- 1Department of Biomedical Engineering, Yonsei University, Wonju, South Korea
- 2Department of Dermatology and Cutaneous Biology Research Institute, Yonsei University College of Medicine, Seoul, South Korea
Skin cancer, previously known to be a common disease in Western countries, is becoming more common in Asian countries. Skin cancer differs from other carcinomas in that it is visible to our eyes. Although skin biopsy is essential for the diagnosis of skin cancer, decisions regarding whether or not to conduct a biopsy are made by an experienced dermatologist. From this perspective, it is easy to obtain and store photos using a smartphone, and artificial intelligence technologies developed to analyze these photos can represent a useful tool to complement the dermatologist's knowledge. In addition, the universal use of dermoscopy, which allows for non-invasive inspection of the upper dermal level of skin lesions with a usual 10-fold magnification, adds to the image storage and analysis techniques, foreshadowing breakthroughs in skin cancer diagnosis. Current problems include the inaccuracy of the available technology and resulting legal liabilities. This paper presents a comprehensive review of the clinical applications of artificial intelligence and a discussion on how it can be implemented in the field of cutaneous oncology.
Introduction
The increasing incidence of skin cancer is a global trend. Skin cancer, which was previously known to be a common disease in Western countries, is occurring more frequently in South Korea. According to the Korean Statistical Information Service1, the number of patients with non-melanoma skin cancer in 2015 was 4,804 (9.4 people per 100,000), an increase over the 1,960 in 2005 and 3,270 in 2010. The increase in incidence rate is thought to be due to the aging population, the increased popularity of outdoor activities, increased ultraviolet exposure, improved access to medical services, and increased awareness of skin cancer among patients (1).
Skin biopsy and histopathologic evaluation are essential in confirming skin cancer. However, it is impossible to confirm all pigmented lesions by biopsy due to pain and scar development. Therefore, it is first necessary to establish whether or not a biopsy is required through a visual inspection performed by an experienced dermatologist. Furthermore, dermatologist needs a device that can detect changes over time in skin lesions and record the lesions in detail so that wrong-site surgery does not occur (2, 3).
With the development of imaging technologies, methods and devices for recording and analyzing what doctors see have progressed rapidly. Universally, dermoscopic imaging irradiates light onto the upper dermal layer, to observe and record more detailed pigment changes. In recent years, development of high-resolution non-invasive diagnostic devices (e.g., confocal microscopy, multiphoton microscopy, etc.) that can detect cellular levels of the skin lesions without biopsy has also been enriched (4–6). In addition, diagnoses of such skin images using artificial intelligence (AI) have been shown to outperform the average diagnosis performances of doctors. These developments are expected to have a significant impact on the diagnosis of skin cancer, the accurate recording of changes in suspicious lesions, and the effectiveness of follow-up skin cancer surgery. For user convenience, applications suitable for general smartphones have become available; however, these are not sufficiently supported by scientific evidence.
In this review, we introduce the basic concepts and clinical applications of AI via a literature review and discuss how these can be implemented in the field of dermatological oncology.
Basic Concepts of Artificial Intelligence
AI is a field of computer science that solves problems by imitating human intelligence, these problems typically require the recognition of patterns in various data. Conventional machine learning refers to machine learning methods that do not involve deep learning; these methods extract features such as those relating to colors, textures, and edges. In conventional machine learning, precise engineering knowledge and extensive experience are required to design feature extractors capable of extracting suitable features. Using these features, conventional machine learning can derive various results and identify correlations.
Deep learning uses deep neural networks to learn features, which are obtained by designing simple but non-linear modules for each layer. Using deep neural networks, very complex functions can be learned. For example, in the field of computer vision, a deep neural network's first layer typically learns the presence of edges at particular orientations and locations within the image. Larger combinations of such edges are identified in the next layer. As the layers become deeper, they learn larger and more specific features (7).
Figure 1 shows the relationship between AI, machine learning, and deep learning. Deep learning falls within the category of machine learning, which falls within the category of AI. In this figure, the examples for conventional machine learning and deep learning are classifications of acral lentiginous melanoma (ALM) and benign nevus (BN) in dermoscopy images. Conventional machine learning extracts specific features from dermoscopy images; for example, the gray-level co-occurrence matrix (GLCM) is used to extract texture features (8). The conventional machine learning method then trains classifiers, using the extracted features to classify ALM and BN. However, deep learning methods learn by extracting various features through deep neural networks. The main difference between conventional machine learning and deep learning is that deep learning extracts various features per layer, without human intervention (9).
We divided the cutaneous oncology publications into those evaluating malignant skin cancers and non-melanoma skin cancers. In addition, each publication was divided into machine learning (excluding deep learning), deep learning, and hybrid methods (a combination of machine learning and deep learning) (Figure 2).
