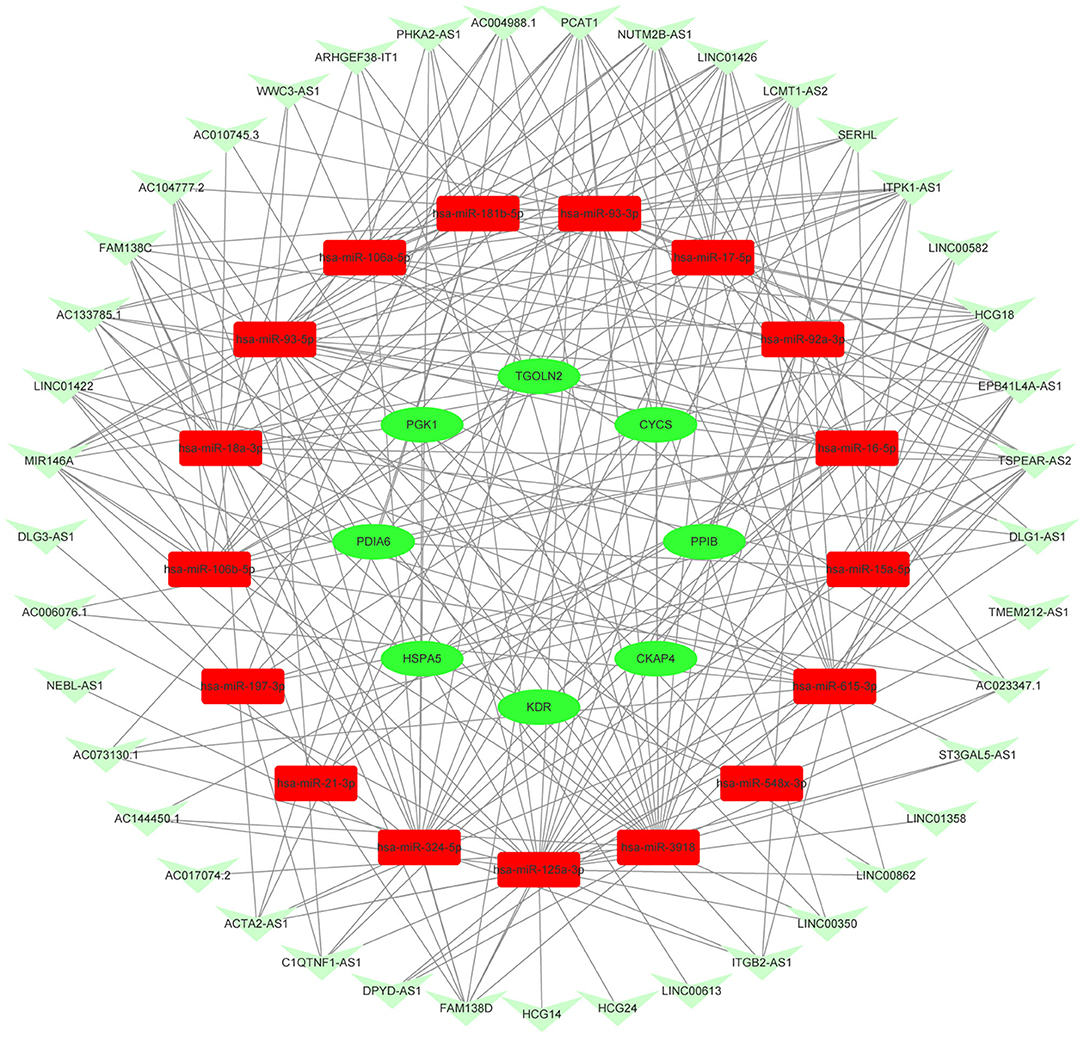
Figure 9. Novel downregulated key mRNAs' ceRNA triple regulatory network. The red rectangle in the network represents upregulated miRNAs. The green ellipse in the network represents downregulated hub genes. The green V in the network represents downregulated lncRNAs.