In terms of machine learning methods, most publications use a feature extractor to extract a feature from an image, they then train the classifier model using these features (e.g., malignant melanoma (MM) vs. BN). Recently, deep convolution neural network (DCNN) have been implemented in many medical-imaging studies (10–12). DCNN use convolution operations to compensate for the problems that arise through neglecting the correlations and pixel localities of multi-layer perceptron (MLP). Thus, deep learning can be used to train a robust classifier model with a variety of data. Figure 3 shows an example of a DCNN for classifying ALMs and BNs in dermoscopic images. The DCNN feature extractor repeatedly applies convolution and max-pooling (to obtain the largest activation for each region) operations to the layer input. This process generates a feature map. The feature map is inputted to a classifier via global average pooling for each channel. The classifier finally determines probabilities for ALM and BN. The result is then compared with the actual label, and the parameters are updated via backpropagation. However, DCNN operations require highly powerful graphics processing units to manage the complex computations and large datasets involved. Although DCNN learning capacities can be limited by insufficient medical-image data, it is possible to fine-tune state-of-art deep learning models that show high performance in ImageNet large-scale visual recognition challenge (ILSVRC), making them suitable for medical purposes (13). In the hybrid method, an ensemble classifier is designed by combining a conventional machine learning method and a deep learning method. For example, after extracting the features of an image using a conventional machine learning method, these extracted features are used as inputs for a DCNN. Another example is that of training a support vector machine (SVM) using a feature map obtained through a DCNN (14). One publication showed that hybrid models outperform both deep learning and conventional machine learning models (15), another publication highlighted the limitations of deep learning and stated a need for hybrid models to overcome these limitations (16). Thus, these two methods can be used effectively to create more accurate models.
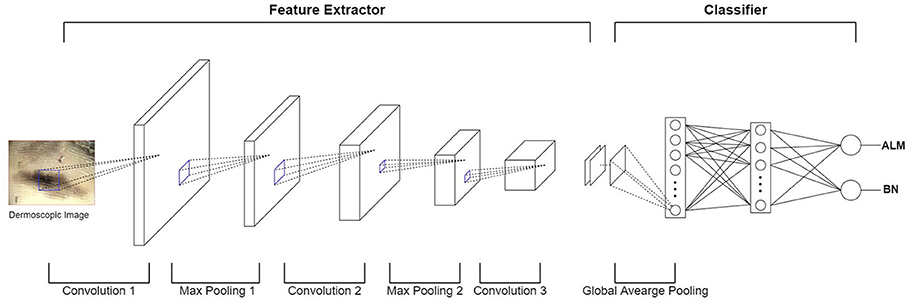
Figure 3. Example of DCNN for classifying ALM and BN in dermoscopic images. In the feature extractor, each layer performs a convolution operation on the input data and then performs a max-pooling operation, thereby reducing the image size and increasing the number of channels. The feature extractor generates a feature map by repeating this process for each layer. After the global average pooling operation, the feature map is used as the input of the classifier layer (fully-connected layer). Finally, the output of the fully-connected layer appears as a probability of ALM or BN.
Every year, the number of articles describing AI implementations in the field of cutaneous oncology increases. By observing the trends of the discipline, it can be seen that studies using conventional machine learning have been decreasing in popularity since 2015 (five publications in 2015, three publications from 2016 to 2017, and one publication after 2018); however, the number of studies conducted using deep learning methods has increased significantly since 2015 (zero publications in 2015, seven publications from 2016 to 2017, and nine publications after 2018). These tendencies are a result of the increasing availability of big data and powerful GPUs. Since 2015, state-of-art deep learning models such as ResNet have also been studied [ResNet competed for the first time in the 2015 ILSVRC (17); it surpassed the human error rate of 5%, achieving an error rate of 3.6%].
Application of Artificial Intelligence in the Diagnosis of Malignant Skin Cancers
Melanoma
A total of 18 publications were identified, six of these described the use of conventional machine learning, nine publications showed the use of deep learning, and two publications presented the use of hybrid models. Among the total 18 publications, 14 used dermoscopic images as the dataset, and the remainder used unspecified or clinical images; nine used more than 500 datasets, and the remainder used <500 datasets. Moreover, in five of the publications, other skin lesion data such as seborrheic keratosis (SK) and basal cell carcinoma (BCC) were used alongside malignant melanomas and nevus. Seven publications presented the area under the curve (AUC) as a performance indicator of the model and the remainder presented accuracy (Acc), sensitivity (Sen), and specificity (Spe) (Tables 1–3).