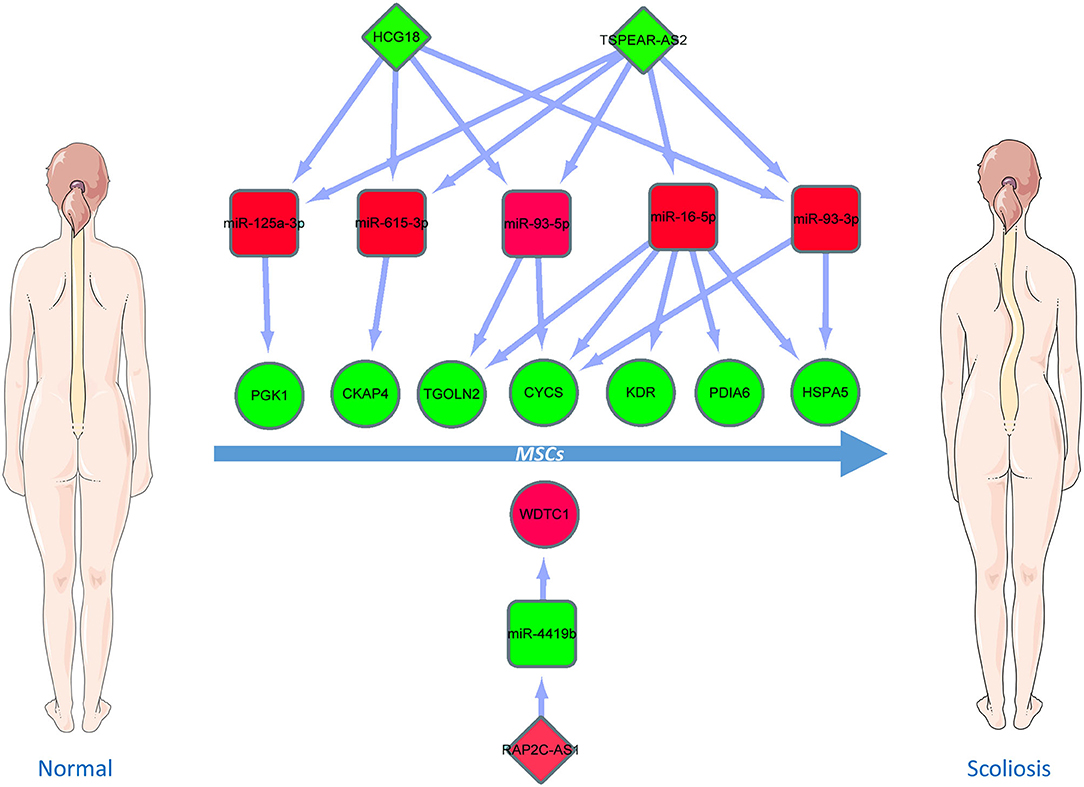
Figure 10. Critical ceRNA triple regulatory network. The rectangle in the network represents miRNAs. The ellipse in the network represents hub genes. The diamond in the network represents lncRNAs. Green represents downregulation, while red represents upregulation.
Discussion
This study was designed to investigate the specific ceRNA network based on the “mRNA–miRNA–lncRNA” order pattern. A total of 1,027 significant DEGs, consisting of 551 upregulated and 476 downregulated DEGs were extracted from the included studies. These genes are involved in multiple biological processes, including protein deglutamylation, small GTPase-mediated signal transduction, phosphatidylinositol 3-kinase signaling, RNA polymerase II transcription coactivator activity, MAP kinase phosphatase activity, negative regulation of the mitotic cell cycle, regulation of endoplasmic reticulum-unfolded protein response, glycolytic process, and positive regulation of the nuclear-transcribed mRNA poly (A) tail shortening. Although Zhuang et al. (8) used a different functional annotation database, they found that small GTPase-mediated signal transduction, phosphatidylinositol 3-kinase signaling, RNA polymerase II transcription coactivator activity, and MAP kinase phosphatase activity were enriched in GO analysis of DEGs. Elevated levels of the small GTPase Cdc42 could induce senescence in male rat MSCs (27). Domingues et al. (28) found that Cofilin-1 levels were elevated in human mesenchymal stem/stromal cells cultured on soft substrates, which could promote Cofilin-1-dependent increased RNA transcription and faster RNA polymerase II-mediated transcription elongation. It has been reported that miR-16 regulated MgCl2-induced promotion of osteogenic differentiation of MSCs by targeting FGF2-mediated activation of the ERK/MAPK pathway (29). Meanwhile, the pathway enrichment analysis of DEGs and key miRNAs revealed the presence of multiple enriched pathways, including the thyroid hormone signaling pathway, p53 signaling pathway, aging, regulation of Akt pathway, and apoptosis. Yoon et al. (30) have found that NRF2 could determine the self-renewal and osteogenic differentiation potential of human MSCs via the P53-SIRT1 axis. Other teams reported that PI3K/Akt signaling is the main contributor to MSC proliferation in response to PDGFRβ activation and Erk activation by PDGFRβ signaling, potently inhibiting the adipocytic differentiation of MSCs by blocking PPARγ and CEBPα expression (31). Through the activation of the AKT/glycogen synthase kinase signaling pathway and suppression of apoptosis, PBX homeobox 1 enhances hair follicle MSC proliferation and reprogramming. Thus, these significant DEGs may be involved in the molecular pathogenesis of AIS initiation and development.
To analyze the relationships and functions of significant DEGs, we obtained PPI networks from STRING database and identified one upregulated hub gene (WDTC1) and eight downregulated hub genes (HSPA5, CYCS, KDR, PGK1, PDIA6, PPIB, CKAP4, and TGOLN2) that may be related to the pathogenesis of AIS. Most of these key genes have been well-investigated in MSCs. For example, WDTC1 is a conserved and single-copy gene in humans (32) and studies have linked the function of WDTC1 to the negative regulation of adipogenesis (33, 34). Besides, Liang et al. have reported that the adipogenic ability of MSCs from AIS girls was lower than that of controls (35). HSPA5 encodes heat shock protein 70 (HSP70), which was involved in proliferation, differentiation, and apoptosis of MSCs (36, 37). CYCS positively regulates MSC apoptosis (16) and is involved in neuronal developmental disorders, amyotrophic lateral sclerosis, and neuron death (38). KDR, also known as VEGF receptor 2, is a critical regulator of MSC osteogenesis following the activation of the ERK/RUNX2 signaling pathway (39). PGK1 overexpression may induce osteoblastic differentiation of bone marrow stromal cells and inhibit osteoclastogenesis (40). These publications partially support the accuracy of potential core genes found in our bioinformatics analyses, while the function of these core genes in MSCs of AIS requires further research.
MiRNAs and lncRNAs are involved in regulating gene expression and function through the mechanism of ceRNA. Previous studies have shown that some miRNAs binding to the key genes that we screened were identical to our analytic results. For example, miR-17-5p and miR-106a upregulation could promote adipogenesis and inhibit osteogenesis by targeting BMP2 in the modulation of human adipose-derived MSCs (41). By targeting Smad5, miR-17-5p and miR-106b-5p could suppress the osteogenic differentiation of C2C12 cells and inhibit bone formation (42). miR-16-5p inhibits osteoclastogenesis in giant cell tumor of bone via the direct inhibition of receptor activator of nuclear factor-κB ligand- (RANKL-) (15). Downregulation of miRNA-16-5p could accelerate fracture healing by negatively regulating Bcl-2 and Cyclin-D1 expression in MC3T3-E1 cells (43). miR-93-5p suppresses the osteogenic differentiation of mouse MSCs (C3H10T1/2) by targeting Smad5 (44). In trauma-induced osteonecrosis of patients with femoral head fractures, increased miRNA-93-5p inhibits osteogenic differentiation by targeting bone morphogenetic protein-2 (45). Considering that the ceRNA hypothesis indicates that cross-talk between lncRNAs and mRNAs is achieved through competitive binding to shared miRNAs, 56 potential key lncRNAs binding to these key miRNAs were identified. However, studies on the role of lncRNA of AIS are few. Only the group of Zhuang has found a novel lncAIS (gene symbol: ENST00000453347) that could suppress osteogenic differentiation of MSCs in AIS (17). Upregulation of BDNF-AS promotes bone marrow-derived MSCs' self-proliferation but inhibits osteogenic differentiation through BDNF regulation (46); BDNF-AS expression was also upregulated in this study. A pathogenesis-associated mRNA–miRNA–lncRNA network in AIS was successfully established, and such a network has been identified in previous literature on osteogenic differentiation. For example, downregulated FAM83H-AS1 modulates the SpA-inhibited osteogenic differentiation in human bone MSCs by FAM83H-AS1/miR-541-3p/WNT3A axis (47). lncRNA MSC-AS1 could promote osteogenic differentiation of bone marrow-derived MSCs through sponging miRNA-140-5p to upregulate BMP2 (48). Collectively, although a series of bioinformatics analyses has yielded some intriguing findings in our current study, further laboratory experiments and large-scale clinical trials are needed in the future. Besides, the artificial selection of ten upregulated and downregulated genes may result in some risk of selection bias.