Deep Learning
Among the deep learning algorithms discussed in the literature, five were fine-tuned using pre-trained models. The remainder were fully trained with new models. In four publications, preprocessing was performed prior to model training. In addition, two publications performed lesion segmentation and classification or segmentation of dermoscopic features. To measure the model performance, one publication (Tschandl, Kittler et al.) compared the results of final-year medical students with those of the model; two publications (Yang et al. and Lee et al.) used the results of dermatological experts as the comparison. From these, Lee et al. showed that experienced dermatologists and inexperienced dermatologists improved their decision making with the help of deep learning models. One publication (Premaladha and Ravichandran) compared the conventional machine learning method 'Hybrid Adaboost-SVM' and a deep learning-based neural network on the same dataset; they showed that the deep learning-based neural network delivered superior performance. Moreover, one publication (Cui et al.) demonstrated that when more data was used, the results of deep learning outperformed conventional machine learning methods.
Conventional Machine Learning
From the conventional machine learning publications, four of the five publications performed feature extraction and then created a classifier. Two of these publications used SVM for the classifier, one used multivariable linear regression, and one used a layered model. In three publications, artifact removal or lesion segmentation were performed prior to feature extraction. On the other hand, one publication (Marchetti, Codella et al.) presented a new model using a fusion method, developed by 25 teams participating in International Symposium on Biomedical Imaging (ISBI) 2016.
Hybrid (Deep Learning + Machine Learning)
In the publications using hybrid methods, one publication (Jafari, Nasr-Esfahani et al.) preprocessed the input images, extracted the patches, and performed segmentation using a convolutional neural network (CNN). In one publication (Xie, Fan et al.), segmentation was performed after preprocessing, using a neural network called self-generating neural network (SGNN); they then presented an ensemble network by designing a feature extractor and classifier. Furthermore, in one publication (Sabbaghi et al.), a deep auto-encoder combined with bag of features (BoF) outperformed the model using a BoF or deep auto-encoder alone.
Non-melanoma Skin Cancer: BCC, Squamous Cell Carcinoma (SCC)
We identified seven deep learning publications, three machine learning publications and three hybrid publications on non-melanoma skin cancer. Several publications discussed MM; however, all of them discussed BCC and three publications discussed SCC, thus we classified the publications into these categories. The results are organized in Tables 4–6.
The results of all publications were presented using an accuracy indicator, and some of these publications using a variety of indicators, such as specificity, sensitivity, precision, and F1 score. The datasets used in each publication were different, making it impossible to compare them directly.
Deep Learning
Rezvantalab et al. compared the abilities of deep learning against the performances of highly trained dermatologists. This publication presented outcomes from various deep learning models. In the BCC classification, the highest AUC of the publication was reported as 99.3%, using DenseNet 201. When compared against dermatologists (AUC 88.82%), the results of deep learning were found superior.
Five publications used datasets of dermoscopic images. One used full-field optical coherence tomography (FFOCT) images, and Jordan Yap et al. used different forms of data including metadata, macroscopic images, and dermoscopic images. Next, they trained a deep learning model using fusion techniques, in which image feature vectors were concatenated with the metadata feature vectors. Two publications by Zhang et al. written in 2017 and 2018, showed interesting results; the 2018 publication improved the previous year's algorithm for utilizing medical information. Their results showed an average improvement of 0.7% over those of the previous year.
Conventional Machine Learning
We identified four publications that used only machine learning techniques. Three publications used dermoscopic images and one used polarization-sensitive optical coherence tomography (PS-OCT) images. Each author used different methods and features.
Marvdashti et al. performed feature extraction and classification using multiple machine learning methods [SVM, k-nearest neighbor (KNN)]. Kharazmi et al. segmented vascular structures using independent component analysis (ICA) and k-means clustering, then classified them using a random forest classifier. Kefel et al. introduced automatically generated borders using geodesic active contour (GAC) and Otsu's threshold for the detection of pink blush features, known as a common feature of BCCs. Subsequently, they classified using logistic regression, based on features such as smoothness and brightness.
Hybrid (Deep Learning + Machine Learning)
Three publications implementing hybrids were identified. Each publication used a different dataset. One publication used optical coherence tomography (OCT) images and another used dermoscopic images. Unusually, the third publication used data downloaded from the Internet, not directly taken.
Annan Li et al. used deep learning for feature extraction, then classified images using the principal component analysis (PCA) and SVM machine learning techniques. They compared deep learning models and assessed the differences in dimensions of the PCA features. Sarkar et al. applied Gaussian blurring to denoise the images and then used the contrast-limited adaptive histogram equalization (CLAHE) algorithm to enhance them. Unlike previous publications, deep learning was used for classification.
Implementation in Smartphones
With the spread of smartphones, the mobile application market has expanded rapidly. Applications can be used in various fields, particularly in the field of dermatology through the use of smartphone cameras. In particular, due to the ubiquity of smartphones, easily accessible mobile apps can make it more efficient to detect and monitor skin cancers during the early stages of development. In addition, with the recent development of smartphone processors and cameras, machine learning techniques can be applied, and skin cancer diagnoses can be conducted through smartphones. Table 7 shows that a lot of research and development on smartphone implementation is carried out. AI technology relevant to skin cancer diagnosis is anticipated to eventually be implemented in smartphones, enabling the reduction of unnecessary hospital visits. Many types of mobile health application are already available.