Conclusion
In summary, through integrated bioinformatics analysis and exhaustive literature search, we constructed a novel triple regulatory network of mRNA–miRNA-lncRNA ceRNA, in which all RNAs have significant predictive value for the pathogenesis of MSCs in AIS. We also identified that the RAP2C-AS1 - miR-4419b - WDTC1, TSPEAR-AS2 - miR-16-5p - CYCS/KDR/PDIA6/TGOLN2/HSPA5, TSPEAR-AS2/HCG18 - miR-93-3p - CYCS/HSPA5, TSPEAR-AS2/HCG18 - miR-93-5p - CYCS/ TGOLN2, TSPEAR-AS2/HCG18 - miR-615-3p – CKAP4 and TSPEAR-AS2/HCG18 - miR-125a-3p - PGK1 axes may play critical roles in the ceRNA network. In addition to the prognostic value of this mRNA–miRNA–lncRNA network for AIS, our findings provide important insights into the molecular mechanism of AIS. However, further studies are needed to validate these findings.
Data Availability Statement
Publicly available datasets were analyzed in this study. This data can be found here: lncRNA (GSE110359); gene and miRNA datasets come from the Supplementary Material in (8, 18).
Author Contributions
C-LS and X-YL designed the study. H-ML wrote the manuscript. YL and J-YD revised and polished the manuscript. H-ML, YL, J-YD, and RZ performed the statistical analysis of the data. All authors read and approved the final manuscript.
Conflict of Interest
The authors declare that the research was conducted in the absence of any commercial or financial relationships that could be construed as a potential conflict of interest.
Supplementary Material
The Supplementary Material for this article can be found online at: https://www.frontiersin.org/articles/10.3389/fmed.2020.583243/full#supplementary-material
Abbreviations
AIS, Adolescent idiopathic scoliosis; MSCs, mesenchymal stem cells; miRNA, microRNA; lncRNA, long non-coding RNA; ceRNA, competing endogenous RNA; GEO, Gene Expression Omnibus; DEGs, differentially expressed genes; GO, gene ontology; KEGG, Kyoto Encyclopedia of Genes and Genomes; PPI, protein-protein interaction; BP, biological process; MF, molecular function; CC, cellular component; STRING, Search Tool for the Retrieval of Interacting Genes.
References
1. Willner S. Prevalence study of trunk asymmetries and structural scoliosis in 10-year-old school children. Spine. (1984) 9:644–7. doi: 10.1097/00007632-198409000-00017
2. Weinstein SL, Dolan LA, Cheng JC, Danielsson A, Morcuende JA. Adolescent idiopathic scoliosis. Lancet. (2008) 371:1527–37. doi: 10.1016/S0140-6736(08)60658-3
3. Li X, Hung VWY, Yu FWP, Hung ALH, Ng BKW, Cheng JCY, et al. Persistent low-normal bone mineral density in adolescent idiopathic scoliosis with different curve severity: a longitudinal study from presentation to beyond skeletal maturity and peak bone mass. Bone. (2019) 133:115217. doi: 10.1016/j.bone.2019.115217
4. Barrios C, Cortes S, Perez-Encinas C, Escriva MD, Benet I, Burgos J, et al. Anthropometry and body composition profile of girls with nonsurgically treated adolescent idiopathic scoliosis. Spine. (2011) 36:1470–7. doi: 10.1097/BRS.0b013e3181f55083
5. Bao H, Liu Z, Yan P, Qiu Y, Zhu F. Disproportionate growth between the spine and pelvis in patients with thoracic adolescent scoliosis: a new look into the pattern's growth. Bone Joint J. (2015) 97-b:1668–74. doi: 10.1302/0301-620X.97B12.35874
6. Song XX, Jin LY, Li XF, Qian L, Shen HX, Liu ZD, et al. Effects of low bone mineral status on biomechanical characteristics in idiopathic scoliotic spinal deformity. World Neurosurg. (2018) 110:e321–9. doi: 10.1016/j.wneu.2017.10.177