Types and Accuracies of Diagnostic Applications Using a Smartphone
According to a recent review (53, 54), numerous applications have already been released, seven of which use image analysis algorithms. Four of the seven applications are not supported by scientific evidence, and these four have been deleted from the app store since the review was conducted; the other three apps are still available. Table 7 provides a summary of the apps. SkinScan, SkinVision, and SpotMole are currently available. SkinVision uses machine learning algorithms and SkinScan and SpotMole use the ABCDE rule (that is asymmetry, border irregularity, color that is not uniform, diameter >6 mm, and evolving size, shape or color). Only one application employs a machine learning technique. The sensitivity and specificity of these applications are shown in the table.
Most diagnosis applications are not accurate (55). Furthermore, only a few inform users using image analysis and machine learning. Most apps are not supported by scientific evidence and require further research.
Problems and Possible Solutions
Inaccuracies in medical applications can result in problems of legal liability. In addition, the transmission of patient information may correspond to telemedicine practices, for which there are certain legal restrictions; these include information protection regulations to prevent third parties accessing data during the transmission process. Even if the accuracy is improved, the advertisements embedded in the application suggest that the technology could be used for commercial advertisements; for example, to attract patients.
To solve this problem, a supervisory institution in which doctors participate is required, along with a connection to remote medical care services. The United States has been steadily attempting to promote telemedicine in its early stages, to address the issue of access to healthcare. Since the establishment of the American Telemedicine Association (ATA)—a telemedicine research institute—in 1993, legislation, including the Federal Telemedicine Act, has been established. It has been applied to more than 50 detailed medical subjects, including heart diseases, and has been successfully implemented in rural areas, prisons, homes, and schools (56).
To obtain good results, it is necessary to focus on securing high-quality data, to form a consensus between the patient and the doctor, and to actively participate in development.
In summary, the evidence for the diagnostic accuracy of smartphone applications is still lacking because few mHealth apps offer services. In addition, because the rate of service or algorithm change is faster than the peer-review publishing process, it is difficult to compare different apps accurately.
Risks of Smartphone Applications
Smartphone applications pose some risk to users, especially if the algorithm returns negative results and delays the detection and treatment of undiagnosed skin cancer. It is very difficult to study false-negative rates because there is no histological evidence. Users may not be able to assess all skin lesions, especially if they are located in areas difficult to reach or to see. Given the generally low specificity of current applications, there would be a few false positives. This would put unnecessary stress on the user and result in unnecessary visits to the dermatologist. Furthermore, through limited trust and awareness, the user may not follow the advice provided by the smartphone application.
Chao et al. described the ethical and privacy issues of smartphone applications (57). Whilst applications have the potential to improve the provisions of medical services, there are important ethical concerns regarding patient confidentiality, informed consent, transparency in data ownership, and protection of data privacy. Many apps require users to agree to their data policies; however, the methods in which patient data are externally mined, used, and shared are often not transparent. Therefore, if a patient's data are stored on a cloud server or released to a third party for data analysis, assessing liability in the event of a breach of personal information is a challenge. In addition, it is unclear how the responsibilities for medical malpractice will be determined if the patient is injured as a result of inaccurate information.
Conclusion
In this review, we analyzed a total of 35 publications. Studies on skin lesions were divided into those assessing malignant melanomas and non-melanoma skin cancers. In addition, studies involving clinical data and OCT images were used alongside those involving the dermoscopic images widely used in dermatology. Because the considered datasets differed between the publications, it was impossible to determine how best to perform the analysis. However, as seen in the publication by Cui et al. deep learning methods obtain better results than conventional machine learning methods if the dataset is large. Also, certain publications have reported comparable or superior results to dermatologist. In particular, recent publications have reported that dermatologists have improved diagnostic accuracy with the help of deep learning (26, 58). Therefore, in the future, computer-aided diagnostics in dermatology will show greater reliance on deep learning methods.
For the convenience of users, the use of a smartphone is necessary. However, an accuracy limitation occurs when applied to smartphones. This problem is due to the limitations of hardware, which used conventional machine learning techniques such as SVM rather than deep learning. However, MobileNet has recently made it possible to use deep learning methods in IoT devices, including smartphones (59). This enables deep learning to be applied to IoT devices for faster performances than large networks, which will lead to more active research into skin lesion detection using applications.
Application inaccuracies can lead to legal problems. To solve this problem, doctors and patients must participate together in the development stage, and an institution for managing and supervising this process is also required.