7. Chen C, Xu C, Zhou T, Gao B, Zhou H, Chen C, et al. Abnormal osteogenic and chondrogenic differentiation of human mesenchymal stem cells from patients with adolescent idiopathic scoliosis in response to melatonin. Mol Med Rep. (2016) 14:1201–9. doi: 10.3892/mmr.2016.5384
8. Zhuang Q, Mao W, Xu P, Li H, Sun Z, Li S, et al. Identification of differential genes expression profiles and pathways of bone marrow mesenchymal stem cells of adolescent idiopathic scoliosis patients by microarray and integrated gene network analysis. Spine. (2016) 41:840–55. doi: 10.1097/BRS.0000000000001394
9. Bartel DP. MicroRNAs: target recognition and regulatory functions. Cell. (2009) 136:215–33. doi: 10.1016/j.cell.2009.01.002
10. Shukla GC, Singh J, Barik S. MicroRNAs: processing, maturation, target recognition and regulatory functions. Mol Cell Pharmacol. (2011) 3:83–92. doi: 10.1117/12.2059601
11. Salmena L, Poliseno L, Tay Y, Kats L, Pandolfi PP. A ceRNA hypothesis: the rosetta stone of a hidden RNA language? Cell. (2011) 146:353–8. doi: 10.1016/j.cell.2011.07.014
12. Karreth FA, Pandolfi PP. ceRNA cross-talk in cancer: when ce-bling rivalries go awry. Cancer Discov. (2013) 3:1113–21. doi: 10.1158/2159-8290.CD-13-0202
13. Guttman M, Amit I, Garber M, French C, Lin MF, Feldser D, et al. Chromatin signature reveals over a thousand highly conserved large non-coding RNAs in mammals. Nature. (2009) 458:223–7. doi: 10.1038/nature07672
14. Zhang J, Li W. Long noncoding RNA CYTOR sponges miR-195 to modulate proliferation, migration, invasion and radiosensitivity in nonsmall cell lung cancer cells. Biosci Rep. (2018) 38:BSR20181599. doi: 10.1042/BSR20181599
15. Sang S, Zhang Z, Qin S, Li C, Dong Y. MicroRNA-16-5p inhibits osteoclastogenesis in giant cell tumor of bone. Biomed Res Int. (2017) 2017:3173547. doi: 10.1155/2017/3173547
16. Li L, Sun Y, Zhang N, Qiu X, Wang L, Luo Q. By regulating miR-182-5p/BCL10/CYCS, sufentanil reduces the apoptosis of umbilical cord mesenchymal stem cells caused by ropivacaine. Biosci Trends. (2019) 13:49–57. doi: 10.5582/bst.2018.01291
17. Zhuang Q, Ye B, Hui S, Du Y, Zhao RC, Li J, et al. Long noncoding RNA lncAIS downregulation in mesenchymal stem cells is implicated in the pathogenesis of adolescent idiopathic scoliosis. Cell Death Differ. (2019) 26:1700–15. doi: 10.1038/s41418-018-0240-2
18. Hui S, Yang Y, Li J, Li N, Xu P, Li H, et al. Differential miRNAs profile and bioinformatics analyses in bone marrow mesenchymal stem cells from adolescent idiopathic scoliosis patients. Spine J. (2019) 19:1584–96. doi: 10.1016/j.spinee.2019.05.003
19. Chen EY, Tan CM, Kou Y, Duan Q, Ayan AM. Enrichr: interactive and collaborative HTML5 gene list enrichment analysis tool. BMC Bioinformatics. (2013) 14:128. doi: 10.1186/1471-2105-14-128
20. Kuleshov MV, Jones MR, Rouillard AD, Fernandez NF, Duan Q, Wang Z, et al. Enrichr: a comprehensive gene set enrichment analysis web server 2016 update. Nucleic Acids Res. (2016) 44:W90–7. doi: 10.1093/nar/gkw377
21. Li J, Han X, Wan Y, Zhang S, Zhao Y, Fan R, et al. TAM 2.0: tool for MicroRNA set analysis. Nucleic Acids Res. (2018) 46:W180–5. doi: 10.1093/nar/gky509
22. Mora A. Gene set analysis methods for the functional interpretation of non-mRNA data-genomic range and ncRNA data. Brief Bioinform. (2019) 21:1495–508. doi: 10.1093/bib/bbz090
23. Szklarczyk D, Morris JH, Cook H, Kuhn M, Wyder S, Simonovic M, et al. The STRING database in 2017: quality-controlled protein-protein association networks, made broadly accessible. Nucleic Acids Res. (2017) 45:D362–8. doi: 10.1093/nar/gkw937