Author Contributions
BO and SY: contributed conception and design of the study, wrote sections of the manuscript, and contributed to manuscript revision. YC and HA: collected data and wrote the first draft of the manuscript. All authors read and approved the submitted version.
Funding
This research was supported in part by the Global Frontier Program, through the Global Frontier Hybrid Interface Materials (GFHIM) of the National Research Foundation of Korea (NRF) funded by the Ministry of Science, ICT & Future Planning (2013M3A6B1078872), in part by the National Research Foundation of Korea (NRF) grant funded by the Korea government (MSIT) (No. 2019R1F1A1058971) and in part by Leaders in Industry-University Cooperation + Project, supported by the Ministry of Education and National Research Foundation of Korea.
Conflict of Interest
The authors declare that the research was conducted in the absence of any commercial or financial relationships that could be construed as a potential conflict of interest.
Abbreviations
ACC, accuracy; AI, artificial intelligence; ALM, acral lentiginous melanoma; AUC, area under the curve; BCC, basal cell carcinoma; BN, benign nevus; BoF, bag of features; CLAHE, contrast-limited adaptive histogram equalization; CNN, convolutional neural network; DCNN, deep convolution neural network; DI, dice score; DLNN, deep learning-based neural network; FFOCT, full-field optical coherence tomography; GAC, geodesic active contour; GLCM, gray-level co-occurrence matrix; ICA, independent component analysis; ILSVRC, ImageNet large-scale visual recognition challenge; ISBI, International Symposium on Biomedical Imaging; ISIC, International Skin Imaging Collaboration; JA, Jaccard index; KNN, k-nearest neighbor; LFN, lesion feature network; LICU, lesion index calculation unit; LIN, lesion indexing network; MLP, multi-layer perceptron; MM, malignant melanoma; NPV, negative predictive values; OCT, optical coherence tomography; PCA, principal component analysis; PPV, positive predictive values; PS-OCT, polarization-sensitive optical coherence tomography; RF, random forest; SCC, squamous cell carcinoma; SGNN, self-generating neural network; SK, seborrheic keratosis; Sen, sensitivity; Spe, specificity; SVM, support vector machine.
Footnotes
References
1. Ha SM, Ko DY, Jeon SY, Kim KH, Song HK. A clinical and statistical study of cutaneous malignant tumors in Busan City and the eastern Gyeongnam Province over 15 years (1996-2010). Korean J Dermatol. (2013) 51:167–72.
2. Alam M, Lee A, Ibrahimi OA, Kim N, Bordeaux J, Chen K, et al. A multistep approach to improving biopsy site identification in dermatology: physician, staff, and patient roles based on a Delphi consensus. JAMA Dermatol. (2014) 150:550–8. doi: 10.1001/jamadermatol.2013.9804
3. St. John J, Walker J, Goldberg D, Maloney EM. Avoiding medical errors in cutaneous site identification: a best practices review. Dermatol Surg. (2016) 42:477–84. doi: 10.1097/DSS.0000000000000683
4. Cinotti E, Couzan C, Perrot J, Habougit C, Labeille B, Cambazard F, et al. In vivo confocal microscopic substrate of grey colour in melanosis. J Eur Acad Dermatol Venereol. (2015) 29:2458–62. doi: 10.1111/jdv.13394
5. Dubois A, Levecq O, Azimani H, Siret D, Barut A, Suppa M, et al. Line-field confocal optical coherence tomography for high-resolution noninvasive imaging of skin tumors. J Biomed Opt. (2018) 23:106007. doi: 10.1117/1.JBO.23.10.106007
6. Oh B-H, Kim KH, Chung K-Y. Skin Imaging Using Ultrasound Imaging, Optical Coherence Tomography, Confocal Microscopy, and Two-Photon Microscopy in Cutaneous Oncology. Front Med. (2019) 6:274. doi: 10.3389/fmed.2019.00274
8. Mohanaiah P, Sathyanarayana P, GuruKumar L. Image texture feature extraction using GLCM approach. Int J Sci Res Publications. (2013) 3:1–5.