24. Shannon P, Markiel A, Ozier O, Baliga NS, Wang JT, Ramage D, et al. Cytoscape: a software environment for integrated models of biomolecular interaction networks. Genome Res. (2003) 13:2498–504. doi: 10.1101/gr.1239303
25. Chou CH, Shrestha S, Yang CD, Chang NW, Lin YL, Liao KW, et al. miRTarBase update 2018: a resource for experimentally validated microRNA-target interactions. Nucleic Acids Res. (2018) 46:D296–302. doi: 10.1093/nar/gkx1067
26. Miranda KC, Huynh T, Tay Y, Ang YS, Tam WL, Thomson AM, et al. A pattern-based method for the identification of MicroRNA binding sites and their corresponding heteroduplexes. Cell. (2006) 126:1203–17. doi: 10.1016/j.cell.2006.07.031
27. Umbayev B, Masoud AR, Tsoy A, Alimbetov D, Olzhayev F, Shramko A, et al. Elevated levels of the small GTPase Cdc42 induces senescence in male rat mesenchymal stem cells. Biogerontology. (2018) 19:287–301. doi: 10.1007/s10522-018-9757-5
28. Domingues C, Geraldo AM, Anjo SI, Matos A, Almeida C, Caramelo I, et al. Cofilin-1 is a mechanosensitive regulator of transcription. Front Cell Dev Biol. (2020) 8:678. doi: 10.3389/fcell.2020.00678
29. Qi H, Liu Y, Wu L, Ni S, Sun J, Xue J, et al. MicroRNA-16, via FGF2 regulation of the ERK/MAPK pathway, is involved in the magnesium-promoted osteogenic differentiation of mesenchymal stem cells. Oxid Med Cell Longev. (2020) 2020:3894926. doi: 10.1155/2020/3894926
30. Yoon DS, Choi Y, Lee JW. Cellular localization of NRF2 determines the self-renewal and osteogenic differentiation potential of human MSCs via the P53-SIRT1 axis. Cell Death Dis. (2016) 7:e2093. doi: 10.1038/cddis.2016.3
31. Gharibi B, Ghuman MS, Hughes FJ. Akt- and Erk-mediated regulation of proliferation and differentiation during PDGFRβ-induced MSC self-renewal. J Cell Mol Med. (2012) 16:2789–801. doi: 10.1111/j.1582-4934.2012.01602.x
32. Hader T, Muller S, Aguilera M, Eulenberg KG, Steuernagel A, Ciossek T, et al. Control of triglyceride storage by a WD40/TPR-domain protein. EMBO Rep. (2003) 4:511–6. doi: 10.1038/sj.embor.embor837
33. Lai CQ, Parnell LD, Arnett DK, Garcia-Bailo B, Tsai MY, Kabagambe EK, et al. WDTC1, the ortholog of drosophila adipose gene, associates with human obesity, modulated by MUFA intake. Obesity. (2009) 17:593–600. doi: 10.1038/oby.2008.561
34. Groh BS, Yan F, Smith MD, Yu Y, Chen X, Xiong Y. The antiobesity factor WDTC1 suppresses adipogenesis via the CRL4WDTC1 E3 ligase. EMBO Rep. (2016) 17:638–47. doi: 10.15252/embr.201540500
35. Liang G, Gao W, Liang A, Ye W, Peng Y, Zhang L, et al. Normal leptin expression, lower adipogenic ability, decreased leptin receptor and hyposensitivity to leptin in adolescent idiopathic scoliosis. PLoS ONE. (2012) 7:e36648. doi: 10.1371/journal.pone.0036648
36. Mosser DD, Caron AW, Bourget L, Denis-Larose C, Massie B. Role of the human heat shock protein hsp70 in protection against stress-induced apoptosis. Mol Cell Biol. (1997) 17:5317–27. doi: 10.1128/MCB.17.9.5317
37. Chen J, Shi ZD, Ji X, Morales J, Zhang J, Kaur N, et al. Enhanced osteogenesis of human mesenchymal stem cells by periodic heat shock in self-assembling peptide hydrogel. Tissue Eng Part A. (2013) 19:716–28. doi: 10.1089/ten.tea.2012.0070
38. Yadav R, Srivastava P. Clustering, Pathway enrichment, and protein-protein interaction analysis of gene expression in neurodevelopmental disorders. Adv Pharmacol Sci. (2018) 2018:3632159. doi: 10.1155/2018/3632159
39. Murakami J, Ishii M, Suehiro F, Ishihata K, Nakamura N, Nishimura M. Vascular endothelial growth factor-C induces osteogenic differentiation of human mesenchymal stem cells through the ERK and RUNX2 pathway. Biochem Biophys Res Commun. (2017) 484:710–8. doi: 10.1016/j.bbrc.2017.02.001
40. Jung Y, Shiozawa Y, Wang J, Wang J, Wang Z, Pedersen EA, et al. Expression of PGK1 by prostate cancer cells induces bone formation. Mol Cancer Res. (2009) 7:1595–604. doi: 10.1158/1541-7786.MCR-09-0072
41. Li H, Li T, Wang S, Wei J, Fan J, Li J, et al. miR-17-5p and miR-106a are involved in the balance between osteogenic and adipogenic differentiation of adipose-derived mesenchymal stem cells. Stem Cell Res. (2013) 10:313–24. doi: 10.1016/j.scr.2012.11.007
42. Fang T, Wu Q, Zhou L, Mu S, Fu Q. miR-106b-5p and miR-17-5p suppress osteogenic differentiation by targeting Smad5 and inhibit bone formation. Exp Cell Res. (2016) 347:74–82. doi: 10.1016/j.yexcr.2016.07.010
43. Sun Y, Xiong Y, Yan C, Chen L, Chen D, Mi B, et al. Downregulation of microRNA-16-5p accelerates fracture healing by promoting proliferation and inhibiting apoptosis of osteoblasts in patients with traumatic brain injury. Am J Transl Res. (2019) 11:4746–60.
44. Xu L, Li X, Liu Y, Kong Q, Long D, Li S. miR-93-5P suppresses osteogenic differentiation of mouse C3H10T1/2 cells by targeting Smad5. Zhongguo Xiu Fu Chong Jian Wai Ke Za Zhi. (2015) 29:1288–94.
45. Zhang Y, Wei QS, Ding WB, Zhang LL, Wang HC, Zhu YJ, et al. Increased microRNA-93-5p inhibits osteogenic differentiation by targeting bone morphogenetic protein-2. PLoS ONE. (2017) 12:e0182678. doi: 10.1371/journal.pone.0182678
46. Feng X, Lin T, Liu X, Yang C, Yang S, Fu D. Long non-coding RNA BDNF-AS modulates osteogenic differentiation of bone marrow-derived mesenchymal stem cells. Mol Cell Biochem. (2018) 445:59–65. doi: 10.1007/s11010-017-3251-2
47. Wu H, Cao F, Zhou W, Wang G, Liu G, Xia T, et al. Long non-coding RNA FAM83H-AS1 modulates the SpA-inhibited osteogenic differentiation in human bone mesenchymal stem cells. Mol Cell Biol. (2019) 40:e00362–19. doi: 10.1128/MCB.00362-19
Keywords: adolescent idiopathic scoliosis, bioinformatics, Competing endogenous RNA (CeRNA), hub genes, lncRNAs, miRNAs
Citation: Li H-M, Liu Y, Ding J-Y, Zhang R, Liu X-Y and Shen C-L (2020) In silico Analysis Excavates A Novel Competing Endogenous RNA Subnetwork in Adolescent Idiopathic Scoliosis. Front. Med. 7:583243. doi: 10.3389/fmed.2020.583243
Received: 14 July 2020; Accepted: 08 October 2020;
Published: 28 October 2020.
Edited by:
Giovanni Tarantino, University of Naples Federico II, ItalyReviewed by:
Petra Hruba, Institute for Clinical and Experimental Medicine (IKEM), CzechiaAntonio Mora, Guangzhou Medical University, China
Copyright © 2020 Li, Liu, Ding, Zhang, Liu and Shen. This is an open-access article distributed under the terms of the Creative Commons Attribution License (CC BY). The use, distribution or reproduction in other forums is permitted, provided the original author(s) and the copyright owner(s) are credited and that the original publication in this journal is cited, in accordance with accepted academic practice. No use, distribution or reproduction is permitted which does not comply with these terms.
*Correspondence: Cai-Liang Shen, shencailiang1616@163.com; orcid.org/0000-0002-9835-6384; Xiao-Ying Liu, liuxiaoying@ahmu.edu.cn
†These authors have contributed equally to this work and share first authorship