9. Krizhevsky A, Sutskever I, Hinton EG. Imagenet classification with deep convolutional neural networks. In: Pereira F, Burges CJC, Bottou L, Weinberger K, editors. Advances in Neural Information Processing Systems (NIPS), Vol. 25. Lake Tahoe, NV (2012). p. 1097–105. doi: 10.1145/3065386
10. Chilamkurthy S, Ghosh R, Tanamala S, Biviji M, Campeau NG, Venugopal VK, et al. Deep learning algorithms for detection of critical findings in head CT scans: a retrospective study. Lancet. (2018) 392:2388–96. doi: 10.1016/S0140-6736(18)31645-3
11. Li X, Zhang S, Zhang Q, Wei X, Pan Y, Zhao J, et al. Diagnosis of thyroid cancer using deep convolutional neural network models applied to sonographic images: a retrospective, multicohort, diagnostic study. Lancet Oncol. (2019) 20:193–201. doi: 10.1016/S1470-2045(18)30762-9
12. Bejnordi BE, Veta M, Van Diest PJ, Van Ginneken B, Karssemeijer N, Litjens G, et al. Diagnostic assessment of deep learning algorithms for detection of lymph node metastases in women with breast cancer. JAMA. (2017) 318:2199–210. doi: 10.1001/jama.2017.14585
13. Tajbakhsh N, Shin JY, Gurudu SR, Hurst RT, Kendall CB, Gotway MB, et al. Convolutional neural networks for medical image analysis: full training or fine tuning? IEEE Trans Med Imaging. (2016) 35:1299–312. doi: 10.1109/TMI.2016.2535302
14. Tang Y. Deep learning using linear support vector machines. arXiv [Preprint]. arXiv:1306.0239. (2013).
15. Szarvas M, Yoshizawa A, Yamamoto M, Ogata J. Pedestrian detection with convolutional neural networks. In: IEEE Proceedings. Intelligent Vehicles Symposium 2005. Las Vegas, NV: IEEE (2005). doi: 10.1109/IVS.2005.1505106
16. O'Mahony N, Campbell S, Carvalho A, Harapanahalli S, Hernandez GV, Krpalkova L, et al. Deep Learning vs. Traditional Computer Vision. In: O'Mahony N, editor. Cham: Springer International Publishing (2020). p. 128–144.
17. He K, Zhang X, Ren S, Sun J. Deep residual learning for image recognition. In: Proceedings of the IEEE Conference on Computer Vision and Pattern Recognition. Las Vegas, NV: IEEE (2016).
18. Li Y, Shen L. Skin lesion analysis towards melanoma detection using deep learning network. Sensors. (2018) 18:556. doi: 10.3390/s18020556
19. Pour MP, Seker H, Shao L. Automated lesion segmentation and dermoscopic feature segmentation for skin cancer analysis. In: 2017 39th Annual International Conference of the IEEE Engineering in Medicine and Biology Society (EMBC). Seogwipo: IEEE (2017). doi: 10.1109/EMBC.2017.8036906
20. Yu C, Yang S, Kim W, Jung J, Chung K-Y, Lee SW, et al. Acral melanoma detection using a convolutional neural network for dermoscopy images. PLoS ONE. (2018) 13:e0193321. doi: 10.1371/journal.pone.0196621
21. Nasr-Esfahani E, Samavi S, Karimi N, Soroushmehr SMR, Jafari MH, Ward K, et al. Melanoma detection by analysis of clinical images using convolutional neural network. In: 2016 38th Annual International Conference of the IEEE Engineering in Medicine and Biology Society (EMBC). Orlando, FL: IEEE (2016). doi: 10.1109/EMBC.2016.7590963
22. Premaladha J, Ravichandran K. Novel approaches for diagnosing melanoma skin lesions through supervised and deep learning algorithms. J Med Syst. (2016) 40:96. doi: 10.1007/s10916-016-0460-2
23. Matsunaga K, Hamada A, Minagawa A, Koga H. Image classification of melanoma, nevus and seborrheic keratosis by deep neural network ensemble. arXiv [Preprint]. arXiv:1703.03108. (2017).
24. Tschandl P, Kittler H, Argenziano G. A pretrained neural network shows similar diagnostic accuracy to medical students in categorizing dermatoscopic images after comparable training conditions. Br J Dermatol. (2017) 177:867–9. doi: 10.1111/bjd.15695
25. Esteva A, Kuprel B, Novoa RA, Ko J, Swetter SM, Blau HM, et al. dermatologist-level classification of skin cancer with deep neural networks. Nature. (2017) 542:115–18. doi: 10.1038/nature21056
26. Lee S, Chu Y, Yoo S, Choi S, Choe S, Koh S, et al. Augmented decision making for acral lentiginous melanoma detection using deep convolutional neural networks. J Eur Acad Dermatol Venereol. (2020) doi: 10.1111/jdv.16185. [Epub ahead of print].
27. Cui X, Wei R, Gong L, Qi R, Zhao Z, Chen H, et al. Assessing the effectiveness of artificial intelligence methods for melanoma: a retrospective review. J Am Acad Dermatol. (2019) 81:1176–80. doi: 10.1016/j.jaad.2019.06.042
28. Sabouri P, GholamHosseini H, Larsson T, Collins J. A cascade classifier for diagnosis of melanoma in clinical images. In: 2014 36th Annual International Conference of the IEEE Engineering in Medicine and Biology Society. Chicago, IL: IEEE. (2014). doi: 10.1109/EMBC.2014.6945177
29. Kaur R, Albano PP, Cole JG, Hagerty J, LeAnder RW, Moss RH, et al. Real-time supervised detection of pink areas in dermoscopic images of melanoma: importance of color shades, texture and location. Skin Res Technol. (2015) 21:466–73. doi: 10.1111/srt.12216
30. Shimizu K, Iyatomi H, Celebi ME, Norton K-A, Tanaka M. Four-class classification of skin lesions with task decomposition strategy. IEEE Trans Biomed Eng. (2014) 62:274–83. doi: 10.1109/TBME.2014.2348323
31. Abedini M, von Cavallar S, Chakravorty R, Davis M, Garnavi R. A cloud-based infrastructure for feedback-driven training and image recognition. Stud Health Technol Inform. (2015) 216:691–5. doi: 10.3233/978-1-61499-564-7-691
32. Marchetti MA, Codella NC, Dusza SW, Gutman DA, Helba B, Kalloo A, et al. Results of the 2016 international skin imaging collaboration international symposium on biomedical imaging challenge: comparison of the accuracy of computer algorithms to dermatologists for the diagnosis of melanoma from dermoscopic images. J Am Acad Dermatol. (2018) 78:270–77.e1. doi: 10.1016/j.jaad.2017.08.016
33. Jafari MH, Nasr-Esfahani E, Karimi N, Soroushmehr SR, Samavi S, Najarian K. Extraction of skin lesions from non-dermoscopic images for surgical excision of melanoma. Int J Comput Assist Radiol Surg. (2017) 12:1021–30. doi: 10.1007/s11548-017-1567-8
34. Xie F, Fan H, Li Y, Jiang Z, Meng R, Bovik A. Melanoma classification on dermoscopy images using a neural network ensemble model. IEEE Trans Med Imaging. (2016) 36:849–58. doi: 10.1109/TMI.2016.2633551
35. Sabbaghi S, Aldeen M, Garnavi R. A deep bag-of-features model for the classification of melanomas in dermoscopy images. In: 2016 38th Annual International Conference of the IEEE Engineering in Medicine and Biology Society (EMBC). Orlando, FL: IEEE (2016). doi: 10.1109/EMBC.2016.7590962
36. Rezvantalab A, Safigholi H, Karimijeshni S. Dermatologist level dermoscopy skin cancer classification using different deep learning convolutional neural networks algorithms. arXiv [Preprint]. arXiv:1810.10348. (2018).
37. Zhang X, Wang S, Liu J, Tao C. Computer-aided diagnosis of four common cutaneous diseases using deep learning algorithm. In: 2017 IEEE International Conference on Bioinformatics and Biomedicine (BIBM). Kansas, MO: IEEE. (2017). doi: 10.1109/BIBM.2017.8217850
38. Vander Putten E, Kambod A, Kambod M. Deep residual neural networks for automated basal cell carcinoma detection. In: 2018 IEEE EMBS International Conference on Biomedical & Health Informatics (BHI). Las Vegas, NV: IEEE. (2018). doi: 10.1109/BHI.2018.8333437
39. Mandache D, Dalimier E, Durkin J, Boceara C, Olivo-Marin J-C, Meas-Yedid V. Basal cell carcinoma detection in full field OCT images using convolutional neural networks. In: 2018 IEEE 15th International Symposium on Biomedical Imaging (ISBI 2018). Washington, DC: IEEE (2018) doi: 10.1109/ISBI.2018.8363689
40. Zhang X, Wang S, Liu J, Tao C. Towards improving diagnosis of skin diseases by combining deep neural network and human knowledge. BMC Med Inform Decis Making. (2018) 18:59. doi: 10.1186/s12911-018-0631-9
41. Yap J, Yolland W, Tschandl P. Multimodal skin lesion classification using deep learning. Exp Dermatol. (2018) 27:1261–67. doi: 10.1111/exd.13777
42. Marvdashti T, Duan L, Aasi SZ, Tang JY, Ellerbee Bowden AK. Classification of basal cell carcinoma in human skin using machine learning and quantitative features captured by polarization sensitive optical coherence tomography. Biomed Opt Express. (2016) 7:3721–35. doi: 10.1364/BOE.7.003721
43. Kharazmi P, AlJasser MI, Lui H, Wang ZJ, Lee KT. Automated detection and segmentation of vascular structures of skin lesions seen in Dermoscopy, with an application to basal cell carcinoma classification. IEEE J Biomed Health Inform. (2016) 21:1675–84. doi: 10.1109/JBHI.2016.2637342
44. Kefel S, Pelin Kefel S, LeAnder R, Kaur R, Kasmi R, Mishra N, et al. Adaptable texture-based segmentation by variance and intensity for automatic detection of semitranslucent and pink blush areas in basal cell carcinoma. Skin Res Technol. (2016) 22:412–22. doi: 10.1111/srt.12281
45. Annan L, Jun C, Ai Ping Y, Srivastava R, Wong DW, Hong Liang T, et al. Automated basal cell carcinoma detection in high-definition optical coherence tomography. Conf Proc IEEE Eng Med Biol Soc. (2016) 2016:2885–88. doi: 10.1109/EMBC.2016.7591332
46. Sarkar R, Chatterjee CC, Hazra A. A novel approach for automatic diagnosis of skin carcinoma from dermoscopic images using parallel deep residual networks. In: International Conference on Advances in Computing and Data Sciences. (Springer) (2019). doi: 10.1007/978-981-13-9939-8_8
47. Dorj U-O, Lee K-K, Choi J-Y, Lee M. The skin cancer classification using deep convolutional neural network. Multimedia Tools Appl. (2018) 77:9909–24. doi: 10.1007/s11042-018-5714-1
48. Chen RH, Snorrason M, Enger SM, Mostafa E, Ko JM, Aoki V, et al. Validation of a skin-lesion image-matching algorithm based on computer vision technology. Telemed e-Health. (2016) 22:45–50. doi: 10.1089/tmj.2014.0249
49. Udrea A, Lupu C. Real-time acquisition of quality verified nonstandardized color images for skin lesions risk assessment—A preliminary study. In: 2014 18th International Conference on System Theory, Control and Computing (ICSTCC). Sinaia: IEEE (2014) doi: 10.1109/ICSTCC.2014.6982415
50. Maier T, Kulichova D, Schotten K, Astrid R, Ruzicka T, Berking C, et al. Accuracy of a smartphone application using fractal image analysis of pigmented moles compared to clinical diagnosis and histological result. J Eur Acad Dermatol Venereol. (2015) 29:663–7. doi: 10.1111/jdv.12648
51. Thissen M, Udrea A, Hacking M, von Braunmuehl T, Ruzicka T. mHealth app for risk assessment of pigmented and nonpigmented skin lesions—a study on sensitivity and specificity in detecting malignancy. Telemed e-Health. (2017) 23:948–54. doi: 10.1089/tmj.2016.0259
52. Ngoo A, Finnane A, McMeniman E, Tan JM, Janda M, Soyer PH. Efficacy of smartphone applications in high-risk pigmented lesions. Austr J Dermatol. (2018) 59:e175–e182. doi: 10.1111/ajd.12599
53. Kassianos A, Emery J, Murchie P, Walter MF. Smartphone applications for melanoma detection by community, patient and generalist clinician users: a review. Br J Dermatol. (2015) 172:1507–18. doi: 10.1111/bjd.13665
54. de Carvalho TM, Noels E, Wakkee M, Udrea A, Nijsten T. Development of smartphone apps for skin cancer risk assessment: progress and promise. JMIR Dermatol. (2019) 2:e13376. doi: 10.2196/13376
55. Wolf JA, Moreau JF, Akilov O, Patton T, English JC, Ho J, et al. Diagnostic inaccuracy of smartphone applications for melanoma detection. JAMA Dermatol. (2013) 149:422–6. doi: 10.1001/jamadermatol.2013.2382
56. Jo JW, Jeong DS, Kim YC. Analysis of trends in dermatology mobile applications in korea. Korean J Dermatol. (2020) 58:7–13.
57. Chao E, Meenan CK, Ferris KL. Smartphone-based applications for skin monitoring and melanoma detection. Dermatol Clin. (2017) 35:551–7. doi: 10.1016/j.det.2017.06.014
58. Han SS, Park I, Chang SE, Lim W, Kim MS, Park GH, et al. Augmented Intelligence Dermatology. Deep neural networks empower medical professionals in diagnosing skin cancer and predicting treatment options for 134 skin disorders. J Invest Dermatol. (2020). doi: 10.1016/j.jid.2020.01.019. [Epub ahead of print].
Keywords: artificial intellegence, cutaneous oncology, skin cancer, machine learning, deep learning
Citation: Chu YS, An HG, Oh BH and Yang S (2020) Artificial Intelligence in Cutaneous Oncology. Front. Med. 7:318. doi: 10.3389/fmed.2020.00318
Received: 27 March 2020; Accepted: 01 June 2020;
Published: 10 July 2020.
Edited by:
Ivan V. Litvinov, McGill University, CanadaCopyright © 2020 Chu, An, Oh and Yang. This is an open-access article distributed under the terms of the Creative Commons Attribution License (CC BY). The use, distribution or reproduction in other forums is permitted, provided the original author(s) and the copyright owner(s) are credited and that the original publication in this journal is cited, in accordance with accepted academic practice. No use, distribution or reproduction is permitted which does not comply with these terms.
*Correspondence: Byung Ho Oh, obh505@gmail.com; Sejung Yang, syang@yonsei.ac.kr